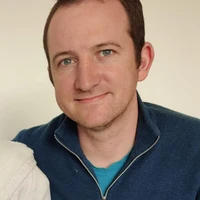
Reinforcement Learning
Reinforcement learning is the computational approach of learning from action by interacting with an environment through trial and error and receiving rewards (negative or positive) as feedback
State
Red traffic light, pedestrians are about to pass.
Action
Stop the car.
Next State
Yellow light, pedestrians have crossed.
About Reinforcement Learning
Use Cases
Gaming
Reinforcement learning is known for its application to video games. Since the games provide a safe environment for the agent to be trained in the sense that it is perfectly defined and controllable, this makes them perfect candidates for experimentation and will help a lot to learn about the capabilities and limitations of various RL algorithms.
There are many videos on the Internet where a game-playing reinforcement learning agent starts with a terrible gaming strategy due to random initialization of its settings, but over iterations, the agent gets better and better with each episode of the training. This paper mainly investigates the performance of RL in popular games such as Minecraft or Dota2. The agent's performance can exceed a human player's, although there are still some challenges mainly related to efficiency in constructing the gaming policy of the reinforcement learning agent.
Trading and Finance
Reinforcement learning is the science to train computers to make decisions and thus has a novel use in trading and finance. All time-series models are helpful in predicting prices, volume and future sales of a product or a stock. Reinforcement based automated agents can decide to sell, buy or hold a stock. It shifts the impact of AI in this field to real time decision making rather than just prediction of prices. The glossary given below will clear some parameters to as to how we can train a model to take these decisions.
Task Variants
Model Based RL
In model based reinforcement learning techniques intend to create a model of the environment, learn the state transition probabilities and the reward function, to find the optimal action. Some typical examples for model based reinforcement learning algorithms are dynamic programming, value iteration and policy iteration.
Model Free RL
In model free reinforcement learning, agent decides on optimal actions based on its experience in the environment and the reward it collects from it. This is one of the most commonly used algorithms beneficial in complex environments, where modeling of state transition probabilities and reward functions are difficult. Some of the examples of model free reinforcement learning are SARSA, Q-Learning, actor-critic and proximal policy optimization (PPO) algorithms.
Glossary
Agent: The learner and the decision maker.
Environment: The part of the world the agent interacts, comprising everything outside the agent.
Observations and states are the information our agent gets from the environment. In the case of a video game, it can be a frame (a screenshot). In the case of the trading agent, it can be the value of a certain stock.
State: Complete description of the state of the environment with no hidden information.
Observation: Partial description of the state, in a partially observed environment.
Action: The decision taken by the agent.
Reward: The numerical feedback signal that the agent receives from the environment based on the chosen action.
Return: Cumulative Reward. In the simplest case, the return is the sum of the rewards.
Episode: For some applications there is a natural notion of final time step. In this case, there is a starting point and an ending point (a terminal state). This creates an episode: a list of States, Actions, Rewards, and new States. For instance, think about Chess: an episode begins at the initial board position and ends when the game is over.
Policy: The Policy is the brain of the Agent, it’s the function that tells what action to take given the state. So it defines the agent’s behavior at a given time. Reinforcement learning methods specify how the agent’s policy is changed as a result of its experience.
Inference
Inference in reinforcement learning differs from other modalities, in which there's a model and test data. In reinforcement learning, once you have trained an agent in an environment, you try to run the trained agent for additional steps to get the average reward.
A typical training cycle consists of gathering experience from the environment, training the agent, and running the agent on a test environment to obtain average reward. Below there's a snippet on how you can interact with the environment using the gymnasium
library, train an agent using stable-baselines3
, evalute the agent on test environment and infer actions from the trained agent.
# Here we are running 20 episodes of CartPole-v1 environment, taking random actions
import gymnasium as gym
env = gym.make("CartPole-v1")
observation, info = env.reset()
for _ in range(20):
action = env.action_space.sample() # samples random action from action sample space
# the agent takes the action
observation, reward, terminated, truncated, info = env.step(action)
# if the agent reaches terminal state, we reset the environment
if terminated or truncated:
print("Environment is reset")
observation = env.reset()
env.close()
Below snippet shows how to train a PPO model on LunarLander-v2 environment using stable-baselines3
library and saving the model
from stable_baselines3 import PPO
# initialize the environment
env = gym.make("LunarLander-v2")
# initialize the model
model = PPO(policy = "MlpPolicy",
env = env,
n_steps = 1024,
batch_size = 64,
n_epochs = 4,
verbose = 1)
# train the model for 1000 time steps
model.learn(total_timesteps = 1000)
# Saving the model in desired directory
model_name = "PPO-LunarLander-v2"
model.save(model_name)
Below code shows how to evaluate an agent trained using stable-baselines3
# Loading a saved model and evaluating the model for 10 episodes
from stable_baselines3.common.evaluation import evaluate_policy
from stable_baselines3 import PPO
env = gym.make("LunarLander-v2")
# Loading the saved model
model = PPO.load("PPO-LunarLander-v2",env=env)
# Initializating the evaluation environment
eval_env = gym.make("LunarLander-v2")
# Running the trained agent on eval_env for 10 time steps and getting the mean reward
mean_reward, std_reward = evaluate_policy(model, eval_env, n_eval_episodes = 10,
deterministic=True)
print(f"mean_reward={mean_reward:.2f} +/- {std_reward}")
Below code snippet shows how to infer actions from an agent trained using stable-baselines3
from stable_baselines3.common.evaluation import evaluate_policy
from stable_baselines3 import PPO
# Loading the saved model
model = PPO.load("PPO-LunarLander-v2",env=env)
# Getting the environment from the trained agent
env = model.get_env()
obs = env.reset()
for i in range(1000):
# getting action predictions from the trained agent
action, _states = model.predict(obs, deterministic=True)
# taking the predicted action in the environment to observe next state and rewards
obs, rewards, dones, info = env.step(action)
For more information, you can check out the documentations of the respective libraries.
Gymnasium Documentation Stable Baselines Documentation
Useful Resources
Would you like to learn more about the topic? Awesome! Here you can find some curated resources that you may find helpful!
- HuggingFace Deep Reinforcement Learning Class
- Introduction to Deep Reinforcement Learning
- Stable Baselines Integration with HuggingFace
- Learn how reinforcement learning is used in conversational agents in this blog: Illustrating Reinforcement Learning from Human Feedback (RLHF)
- Reinforcement Learning from Human Feedback From Zero to ChatGPT
- Guide on Multi-Agent Competition Systems
Notebooks
- Train a Deep Reinforcement Learning lander agent to land correctly on the Moon 🌕 using Stable-Baselines3
- Introduction to Unity MLAgents
- Training Decision Transformers with 🤗 transformers
This page was made possible thanks to the efforts of Ram Ananth, Emilio Lehoucq, Sagar Mathpal and Osman Alenbey.
Compatible libraries
No example widget is defined for this task.
Note Contribute by proposing a widget for this task !
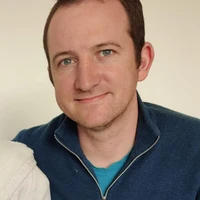
Note A Reinforcement Learning model trained on expert data from the Gym Hopper environment
Note A PPO agent playing seals/CartPole-v0 using the stable-baselines3 library and the RL Zoo.
Note A curation of widely used datasets for Data Driven Deep Reinforcement Learning (D4RL)
Note An application for a cute puppy agent learning to catch a stick.
Note An application to play Snowball Fight with a reinforcement learning agent.
- Discounted Total Reward
- Accumulated reward across all time steps discounted by a factor that ranges between 0 and 1 and determines how much the agent optimizes for future relative to immediate rewards. Measures how good is the policy ultimately found by a given algorithm considering uncertainty over the future.
- Mean Reward
- Average return obtained after running the policy for a certain number of evaluation episodes. As opposed to total reward, mean reward considers how much reward a given algorithm receives while learning.
- Level of Performance After Some Time
- Measures how good a given algorithm is after a predefined time. Some algorithms may be guaranteed to converge to optimal behavior across many time steps. However, an agent that reaches an acceptable level of optimality after a given time horizon may be preferable to one that ultimately reaches optimality but takes a long time.