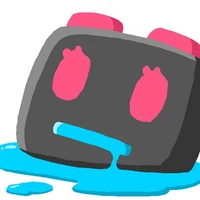
Datasets:
uid
int64 0
8.65k
| source
stringclasses 10
values | label
class label 2
classes | diacritic
stringclasses 9
values | sentence
stringlengths 3
58
| original
stringlengths 2
55
| translation
stringclasses 0
values | gloss
bool 0
classes | linguistic_phenomenon
dict |
---|---|---|---|---|---|---|---|---|
3,924 | Kuroda_1965 | 1acceptable
| g | ジョンはビルに本を買われた。 | ジョンはビルに本を買われた。 | null | null | {
"argument_structure": null,
"binding": null,
"control_raising": null,
"ellipsis": null,
"filler_gap": null,
"island_effects": null,
"morphology": null,
"nominal_structure": null,
"negative_polarity_concord_items": null,
"quantifier": null,
"verbal_agreement": null,
"simple": null
} |
3,014 | Kuno_1973 | 1acceptable
| g | ジョンは目を覚まし顔を洗った。 | ジョンは目を覚まし顔を洗った。 | null | null | {
"argument_structure": null,
"binding": null,
"control_raising": null,
"ellipsis": null,
"filler_gap": null,
"island_effects": null,
"morphology": null,
"nominal_structure": null,
"negative_polarity_concord_items": null,
"quantifier": null,
"verbal_agreement": null,
"simple": null
} |
7,921 | Tsujimura_1999 | 1acceptable
| g | 太郎が二郎に自分について話した。 | 太郎が二郎に自分について話した。 | null | null | {
"argument_structure": null,
"binding": null,
"control_raising": null,
"ellipsis": null,
"filler_gap": null,
"island_effects": null,
"morphology": null,
"nominal_structure": null,
"negative_polarity_concord_items": null,
"quantifier": null,
"verbal_agreement": null,
"simple": null
} |
1,196 | Inoue_1976 | 1acceptable
| g | ジョンの手の長さでは、誰にもひけを取らない。 | ジョンの手の長さでは、誰にもひけを取らない。 | null | null | {
"argument_structure": null,
"binding": null,
"control_raising": null,
"ellipsis": null,
"filler_gap": null,
"island_effects": null,
"morphology": null,
"nominal_structure": null,
"negative_polarity_concord_items": null,
"quantifier": null,
"verbal_agreement": null,
"simple": null
} |
7,525 | Shibatani_1990 | 1acceptable
| g | 太郎あh非常に速く走る。 | 非常に速く走る。 | null | null | {
"argument_structure": null,
"binding": null,
"control_raising": null,
"ellipsis": null,
"filler_gap": null,
"island_effects": null,
"morphology": null,
"nominal_structure": null,
"negative_polarity_concord_items": null,
"quantifier": null,
"verbal_agreement": null,
"simple": null
} |
8,030 | Tsujimura_1999 | 1acceptable
| g | 日本語学科の新設が学長によって発表された。 | 日本語学科の新設が学長によって発表された。 | null | null | {
"argument_structure": null,
"binding": null,
"control_raising": null,
"ellipsis": null,
"filler_gap": null,
"island_effects": null,
"morphology": null,
"nominal_structure": null,
"negative_polarity_concord_items": null,
"quantifier": null,
"verbal_agreement": null,
"simple": null
} |
5,935 | Shibatani_1976 | 1acceptable
| g | 親の私に無断で大事な娘に会われては困る。 | 親の私に無断で大事な娘に会われては困る。 | null | null | {
"argument_structure": null,
"binding": null,
"control_raising": null,
"ellipsis": null,
"filler_gap": null,
"island_effects": null,
"morphology": null,
"nominal_structure": null,
"negative_polarity_concord_items": null,
"quantifier": null,
"verbal_agreement": null,
"simple": null
} |
3,436 | Kuno_1973 | 1acceptable
| g | ジョンはメアリーに行かせる。 | ジョンはメアリーに行かせる。 | null | null | {
"argument_structure": null,
"binding": null,
"control_raising": null,
"ellipsis": null,
"filler_gap": null,
"island_effects": null,
"morphology": null,
"nominal_structure": null,
"negative_polarity_concord_items": null,
"quantifier": null,
"verbal_agreement": null,
"simple": null
} |
4,105 | Kuroda_1992 | 1acceptable
| g | 統辞構造論もジョンさえ読んだ。 | 統辞構造論もジョンさえ読んだ。 | null | null | {
"argument_structure": null,
"binding": null,
"control_raising": null,
"ellipsis": null,
"filler_gap": null,
"island_effects": null,
"morphology": null,
"nominal_structure": null,
"negative_polarity_concord_items": null,
"quantifier": null,
"verbal_agreement": null,
"simple": null
} |
6,113 | Shibatani_1976 | 1acceptable
| g | 太郎は花子を自分の車から降ろした。 | 太郎は花子を自分の車から降ろした。 | null | null | {
"argument_structure": null,
"binding": null,
"control_raising": null,
"ellipsis": null,
"filler_gap": null,
"island_effects": null,
"morphology": null,
"nominal_structure": null,
"negative_polarity_concord_items": null,
"quantifier": null,
"verbal_agreement": null,
"simple": null
} |
2,730 | Kuno_1973 | 1acceptable
| g | ここは寒すぎる。 | ここは寒すぎる。 | null | null | {
"argument_structure": null,
"binding": null,
"control_raising": null,
"ellipsis": null,
"filler_gap": null,
"island_effects": null,
"morphology": null,
"nominal_structure": null,
"negative_polarity_concord_items": null,
"quantifier": null,
"verbal_agreement": null,
"simple": null
} |
3,754 | Kuroda_1965 | 1acceptable
| g | ジョンが本を買ったかビルが本を買ったかです。 | ジョンが本を買ったかビルが本を買ったかです。 | null | null | {
"argument_structure": null,
"binding": null,
"control_raising": null,
"ellipsis": null,
"filler_gap": null,
"island_effects": null,
"morphology": null,
"nominal_structure": null,
"negative_polarity_concord_items": null,
"quantifier": null,
"verbal_agreement": null,
"simple": null
} |
6,437 | Shibatani_1976 | 1acceptable
| g | 父が死んだという知らせを聞いたのはその時だった。 | 父が死んだという知らせを聞いたのはその時だった。 | null | null | {
"argument_structure": null,
"binding": null,
"control_raising": null,
"ellipsis": null,
"filler_gap": null,
"island_effects": null,
"morphology": null,
"nominal_structure": null,
"negative_polarity_concord_items": null,
"quantifier": null,
"verbal_agreement": null,
"simple": null
} |
4,735 | Miyagawa_and_Saito | 1acceptable
| g | 美智子は何をしていますか? | 美智子は何をしていますか? | null | null | {
"argument_structure": null,
"binding": null,
"control_raising": null,
"ellipsis": null,
"filler_gap": null,
"island_effects": null,
"morphology": null,
"nominal_structure": null,
"negative_polarity_concord_items": null,
"quantifier": null,
"verbal_agreement": null,
"simple": null
} |
6,289 | Shibatani_1976 | 1acceptable
| g | 私はボスに辞職することを頼んだ。 | 私はボスに辞職することを頼んだ。 | null | null | {
"argument_structure": null,
"binding": null,
"control_raising": null,
"ellipsis": null,
"filler_gap": null,
"island_effects": null,
"morphology": null,
"nominal_structure": null,
"negative_polarity_concord_items": null,
"quantifier": null,
"verbal_agreement": null,
"simple": null
} |
5,854 | Shibatani_1976 | 1acceptable
| g | ジョンはメアリーに自分が馬鹿であることを教えた。 | ジョンはメアリーに自分が馬鹿であることを教えた。 | null | null | {
"argument_structure": null,
"binding": null,
"control_raising": null,
"ellipsis": null,
"filler_gap": null,
"island_effects": null,
"morphology": null,
"nominal_structure": null,
"negative_polarity_concord_items": null,
"quantifier": null,
"verbal_agreement": null,
"simple": null
} |
838 | Inoue_1976 | 1acceptable
| g | 山田君は、加藤君を自分の部屋で勉強させた。 | 山田君は、加藤君を自分の部屋で勉強させた。 | null | null | {
"argument_structure": null,
"binding": null,
"control_raising": null,
"ellipsis": null,
"filler_gap": null,
"island_effects": null,
"morphology": null,
"nominal_structure": null,
"negative_polarity_concord_items": null,
"quantifier": null,
"verbal_agreement": null,
"simple": null
} |
7,303 | Shibatani_1976 | 1acceptable
| g | 山田先生はこの本をお読みにはなりませんでした。 | 山田先生はこの本をお読みにはなりませんでした。 | null | null | {
"argument_structure": null,
"binding": null,
"control_raising": null,
"ellipsis": null,
"filler_gap": null,
"island_effects": null,
"morphology": null,
"nominal_structure": null,
"negative_polarity_concord_items": null,
"quantifier": null,
"verbal_agreement": null,
"simple": null
} |
1,928 | Inoue_1976 | 1acceptable
| g | 山岡さんが読んでいた新聞を、隣の人が覗き込んだ。 | 山岡さんが読んでいた新聞を、隣の人が覗き込んだ。 | null | null | {
"argument_structure": null,
"binding": null,
"control_raising": null,
"ellipsis": null,
"filler_gap": null,
"island_effects": null,
"morphology": null,
"nominal_structure": null,
"negative_polarity_concord_items": null,
"quantifier": null,
"verbal_agreement": null,
"simple": null
} |
7,987 | Tsujimura_1999 | 1acceptable
| g | 学生が先生を批判した。 | 学生が先生を批判した。 | null | null | {
"argument_structure": null,
"binding": null,
"control_raising": null,
"ellipsis": null,
"filler_gap": null,
"island_effects": null,
"morphology": null,
"nominal_structure": null,
"negative_polarity_concord_items": null,
"quantifier": null,
"verbal_agreement": null,
"simple": null
} |
8,283 | Tsujimura_1999 | 1acceptable
| g | 太郎がペンキを壁に塗った。 | 太郎がペンキを壁に塗った。 | null | null | {
"argument_structure": null,
"binding": null,
"control_raising": null,
"ellipsis": null,
"filler_gap": null,
"island_effects": null,
"morphology": null,
"nominal_structure": null,
"negative_polarity_concord_items": null,
"quantifier": null,
"verbal_agreement": null,
"simple": null
} |
7,094 | Shibatani_1976 | 1acceptable
| g | 先生が私に貴重な蔵書を貸してくださった。 | 先生が私に貴重な蔵書を貸してくださった。 | null | null | {
"argument_structure": null,
"binding": null,
"control_raising": null,
"ellipsis": null,
"filler_gap": null,
"island_effects": null,
"morphology": null,
"nominal_structure": null,
"negative_polarity_concord_items": null,
"quantifier": null,
"verbal_agreement": null,
"simple": null
} |
6,360 | Shibatani_1976 | 1acceptable
| g | 彼女のことを考えた。 | 彼女のことを考えた。 | null | null | {
"argument_structure": null,
"binding": null,
"control_raising": null,
"ellipsis": null,
"filler_gap": null,
"island_effects": null,
"morphology": null,
"nominal_structure": null,
"negative_polarity_concord_items": null,
"quantifier": null,
"verbal_agreement": null,
"simple": null
} |
6,060 | Shibatani_1976 | 0unacceptable
| * | 思いやりのある医者は昏睡状態にあり助かる見込みのない病人に死なせた。 | 思いやりのある医者は昏睡状態にあり助かる見込みのない病人に死なせた。 | null | null | {
"argument_structure": null,
"binding": null,
"control_raising": null,
"ellipsis": null,
"filler_gap": null,
"island_effects": null,
"morphology": null,
"nominal_structure": null,
"negative_polarity_concord_items": null,
"quantifier": null,
"verbal_agreement": null,
"simple": null
} |
7,033 | Shibatani_1976 | 1acceptable
| g | 私は、小説は書けまい。 | 私は、小説は書けまい。 | null | null | {
"argument_structure": null,
"binding": null,
"control_raising": null,
"ellipsis": null,
"filler_gap": null,
"island_effects": null,
"morphology": null,
"nominal_structure": null,
"negative_polarity_concord_items": null,
"quantifier": null,
"verbal_agreement": null,
"simple": null
} |
7,182 | Shibatani_1976 | 1acceptable
| g | 太郎は紙である袋を拾った。 | 紙である袋 | null | null | {
"argument_structure": null,
"binding": null,
"control_raising": null,
"ellipsis": null,
"filler_gap": null,
"island_effects": null,
"morphology": null,
"nominal_structure": null,
"negative_polarity_concord_items": null,
"quantifier": null,
"verbal_agreement": null,
"simple": null
} |
5,097 | Miyagawa_and_Saito | 0unacceptable
| * | ジョンかビルはメアリーが何を買った後で出かけたの? | ジョンかビルはメアリーが何を買った後で出かけたの? | null | null | {
"argument_structure": null,
"binding": null,
"control_raising": null,
"ellipsis": null,
"filler_gap": null,
"island_effects": null,
"morphology": null,
"nominal_structure": null,
"negative_polarity_concord_items": null,
"quantifier": null,
"verbal_agreement": null,
"simple": null
} |
7,729 | Shibatani_1990 | 1acceptable
| g | 太郎が走ったので、僕は二郎にもそうさせた。 | 太郎が走ったので、僕は二郎にもそうさせた。 | null | null | {
"argument_structure": null,
"binding": null,
"control_raising": null,
"ellipsis": null,
"filler_gap": null,
"island_effects": null,
"morphology": null,
"nominal_structure": null,
"negative_polarity_concord_items": null,
"quantifier": null,
"verbal_agreement": null,
"simple": null
} |
1,329 | Inoue_1976 | 1acceptable
| g | 彼らの風船が徐々に膨らんでいった。 | 彼らの風船が徐々に膨らんでいった。 | null | null | {
"argument_structure": null,
"binding": null,
"control_raising": null,
"ellipsis": null,
"filler_gap": null,
"island_effects": null,
"morphology": null,
"nominal_structure": null,
"negative_polarity_concord_items": null,
"quantifier": null,
"verbal_agreement": null,
"simple": null
} |
2,098 | Inoue_1976 | 1acceptable
| g | 学生は、自分達の部屋に立て籠った。 | 学生は、自分達の部屋に立て籠った。 | null | null | {
"argument_structure": null,
"binding": null,
"control_raising": null,
"ellipsis": null,
"filler_gap": null,
"island_effects": null,
"morphology": null,
"nominal_structure": null,
"negative_polarity_concord_items": null,
"quantifier": null,
"verbal_agreement": null,
"simple": null
} |
8,194 | Tsujimura_1999 | 1acceptable
| g | 敵を待ち構える。 | 敵を待ち構える。 | null | null | {
"argument_structure": null,
"binding": null,
"control_raising": null,
"ellipsis": null,
"filler_gap": null,
"island_effects": null,
"morphology": null,
"nominal_structure": null,
"negative_polarity_concord_items": null,
"quantifier": null,
"verbal_agreement": null,
"simple": null
} |
5,788 | Shibatani_1976 | 1acceptable
| g | 自分の机を部屋に居た学生に加藤氏が拭かせた。 | 自分の机を部屋に居た学生に加藤氏が拭かせた。 | null | null | {
"argument_structure": null,
"binding": null,
"control_raising": null,
"ellipsis": null,
"filler_gap": null,
"island_effects": null,
"morphology": null,
"nominal_structure": null,
"negative_polarity_concord_items": null,
"quantifier": null,
"verbal_agreement": null,
"simple": null
} |
4,257 | Kuroda_1992 | 1acceptable
| g | 私は気を失っているところを捜索隊によって救助された。 | 私は気を失っているところを捜索隊によって救助された。 | null | null | {
"argument_structure": null,
"binding": null,
"control_raising": null,
"ellipsis": null,
"filler_gap": null,
"island_effects": null,
"morphology": null,
"nominal_structure": null,
"negative_polarity_concord_items": null,
"quantifier": null,
"verbal_agreement": null,
"simple": null
} |
5,218 | Miyagawa_and_Saito | 0unacceptable
| * | ロジャーは4杯のご飯をどんぶりに食べた。 | ロジャーは4杯のご飯をどんぶりに食べた。 | null | null | {
"argument_structure": null,
"binding": null,
"control_raising": null,
"ellipsis": null,
"filler_gap": null,
"island_effects": null,
"morphology": null,
"nominal_structure": null,
"negative_polarity_concord_items": null,
"quantifier": null,
"verbal_agreement": null,
"simple": null
} |
2,872 | Kuno_1973 | 0unacceptable
| * | 寒くなったなら、暖房を入れた。 | 寒くなったなら、暖房を入れた。 | null | null | {
"argument_structure": null,
"binding": null,
"control_raising": null,
"ellipsis": null,
"filler_gap": null,
"island_effects": null,
"morphology": null,
"nominal_structure": null,
"negative_polarity_concord_items": null,
"quantifier": null,
"verbal_agreement": null,
"simple": null
} |
1,926 | Inoue_1976 | 1acceptable
| g | 会議に出ている人々が、ここに集っている。 | 会議に出ている人々が、ここに集っている。 | null | null | {
"argument_structure": null,
"binding": null,
"control_raising": null,
"ellipsis": null,
"filler_gap": null,
"island_effects": null,
"morphology": null,
"nominal_structure": null,
"negative_polarity_concord_items": null,
"quantifier": null,
"verbal_agreement": null,
"simple": null
} |
3,535 | Kuno_1973 | 1acceptable
| g | 山が木が綺麗だ。 | 山が木が綺麗だ。 | null | null | {
"argument_structure": null,
"binding": null,
"control_raising": null,
"ellipsis": null,
"filler_gap": null,
"island_effects": null,
"morphology": null,
"nominal_structure": null,
"negative_polarity_concord_items": null,
"quantifier": null,
"verbal_agreement": null,
"simple": null
} |
1,936 | Inoue_1976 | 0unacceptable
| * | 花子さんが連れている子供は太郎だった。 | 花子さんが連れている子供は太郎だった。 | null | null | {
"argument_structure": null,
"binding": null,
"control_raising": null,
"ellipsis": null,
"filler_gap": null,
"island_effects": null,
"morphology": null,
"nominal_structure": null,
"negative_polarity_concord_items": null,
"quantifier": null,
"verbal_agreement": null,
"simple": null
} |
7,151 | Shibatani_1976 | 1acceptable
| g | 太郎は蒸気機関車に乗った。 | 蒸気機関車 | null | null | {
"argument_structure": null,
"binding": null,
"control_raising": null,
"ellipsis": null,
"filler_gap": null,
"island_effects": null,
"morphology": null,
"nominal_structure": null,
"negative_polarity_concord_items": null,
"quantifier": null,
"verbal_agreement": null,
"simple": null
} |
4,998 | Miyagawa_and_Saito | 1acceptable
| g | うちの動物園では3頭のカバがオスだ。 | うちの動物園では3頭のカバがオスだ。 | null | null | {
"argument_structure": null,
"binding": null,
"control_raising": null,
"ellipsis": null,
"filler_gap": null,
"island_effects": null,
"morphology": null,
"nominal_structure": null,
"negative_polarity_concord_items": null,
"quantifier": null,
"verbal_agreement": null,
"simple": null
} |
2,514 | Kuno_1973 | 1acceptable
| g | メアリーがジョンと勉強した。 | メアリーがジョンと勉強した。 | null | null | {
"argument_structure": null,
"binding": null,
"control_raising": null,
"ellipsis": null,
"filler_gap": null,
"island_effects": null,
"morphology": null,
"nominal_structure": null,
"negative_polarity_concord_items": null,
"quantifier": null,
"verbal_agreement": null,
"simple": null
} |
3,520 | Kuno_1973 | 1acceptable
| g | 出場者のうちの3人が、20箱の饅頭を食べた。 | 出場者のうちの3人が、20箱の饅頭を食べた。 | null | null | {
"argument_structure": null,
"binding": null,
"control_raising": null,
"ellipsis": null,
"filler_gap": null,
"island_effects": null,
"morphology": null,
"nominal_structure": null,
"negative_polarity_concord_items": null,
"quantifier": null,
"verbal_agreement": null,
"simple": null
} |
2,033 | Inoue_1976 | 1acceptable
| g | 自分の子供の手術をした医者が山田さんに尊敬された。 | 自分の子供の手術をした医者が山田さんに尊敬された。 | null | null | {
"argument_structure": null,
"binding": null,
"control_raising": null,
"ellipsis": null,
"filler_gap": null,
"island_effects": null,
"morphology": null,
"nominal_structure": null,
"negative_polarity_concord_items": null,
"quantifier": null,
"verbal_agreement": null,
"simple": null
} |
6,979 | Shibatani_1976 | 1acceptable
| g | 昨日太郎は、いつも寄り添っている二郎と花子の間に割り込んだ。 | 昨日太郎は、いつも寄り添っている二郎と花子の間に割り込んだ。 | null | null | {
"argument_structure": null,
"binding": null,
"control_raising": null,
"ellipsis": null,
"filler_gap": null,
"island_effects": null,
"morphology": null,
"nominal_structure": null,
"negative_polarity_concord_items": null,
"quantifier": null,
"verbal_agreement": null,
"simple": null
} |
3,340 | Kuno_1973 | 1acceptable
| g | ジョンは買う本を読んだ。 | ジョンは買う本を読んだ。 | null | null | {
"argument_structure": null,
"binding": null,
"control_raising": null,
"ellipsis": null,
"filler_gap": null,
"island_effects": null,
"morphology": null,
"nominal_structure": null,
"negative_polarity_concord_items": null,
"quantifier": null,
"verbal_agreement": null,
"simple": null
} |
3,475 | Kuno_1973 | 0unacceptable
| * | メアリーがジョンに会われた。 | メアリーがジョンに会われた。 | null | null | {
"argument_structure": null,
"binding": null,
"control_raising": null,
"ellipsis": null,
"filler_gap": null,
"island_effects": null,
"morphology": null,
"nominal_structure": null,
"negative_polarity_concord_items": null,
"quantifier": null,
"verbal_agreement": null,
"simple": null
} |
1,486 | Inoue_1976 | 1acceptable
| g | 太郎は、家事で家財道具を焼いた。 | 太郎は、家事で家財道具を焼いた。 | null | null | {
"argument_structure": null,
"binding": null,
"control_raising": null,
"ellipsis": null,
"filler_gap": null,
"island_effects": null,
"morphology": null,
"nominal_structure": null,
"negative_polarity_concord_items": null,
"quantifier": null,
"verbal_agreement": null,
"simple": null
} |
4,404 | Kuroda_1992 | 1acceptable
| g | ジョンは言語学者が好きだ。 | ジョンは言語学者が好きだ。 | null | null | {
"argument_structure": null,
"binding": null,
"control_raising": null,
"ellipsis": null,
"filler_gap": null,
"island_effects": null,
"morphology": null,
"nominal_structure": null,
"negative_polarity_concord_items": null,
"quantifier": null,
"verbal_agreement": null,
"simple": null
} |
2,933 | Kuno_1973 | 1acceptable
| g | 来年の夏になったら、ニューヨークに行きます。 | 来年の夏になったら、ニューヨークに行きます。 | null | null | {
"argument_structure": null,
"binding": null,
"control_raising": null,
"ellipsis": null,
"filler_gap": null,
"island_effects": null,
"morphology": null,
"nominal_structure": null,
"negative_polarity_concord_items": null,
"quantifier": null,
"verbal_agreement": null,
"simple": null
} |
2,581 | Kuno_1973 | 1acceptable
| g | ジョンが東京に行き、メアリーが大阪に行く。 | ジョンが東京に行き、メアリーが大阪に行く。 | null | null | {
"argument_structure": null,
"binding": null,
"control_raising": null,
"ellipsis": null,
"filler_gap": null,
"island_effects": null,
"morphology": null,
"nominal_structure": null,
"negative_polarity_concord_items": null,
"quantifier": null,
"verbal_agreement": null,
"simple": null
} |
8,136 | Tsujimura_1999 | 1acceptable
| g | ジョンは誰がパーティーに来るか大体知っている。 | ジョンは誰がパーティーに来るか大体知っている。 | null | null | {
"argument_structure": null,
"binding": null,
"control_raising": null,
"ellipsis": null,
"filler_gap": null,
"island_effects": null,
"morphology": null,
"nominal_structure": null,
"negative_polarity_concord_items": null,
"quantifier": null,
"verbal_agreement": null,
"simple": null
} |
3,828 | Kuroda_1965 | 1acceptable
| g | 何が倒れたか? | 何が倒れたか? | null | null | {
"argument_structure": null,
"binding": null,
"control_raising": null,
"ellipsis": null,
"filler_gap": null,
"island_effects": null,
"morphology": null,
"nominal_structure": null,
"negative_polarity_concord_items": null,
"quantifier": null,
"verbal_agreement": null,
"simple": null
} |
7,225 | Shibatani_1976 | 0unacceptable
| ?? | 金買い | 金買い | null | null | {
"argument_structure": null,
"binding": null,
"control_raising": null,
"ellipsis": null,
"filler_gap": null,
"island_effects": null,
"morphology": null,
"nominal_structure": null,
"negative_polarity_concord_items": null,
"quantifier": null,
"verbal_agreement": null,
"simple": null
} |
5,131 | Miyagawa_and_Saito | 0unacceptable
| * | 花子は京都になぜ来た人を呼びましたか? | 花子は京都になぜ来た人を呼びましたか? | null | null | {
"argument_structure": null,
"binding": null,
"control_raising": null,
"ellipsis": null,
"filler_gap": null,
"island_effects": null,
"morphology": null,
"nominal_structure": null,
"negative_polarity_concord_items": null,
"quantifier": null,
"verbal_agreement": null,
"simple": null
} |
4,827 | Miyagawa_and_Saito | 1acceptable
| g | 雅美が裕美を自分の部屋で褒めた。 | 雅美が裕美を自分の部屋で褒めた。 | null | null | {
"argument_structure": null,
"binding": null,
"control_raising": null,
"ellipsis": null,
"filler_gap": null,
"island_effects": null,
"morphology": null,
"nominal_structure": null,
"negative_polarity_concord_items": null,
"quantifier": null,
"verbal_agreement": null,
"simple": null
} |
3,083 | Kuno_1973 | 1acceptable
| g | ジョンはメアリーが犯人であることを推定した。 | ジョンはメアリーが犯人であることを推定した。 | null | null | {
"argument_structure": null,
"binding": null,
"control_raising": null,
"ellipsis": null,
"filler_gap": null,
"island_effects": null,
"morphology": null,
"nominal_structure": null,
"negative_polarity_concord_items": null,
"quantifier": null,
"verbal_agreement": null,
"simple": null
} |
3,225 | Kuno_1973 | 1acceptable
| g | その子供が可愛がっていた犬が死んでしまった。 | その子供が可愛がっていた犬が死んでしまった。 | null | null | {
"argument_structure": null,
"binding": null,
"control_raising": null,
"ellipsis": null,
"filler_gap": null,
"island_effects": null,
"morphology": null,
"nominal_structure": null,
"negative_polarity_concord_items": null,
"quantifier": null,
"verbal_agreement": null,
"simple": null
} |
5,814 | Shibatani_1976 | 1acceptable
| g | いつも自分の部屋にいる友達が花子を心配させた。 | いつも自分の部屋にいる友達が花子を心配させた。 | null | null | {
"argument_structure": null,
"binding": null,
"control_raising": null,
"ellipsis": null,
"filler_gap": null,
"island_effects": null,
"morphology": null,
"nominal_structure": null,
"negative_polarity_concord_items": null,
"quantifier": null,
"verbal_agreement": null,
"simple": null
} |
4,290 | Kuroda_1992 | 1acceptable
| g | CIAが大統領を殺してしまった。 | CIAが大統領を殺してしまった。 | null | null | {
"argument_structure": null,
"binding": null,
"control_raising": null,
"ellipsis": null,
"filler_gap": null,
"island_effects": null,
"morphology": null,
"nominal_structure": null,
"negative_polarity_concord_items": null,
"quantifier": null,
"verbal_agreement": null,
"simple": null
} |
6,459 | Shibatani_1976 | 0unacceptable
| * | 彼女は買い物に行くというのが嫌いだ。 | 彼女は買い物に行くというのが嫌いだ。 | null | null | {
"argument_structure": null,
"binding": null,
"control_raising": null,
"ellipsis": null,
"filler_gap": null,
"island_effects": null,
"morphology": null,
"nominal_structure": null,
"negative_polarity_concord_items": null,
"quantifier": null,
"verbal_agreement": null,
"simple": null
} |
5,737 | Shibatani_1976 | 1acceptable
| g | ジョンは自分が書いた手紙を破り据えた。 | ジョンは自分が書いた手紙を破り据えた。 | null | null | {
"argument_structure": null,
"binding": null,
"control_raising": null,
"ellipsis": null,
"filler_gap": null,
"island_effects": null,
"morphology": null,
"nominal_structure": null,
"negative_polarity_concord_items": null,
"quantifier": null,
"verbal_agreement": null,
"simple": null
} |
8,025 | Tsujimura_1999 | 1acceptable
| g | ヨーロッパの国が資本家たちによって6つその美しい自然を破壊されている。 | ヨーロッパの国が資本家たちによって6つその美しい自然を破壊されている。 | null | null | {
"argument_structure": null,
"binding": null,
"control_raising": null,
"ellipsis": null,
"filler_gap": null,
"island_effects": null,
"morphology": null,
"nominal_structure": null,
"negative_polarity_concord_items": null,
"quantifier": null,
"verbal_agreement": null,
"simple": null
} |
8,163 | Tsujimura_1999 | 1acceptable
| g | 先生はお痩せにさえなった。 | 先生はお痩せにさえなった。 | null | null | {
"argument_structure": null,
"binding": null,
"control_raising": null,
"ellipsis": null,
"filler_gap": null,
"island_effects": null,
"morphology": null,
"nominal_structure": null,
"negative_polarity_concord_items": null,
"quantifier": null,
"verbal_agreement": null,
"simple": null
} |
5,706 | Shibatani_1976 | 1acceptable
| g | ジョンは自分が教えている学生と結婚したがっているよ。 | ジョンは自分が教えている学生と結婚したがっているよ。 | null | null | {
"argument_structure": null,
"binding": null,
"control_raising": null,
"ellipsis": null,
"filler_gap": null,
"island_effects": null,
"morphology": null,
"nominal_structure": null,
"negative_polarity_concord_items": null,
"quantifier": null,
"verbal_agreement": null,
"simple": null
} |
4,669 | Kuroda_1992 | 1acceptable
| g | 盃が正男によって酒を満たされた。 | 盃が正男によって酒を満たされた。 | null | null | {
"argument_structure": null,
"binding": null,
"control_raising": null,
"ellipsis": null,
"filler_gap": null,
"island_effects": null,
"morphology": null,
"nominal_structure": null,
"negative_polarity_concord_items": null,
"quantifier": null,
"verbal_agreement": null,
"simple": null
} |
4,227 | Kuroda_1992 | 1acceptable
| g | 白いボールが王によって高々と打ち上げられた。 | 白いボールが王によって高々と打ち上げられた。 | null | null | {
"argument_structure": null,
"binding": null,
"control_raising": null,
"ellipsis": null,
"filler_gap": null,
"island_effects": null,
"morphology": null,
"nominal_structure": null,
"negative_polarity_concord_items": null,
"quantifier": null,
"verbal_agreement": null,
"simple": null
} |
6,313 | Shibatani_1976 | 0unacceptable
| ?* | 部屋に入ると私は子供がタバコを喫むのを見つけた。 | 部屋に入ると私は子供がタバコを喫むのを見つけた。 | null | null | {
"argument_structure": null,
"binding": null,
"control_raising": null,
"ellipsis": null,
"filler_gap": null,
"island_effects": null,
"morphology": null,
"nominal_structure": null,
"negative_polarity_concord_items": null,
"quantifier": null,
"verbal_agreement": null,
"simple": null
} |
2,183 | Kuno_1973 | 1acceptable
| g | 太郎は1本の木を植えた。 | 1本の木 | null | null | {
"argument_structure": null,
"binding": null,
"control_raising": null,
"ellipsis": null,
"filler_gap": null,
"island_effects": null,
"morphology": null,
"nominal_structure": null,
"negative_polarity_concord_items": null,
"quantifier": null,
"verbal_agreement": null,
"simple": null
} |
786 | Inoue_1976 | 1acceptable
| g | 我々がこの鍋で揚げ物をする。 | 我々がこの鍋で揚げ物をする。 | null | null | {
"argument_structure": null,
"binding": null,
"control_raising": null,
"ellipsis": null,
"filler_gap": null,
"island_effects": null,
"morphology": null,
"nominal_structure": null,
"negative_polarity_concord_items": null,
"quantifier": null,
"verbal_agreement": null,
"simple": null
} |
65 | Gunji_1987 | 1acceptable
| g | 健は直美に海外旅行を進めた。 | 健は直美に海外旅行を進めた。 | null | null | {
"argument_structure": null,
"binding": null,
"control_raising": null,
"ellipsis": null,
"filler_gap": null,
"island_effects": null,
"morphology": null,
"nominal_structure": null,
"negative_polarity_concord_items": null,
"quantifier": null,
"verbal_agreement": null,
"simple": null
} |
932 | Inoue_1976 | 1acceptable
| g | ジョンがメアリーと友達だ。 | ジョンがメアリーと友達だ。 | null | null | {
"argument_structure": null,
"binding": null,
"control_raising": null,
"ellipsis": null,
"filler_gap": null,
"island_effects": null,
"morphology": null,
"nominal_structure": null,
"negative_polarity_concord_items": null,
"quantifier": null,
"verbal_agreement": null,
"simple": null
} |
6,434 | Shibatani_1976 | 1acceptable
| g | 総理大臣が自殺したという報告をラジオで聞いた。 | 総理大臣が自殺したという報告をラジオで聞いた。 | null | null | {
"argument_structure": null,
"binding": null,
"control_raising": null,
"ellipsis": null,
"filler_gap": null,
"island_effects": null,
"morphology": null,
"nominal_structure": null,
"negative_polarity_concord_items": null,
"quantifier": null,
"verbal_agreement": null,
"simple": null
} |
8,582 | Tsujimura_2013 | 0unacceptable
| * | 子供が二郎によって美智子に褒めさせられた。 | 子供が二郎によって美智子に褒めさせられた。 | null | null | {
"argument_structure": null,
"binding": null,
"control_raising": null,
"ellipsis": null,
"filler_gap": null,
"island_effects": null,
"morphology": null,
"nominal_structure": null,
"negative_polarity_concord_items": null,
"quantifier": null,
"verbal_agreement": null,
"simple": null
} |
4,484 | Kuroda_1992 | 1acceptable
| g | この種の事故が損害賠償を請求しにくい。 | この種の事故が損害賠償を請求しにくい。 | null | null | {
"argument_structure": null,
"binding": null,
"control_raising": null,
"ellipsis": null,
"filler_gap": null,
"island_effects": null,
"morphology": null,
"nominal_structure": null,
"negative_polarity_concord_items": null,
"quantifier": null,
"verbal_agreement": null,
"simple": null
} |
7,593 | Shibatani_1990 | 1acceptable
| g | 子供が母親に叱られて泣いた。 | 子供が母親に叱られて泣いた。 | null | null | {
"argument_structure": null,
"binding": null,
"control_raising": null,
"ellipsis": null,
"filler_gap": null,
"island_effects": null,
"morphology": null,
"nominal_structure": null,
"negative_polarity_concord_items": null,
"quantifier": null,
"verbal_agreement": null,
"simple": null
} |
5,990 | Shibatani_1976 | 1acceptable
| g | 太郎が働く。 | 太郎が働く。 | null | null | {
"argument_structure": null,
"binding": null,
"control_raising": null,
"ellipsis": null,
"filler_gap": null,
"island_effects": null,
"morphology": null,
"nominal_structure": null,
"negative_polarity_concord_items": null,
"quantifier": null,
"verbal_agreement": null,
"simple": null
} |
521 | Inoue_1976 | 0unacceptable
| * | ジョンが仕事を押し付けられたのは妹だ。 | ジョンが仕事を押し付けられたのは妹だ。 | null | null | {
"argument_structure": null,
"binding": null,
"control_raising": null,
"ellipsis": null,
"filler_gap": null,
"island_effects": null,
"morphology": null,
"nominal_structure": null,
"negative_polarity_concord_items": null,
"quantifier": null,
"verbal_agreement": null,
"simple": null
} |
7,619 | Shibatani_1990 | 1acceptable
| g | 筧先生が教室で英語を教えている。 | 筧先生が教室で英語を教えている。 | null | null | {
"argument_structure": null,
"binding": null,
"control_raising": null,
"ellipsis": null,
"filler_gap": null,
"island_effects": null,
"morphology": null,
"nominal_structure": null,
"negative_polarity_concord_items": null,
"quantifier": null,
"verbal_agreement": null,
"simple": null
} |
892 | Inoue_1976 | 1acceptable
| g | 君に読める本はこれだ。 | 君に読める本 | null | null | {
"argument_structure": null,
"binding": null,
"control_raising": null,
"ellipsis": null,
"filler_gap": null,
"island_effects": null,
"morphology": null,
"nominal_structure": null,
"negative_polarity_concord_items": null,
"quantifier": null,
"verbal_agreement": null,
"simple": null
} |
6,842 | Shibatani_1976 | 1acceptable
| g | 電気が点けてある。 | 電気が点けてある。 | null | null | {
"argument_structure": null,
"binding": null,
"control_raising": null,
"ellipsis": null,
"filler_gap": null,
"island_effects": null,
"morphology": null,
"nominal_structure": null,
"negative_polarity_concord_items": null,
"quantifier": null,
"verbal_agreement": null,
"simple": null
} |
7,649 | Shibatani_1990 | 1acceptable
| g | 太郎は背が高いことを自慢している。 | 太郎は背が高いことを自慢している。 | null | null | {
"argument_structure": null,
"binding": null,
"control_raising": null,
"ellipsis": null,
"filler_gap": null,
"island_effects": null,
"morphology": null,
"nominal_structure": null,
"negative_polarity_concord_items": null,
"quantifier": null,
"verbal_agreement": null,
"simple": null
} |
6,563 | Shibatani_1976 | 1acceptable
| g | 誰も行くまい。 | 誰も行くまい。 | null | null | {
"argument_structure": null,
"binding": null,
"control_raising": null,
"ellipsis": null,
"filler_gap": null,
"island_effects": null,
"morphology": null,
"nominal_structure": null,
"negative_polarity_concord_items": null,
"quantifier": null,
"verbal_agreement": null,
"simple": null
} |
7,585 | Shibatani_1990 | 1acceptable
| g | そこへ教師が登場して、独り言を言う。 | そこへ教師が登場して、独り言を言う。 | null | null | {
"argument_structure": null,
"binding": null,
"control_raising": null,
"ellipsis": null,
"filler_gap": null,
"island_effects": null,
"morphology": null,
"nominal_structure": null,
"negative_polarity_concord_items": null,
"quantifier": null,
"verbal_agreement": null,
"simple": null
} |
5,980 | Shibatani_1976 | 1acceptable
| g | 田中さんは田中さんの選挙区で演説した。 | 田中さんは田中さんの選挙区で演説した。 | null | null | {
"argument_structure": null,
"binding": null,
"control_raising": null,
"ellipsis": null,
"filler_gap": null,
"island_effects": null,
"morphology": null,
"nominal_structure": null,
"negative_polarity_concord_items": null,
"quantifier": null,
"verbal_agreement": null,
"simple": null
} |
2,694 | Kuno_1973 | 1acceptable
| g | サインをしてやってください。 | サインをしてやってください。 | null | null | {
"argument_structure": null,
"binding": null,
"control_raising": null,
"ellipsis": null,
"filler_gap": null,
"island_effects": null,
"morphology": null,
"nominal_structure": null,
"negative_polarity_concord_items": null,
"quantifier": null,
"verbal_agreement": null,
"simple": null
} |
7,421 | Shibatani_1976 | 1acceptable
| g | 佐藤先生はファウストをお読みになり始めた。 | 佐藤先生はファウストをお読みになり始めた。 | null | null | {
"argument_structure": null,
"binding": null,
"control_raising": null,
"ellipsis": null,
"filler_gap": null,
"island_effects": null,
"morphology": null,
"nominal_structure": null,
"negative_polarity_concord_items": null,
"quantifier": null,
"verbal_agreement": null,
"simple": null
} |
5,360 | Shibatani_1976 | 1acceptable
| g | 逃げるが最上の策だ。 | 逃げるが最上の策だ。 | null | null | {
"argument_structure": null,
"binding": null,
"control_raising": null,
"ellipsis": null,
"filler_gap": null,
"island_effects": null,
"morphology": null,
"nominal_structure": null,
"negative_polarity_concord_items": null,
"quantifier": null,
"verbal_agreement": null,
"simple": null
} |
7,851 | Shibatani_1990 | 1acceptable
| g | 太郎は行くつもりらしい。 | 太郎は行くつもりらしい。 | null | null | {
"argument_structure": null,
"binding": null,
"control_raising": null,
"ellipsis": null,
"filler_gap": null,
"island_effects": null,
"morphology": null,
"nominal_structure": null,
"negative_polarity_concord_items": null,
"quantifier": null,
"verbal_agreement": null,
"simple": null
} |
7,116 | Shibatani_1976 | 1acceptable
| g | 外国行きの切符を手にした。 | 外国行き | null | null | {
"argument_structure": null,
"binding": null,
"control_raising": null,
"ellipsis": null,
"filler_gap": null,
"island_effects": null,
"morphology": null,
"nominal_structure": null,
"negative_polarity_concord_items": null,
"quantifier": null,
"verbal_agreement": null,
"simple": null
} |
900 | Inoue_1976 | 1acceptable
| g | お寺がたくさんある町は素敵だ。 | お寺がたくさんある町 | null | null | {
"argument_structure": null,
"binding": null,
"control_raising": null,
"ellipsis": null,
"filler_gap": null,
"island_effects": null,
"morphology": null,
"nominal_structure": null,
"negative_polarity_concord_items": null,
"quantifier": null,
"verbal_agreement": null,
"simple": null
} |
6,778 | Shibatani_1976 | 1acceptable
| g | 田中さんは、そのとき学生だった。 | 田中さんは、そのとき学生だった。 | null | null | {
"argument_structure": null,
"binding": null,
"control_raising": null,
"ellipsis": null,
"filler_gap": null,
"island_effects": null,
"morphology": null,
"nominal_structure": null,
"negative_polarity_concord_items": null,
"quantifier": null,
"verbal_agreement": null,
"simple": null
} |
1,326 | Inoue_1976 | 1acceptable
| g | 市役所前の道路が広がった。 | 市役所前の道路が広がった。 | null | null | {
"argument_structure": null,
"binding": null,
"control_raising": null,
"ellipsis": null,
"filler_gap": null,
"island_effects": null,
"morphology": null,
"nominal_structure": null,
"negative_polarity_concord_items": null,
"quantifier": null,
"verbal_agreement": null,
"simple": null
} |
419 | Inoue_1976 | 1acceptable
| g | 池田氏は息子を秀才だと信じている。 | 池田氏は息子を秀才だと信じている。 | null | null | {
"argument_structure": null,
"binding": null,
"control_raising": null,
"ellipsis": null,
"filler_gap": null,
"island_effects": null,
"morphology": null,
"nominal_structure": null,
"negative_polarity_concord_items": null,
"quantifier": null,
"verbal_agreement": null,
"simple": null
} |
7,909 | Tsujimura_1999 | 1acceptable
| g | 正男が太郎と花子にお互いを紹介した。 | 正男が太郎と花子にお互いを紹介した。 | null | null | {
"argument_structure": null,
"binding": null,
"control_raising": null,
"ellipsis": null,
"filler_gap": null,
"island_effects": null,
"morphology": null,
"nominal_structure": null,
"negative_polarity_concord_items": null,
"quantifier": null,
"verbal_agreement": null,
"simple": null
} |
4,228 | Kuroda_1992 | 0unacceptable
| * | 白いボールが王に高々と打ち上げられた。 | 白いボールが王に高々と打ち上げられた。 | null | null | {
"argument_structure": null,
"binding": null,
"control_raising": null,
"ellipsis": null,
"filler_gap": null,
"island_effects": null,
"morphology": null,
"nominal_structure": null,
"negative_polarity_concord_items": null,
"quantifier": null,
"verbal_agreement": null,
"simple": null
} |
357 | Inoue_1976 | 1acceptable
| g | 隣の家が売りに出ているそうだ。 | 隣の家が売りに出ているそうだ。 | null | null | {
"argument_structure": null,
"binding": null,
"control_raising": null,
"ellipsis": null,
"filler_gap": null,
"island_effects": null,
"morphology": null,
"nominal_structure": null,
"negative_polarity_concord_items": null,
"quantifier": null,
"verbal_agreement": null,
"simple": null
} |
1,005 | Inoue_1976 | 1acceptable
| g | その人が死んだのに誰も悲しまなかった。 | その人が死んだのに誰も悲しまなかった。 | null | null | {
"argument_structure": null,
"binding": null,
"control_raising": null,
"ellipsis": null,
"filler_gap": null,
"island_effects": null,
"morphology": null,
"nominal_structure": null,
"negative_polarity_concord_items": null,
"quantifier": null,
"verbal_agreement": null,
"simple": null
} |
6,279 | Shibatani_1976 | 1acceptable
| g | 二郎は手紙を出すことを忘れていた。 | 二郎は手紙を出すことを忘れていた。 | null | null | {
"argument_structure": null,
"binding": null,
"control_raising": null,
"ellipsis": null,
"filler_gap": null,
"island_effects": null,
"morphology": null,
"nominal_structure": null,
"negative_polarity_concord_items": null,
"quantifier": null,
"verbal_agreement": null,
"simple": null
} |
2,545 | Kuno_1973 | 0unacceptable
| * | 私はスリと遭った。 | 私はスリと遭った。 | null | null | {
"argument_structure": null,
"binding": null,
"control_raising": null,
"ellipsis": null,
"filler_gap": null,
"island_effects": null,
"morphology": null,
"nominal_structure": null,
"negative_polarity_concord_items": null,
"quantifier": null,
"verbal_agreement": null,
"simple": null
} |
7,229 | Shibatani_1976 | 1acceptable
| g | 手紙を書く。 | 手紙を書く。 | null | null | {
"argument_structure": null,
"binding": null,
"control_raising": null,
"ellipsis": null,
"filler_gap": null,
"island_effects": null,
"morphology": null,
"nominal_structure": null,
"negative_polarity_concord_items": null,
"quantifier": null,
"verbal_agreement": null,
"simple": null
} |
Dataset Card for JGLUE
This dataset loading script is developed on GitHub. Please feel free to open an issue or pull request.
Dataset Summary
From JGLUE's README.md:
JGLUE, Japanese General Language Understanding Evaluation, is built to measure the general NLU ability in Japanese. JGLUE has been constructed from scratch without translation. We hope that JGLUE will facilitate NLU research in Japanese.
JGLUE has been constructed by a joint research project of Yahoo Japan Corporation and Kawahara Lab at Waseda University.
Supported Tasks and Leaderboards
From JGLUE's README.md:
JGLUE consists of the tasks of text classification, sentence pair classification, and QA. Each task consists of multiple datasets.
Supported Tasks
MARC-ja
From JGLUE's README.md:
MARC-ja is a dataset of the text classification task. This dataset is based on the Japanese portion of Multilingual Amazon Reviews Corpus (MARC) (Keung+, 2020).
JCoLA
From JCoLA's README.md
JCoLA (Japanese Corpus of Linguistic Accept010 ability) is a novel dataset for targeted syntactic evaluations of language models in Japanese, which consists of 10,020 sentences with acceptability judgments by linguists. The sentences are manually extracted from linguistics journals, handbooks and textbooks. JCoLA is included in JGLUE benchmark (Kurihara et al., 2022).
JSTS
From JGLUE's README.md:
JSTS is a Japanese version of the STS (Semantic Textual Similarity) dataset. STS is a task to estimate the semantic similarity of a sentence pair. The sentences in JSTS and JNLI (described below) are extracted from the Japanese version of the MS COCO Caption Dataset, the YJ Captions Dataset (Miyazaki and Shimizu, 2016).
JNLI
From JGLUE's README.md:
JNLI is a Japanese version of the NLI (Natural Language Inference) dataset. NLI is a task to recognize the inference relation that a premise sentence has to a hypothesis sentence. The inference relations are entailment, contradiction, and neutral.
JSQuAD
From JGLUE's README.md:
JSQuAD is a Japanese version of SQuAD (Rajpurkar+, 2018), one of the datasets of reading comprehension. Each instance in the dataset consists of a question regarding a given context (Wikipedia article) and its answer. JSQuAD is based on SQuAD 1.1 (there are no unanswerable questions). We used the Japanese Wikipedia dump as of 20211101.
JCommonsenseQA
From JGLUE's README.md:
JCommonsenseQA is a Japanese version of CommonsenseQA (Talmor+, 2019), which is a multiple-choice question answering dataset that requires commonsense reasoning ability. It is built using crowdsourcing with seeds extracted from the knowledge base ConceptNet.
Leaderboard
From JGLUE's README.md:
A leaderboard will be made public soon. The test set will be released at that time.
Languages
The language data in JGLUE is in Japanese (BCP-47 ja-JP).
Dataset Structure
Data Instances
When loading a specific configuration, users has to append a version dependent suffix:
MARC-ja
from datasets import load_dataset
dataset = load_dataset("shunk031/JGLUE", name="MARC-ja")
print(dataset)
# DatasetDict({
# train: Dataset({
# features: ['sentence', 'label', 'review_id'],
# num_rows: 187528
# })
# validation: Dataset({
# features: ['sentence', 'label', 'review_id'],
# num_rows: 5654
# })
# })
JCoLA
from datasets import load_dataset
dataset = load_dataset("shunk031/JGLUE", name="JCoLA")
print(dataset)
# DatasetDict({
# train: Dataset({
# features: ['uid', 'source', 'label', 'diacritic', 'sentence', 'original', 'translation', 'gloss', 'simple', 'linguistic_phenomenon'],
# num_rows: 6919
# })
# validation: Dataset({
# features: ['uid', 'source', 'label', 'diacritic', 'sentence', 'original', 'translation', 'gloss', 'simple', 'linguistic_phenomenon'],
# num_rows: 865
# })
# validation_out_of_domain: Dataset({
# features: ['uid', 'source', 'label', 'diacritic', 'sentence', 'original', 'translation', 'gloss', 'simple', 'linguistic_phenomenon'],
# num_rows: 685
# })
# validation_out_of_domain_annotated: Dataset({
# features: ['uid', 'source', 'label', 'diacritic', 'sentence', 'original', 'translation', 'gloss', 'simple', 'linguistic_phenomenon'],
# num_rows: 685
# })
# })
An example of the JCoLA dataset (validation - out of domain annotated) looks as follows:
{
"uid": 9109,
"source": "Asano_and_Ura_2010",
"label": 1,
"diacritic": "g",
"sentence": "太郎のゴミの捨て方について話した。",
"original": "太郎のゴミの捨て方",
"translation": "‘The way (for Taro) to throw out garbage’",
"gloss": true,
"linguistic_phenomenon": {
"argument_structure": true,
"binding": false,
"control_raising": false,
"ellipsis": false,
"filler_gap": false,
"island_effects": false,
"morphology": false,
"nominal_structure": false,
"negative_polarity_concord_items": false,
"quantifier": false,
"verbal_agreement": false,
"simple": false
}
}
JSTS
from datasets import load_dataset
dataset = load_dataset("shunk031/JGLUE", name="JSTS")
print(dataset)
# DatasetDict({
# train: Dataset({
# features: ['sentence_pair_id', 'yjcaptions_id', 'sentence1', 'sentence2', 'label'],
# num_rows: 12451
# })
# validation: Dataset({
# features: ['sentence_pair_id', 'yjcaptions_id', 'sentence1', 'sentence2', 'label'],
# num_rows: 1457
# })
# })
An example of the JSTS dataset looks as follows:
{
"sentence_pair_id": "691",
"yjcaptions_id": "127202-129817-129818",
"sentence1": "街中の道路を大きなバスが走っています。 (A big bus is running on the road in the city.)",
"sentence2": "道路を大きなバスが走っています。 (There is a big bus running on the road.)",
"label": 4.4
}
JNLI
from datasets import load_dataset
dataset = load_dataset("shunk031/JGLUE", name="JNLI")
print(dataset)
# DatasetDict({
# train: Dataset({
# features: ['sentence_pair_id', 'yjcaptions_id', 'sentence1', 'sentence2', 'label'],
# num_rows: 20073
# })
# validation: Dataset({
# features: ['sentence_pair_id', 'yjcaptions_id', 'sentence1', 'sentence2', 'label'],
# num_rows: 2434
# })
# })
An example of the JNLI dataset looks as follows:
{
"sentence_pair_id": "1157",
"yjcaptions_id": "127202-129817-129818",
"sentence1": "街中の道路を大きなバスが走っています。 (A big bus is running on the road in the city.)",
"sentence2": "道路を大きなバスが走っています。 (There is a big bus running on the road.)",
"label": "entailment"
}
JSQuAD
from datasets import load_dataset
dataset = load_dataset("shunk031/JGLUE", name="JSQuAD")
print(dataset)
# DatasetDict({
# train: Dataset({
# features: ['id', 'title', 'context', 'question', 'answers', 'is_impossible'],
# num_rows: 62859
# })
# validation: Dataset({
# features: ['id', 'title', 'context', 'question', 'answers', 'is_impossible'],
# num_rows: 4442
# })
# })
An example of the JSQuAD looks as follows:
{
"id": "a1531320p0q0",
"title": "東海道新幹線",
"context": "東海道新幹線 [SEP] 1987 年(昭和 62 年)4 月 1 日の国鉄分割民営化により、JR 東海が運営を継承した。西日本旅客鉄道(JR 西日本)が継承した山陽新幹線とは相互乗り入れが行われており、東海道新幹線区間のみで運転される列車にも JR 西日本所有の車両が使用されることがある。2020 年(令和 2 年)3 月現在、東京駅 - 新大阪駅間の所要時間は最速 2 時間 21 分、最高速度 285 km/h で運行されている。",
"question": "2020 年(令和 2 年)3 月現在、東京駅 - 新大阪駅間の最高速度はどのくらいか。",
"answers": {
"text": ["285 km/h"],
"answer_start": [182]
},
"is_impossible": false
}
JCommonsenseQA
from datasets import load_dataset
dataset = load_dataset("shunk031/JGLUE", name="JCommonsenseQA")
print(dataset)
# DatasetDict({
# train: Dataset({
# features: ['q_id', 'question', 'choice0', 'choice1', 'choice2', 'choice3', 'choice4', 'label'],
# num_rows: 8939
# })
# validation: Dataset({
# features: ['q_id', 'question', 'choice0', 'choice1', 'choice2', 'choice3', 'choice4', 'label'],
# num_rows: 1119
# })
# })
An example of the JCommonsenseQA looks as follows:
{
"q_id": 3016,
"question": "会社の最高責任者を何というか? (What do you call the chief executive officer of a company?)",
"choice0": "社長 (president)",
"choice1": "教師 (teacher)",
"choice2": "部長 (manager)",
"choice3": "バイト (part-time worker)",
"choice4": "部下 (subordinate)",
"label": 0
}
Data Fields
MARC-ja
sentence_pair_id
: ID of the sentence pairyjcaptions_id
: sentence ids in yjcaptions (explained below)sentence1
: first sentencesentence2
: second sentencelabel
: sentence similarity: 5 (equivalent meaning) - 0 (completely different meaning)
Explanation for yjcaptions_id
From JGLUE's README.md, there are the following two cases:
- sentence pairs in one image:
(image id)-(sentence1 id)-(sentence2 id)
- e.g., 723-844-847
- a sentence id starting with "g" means a sentence generated by a crowdworker (e.g., 69501-75698-g103): only for JNLI
- sentence pairs in two images:
(image id of sentence1)_(image id of sentence2)-(sentence1 id)-(sentence2 id)
- e.g., 91337_217583-96105-91680
JCoLA
From JCoLA's README.md and JCoLA's paper
uid
: unique id of the sentencesource
: author and the year of publication of the source articlelabel
: acceptability judgement label (0 for unacceptable, 1 for acceptable)diacritic
: acceptability judgement as originally notated in the source articlesentence
: sentence (modified by the author if needed)original
: original sentence as presented in the source articletranslation
: English translation of the sentence as presentend in the source article (if any)gloss
: gloss of the sentence as presented in the source article (if any)linguistic_phenomenon
argument_structure
: acceptability judgements based on the order of arguments and case markingbinding
: acceptability judgements based on the binding of noun phrasescontrol_raising
: acceptability judgements based on predicates that are categorized as control or raisingellipsis
: acceptability judgements based on the possibility of omitting elements in the sentencesfiller_gap
: acceptability judgements based on the dependency between the moved element and the gapisland effects
: acceptability judgements based on the restrictions on filler-gap dependencies such as wh-movementsmorphology
: acceptability judgements based on the morphologynominal_structure
: acceptability judgements based on the internal structure of noun phrasesnegative_polarity_concord_items
: acceptability judgements based on the restrictions on where negative polarity/concord items (NPIs/NCIs) can appearquantifiers
: acceptability judgements based on the distribution of quantifiers such as floating quantifiersverbal_agreement
: acceptability judgements based on the dependency between subjects and verbssimple
: acceptability judgements that do not have marked syntactic structures
JNLI
sentence_pair_id
: ID of the sentence pairyjcaptions_id
: sentence ids in the yjcaptionssentence1
: premise sentencesentence2
: hypothesis sentencelabel
: inference relation
JSQuAD
title
: title of a Wikipedia articleparagraphs
: a set of paragraphsqas
: a set of pairs of a question and its answerquestion
: questionid
: id of a questionanswers
: a set of answerstext
: answer textanswer_start
: start position (character index)is_impossible
: all the values are falsecontext
: a concatenation of the title and paragraph
JCommonsenseQA
q_id
: ID of the questionquestion
: questionchoice{0..4}
: choicelabel
: correct choice id
Data Splits
From JGLUE's README.md:
Only train/dev sets are available now, and the test set will be available after the leaderboard is made public.
From JCoLA's paper:
The in-domain data is split into training data (6,919 instances), development data (865 instances), and test data (865 instances). On the other hand, the out-of-domain data is only used for evaluation, and divided into development data (685 instances) and test data (686 instances).
Task | Dataset | Train | Dev | Test |
---|---|---|---|---|
Text Classification | MARC-ja | 187,528 | 5,654 | 5,639 |
JCoLA | 6,919 | 865† / 685‡ | 865† / 685‡ | |
Sentence Pair Classification | JSTS | 12,451 | 1,457 | 1,589 |
JNLI | 20,073 | 2,434 | 2,508 | |
Question Answering | JSQuAD | 62,859 | 4,442 | 4,420 |
JCommonsenseQA | 8,939 | 1,119 | 1,118 |
JCoLA: † in domain. ‡ out of domain.
Dataset Creation
Curation Rationale
From JGLUE's paper:
JGLUE is designed to cover a wide range of GLUE and SuperGLUE tasks and consists of three kinds of tasks: text classification, sentence pair classification, and question answering.
Source Data
Initial Data Collection and Normalization
[More Information Needed]
Who are the source language producers?
- The source language producers are users of Amazon (MARC-ja), crowd-workers of Yahoo! Crowdsourcing (JSTS, JNLI and JCommonsenseQA), writers of the Japanese Wikipedia (JSQuAD), crowd-workers of Lancers.
Annotations
Annotation process
MARC-ja
From JGLUE's paper:
As one of the text classification datasets, we build a dataset based on the Multilingual Amazon Reviews Corpus (MARC) (Keung et al., 2020). MARC is a multilingual corpus of product reviews with 5-level star ratings (1-5) on the Amazon shopping site. This corpus covers six languages, including English and Japanese. For JGLUE, we use the Japanese part of MARC and to make it easy for both humans and computers to judge a class label, we cast the text classification task as a binary classification task, where 1- and 2-star ratings are converted to “negative”, and 4 and 5 are converted to “positive”. We do not use reviews with a 3-star rating.
One of the problems with MARC is that it sometimes contains data where the rating diverges from the review text. This happens, for example, when a review with positive content is given a rating of 1 or 2. These data degrade the quality of our dataset. To improve the quality of the dev/test instances used for evaluation, we crowdsource a positive/negative judgment task for approximately 12,000 reviews. We adopt only reviews with the same votes from 7 or more out of 10 workers and assign a label of the maximum votes to these reviews. We divide the resulting reviews into dev/test data.
We obtained 5,654 and 5,639 instances for the dev and test data, respectively, through the above procedure. For the training data, we extracted 187,528 instances directly from MARC without performing the cleaning procedure because of the large number of training instances. The statistics of MARC-ja are listed in Table 2. For the evaluation metric for MARC-ja, we use accuracy because it is a binary classification task of texts.
JCoLA
From JCoLA's paper:
3 JCoLA
In this study, we introduce JCoLA (Japanese Corpus of Linguistic Acceptability), which will be the first large-scale acceptability judgment task dataset focusing on Japanese. JCoLA consists of sentences from textbooks and handbooks on Japanese syntax, as well as from journal articles on Japanese syntax that are published in JEAL (Journal of East Asian Linguistics), one of the prestigious journals in theoretical linguistics.
3.1 Data Collection
Sentences in JCoLA were collected from prominent textbooks and handbooks focusing on Japanese syntax. In addition to the main text, example sentences included in the footnotes were also considered for collection. We also collected acceptability judgments from journal articles on Japanese syntax published in JEAL (Journal of East Asian Linguistics): one of the prestigious journals in the-oretical linguistics. Specifically, we examined all the articles published in JEAL between 2006 and 2015 (133 papers in total), and extracted 2,252 acceptability judgments from 26 papers on Japanese syntax (Table 2). Acceptability judgments include sentences in appendices and footnotes, but not sentences presented for analyses of syntactic structures (e.g. sentences with brackets to show their syntactic structures). As a result, a total of 11,984 example. sentences were collected. Using this as a basis, JCoLA was constructed through the methodology explained in the following sections.
JSTS and JNLI
From JGLUE's paper:
For the sentence pair classification datasets, we construct a semantic textual similarity (STS) dataset, JSTS, and a natural language inference (NLI) dataset, JNLI.
Overview
STS is a task of estimating the semantic similarity of a sentence pair. Gold similarity is usually assigned as an average of the integer values 0 (completely different meaning) to 5 (equivalent meaning) assigned by multiple workers through crowdsourcing.
NLI is a task of recognizing the inference relation that a premise sentence has to a hypothesis sentence. Inference relations are generally defined by three labels: “entailment”, “contradiction”, and “neutral”. Gold inference relations are often assigned by majority voting after collecting answers from multiple workers through crowdsourcing.
For the STS and NLI tasks, STS-B (Cer et al., 2017) and MultiNLI (Williams et al., 2018) are included in GLUE, respectively. As Japanese datasets, JSNLI (Yoshikoshi et al., 2020) is a machine translated dataset of the NLI dataset SNLI (Stanford NLI), and JSICK (Yanaka and Mineshima, 2021) is a human translated dataset of the STS/NLI dataset SICK (Marelli et al., 2014). As mentioned in Section 1, these have problems originating from automatic/manual translations. To solve this problem, we construct STS/NLI datasets in Japanese from scratch. We basically extract sentence pairs in JSTS and JNLI from the Japanese version of the MS COCO Caption Dataset (Chen et al., 2015), the YJ Captions Dataset (Miyazaki and Shimizu, 2016). Most of the sentence pairs in JSTS and JNLI overlap, allowing us to analyze the relationship between similarities and inference relations for the same sentence pairs like SICK and JSICK.
The similarity value in JSTS is assigned a real number from 0 to 5 as in STS-B. The inference relation in JNLI is assigned from the above three labels as in SNLI and MultiNLI. The definitions of the inference relations are also based on SNLI.
Method of Construction
Our construction flow for JSTS and JNLI is shown in Figure 1. Basically, two captions for the same image of YJ Captions are used as sentence pairs. For these sentence pairs, similarities and NLI relations of entailment and neutral are obtained by crowdsourcing. However, it is difficult to collect sentence pairs with low similarity and contradiction relations from captions for the same image. To solve this problem, we collect sentence pairs with low similarity from captions for different images. We collect contradiction relations by asking workers to write contradictory sentences for a given caption.
The detailed construction procedure for JSTS and JNLI is described below.
- We crowdsource an STS task using two captions for the same image from YJ Captions. We ask five workers to answer the similarity between two captions and take the mean value as the gold similarity. We delete sentence pairs with a large variance in the answers because such pairs have poor answer quality. We performed this task on 16,000 sentence pairs and deleted sentence pairs with a similarity variance of 1.0 or higher, resulting in the collection of 10,236 sentence pairs with gold similarity. We refer to this collected data as JSTS-A.
- To collect sentence pairs with low similarity, we crowdsource the same STS task as Step 1 using sentence pairs of captions for different images. We conducted this task on 4,000 sentence pairs and collected 2,970 sentence pairs with gold similarity. We refer to this collected data as JSTS-B.
- For JSTS-A, we crowdsource an NLI task. Since inference relations are directional, we obtain inference relations in both directions for sentence pairs. As mentioned earlier,it is difficult to collect instances of contradiction from JSTS-A, which was collected from the captions of the same images,and thus we collect instances of entailment and neutral in this step. We collect inference relation answers from 10 workers. If six or more people give the same answer, we adopt it as the gold label if it is entailment or neutral. To obtain inference relations in both directions for JSTS-A, we performed this task on 20,472 sentence pairs, twice as many as JSTS-A. As a result, we collected inference relations for 17,501 sentence pairs. We refer to this collected data as JNLI-A. We do not use JSTS-B for the NLI task because it is difficult to define and determine the inference relations between captions of different images.
- To collect NLI instances of contradiction, we crowdsource a task of writing four contradictory sentences for each caption in YJCaptions. From the written sentences, we remove sentence pairs with an edit distance of 0.75 or higher to remove low-quality sentences, such as short sentences and sentences with low relevance to the original sentence. Furthermore, we perform a one-way NLI task with 10 workers to verify whether the created sentence pairs are contradictory. Only the sentence pairs answered as contradiction by at least six workers are adopted. Finally,since the contradiction relation has no direction, we automatically assign contradiction in the opposite direction of the adopted sentence pairs. Using 1,800 captions, we acquired 7,200 sentence pairs, from which we collected 3,779 sentence pairs to which we assigned the one-way contradiction relation.By automatically assigning the contradiction relation in the opposite direction, we doubled the number of instances to 7,558. We refer to this collected data as JNLI-C.
- For the 3,779 sentence pairs collected in Step 4, we crowdsource an STS task, assigning similarity and filtering in the same way as in Steps1 and 2. In this way, we collected 2,303 sentence pairs with gold similarity from 3,779 pairs. We refer to this collected data as JSTS-C.
JSQuAD
From JGLUE's paper:
As QA datasets, we build a Japanese version of SQuAD (Rajpurkar et al., 2016), one of the datasets of reading comprehension, and a Japanese version ofCommonsenseQA, which is explained in the next section.
Reading comprehension is the task of reading a document and answering questions about it. Many reading comprehension evaluation sets have been built in English, followed by those in other languages or multilingual ones.
In Japanese, reading comprehension datasets for quizzes (Suzukietal.,2018) and those in the drivingdomain (Takahashi et al., 2019) have been built, but none are in the general domain. We use Wikipedia to build a dataset for the general domain. The construction process is basically based on SQuAD 1.1 (Rajpurkar et al., 2016).
First, to extract high-quality articles from Wikipedia, we use Nayuki, which estimates the quality of articles on the basis of hyperlinks in Wikipedia. We randomly chose 822 articles from the top-ranked 10,000 articles. For example, the articles include “熊本県 (Kumamoto Prefecture)” and “フランス料理 (French cuisine)”. Next, we divide an article into paragraphs, present each paragraph to crowdworkers, and ask them to write questions and answers that can be answered if one understands the paragraph. Figure 2 shows an example of JSQuAD. We ask workers to write two additional answers for the dev and test sets to make the system evaluation robust.
JCommonsenseQA
From JGLUE's paper:
Overview
JCommonsenseQA is a Japanese version of CommonsenseQA (Talmor et al., 2019), which consists of five choice QA to evaluate commonsense reasoning ability. Figure 3 shows examples of JCommonsenseQA. In the same way as CommonsenseQA, JCommonsenseQA is built using crowdsourcing with seeds extracted from the knowledge base ConceptNet (Speer et al., 2017). ConceptNet is a multilingual knowledge base that consists of triplets of two concepts and their relation. The triplets are directional and represented as (source concept, relation, target concept), for example (bullet train, AtLocation, station).
Method of Construction
The construction flow for JCommonsenseQA is shown in Figure 4. First, we collect question sets (QSs) from ConceptNet, each of which consists of a source concept and three target concepts that have the same relation to the source concept. Next, for each QS, we crowdAtLocation 2961source a task of writing a question with only one target concept as the answer and a task of adding two distractors. We describe the detailed construction procedure for JCommonsenseQA below, showing how it differs from CommonsenseQA.
- We collect Japanese QSs from ConceptNet. CommonsenseQA uses only forward relations (source concept, relation, target concept) excluding general ones such as “RelatedTo” and “IsA”. JCommonsenseQA similarly uses a set of 22 relations5, excluding general ones, but the direction of the relations is bidirectional to make the questions more diverse. In other words, we also use relations in the opposite direction (source concept, relation−1, target concept).6 With this setup, we extracted 43,566 QSs with Japanese source/target concepts and randomly selected 7,500 from them.
- Some low-quality questions in CommonsenseQA contain distractors that can be considered to be an answer. To improve the quality of distractors, we add the following two processes that are not adopted in CommonsenseQA. First, if three target concepts of a QS include a spelling variation or a synonym of one another, this QS is removed. To identify spelling variations, we use the word ID of the morphological dictionary Juman Dic7. Second, we crowdsource a task of judging whether target concepts contain a synonym. As a result, we adopted 5,920 QSs from 7,500.
- For each QS, we crowdsource a task of writing a question sentence in which only one from the three target concepts is an answer. In the example shown in Figure 4, “駅 (station)” is an answer, and the others are distractors. To remove low quality question sentences, we remove the following question sentences.
- Question sentences that contain a choice word(this is because such a question is easily solved).
- Question sentences that contain the expression “XX characters”.8 (XX is a number).
- Improperly formatted question sentences that do not end with “?”.
- As a result, 5,920 × 3 = 17,760question sentences were created, from which we adopted 15,310 by removing inappropriate question sentences.
- In CommonsenseQA, when adding distractors, one is selected from ConceptNet, and the other is created by crowdsourcing. In JCommonsenseQA, to have a wider variety of distractors, two distractors are created by crowdsourcing instead of selecting from ConceptNet. To improve the quality of the questions9, we remove questions whose added distractors fall into one of the following categories:
- Distractors are included in a question sentence.
- Distractors overlap with one of existing choices.
- As a result, distractors were added to the 15,310 questions, of which we adopted 13,906.
- We asked three crowdworkers to answer each question and adopt only those answered correctly by at least two workers. As a result, we adopted 11,263 out of the 13,906 questions.
Who are the annotators?
From JGLUE's README.md:
We use Yahoo! Crowdsourcing for all crowdsourcing tasks in constructing the datasets.
From JCoLA's paper:
As a reference for the upper limit of accuracy in JCoLA, human acceptability judgment experiments were conducted on Lancers2 with a subset of the JCoLA data.
Personal and Sensitive Information
[More Information Needed]
Considerations for Using the Data
Social Impact of Dataset
From JGLUE's paper:
We build a Japanese NLU benchmark, JGLUE, from scratch without translation to measure the general NLU ability in Japanese. We hope that JGLUE will facilitate NLU research in Japanese.
Discussion of Biases
[More Information Needed]
Other Known Limitations
From JCoLA's paper:
All the sentences included in JCoLA have been extracted from textbooks, handbooks and journal articles on theoretical syntax. Therefore, those sentences are guaranteed to be theoretically meaningful, making JCoLA a challenging dataset. However, the distribution of linguistic phenomena directly reflects that of the source literature and thus turns out to be extremely skewed. Indeed, as can be seen in Table 3, while the number of sentences exceeds 100 for most linguistic phenomena, there are several linguistic phenomena for which there are only about 10 sentences. In addition, since it is difficult to force language models to interpret sentences given specific contexts, those sentences whose unacceptability depends on contexts were inevitably removed from JCoLA. This removal process resulted in the deletion of unacceptable sentences from some linguistic phenomena (such as ellipsis), consequently skewing the balance between acceptable and unacceptable sentences (with a higher proportion of acceptable sentences).
Additional Information
- 日本語言語理解ベンチマーク JGLUE の構築 〜 自然言語処理モデルの評価用データセットを公開しました - Yahoo! JAPAN Tech Blog https://techblog.yahoo.co.jp/entry/2022122030379907/
Dataset Curators
MARC-ja
- Keung, Phillip, et al. "The Multilingual Amazon Reviews Corpus." Proceedings of the 2020 Conference on Empirical Methods in Natural Language Processing (EMNLP). 2020.
JCoLA
- Someya, Sugimoto, and Oseki. "JCoLA: Japanese Corpus of Linguistic Acceptability." arxiv preprint arXiv:2309.12676 (2023).
JSTS and JNLI
- Miyazaki, Takashi, and Nobuyuki Shimizu. "Cross-lingual image caption generation." Proceedings of the 54th Annual Meeting of the Association for Computational Linguistics (Volume 1: Long Papers). 2016.
JSQuAD
The JGLUE's 'authors curated the original data for JSQuAD from the Japanese wikipedia dump.
JCommonsenseQA
In the same way as CommonsenseQA, JCommonsenseQA is built using crowdsourcing with seeds extracted from the knowledge base ConceptNet
Licensing Information
JGLUE
From JGLUE's README.md':
This work is licensed under a Creative Commons Attribution-ShareAlike 4.0 International License.
JCoLA
From JCoLA's README.md':
The text in this corpus is excerpted from the published works, and copyright (where applicable) remains with the original authors or publishers. We expect that research use within Japan is legal under fair use, but make no guarantee of this.
Citation Information
JGLUE
@inproceedings{kurihara-lrec-2022-jglue,
title={JGLUE: Japanese general language understanding evaluation},
author={Kurihara, Kentaro and Kawahara, Daisuke and Shibata, Tomohide},
booktitle={Proceedings of the Thirteenth Language Resources and Evaluation Conference},
pages={2957--2966},
year={2022},
url={https://aclanthology.org/2022.lrec-1.317/}
}
@inproceedings{kurihara-nlp-2022-jglue,
title={JGLUE: 日本語言語理解ベンチマーク},
author={栗原健太郎 and 河原大輔 and 柴田知秀},
booktitle={言語処理学会第 28 回年次大会},
pages={2023--2028},
year={2022},
url={https://www.anlp.jp/proceedings/annual_meeting/2022/pdf_dir/E8-4.pdf},
note={in Japanese}
}
MARC-ja
@inproceedings{marc_reviews,
title={The Multilingual Amazon Reviews Corpus},
author={Keung, Phillip and Lu, Yichao and Szarvas, György and Smith, Noah A.},
booktitle={Proceedings of the 2020 Conference on Empirical Methods in Natural Language Processing},
year={2020}
}
JCoLA
@article{someya-arxiv-2023-jcola,
title={JCoLA: Japanese Corpus of Linguistic Acceptability},
author={Taiga Someya and Yushi Sugimoto and Yohei Oseki},
year={2023},
eprint={2309.12676},
archivePrefix={arXiv},
primaryClass={cs.CL}
}
@inproceedings{someya-nlp-2022-jcola,
title={日本語版 CoLA の構築},
author={染谷 大河 and 大関 洋平},
booktitle={言語処理学会第 28 回年次大会},
pages={1872--1877},
year={2022},
url={https://www.anlp.jp/proceedings/annual_meeting/2022/pdf_dir/E7-1.pdf},
note={in Japanese}
}
JSTS and JNLI
@inproceedings{miyazaki2016cross,
title={Cross-lingual image caption generation},
author={Miyazaki, Takashi and Shimizu, Nobuyuki},
booktitle={Proceedings of the 54th Annual Meeting of the Association for Computational Linguistics (Volume 1: Long Papers)},
pages={1780--1790},
year={2016}
}
Contributions
Thanks to Kentaro Kurihara, Daisuke Kawahara, and Tomohide Shibata for creating JGLUE dataset. Thanks to Taiga Someya for creating JCoLA dataset.
- Downloads last month
- 11,233
Models trained or fine-tuned on shunk031/JGLUE
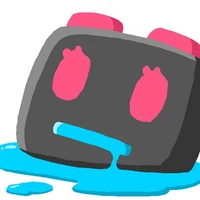