Please open a proper issue in the corresponding Github repo (is it a huggingface_hub issue? a gradio one? etc.) with more details and especially a reproducible example of the issue you are having. If it is a HTTP request issue, try to isolate it first. (the more details you can provider, the better it is for the maintainers)
Lucain Pouget PRO
AI & ML interests
Recent Activity
Organizations
Wauplin's activity
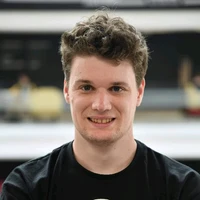
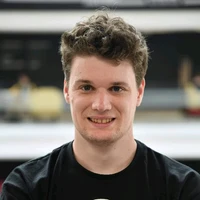
My spaces use models that, for some reason, state that they are unavailable to be used by any of the inference providers, despite them working fine two months ago
The Huggingface's serverless Inference API wasn't a production-ready service. It was only meant to easily experiment and prototype ML apps. We started rolling out inference providers to tackle this topic and make things more future-proof. Regarding the specific problem you have, it's hard to help without knowing the specific models you were using back then. The most likely is that these models have been removed from HF Inference API infra as we are now focusing on making fewer but high-impact models available.
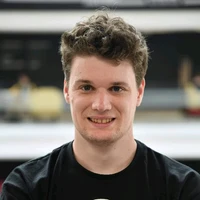
Full release notes: https://github.com/huggingface/huggingface_hub/releases/tag/v0.30.0.
🚀 Ready. Xet. Go!
Xet is a groundbreaking new protocol for storing large objects in Git repositories, designed to replace Git LFS. Unlike LFS, which deduplicates files, Xet operates at the chunk level—making it a game-changer for AI builders collaborating on massive models and datasets. Our Python integration is powered by [xet-core](https://github.com/huggingface/xet-core), a Rust-based package that handles all the low-level details.
You can start using Xet today by installing the optional dependency:
pip install -U huggingface_hub[hf_xet]
With that, you can seamlessly download files from Xet-enabled repositories! And don’t worry—everything remains fully backward-compatible if you’re not ready to upgrade yet.
Blog post: https://huggingface.co/blog/xet-on-the-hub
Docs: https://huggingface.co/docs/hub/en/storage-backends#xet
⚡ Inference Providers
- We’re thrilled to introduce Cerebras and Cohere as official inference providers! This expansion strengthens the Hub as the go-to entry point for running inference on open-weight models.
- Novita is now our 3rd provider to support text-to-video task after Fal.ai and Replicate.
- Centralized billing: manage your budget and set team-wide spending limits for Inference Providers! Available to all Enterprise Hub organizations.
from huggingface_hub import InferenceClient
client = InferenceClient(provider="fal-ai", bill_to="my-cool-company")
image = client.text_to_image(
"A majestic lion in a fantasy forest",
model="black-forest-labs/FLUX.1-schnell",
)
image.save("lion.png")
- No more timeouts when generating videos, thanks to async calls. Available right now for Fal.ai, expecting more providers to leverage the same structure very soon!
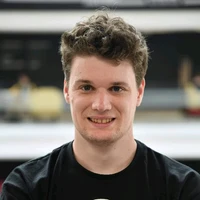
you can do that by converting your local image to base64 and sending it to the API.
recently I made some changes to my library "loadimg" that allows you to make converting images to base64 a breeze.
🔗 https://github.com/not-lain/loadimg
API request example 🛠️:
from loadimg import load_img
from huggingface_hub import InferenceClient
# or load a local image
my_b64_img = load_img(imgPath_url_pillow_or_numpy ,output_type="base64" )
client = InferenceClient(api_key="hf_xxxxxxxxxxxxxxxxxxxxxxxxxxxxxxxxx")
messages = [
{
"role": "user",
"content": [
{
"type": "text",
"text": "Describe this image in one sentence."
},
{
"type": "image_url",
"image_url": {
"url": my_b64_img # base64 allows using images without uploading them to the web
}
}
]
}
]
stream = client.chat.completions.create(
model="meta-llama/Llama-3.2-11B-Vision-Instruct",
messages=messages,
max_tokens=500,
stream=True
)
for chunk in stream:
print(chunk.choices[0].delta.content, end="")
Let's go! 🔥🔥🔥
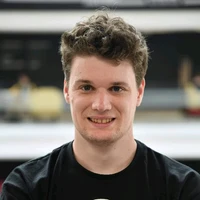
Six months after joining Hugging Face the Xet team is kicking off the first migrations from LFS to our storage for a number of repositories on the Hub.
More on the nitty gritty details behind the migration soon, but here are the big takeaways:
🤖 We've successfully completed the first migrations from LFS -> Xet to test the infrastructure and prepare for a wider release
✅ No action on your part needed - you can work with a Xet-backed repo like any other repo on the Hub (for now - major improvements on their way!)
👀 Keep an eye out for the Xet logo to see if a repo you know is on our infra! See the screenshots below to spot the difference 👇
⏩ ⏩ ⏩ Blazing uploads and downloads coming soon. W’re gearing up for a full integration with the Hub's Python library that will make building on the Hub faster than ever - special thanks to @celinah and @Wauplin for their assistance.
🎉 Want Early Access? If you’re curious and want to test it out the bleeding edge that will power the development experience on the Hub, we’d love to partner with you. Let me know!
This is the culmination of a lot of effort from the entire team. Big round of applause to @sirahd @brianronan @jgodlewski @hoytak @seanses @assafvayner @znation @saba9 @rajatarya @port8080 @yuchenglow
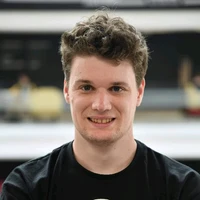
TL;DR:
- public storage is free, and (unless blatant abuse) unlimited. We do ask that you consider upgrading to PRO and/or Enterprise Hub if possible
- private storage is paid above a significant free tier (1TB if you have a paid account, 100GB otherwise)
docs: https://huggingface.co/docs/hub/storage-limits
We optimize our infrastructure continuously to scale our storage for the coming years of growth in Machine learning, to the benefit of the community 🔥
cc: @reach-vb @pierric @victor and the HF team
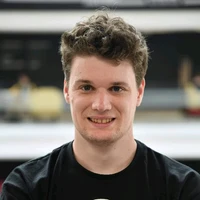
Here's help: We're launching our Year in Review on what actually matters, starting today!
Fresh content dropping daily until year end. Come along for the ride - first piece out now with @clem 's predictions for 2025.
Think of it as your end-of-year AI chocolate calendar.
Kudos to @BrigitteTousi @clefourrier @Wauplin @thomwolf for making it happen. We teamed up with aiworld.eu for awesome visualizations to make this digestible—it's a charm to work with their team.
Check it out: huggingface/open-source-ai-year-in-review-2024
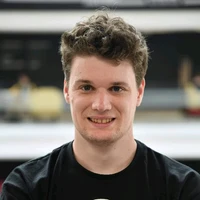
1,000 spots available first-come first serve with some surprises during the stream!
You can register and add to your calendar here: https://streamyard.com/watch/JS2jHsUP3NDM
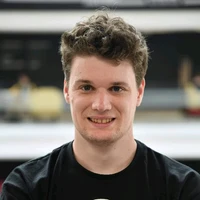
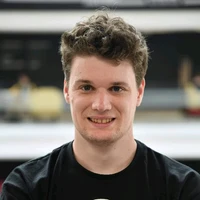
We've just released 𝚑𝚞𝚐𝚐𝚒𝚗𝚐𝚏𝚊𝚌𝚎_𝚑𝚞𝚋 v0.25.0 and it's packed with powerful new features and improvements!
✨ 𝗧𝗼𝗽 𝗛𝗶𝗴𝗵𝗹𝗶𝗴𝗵𝘁𝘀:
• 📁 𝗨𝗽𝗹𝗼𝗮𝗱 𝗹𝗮𝗿𝗴𝗲 𝗳𝗼𝗹𝗱𝗲𝗿𝘀 with ease using
huggingface-cli upload-large-folder
. Designed for your massive models and datasets. Much recommended if you struggle to upload your Llama 70B fine-tuned model 🤡• 🔎 𝗦𝗲𝗮𝗿𝗰𝗵 𝗔𝗣𝗜: new search filters (gated status, inference status) and fetch trending score.
• ⚡𝗜𝗻𝗳𝗲𝗿𝗲𝗻𝗰𝗲𝗖𝗹𝗶𝗲𝗻𝘁: major improvements simplifying chat completions and handling async tasks better.
We’ve also introduced tons of bug fixes and quality-of-life improvements - thanks to the awesome contributions from our community! 💪
💡 Check out the release notes: Wauplin/huggingface_hub#8
Want to try it out? Install the release with:
pip install huggingface_hub==0.25.0
Thanks for the ping @clem !
This documentation is more recent regarding HfApi
(the Python client). You have methods like model_info
and list_models
to get details about models (and similarly with datasets and Spaces). In addition to the package reference, we also have a small guide on how to use it.
Otherwise, if you are interested in the HTTP endpoint to build your requests yourself, here is the API reference.