Deep RL Course documentation
Introduction to Q-Learning
Introduction to Q-Learning
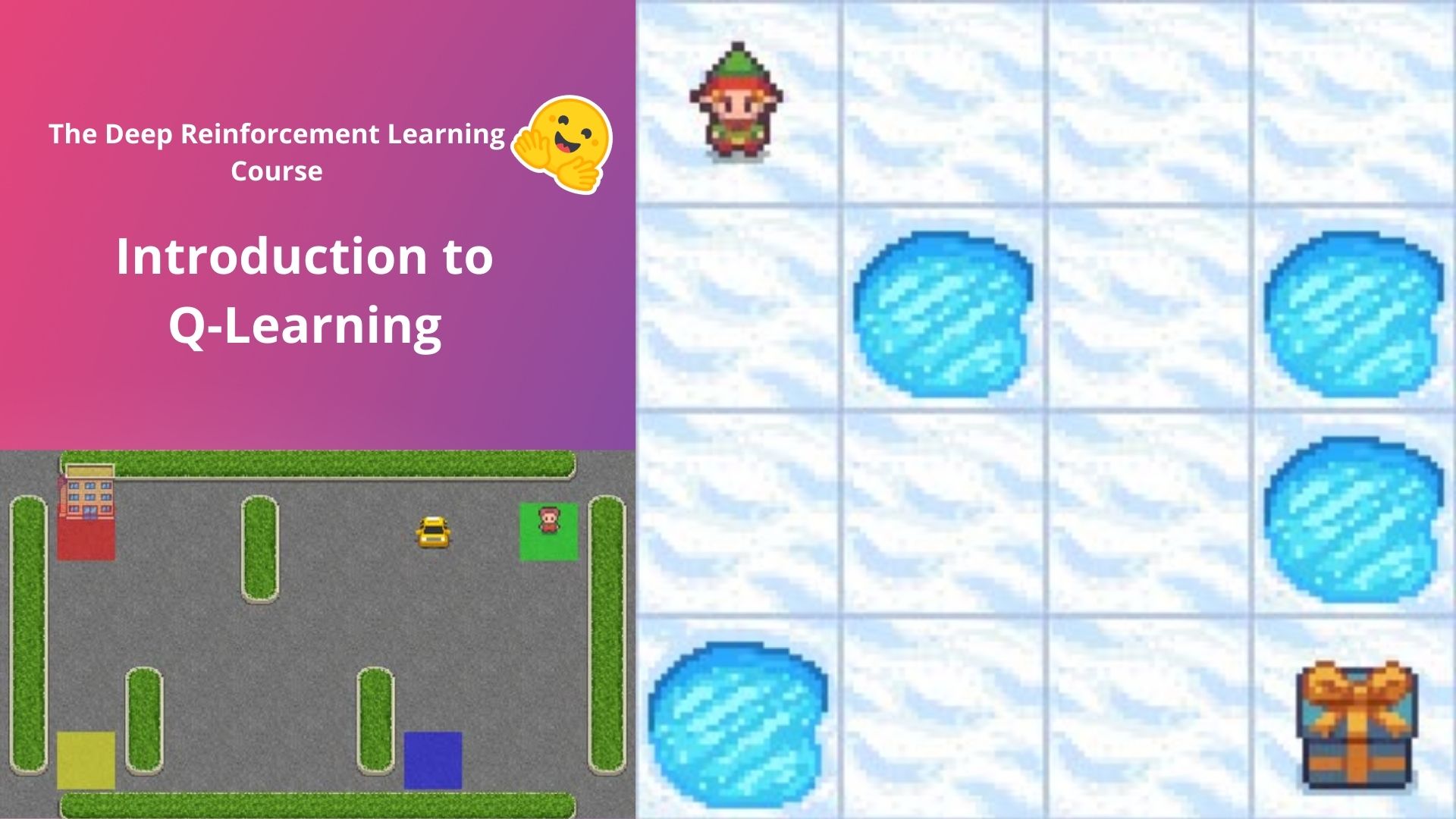
In the first unit of this class, we learned about Reinforcement Learning (RL), the RL process, and the different methods to solve an RL problem. We also trained our first agents and uploaded them to the Hugging Face Hub.
In this unit, we’re going to dive deeper into one of the Reinforcement Learning methods: value-based methods and study our first RL algorithm: Q-Learning.
We’ll also implement our first RL agent from scratch, a Q-Learning agent, and will train it in two environments:
- Frozen-Lake-v1 (non-slippery version): where our agent will need to go from the starting state (S) to the goal state (G) by walking only on frozen tiles (F) and avoiding holes (H).
- An autonomous taxi: where our agent will need to learn to navigate a city to transport its passengers from point A to point B.
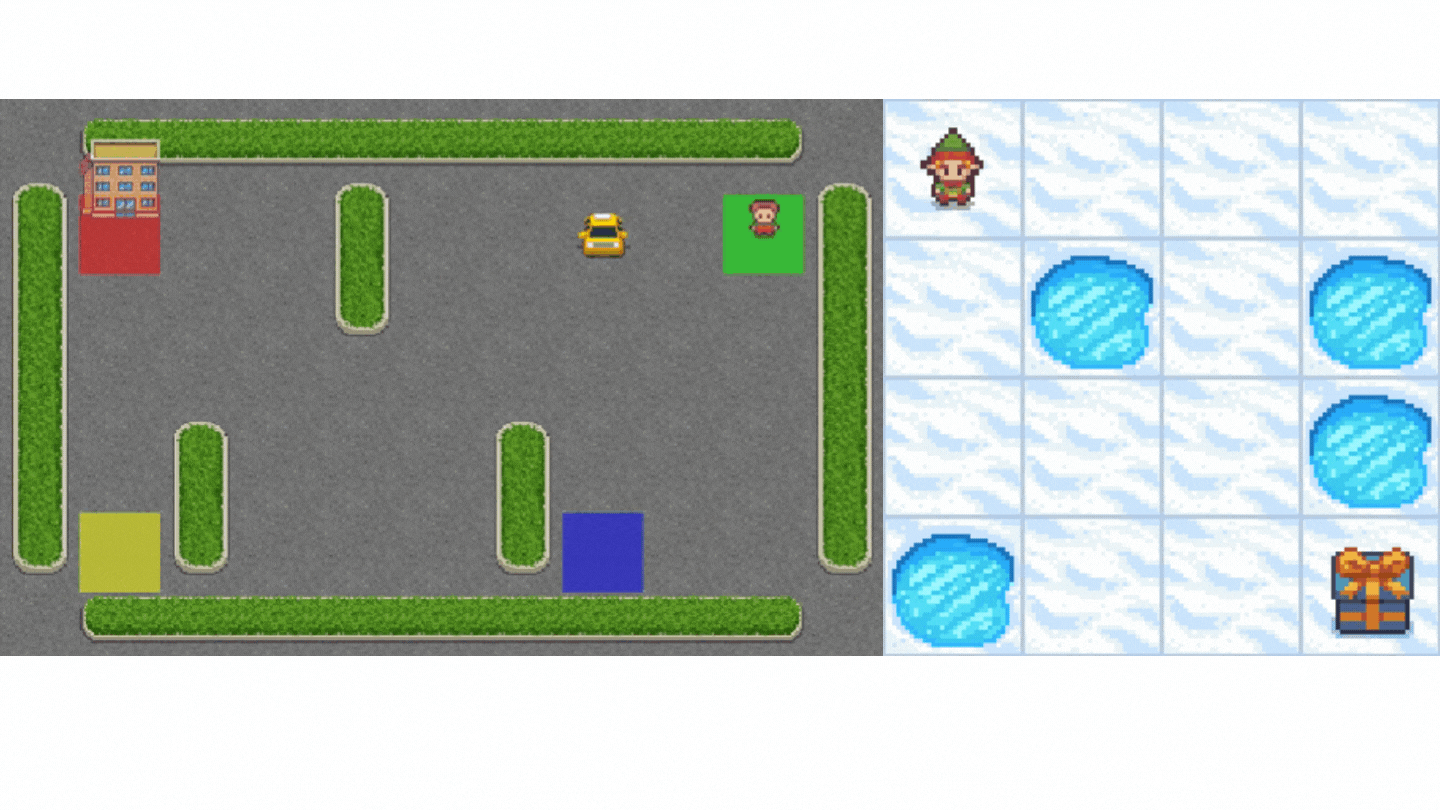
Concretely, we will:
- Learn about value-based methods.
- Learn about the differences between Monte Carlo and Temporal Difference Learning.
- Study and implement our first RL algorithm: Q-Learning.
This unit is fundamental if you want to be able to work on Deep Q-Learning: the first Deep RL algorithm that played Atari games and beat the human level on some of them (breakout, space invaders, etc).
So let’s get started! 🚀
< > Update on GitHub