Diffusers documentation
T2I-Adapter
T2I-Adapter
T2I-Adapter is an adapter that enables controllable generation like ControlNet. A T2I-Adapter works by learning a mapping between a control signal (for example, a depth map) and a pretrained model’s internal knowledge. The adapter is plugged in to the base model to provide extra guidance based on the control signal during generation.
Load a T2I-Adapter conditioned on a specific control, such as canny edge, and pass it to the pipeline in from_pretrained().
import torch
from diffusers import T2IAdapter, StableDiffusionXLAdapterPipeline, AutoencoderKL
t2i_adapter = T2IAdapter.from_pretrained(
"TencentARC/t2i-adapter-canny-sdxl-1.0",
torch_dtype=torch.float16,
)
Generate a canny image with opencv-python.
import cv2
import numpy as np
from PIL import Image
from diffusers.utils import load_image
original_image = load_image(
"https://huggingface.co/datasets/huggingface/documentation-images/resolve/main/diffusers/non-enhanced-prompt.png"
)
image = np.array(original_image)
low_threshold = 100
high_threshold = 200
image = cv2.Canny(image, low_threshold, high_threshold)
image = image[:, :, None]
image = np.concatenate([image, image, image], axis=2)
canny_image = Image.fromarray(image)
Pass the canny image to the pipeline to generate an image.
vae = AutoencoderKL.from_pretrained("madebyollin/sdxl-vae-fp16-fix", torch_dtype=torch.float16)
pipeline = StableDiffusionXLAdapterPipeline.from_pretrained(
"stabilityai/stable-diffusion-xl-base-1.0",
adapter=t2i_adapter,
vae=vae,
torch_dtype=torch.float16,
).to("cuda")
prompt = """
A photorealistic overhead image of a cat reclining sideways in a flamingo pool floatie holding a margarita.
The cat is floating leisurely in the pool and completely relaxed and happy.
"""
pipeline(
prompt,
image=canny_image,
num_inference_steps=100,
guidance_scale=10,
).images[0]
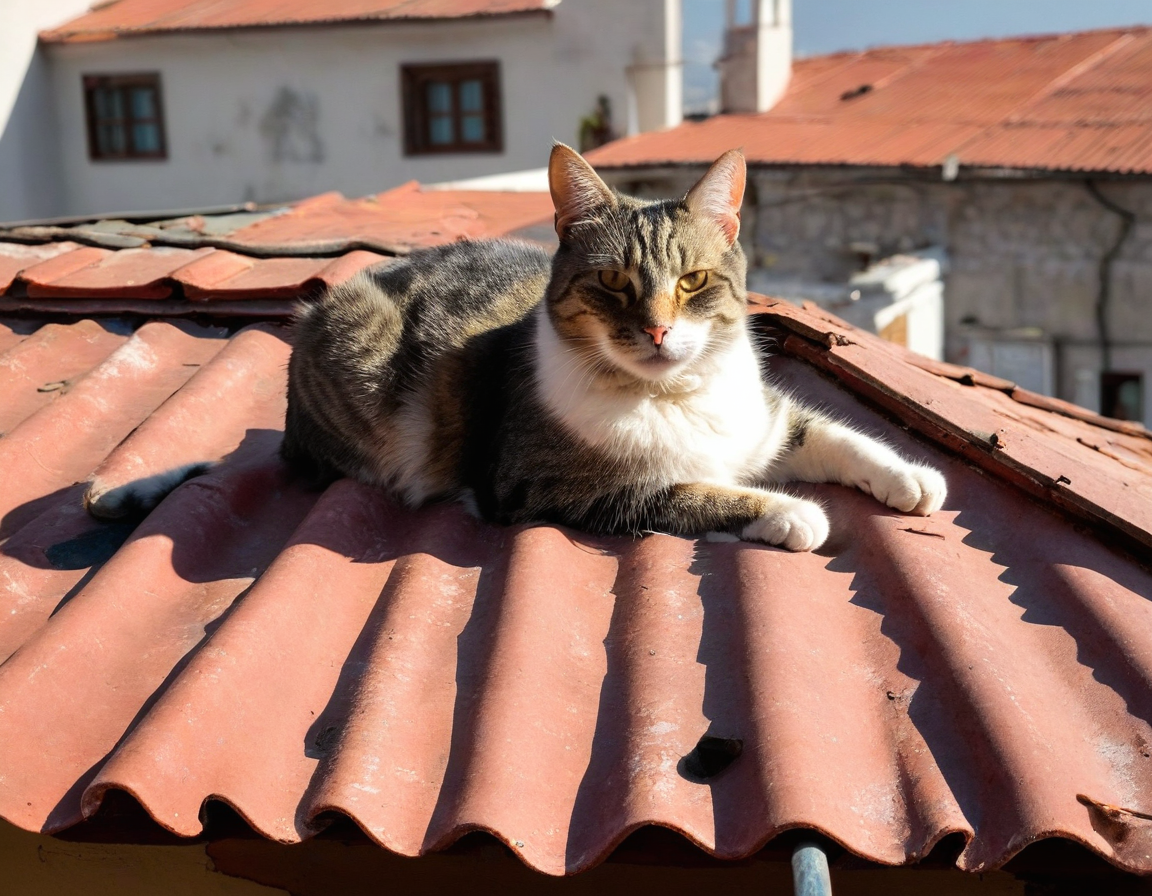


MultiAdapter
You can compose multiple controls, such as canny image and a depth map, with the MultiAdapter
class.
The example below composes a canny image and depth map.
Load the control images and T2I-Adapters as a list.
import torch
from diffusers.utils import load_image
from diffusers import StableDiffusionXLAdapterPipeline, AutoencoderKL, MultiAdapter, T2IAdapter
canny_image = load_image(
"https://huggingface.co/datasets/huggingface/documentation-images/resolve/main/diffusers/canny-cat.png"
)
depth_image = load_image(
"https://huggingface.co/datasets/huggingface/documentation-images/resolve/main/diffusers/sdxl_depth_image.png"
)
controls = [canny_image, depth_image]
prompt = ["""
a relaxed rabbit sitting on a striped towel next to a pool with a tropical drink nearby,
bright sunny day, vacation scene, 35mm photograph, film, professional, 4k, highly detailed
"""]
adapters = MultiAdapter(
[
T2IAdapter.from_pretrained("TencentARC/t2i-adapter-canny-sdxl-1.0", torch_dtype=torch.float16),
T2IAdapter.from_pretrained("TencentARC/t2i-adapter-depth-midas-sdxl-1.0", torch_dtype=torch.float16),
]
)
Pass the adapters, prompt, and control images to StableDiffusionXLAdapterPipeline. Use the adapter_conditioning_scale
parameter to determine how much weight to assign to each control.
vae = AutoencoderKL.from_pretrained("madebyollin/sdxl-vae-fp16-fix", torch_dtype=torch.float16)
pipeline = StableDiffusionXLAdapterPipeline.from_pretrained(
"stabilityai/stable-diffusion-xl-base-1.0",
torch_dtype=torch.float16,
vae=vae,
adapter=adapters,
).to("cuda")
pipeline(
prompt,
image=controls,
height=1024,
width=1024,
adapter_conditioning_scale=[0.7, 0.7]
).images[0]


