cucunaga
cucunaga
AI & ML interests
None yet
Recent Activity
View all activity
Organizations
cucunaga's activity
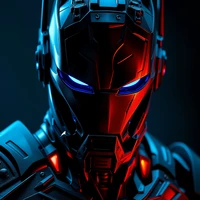
reacted to
OFT's
post with 😔👍👀
21 days ago
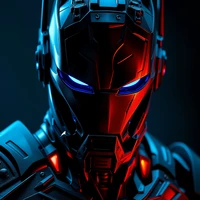
reacted to
danielhanchen's
post with 🔥👀❤️
21 days ago
Post
3352
You can now run DeepSeek-V3-0324 on your own local device!
Run our Dynamic 2.42 and 2.71-bit DeepSeek GGUFs: unsloth/DeepSeek-V3-0324-GGUF
You can run them on llama.cpp and other inference engines. See our guide here: https://docs.unsloth.ai/basics/tutorial-how-to-run-deepseek-v3-0324-locally
Run our Dynamic 2.42 and 2.71-bit DeepSeek GGUFs: unsloth/DeepSeek-V3-0324-GGUF
You can run them on llama.cpp and other inference engines. See our guide here: https://docs.unsloth.ai/basics/tutorial-how-to-run-deepseek-v3-0324-locally
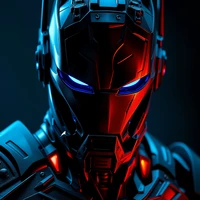
reacted to
MonsterMMORPG's
post with 🤗❤️🔥
21 days ago
Post
2190
I have Compared Kohya vs OneTrainer for FLUX Dev Finetuning / DreamBooth Training
OneTrainer can train FLUX Dev with Text-Encoders unlike Kohya so I wanted to try it.
Unfortunately, the developer doesn't want to add feature to save trained Clip L or T5 XXL as safetensors or merge them into output so basically they are useless without so much extra effort.
I still went ahead and wanted to test EMA training. EMA normally improves quality significantly in SD 1.5 training. With FLUX I have to use CPU for EMA and it was really slow but i wanted to test.
I have tried to replicate Kohya config. The below you will see results. Sadly the quality is nothing sort of. More research has to be made and since we still don't get text-encoder training due to developer decision, I don't see any benefit of using OneTrainer for FLUX training instead of using Koha.
1st image : Kohya best config : https://www.patreon.com/posts/112099700
2nd image : One Trainer Kohya config with EMA update every 1 step
3rd image : One Trainer Kohya config with EMA update every 5 steps
4th image : One Trainer Kohya config
5th image : One Trainer Kohya config but Timestep Shift is 1 instead of 3.1582
I am guessing that Timestep Shift of OneTrainer is not same as Discrete Flow Shift of Kohya
Probably I need to work and do more test and i can improve results but i don't see any reason to do atm. If Clip Training + merging it into safetensors file was working, I was gonna pursue it
These are not cherry pick results all are from 1st test grid
OneTrainer can train FLUX Dev with Text-Encoders unlike Kohya so I wanted to try it.
Unfortunately, the developer doesn't want to add feature to save trained Clip L or T5 XXL as safetensors or merge them into output so basically they are useless without so much extra effort.
I still went ahead and wanted to test EMA training. EMA normally improves quality significantly in SD 1.5 training. With FLUX I have to use CPU for EMA and it was really slow but i wanted to test.
I have tried to replicate Kohya config. The below you will see results. Sadly the quality is nothing sort of. More research has to be made and since we still don't get text-encoder training due to developer decision, I don't see any benefit of using OneTrainer for FLUX training instead of using Koha.
1st image : Kohya best config : https://www.patreon.com/posts/112099700
2nd image : One Trainer Kohya config with EMA update every 1 step
3rd image : One Trainer Kohya config with EMA update every 5 steps
4th image : One Trainer Kohya config
5th image : One Trainer Kohya config but Timestep Shift is 1 instead of 3.1582
I am guessing that Timestep Shift of OneTrainer is not same as Discrete Flow Shift of Kohya
Probably I need to work and do more test and i can improve results but i don't see any reason to do atm. If Clip Training + merging it into safetensors file was working, I was gonna pursue it
These are not cherry pick results all are from 1st test grid
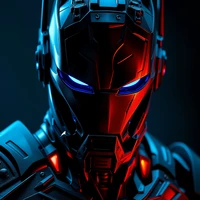
reacted to
clem's
post with 🔥
21 days ago
Post
3983
Before 2020, most of the AI field was open and collaborative. For me, that was the key factor that accelerated scientific progress and made the impossible possible—just look at the “T” in ChatGPT, which comes from the Transformer architecture openly shared by Google.
Then came the myth that AI was too dangerous to share, and companies started optimizing for short-term revenue. That led many major AI labs and researchers to stop sharing and collaborating.
With OAI and sama now saying they're willing to share open weights again, we have a real chance to return to a golden age of AI progress and democratization—powered by openness and collaboration, in the US and around the world.
This is incredibly exciting. Let’s go, open science and open-source AI!
Then came the myth that AI was too dangerous to share, and companies started optimizing for short-term revenue. That led many major AI labs and researchers to stop sharing and collaborating.
With OAI and sama now saying they're willing to share open weights again, we have a real chance to return to a golden age of AI progress and democratization—powered by openness and collaboration, in the US and around the world.
This is incredibly exciting. Let’s go, open science and open-source AI!
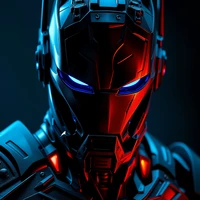
reacted to
Reality123b's
post with 👍
21 days ago
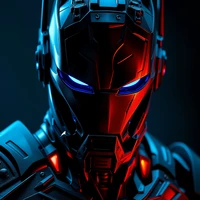
reacted to
ZhiyuanthePony's
post with 🤗
21 days ago
Post
2581
🎉 Thrilled to share our #CVPR2025 accepted work:
Progressive Rendering Distillation: Adapting Stable Diffusion for Instant Text-to-Mesh Generation without 3D Data (2503.21694)
🔥 Key Innovations:
1️⃣ First to adapt SD for direct textured mesh generation (1-2s inference)
2️⃣ Novel teacher-student framework leveraging multi-view diffusion models ([MVDream](https://arxiv.org/abs/2308.16512) & [RichDreamer](https://arxiv.org/abs/2311.16918))
3️⃣ Parameter-efficient tuning - only +2.6% params over base SD
4️⃣ 3D data-free training liberates model from dataset constraints
💡 Why matters?
→ A novel 3D-Data-Free paradigm
→ Outperforms data-driven methods on creative concept generation
→ Unlocks web-scale text corpus for 3D content creation
🌐 Project: https://theericma.github.io/TriplaneTurbo/
🎮 Demo: ZhiyuanthePony/TriplaneTurbo
💻 Code: https://github.com/theEricMa/TriplaneTurbo
Progressive Rendering Distillation: Adapting Stable Diffusion for Instant Text-to-Mesh Generation without 3D Data (2503.21694)
🔥 Key Innovations:
1️⃣ First to adapt SD for direct textured mesh generation (1-2s inference)
2️⃣ Novel teacher-student framework leveraging multi-view diffusion models ([MVDream](https://arxiv.org/abs/2308.16512) & [RichDreamer](https://arxiv.org/abs/2311.16918))
3️⃣ Parameter-efficient tuning - only +2.6% params over base SD
4️⃣ 3D data-free training liberates model from dataset constraints
💡 Why matters?
→ A novel 3D-Data-Free paradigm
→ Outperforms data-driven methods on creative concept generation
→ Unlocks web-scale text corpus for 3D content creation
🌐 Project: https://theericma.github.io/TriplaneTurbo/
🎮 Demo: ZhiyuanthePony/TriplaneTurbo
💻 Code: https://github.com/theEricMa/TriplaneTurbo
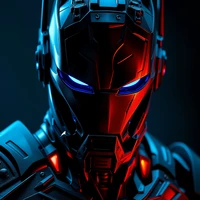
reacted to
ZennyKenny's
post with 👍
21 days ago
Post
2125
A few new Russian-language synthetic datasets. The labelling is good, but some of the syntax and grammar is not great.
Great for Russian-language classification models, probably not great for fine-tuning Russian-langauge text generation.
- Virtual Assistant Query / Responses: ZennyKenny/ru_virtual_assistant_chatgpt_distill
- LLM Query / Responses: ZennyKenny/russian_llm_response_chatgpt_distill
Crazy how much language drift is still an issue, especially given that Russian constitutes nearly 5% of the content on the internet.
Great for Russian-language classification models, probably not great for fine-tuning Russian-langauge text generation.
- Virtual Assistant Query / Responses: ZennyKenny/ru_virtual_assistant_chatgpt_distill
- LLM Query / Responses: ZennyKenny/russian_llm_response_chatgpt_distill
Crazy how much language drift is still an issue, especially given that Russian constitutes nearly 5% of the content on the internet.
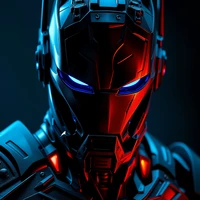
reacted to
Smooke's
post with 👀
21 days ago
Post
1731
AI Search Traffic Marketshare for Calling HackerNoon Blogs: 52% OpenAI, 30% Amazon & 18% Perplexity: https://hackernoon.com/ai-search-traffic-marketshare-for-calling-hackernoon-blogs-52percent-openai-30percent-amazon-and-18percent-perplexity
OpenAI (51.8%) leads AI search traffic market share, based on my analysis of end-user–initiated AI Assistant and AI Search requests to HackerNoon. While Amazon (30.4%) and Perplexity (17.9%) also secured significant portions of the market, the total volume of requests (1,915,670 in 30 days) and competition among AI search providers indicate increasing reliance on AI for information retrieval and presentation.
This analysis aggregates AI Assistant and AI Search queries to approximate end-user–initiated AI search traffic across HackerNoon URLs. Non-human traffic such as web crawlers, bots, and automated scripts have been filtered out to ensure data reflects only human-initiated requests. The dataset reviewed comprises instances where AI systems recommended HackerNoon content in response to human queries. Between February 28 and March 28, 2025, HackerNoon received 1,915,670 AI-referred search requests. OpenAI accounted for 991,580 requests, Amazon accounted for 581,990 requests , and Perplexity accounted for 342,100 requests, according to Cloudflare AI Audit tool, which currently tracks these top providers. HackerNoon is a technical audience, so our data is better positioned to answer questions like, if you work in tech what AI search engine do you rely on?
Continue Reading... https://hackernoon.com/ai-search-traffic-marketshare-for-calling-hackernoon-blogs-52percent-openai-30percent-amazon-and-18percent-perplexity
OpenAI (51.8%) leads AI search traffic market share, based on my analysis of end-user–initiated AI Assistant and AI Search requests to HackerNoon. While Amazon (30.4%) and Perplexity (17.9%) also secured significant portions of the market, the total volume of requests (1,915,670 in 30 days) and competition among AI search providers indicate increasing reliance on AI for information retrieval and presentation.
This analysis aggregates AI Assistant and AI Search queries to approximate end-user–initiated AI search traffic across HackerNoon URLs. Non-human traffic such as web crawlers, bots, and automated scripts have been filtered out to ensure data reflects only human-initiated requests. The dataset reviewed comprises instances where AI systems recommended HackerNoon content in response to human queries. Between February 28 and March 28, 2025, HackerNoon received 1,915,670 AI-referred search requests. OpenAI accounted for 991,580 requests, Amazon accounted for 581,990 requests , and Perplexity accounted for 342,100 requests, according to Cloudflare AI Audit tool, which currently tracks these top providers. HackerNoon is a technical audience, so our data is better positioned to answer questions like, if you work in tech what AI search engine do you rely on?
Continue Reading... https://hackernoon.com/ai-search-traffic-marketshare-for-calling-hackernoon-blogs-52percent-openai-30percent-amazon-and-18percent-perplexity
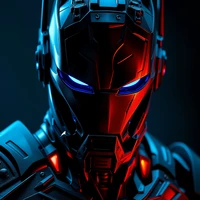
reacted to
DualityAI-RebekahBogdanoff's
post with ❤️
21 days ago
Post
2559
Curious how Duality AI crafts synthetic data that can bridge the sim2real gap?
We just published an article here on HuggingFace outlining our process, with bonus dataset releases! Read it here: https://huggingface.co/blog/DualityAI-RebekahBogdanoff/training-yolov8-with-synthetic-data-from-falcon
We just published an article here on HuggingFace outlining our process, with bonus dataset releases! Read it here: https://huggingface.co/blog/DualityAI-RebekahBogdanoff/training-yolov8-with-synthetic-data-from-falcon
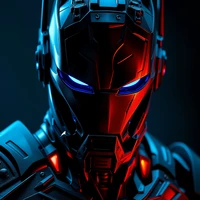
reacted to
Yehor's
post with 👀
21 days ago
Post
1933
Made a simple Python script to generate Argilla project for audio annotation from a dataset:
https://github.com/egorsmkv/argilla-audio-annotation
https://github.com/egorsmkv/argilla-audio-annotation
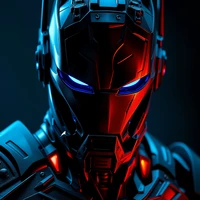
reacted to
hesamation's
post with ❤️
21 days ago
Post
2698
What, How, Where, and How Well? This paper reviews test-time scaling methods and all you need to know about them:
> parallel, sequential, hybrid, internal scaling
> how to scale (SFT, RL, search, verification)
> metrics and evals of test-time scaling
🔗paper: What, How, Where, and How Well? A Survey on Test-Time Scaling in Large Language Models (2503.24235)
If you want to learn what inference-time compute scaling is @rasbt has a great blog post on that:
https://magazine.sebastianraschka.com/p/state-of-llm-reasoning-and-inference-scaling
> parallel, sequential, hybrid, internal scaling
> how to scale (SFT, RL, search, verification)
> metrics and evals of test-time scaling
🔗paper: What, How, Where, and How Well? A Survey on Test-Time Scaling in Large Language Models (2503.24235)
If you want to learn what inference-time compute scaling is @rasbt has a great blog post on that:
https://magazine.sebastianraschka.com/p/state-of-llm-reasoning-and-inference-scaling
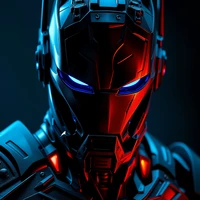
reacted to
vincentg64's
post with 🔥
21 days ago
Post
2287
The Rise of Specialized LLMs for Enterprise -https://mltblog.com/3QXXE4I
In this article, I discuss the main problems of standard LLMs (OpenAI and the likes), and how the new generation of LLMs addresses these issues. The focus is on Enterprise LLMs.
LLMs with Billions of Parameters: Most of the LLMs still fall in that category. The first ones (ChatGPT) appeared around 2022, though Bert is an early precursor. Most recent books discussing LLMs still define them as transformer architecture with deep neural networks (DNNs), costly training, and reliance on GPUs. The training is optimized to predict the next tokens or missing tokens. However, this task is remotely relevant to what modern LLMs now deliver to the user, see here. Yet it requires time and intensive computer resources. Indeed, this type of architecture works best with billions or trillions of tokens. In the end, most of these tokens are noise, requiring smart distillation for performance improvement.
The main issues are:
➡️ Performance: Requires GPU and large corpuses as input data. Re-training is expensive. Hallucinations are still a problem. Fine-tuning is delicate (Blackbox). You need prompt engineering to get the best results. Mixtures of experts (multiple sub-LLMs, DeepSeek) is one step towards improving accuracy.
➡️ Cost: Besides the GPU costs, the pricing model charges by the token, incentivizing vendors to use models with billions of tokens.
Read full article describing more issues and how LLM 2.0 addresses them, at https://mltblog.com/3QXXE4I
More links:
- To receive latest updates: https://mltblog.com/4iTvQec
- About LLM 2.0: https://mltblog.com/4g2sKTv
- PowerPoint presentation: https://mltblog.com/43DYviE
- Our company website: https://mlt
In this article, I discuss the main problems of standard LLMs (OpenAI and the likes), and how the new generation of LLMs addresses these issues. The focus is on Enterprise LLMs.
LLMs with Billions of Parameters: Most of the LLMs still fall in that category. The first ones (ChatGPT) appeared around 2022, though Bert is an early precursor. Most recent books discussing LLMs still define them as transformer architecture with deep neural networks (DNNs), costly training, and reliance on GPUs. The training is optimized to predict the next tokens or missing tokens. However, this task is remotely relevant to what modern LLMs now deliver to the user, see here. Yet it requires time and intensive computer resources. Indeed, this type of architecture works best with billions or trillions of tokens. In the end, most of these tokens are noise, requiring smart distillation for performance improvement.
The main issues are:
➡️ Performance: Requires GPU and large corpuses as input data. Re-training is expensive. Hallucinations are still a problem. Fine-tuning is delicate (Blackbox). You need prompt engineering to get the best results. Mixtures of experts (multiple sub-LLMs, DeepSeek) is one step towards improving accuracy.
➡️ Cost: Besides the GPU costs, the pricing model charges by the token, incentivizing vendors to use models with billions of tokens.
Read full article describing more issues and how LLM 2.0 addresses them, at https://mltblog.com/3QXXE4I
More links:
- To receive latest updates: https://mltblog.com/4iTvQec
- About LLM 2.0: https://mltblog.com/4g2sKTv
- PowerPoint presentation: https://mltblog.com/43DYviE
- Our company website: https://mlt
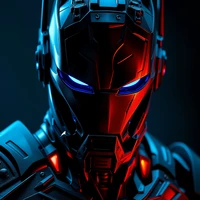
reacted to
DualityAI-RebekahBogdanoff's
post with 🚀🔥
21 days ago
Post
2559
Curious how Duality AI crafts synthetic data that can bridge the sim2real gap?
We just published an article here on HuggingFace outlining our process, with bonus dataset releases! Read it here: https://huggingface.co/blog/DualityAI-RebekahBogdanoff/training-yolov8-with-synthetic-data-from-falcon
We just published an article here on HuggingFace outlining our process, with bonus dataset releases! Read it here: https://huggingface.co/blog/DualityAI-RebekahBogdanoff/training-yolov8-with-synthetic-data-from-falcon