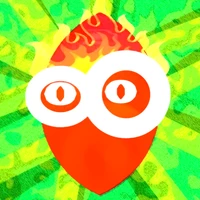
Datasets:
The BioLORD Dataset (v1)
This dataset was constructed to enable training text embedding models producing similar representations for biomedical concept names and their definitions. Pairs of biomedical concepts names and descriptions of the concept are contrasted against each other, such that the model becomes able to find which names and descriptions are paired together within a batch.
Citation
This dataset accompanies the BioLORD: Learning Ontological Representations from Definitions paper, accepted in the EMNLP 2022 Findings. When you use this dataset, please cite the original paper as follows:
@inproceedings{remy-etal-2022-biolord,
title = "{B}io{LORD}: Learning Ontological Representations from Definitions for Biomedical Concepts and their Textual Descriptions",
author = "Remy, François and
Demuynck, Kris and
Demeester, Thomas",
booktitle = "Findings of the Association for Computational Linguistics: EMNLP 2022",
month = dec,
year = "2022",
address = "Abu Dhabi, United Arab Emirates",
publisher = "Association for Computational Linguistics",
url = "https://aclanthology.org/2022.findings-emnlp.104",
pages = "1454--1465",
abstract = "This work introduces BioLORD, a new pre-training strategy for producing meaningful representations for clinical sentences and biomedical concepts. State-of-the-art methodologies operate by maximizing the similarity in representation of names referring to the same concept, and preventing collapse through contrastive learning. However, because biomedical names are not always self-explanatory, it sometimes results in non-semantic representations. BioLORD overcomes this issue by grounding its concept representations using definitions, as well as short descriptions derived from a multi-relational knowledge graph consisting of biomedical ontologies. Thanks to this grounding, our model produces more semantic concept representations that match more closely the hierarchical structure of ontologies. BioLORD establishes a new state of the art for text similarity on both clinical sentences (MedSTS) and biomedical concepts (MayoSRS).",
}
Contents
The dataset contains 100M pairs (86M with descriptions, 14M with definitions).
📝 Example of definitions:
- Site Training Documentation (Document type): Document type described as records that verify completion of clinical trial site training for the site medical investigator and his/her staff.
- Arteries, Gastric (Arteries): Arteries described as either of two arteries (left gastric and right gastric) that supply blood to the stomach and lesser curvature.
- Dental Materials, Cement, Zinc Phosphate (Biomedical or Dental Material): Biomedical or Dental Material described as cement dental materials, whose main components are phosphoric acid and zinc oxide, designed to produce a mechanical interlocking effect upon hardening inside the mouth. These cements consist of a basic powder (zinc oxide), an acidic liquid (phosphoric acid), and water that are mixed together in a viscous paste immediately before use, setting to a hard mass. Zinc phosphate cements have proper thermal and chemical resistance in the oral environment; they also should be resistant to dissolution in oral fluids. Zinc phosphate cements must be placed on a dental cavity liner or sealer to avoid pulp irritation. They are used in dentists' offices as cementing medium of inlays, crowns, bridges and orthodontic appliances (e.g., bands, brackets), as intermediate bases, or as temporary restorative materials.
- DTI (Diffusion weighted imaging): Diffusion weighted imaging described as a type of diffusion-weighted magnetic resonance imaging (DW-MRI) that maps the diffusion of water in three dimensions, the principal purpose of which is to image the white matter of the brain, specifically measuring the anisotropy, location, and orientation of the neural tracts, which can demonstrate microstructural changes or differences with neuropathology and treatment.
- arousal (psychic activity level): Nervous System Physiological Phenomena described as cortical vigilance or readiness of tone, presumed to be in response to sensory stimulation via the reticular activating system.
📝 Example of descriptions:
- Mesial fovea (Body Space or Junction): something which is a Region of surface of organ
- Thyroid associated opthalmopathies (Disease or Syndrome): something which has finding site orbit
- Internal fixation of bone of radius (Therapeutic or Preventive Procedure): SHOULDER AND ARM: SURGICAL REPAIRS, CLOSURES AND RECONSTRUCTIONS which has method Fixation - action
- gardnerella (Gram-variable bacterium): something which is a Gram-variable coccobacillus
- Hydropane (Organic Chemical): Organic Chemical which is ingredient of homatropine / hydrocodone Oral Solution [Hydropane]
- Duane anomaly, myopathy, scoliosis syndrome (Multiple system malformation syndrome): Scoliosis, unspecified which has finding site Nervous system structure
Another set of 20M descriptions based on the same knowledge graph serves as a development set (86M generations certainly do not exhaust the graph). However, this would not be a suitable test set. Instead, a test of time consisting of new concepts currently absent from UMLS would make more sense, but this will have to wait until enough new concepts have been added to UMLS.
License
My own contributions for this dataset are covered by the MIT license. However, given the data used to generate this dataset originates from UMLS, you will need to ensure you have proper licensing of UMLS before using this dataset. UMLS is free of charge in most countries, but you might have to create an account and report on your usage of the data yearly to keep a valid license.
- Downloads last month
- 38
Models trained or fine-tuned on FremyCompany/BioLORD-Dataset
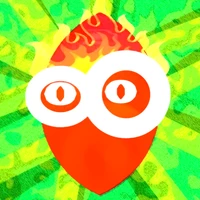