Model Card for thibaud-perrin/hibo-mistral-7b-fc-v1.3
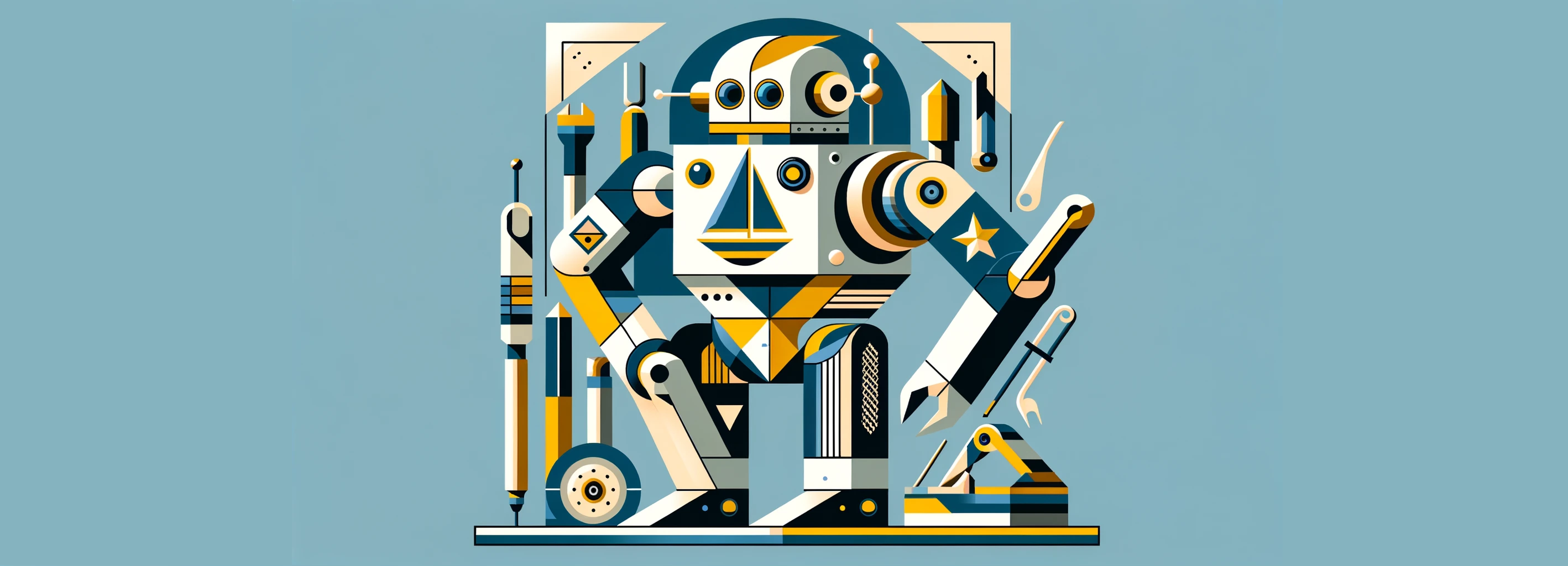
This model is a fine-tuned version of the mistralai/Mistral-7B-v0.1
for the purpose of instruction following and function calling tasks. It is designed to understand and generate responses based on given instructions or function calls.
Model Details
Model Description
Developed by Thibaud Perrin, this model is fine-tuned specifically for the task of interpreting instructions and generating appropriate responses or function calls in English. It leverages the power of the Mistral-7B model, adapting its capabilities to more targeted use cases.
- Developed by: Thibaud Perrin
- Model type: CAUSAL_LM
- Language(s) (NLP): English
- License: MIT
- Finetuned from model: Mistral-7B
Uses
This model is intended for developers, researchers, and hobbyists looking for a pre-trained model capable of understanding and responding to instructions or executing function calls within a given context.
Direct Use
The model can be directly used via the Hugging Face Transformers library for generating text based on prompts related to instructions or function calls.
Out-of-Scope Use
This model is not intended for high-stakes decisions or scenarios where misunderstanding instructions could lead to significant consequences.
Bias, Risks, and Limitations
As with any language model, there's a risk of generating biased or inappropriate content. Users should be cautious and evaluate the model's outputs within their specific context.
Recommendations
Users should monitor the model's outputs and apply additional filtering or moderation as needed to ensure the generated content is appropriate for their use case.
How to Get Started with the Model
from transformers import AutoModelForCausalLM, AutoTokenizer, TextStreamer
model_identifier = "thibaud-perrin/hibo-mistral-7b-fc-v1.3"
model = AutoModelForCausalLM.from_pretrained(
model_identifier,
low_cpu_mem_usage=True,
return_dict=True,
torch_dtype=torch.bfloat16,
device_map={"": 0},
)
tokenizer = AutoTokenizer.from_pretrained(model_identifier)
device = 'cuda:0'
# device = 'cpu'
model.config.use_cache = True
model.eval()
model.to(device)
def stream(user_prompt):
system_prompt = """You are a helpful assistant with access to the following functions. Use them if required -
{
"name": "get_stock_price",
"description": "Get the current stock price of a company",
"parameters": {
"type": "object",
"properties": {
"company_name": {
"type": "string",
"description": "The name of the company"
},
"exchange": {
"type": "string",
"description": "The stock exchange where the company is listed"
}
},
"required": [
"company_name",
"exchange"
]
}
}
"""
messages = [
{"role": "system", "content": system_prompt},
{"role": "user", "content": user_prompt.strip()}
]
transformed_data = tokenizer.apply_chat_template(messages, tokenize=False, add_generation_prompt=False)
eos_token_id = tokenizer.eos_token_id
inputs = tokenizer([transformed_data], return_tensors="pt", add_special_tokens=True).to(device)
streamer = TextStreamer(tokenizer, skip_prompt=True, skip_special_tokens=False)
_ = model.generate(**inputs, streamer=streamer, max_new_tokens=512, eos_token_id=tokenizer.eos_token_id, early_stopping=True)
stream("Hi, can you tell me the current stock price of Apple on NASDAQ? ")
Training Details
Training Data
The model was trained using the dataset thibaud-perrin/hibo-function-calling-v1
, which consists of various instruction-following and function-calling examples.
Summary
The fine-tuned model demonstrates a significant improvement in understanding and generating instruction-based responses compared to the base Mistral-7B model. However this model has been trained, only on the first 50_000 rows of the dataset, with one epoch.
Environmental Impact
- Hardware Type: A100 - 40GB
- Hours used: 48H
- Cloud Provider: Google Colab
- Compute Region: France
- Carbon Emitted: Estimates needed
π Citation
Please cite this dataset using the following BibTeX entry:
@misc{hibo-mistral-7b-fc-v1.3,
author = Thibaud Perrin,
title = hibo-mistral-7b-fc-v1.3: An instruct Model for Function Calling in Conversational AI,
year = 2024,
publisher = Hugging Face,
}
- Downloads last month
- 0