Gorilla-openfunctions-v0 - Sharded
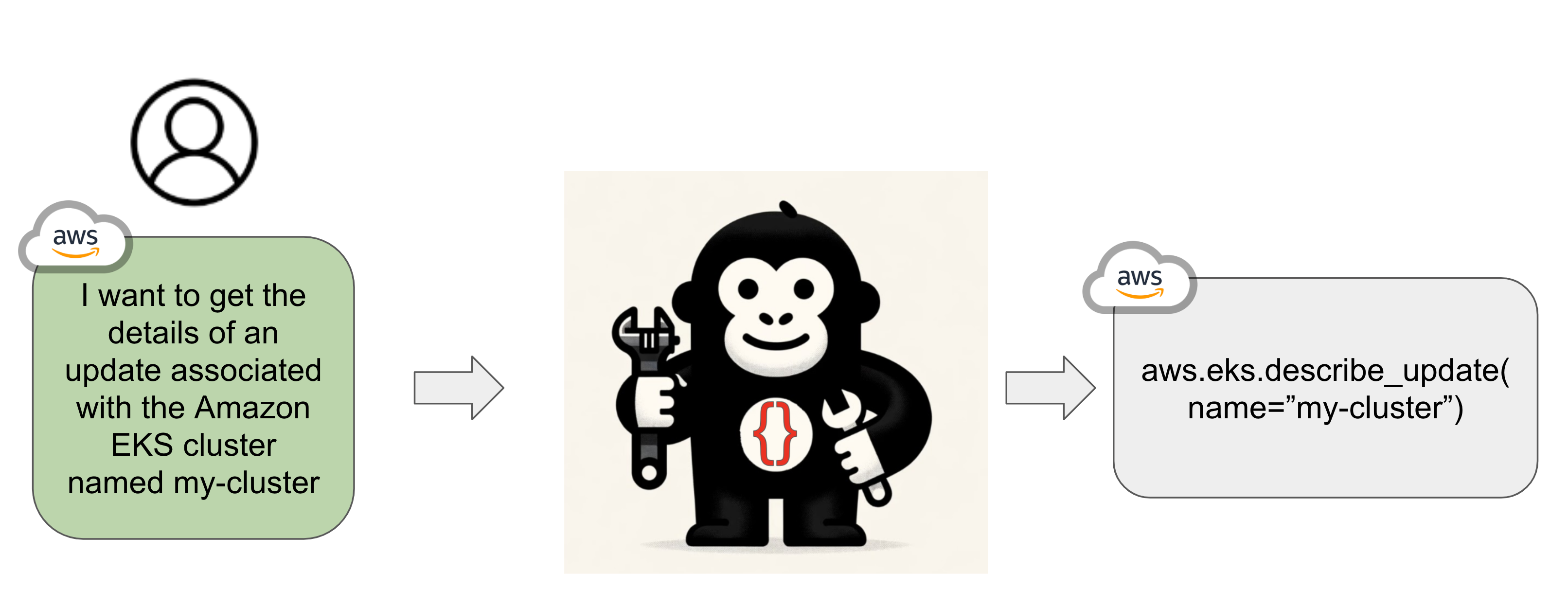
π§©π§©π§© Just a sharded version of gorilla-openfunctions-v0.
π» Using this version, you can smoothly load the model on Colab and play with it!
From the original model card:
Gorilla OpenFunctions extends Large Language Model(LLM) Chat Completion feature to formulate executable APIs call given natural language instructions and API context.
π£ NEW: try this model for Information Extraction
π§ͺπ¦ Needle in a Jungle - Information Extraction via LLMs
I did an experiment: a notebook that extracts information from a given URL (or text) based on a user-provided structure.
Stack: ποΈ Haystack LLM framework + Gorilla OpenFunctions
- π Post full of details
- π Notebook
General Usage
This version of the model is meant primarily to run smoothly on Colab. I suggest loading the model with 8-bit quantization, so that you have some free GPU to perform inference.
However, it is perfectly fine to load the model in half-precision or with stronger quantization (4-bit).
! pip install transformers accelerate bitsandbytes
import json
import torch
from transformers import pipeline
def get_prompt(user_query: str, functions: list = []) -> str:
"""
Generates a conversation prompt based on the user's query and a list of functions.
Parameters:
- user_query (str): The user's query.
- functions (list): A list of functions to include in the prompt.
Returns:
- str: The formatted conversation prompt.
"""
if len(functions) == 0:
return f"USER: <<question>> {user_query}\nASSISTANT: "
functions_string = json.dumps(functions)
return f"USER: <<question>> {user_query} <<function>> {functions_string}\nASSISTANT: "
# Pipeline setup
pipe = pipeline(
"text-generation",
model="anakin87/gorilla-openfunctions-v0-sharded",
device_map="auto",
model_kwargs={"load_in_8bit":True, "torch_dtype":torch.float16},
max_new_tokens=128,
batch_size=16
)
# Example usage
query: str = "Call me an Uber ride type \"Plus\" in Berkeley at zipcode 94704 in 10 minutes"
functions = [
{
"name": "Uber Carpool",
"api_name": "uber.ride",
"description": "Find suitable ride for customers given the location, type of ride, and the amount of time the customer is willing to wait as parameters",
"parameters": [
{"name": "loc", "description": "Location of the starting place of the Uber ride"},
{"name": "type", "enum": ["plus", "comfort", "black"], "description": "Types of Uber ride user is ordering"},
{"name": "time", "description": "The amount of time in minutes the customer is willing to wait"}
]
}
]
# Generate prompt and obtain model output
prompt = get_prompt(query, functions=functions)
output = pipe(prompt)
print(output[0]['generated_text'].rpartition("ASSISTANT:")[-1].strip())
# uber.ride(loc="berkeley", type="plus", time=10)
- Downloads last month
- 20