The retrain-pipelines org and I joined the waitlist today. Been looking forward to this for some time. Curious to see the outcome. The promise got me hooked from day 1. The tech as presented does have potential.
Aurélien-Morgan CLAUDON
Aurelien-Morgan
AI & ML interests
None yet
Recent Activity
new activity
about 2 hours ago
huggingface/HuggingDiscussions:[FEEDBACK] Follow
Organizations
Aurelien-Morgan's activity
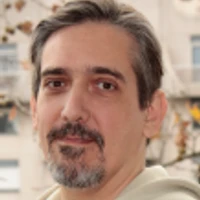
reacted to
jsulz's
post with ❤️
about 2 hours ago
Post
1125
It's finally here ❤️
Build faster than ever with lightning fast upload and download speeds starting today on the Hub ⚡
Xet storage is rolling out access across the Hub - join the waitlist here https://huggingface.co/join/xet
You can apply for yourself, or your entire organization. Head over to your account settings for more information or join anywhere you see the Xet logo on a repository you know.
Have questions? Join the conversation below 👇 or open a discussion on the Xet team page xet-team/README
Build faster than ever with lightning fast upload and download speeds starting today on the Hub ⚡
Xet storage is rolling out access across the Hub - join the waitlist here https://huggingface.co/join/xet
You can apply for yourself, or your entire organization. Head over to your account settings for more information or join anywhere you see the Xet logo on a repository you know.
Have questions? Join the conversation below 👇 or open a discussion on the Xet team page xet-team/README
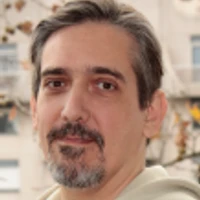
reacted to
thomwolf's
post with 🚀
2 days ago
Post
2021
We've kept pushing our Open-R1 project, an open initiative to replicate and extend the techniques behind DeepSeek-R1.
And even we were mind-blown by the results we got with this latest model we're releasing: ⚡️OlympicCoder ( open-r1/OlympicCoder-7B and open-r1/OlympicCoder-32B)
It's beating Claude 3.7 on (competitive) programming –a domain Anthropic has been historically really strong at– and it's getting close to o1-mini/R1 on olympiad level coding with just 7B parameters!
And the best part is that we're open-sourcing all about its training dataset, the new IOI benchmark, and more in our Open-R1 progress report #3: https://huggingface.co/blog/open-r1/update-3
Datasets are are releasing:
- open-r1/codeforces
- open-r1/codeforces-cots
- open-r1/ioi
- open-r1/ioi-test-cases
- open-r1/ioi-sample-solutions
- open-r1/ioi-cots
- open-r1/ioi-2024-model-solutions
And even we were mind-blown by the results we got with this latest model we're releasing: ⚡️OlympicCoder ( open-r1/OlympicCoder-7B and open-r1/OlympicCoder-32B)
It's beating Claude 3.7 on (competitive) programming –a domain Anthropic has been historically really strong at– and it's getting close to o1-mini/R1 on olympiad level coding with just 7B parameters!
And the best part is that we're open-sourcing all about its training dataset, the new IOI benchmark, and more in our Open-R1 progress report #3: https://huggingface.co/blog/open-r1/update-3
Datasets are are releasing:
- open-r1/codeforces
- open-r1/codeforces-cots
- open-r1/ioi
- open-r1/ioi-test-cases
- open-r1/ioi-sample-solutions
- open-r1/ioi-cots
- open-r1/ioi-2024-model-solutions
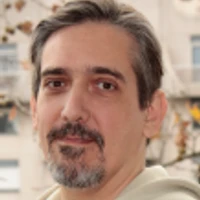
reacted to
julien-c's
post with 😎
2 days ago
Post
2137
Important notice 🚨
For Inference Providers who have built support for our Billing API (currently: Fal, Novita, HF-Inference – with more coming soon), we've started enabling Pay as you go (=PAYG)
What this means is that you can use those Inference Providers beyond the free included credits, and they're charged to your HF account.
You can see it on this view: any provider that does not have a "Billing disabled" badge, is PAYG-compatible.
For Inference Providers who have built support for our Billing API (currently: Fal, Novita, HF-Inference – with more coming soon), we've started enabling Pay as you go (=PAYG)
What this means is that you can use those Inference Providers beyond the free included credits, and they're charged to your HF account.
You can see it on this view: any provider that does not have a "Billing disabled" badge, is PAYG-compatible.
You gonna revive the LinkedIn newsletter someday ? Think it was cool 😭😭
https://www.linkedin.com/newsletters/7233909926606053377/
Seen many astonishing results from people posting examples of this model in action.
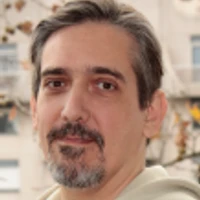
reacted to
AdinaY's
post with 🤯
14 days ago
Post
2712
Wan2.1 🔥📹 new OPEN video model by Alibaba Wan team!
Model: Wan-AI/Wan2.1-T2V-14B
Demo: Wan-AI/Wan2.1
✨Apache 2.0
✨8.19GB VRAM, runs on most GPUs
✨Multi-Tasking: T2V, I2V, Video Editing, T2I, V2A
✨Text Generation: Supports Chinese & English
✨Powerful Video VAE: Encode/decode 1080P w/ temporal precision
Model: Wan-AI/Wan2.1-T2V-14B
Demo: Wan-AI/Wan2.1
✨Apache 2.0
✨8.19GB VRAM, runs on most GPUs
✨Multi-Tasking: T2V, I2V, Video Editing, T2I, V2A
✨Text Generation: Supports Chinese & English
✨Powerful Video VAE: Encode/decode 1080P w/ temporal precision
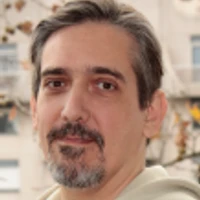
reacted to
freddyaboulton's
post with 🤗🔥
16 days ago
Post
3158
Getting WebRTC and Websockets right in python is very tricky. If you've tried to wrap an LLM in a real-time audio layer then you know what I'm talking about.
That's where FastRTC comes in! It makes WebRTC and Websocket streams super easy with minimal code and overhead.
Check out our org: hf.co/fastrtc
That's where FastRTC comes in! It makes WebRTC and Websocket streams super easy with minimal code and overhead.
Check out our org: hf.co/fastrtc
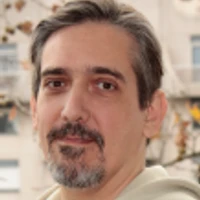
reacted to
lysandre's
post with ❤️
19 days ago
Post
5619
SmolVLM-2 and SigLIP-2 are now part of
They're added on top of the v4.49.0 release, and can be installed from the following tags:
This marks a new beginning for the release process of transformers. For the past five years, we've been doing monthly releases featuring many models (v4.49.0, the latest release, features 9 new architectures).
Starting with SmolVLM-2 & SigLIP2, we'll now additionally release tags supporting new models on a stable branch. These models are therefore directly available for use by installing from the tag itself. These tags will continue to be updated with fixes applied to these models.
Going forward, continue expecting software releases following semantic versioning: v4.50.0 will have ~10 new architectures compared to v4.49.0, as well as a myriad of new features, improvements and bug fixes. Accompanying these software releases, we'll release tags offering brand new models as fast as possible, to make them accessible to all immediately.
transformers
in dedicated releases!They're added on top of the v4.49.0 release, and can be installed from the following tags:
v4.49.0-SmolVLM-2
and v4.49.0-SigLIP-2
.This marks a new beginning for the release process of transformers. For the past five years, we've been doing monthly releases featuring many models (v4.49.0, the latest release, features 9 new architectures).
Starting with SmolVLM-2 & SigLIP2, we'll now additionally release tags supporting new models on a stable branch. These models are therefore directly available for use by installing from the tag itself. These tags will continue to be updated with fixes applied to these models.
Going forward, continue expecting software releases following semantic versioning: v4.50.0 will have ~10 new architectures compared to v4.49.0, as well as a myriad of new features, improvements and bug fixes. Accompanying these software releases, we'll release tags offering brand new models as fast as possible, to make them accessible to all immediately.
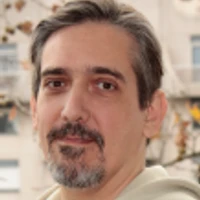
reacted to
jsulz's
post with 🚀❤️
21 days ago
Post
3414
Time flies!
Six months after joining Hugging Face the Xet team is kicking off the first migrations from LFS to our storage for a number of repositories on the Hub.
More on the nitty gritty details behind the migration soon, but here are the big takeaways:
🤖 We've successfully completed the first migrations from LFS -> Xet to test the infrastructure and prepare for a wider release
✅ No action on your part needed - you can work with a Xet-backed repo like any other repo on the Hub (for now - major improvements on their way!)
👀 Keep an eye out for the Xet logo to see if a repo you know is on our infra! See the screenshots below to spot the difference 👇
⏩ ⏩ ⏩ Blazing uploads and downloads coming soon. W’re gearing up for a full integration with the Hub's Python library that will make building on the Hub faster than ever - special thanks to @celinah and @Wauplin for their assistance.
🎉 Want Early Access? If you’re curious and want to test it out the bleeding edge that will power the development experience on the Hub, we’d love to partner with you. Let me know!
This is the culmination of a lot of effort from the entire team. Big round of applause to @sirahd @brianronan @jgodlewski @hoytak @seanses @assafvayner @znation @saba9 @rajatarya @port8080 @yuchenglow
Six months after joining Hugging Face the Xet team is kicking off the first migrations from LFS to our storage for a number of repositories on the Hub.
More on the nitty gritty details behind the migration soon, but here are the big takeaways:
🤖 We've successfully completed the first migrations from LFS -> Xet to test the infrastructure and prepare for a wider release
✅ No action on your part needed - you can work with a Xet-backed repo like any other repo on the Hub (for now - major improvements on their way!)
👀 Keep an eye out for the Xet logo to see if a repo you know is on our infra! See the screenshots below to spot the difference 👇
⏩ ⏩ ⏩ Blazing uploads and downloads coming soon. W’re gearing up for a full integration with the Hub's Python library that will make building on the Hub faster than ever - special thanks to @celinah and @Wauplin for their assistance.
🎉 Want Early Access? If you’re curious and want to test it out the bleeding edge that will power the development experience on the Hub, we’d love to partner with you. Let me know!
This is the culmination of a lot of effort from the entire team. Big round of applause to @sirahd @brianronan @jgodlewski @hoytak @seanses @assafvayner @znation @saba9 @rajatarya @port8080 @yuchenglow
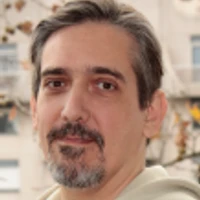
reacted to
fdaudens's
post with ❤️
21 days ago
Post
5807
🎯 Perplexity drops their FIRST open-weight model on Hugging Face: A decensored DeepSeek-R1 with full reasoning capabilities. Tested on 1000+ examples for unbiased responses.
Check it out: perplexity-ai/r1-1776
Blog post: https://perplexity.ai/hub/blog/open-sourcing-r1-1776
Check it out: perplexity-ai/r1-1776
Blog post: https://perplexity.ai/hub/blog/open-sourcing-r1-1776
Real' interesting.
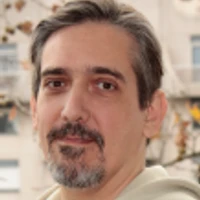
reacted to
AdinaY's
post with 🔥
22 days ago
Post
2444
The latest paper of DeepSeek is now available on the Daily Papers page 🚀
You can reach out to the authors directly on this page👇
Native Sparse Attention: Hardware-Aligned and Natively Trainable Sparse Attention (2502.11089)
You can reach out to the authors directly on this page👇
Native Sparse Attention: Hardware-Aligned and Natively Trainable Sparse Attention (2502.11089)
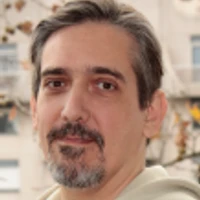
reacted to
merve's
post with ❤️🚀
22 days ago
Post
5936
Google just released PaliGemma 2 Mix: new versatile instruction vision language models 🔥
> Three new models: 3B, 10B, 28B with res 224, 448 💙
> Can do vision language tasks with open-ended prompts, understand documents, and segment or detect anything 🤯
Read more https://huggingface.co/blog/paligemma2mix
Try the demo google/paligemma2-10b-mix
All models are here google/paligemma-2-mix-67ac6a251aaf3ee73679dcc4
> Three new models: 3B, 10B, 28B with res 224, 448 💙
> Can do vision language tasks with open-ended prompts, understand documents, and segment or detect anything 🤯
Read more https://huggingface.co/blog/paligemma2mix
Try the demo google/paligemma2-10b-mix
All models are here google/paligemma-2-mix-67ac6a251aaf3ee73679dcc4
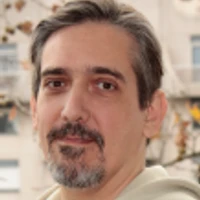
reacted to
fdaudens's
post with ❤️
29 days ago
Post
2691
⭐️ The AI Energy Score project just launched - this is a game-changer for making informed decisions about AI deployment.
You can now see exactly how much energy your chosen model will consume, with a simple 5-star rating system. Think appliance energy labels, but for AI.
Looking at transcription models on the leaderboard is fascinating: choosing between whisper-tiny or whisper-large-v3 can make a 7x difference. Real-time data on these tradeoffs changes everything.
166 models already evaluated across 10 different tasks, from text generation to image classification. The whole thing is public and you can submit your own models to test.
Why this matters:
- Teams can pick efficient models that still get the job done
- Developers can optimize for energy use from day one
- Organizations can finally predict their AI environmental impact
If you're building with AI at any scale, definitely worth checking out.
👉 leaderboard: https://lnkd.in/esrSxetj
👉 blog post: https://lnkd.in/eFJvzHi8
Huge work led by @sasha with @bgamazay @yjernite @sarahooker @regisss @meg
You can now see exactly how much energy your chosen model will consume, with a simple 5-star rating system. Think appliance energy labels, but for AI.
Looking at transcription models on the leaderboard is fascinating: choosing between whisper-tiny or whisper-large-v3 can make a 7x difference. Real-time data on these tradeoffs changes everything.
166 models already evaluated across 10 different tasks, from text generation to image classification. The whole thing is public and you can submit your own models to test.
Why this matters:
- Teams can pick efficient models that still get the job done
- Developers can optimize for energy use from day one
- Organizations can finally predict their AI environmental impact
If you're building with AI at any scale, definitely worth checking out.
👉 leaderboard: https://lnkd.in/esrSxetj
👉 blog post: https://lnkd.in/eFJvzHi8
Huge work led by @sasha with @bgamazay @yjernite @sarahooker @regisss @meg
Those guys ship !
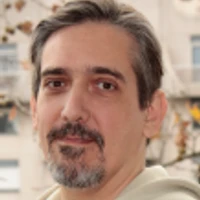
reacted to
AdinaY's
post with 🔥
29 days ago
Post
3560
InspireMusic 🎵🔥 an open music generation framework by Alibaba FunAudio Lab
Model: FunAudioLLM/InspireMusic-1.5B-Long
Demo: FunAudioLLM/InspireMusic
✨ Music, songs, audio - ALL IN ONE
✨ High quality audio: 24kHz & 48kHz sampling rates
✨ Long-Form Generation: enables extended audio creation
✨ Efficient Fine-Tuning: precision (BF16, FP16, FP32) with user-friendly scripts
Model: FunAudioLLM/InspireMusic-1.5B-Long
Demo: FunAudioLLM/InspireMusic
✨ Music, songs, audio - ALL IN ONE
✨ High quality audio: 24kHz & 48kHz sampling rates
✨ Long-Form Generation: enables extended audio creation
✨ Efficient Fine-Tuning: precision (BF16, FP16, FP32) with user-friendly scripts