Spaces:
Runtime error
Runtime error
File size: 29,407 Bytes
ca4ab65 2f9d73b 73f7e39 850858e 548d450 eaf258a 850858e 548d450 850858e 548d450 850858e 06f9c28 850858e fda5a48 850858e fda5a48 850858e fda5a48 850858e fda5a48 850858e fda5a48 850858e 06f9c28 850858e 06f9c28 850858e 06f9c28 850858e 06f9c28 850858e fda5a48 850858e 06f9c28 850858e 548d450 27b97d0 548d450 e846f26 548d450 e7f0569 548d450 850858e 9d72e91 4c8ff7b 9d72e91 4c8ff7b 9d72e91 4c8ff7b 9d72e91 40deb01 4c8ff7b 40deb01 73f7e39 |
1 2 3 4 5 6 7 8 9 10 11 12 13 14 15 16 17 18 19 20 21 22 23 24 25 26 27 28 29 30 31 32 33 34 35 36 37 38 39 40 41 42 43 44 45 46 47 48 49 50 51 52 53 54 55 56 57 58 59 60 61 62 63 64 65 66 67 68 69 70 71 72 73 74 75 76 77 78 79 80 81 82 83 84 85 86 87 88 89 90 91 92 93 94 95 96 97 98 99 100 101 102 103 104 105 106 107 108 109 110 111 112 113 114 115 116 117 118 119 120 121 122 123 124 125 126 127 128 129 130 131 132 133 134 135 136 137 138 139 140 141 142 143 144 145 146 147 148 149 150 151 152 153 154 155 156 157 158 159 160 161 162 163 164 165 166 167 168 169 170 171 172 173 174 175 176 177 178 179 180 181 182 183 184 185 186 187 188 189 190 191 192 193 194 195 196 197 198 199 200 201 202 203 204 205 206 207 208 209 210 211 212 213 214 215 216 217 218 219 220 221 222 223 224 225 226 227 228 229 230 231 232 233 234 235 236 237 238 239 240 241 242 243 244 245 246 247 248 249 250 251 252 253 254 255 256 257 258 259 260 261 262 263 264 265 266 267 268 269 270 271 272 273 274 275 276 277 278 279 280 281 282 283 284 285 286 287 288 289 290 291 292 293 294 295 296 297 298 299 300 301 302 303 304 305 306 307 308 309 310 311 312 313 314 315 316 317 318 319 320 321 322 323 324 325 326 327 328 329 330 331 332 333 334 335 336 337 338 339 340 341 342 343 344 345 346 347 348 349 350 351 352 353 354 355 356 357 358 359 360 361 362 363 364 365 366 367 368 369 370 371 372 373 374 375 376 377 378 379 380 381 382 383 384 385 386 387 388 389 390 391 392 393 394 395 396 397 398 399 400 401 402 403 404 405 406 407 408 409 410 411 412 413 414 415 416 417 418 419 420 421 422 423 424 425 426 427 428 429 430 431 432 433 434 435 436 437 438 439 440 441 442 443 444 445 446 447 448 449 450 451 452 453 454 455 456 457 458 459 460 461 462 463 464 465 466 467 468 469 470 471 472 473 474 475 476 477 478 479 480 481 482 483 484 485 486 487 488 489 490 491 492 493 494 495 496 497 498 499 500 501 502 503 504 505 506 507 508 509 510 511 512 513 514 515 516 517 518 519 520 521 522 523 524 525 526 527 |
---
title: Csgy6613 Project Rk2546
emoji: 🌍
colorFrom: pink
colorTo: yellow
sdk: streamlit
sdk_version: 1.17.0
app_file: src/main.py
pinned: false
---
# cs-gy-6613-project-rk2546
## Milestone 4
[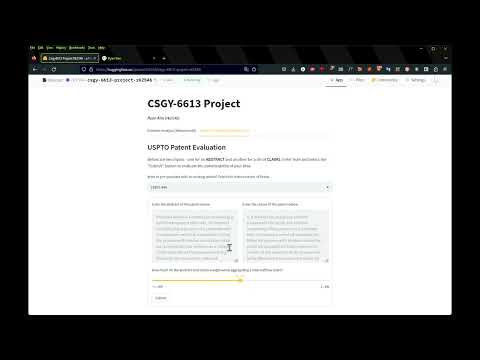](https://youtu.be/csSGBwIE7nk "Project Video")
### **Landing Page**
[Visit the Landing Page here!](https://www.rkim.dev/#/portfolio/projects/patent_prediction)
### **Code Breakdown**
The USPTO application is divided into several directories. Overall, the important files are present in the application as such:
````
- data/
- train.json
- val.json
- src/
- main.py
- train.ipynb
- val.ipynb
````
Both `train.json` and `val.json` contain the original USPTO data, sized down to contain only the relevant data from each recorded patent and split between training and validation data. The validation data `val.json` is used in the online USPTO application as a set of pre-set patents that a user can select when using the USPTO patent prediction function. That, and the `val.ipynb` file was used to validate the model's accuracy.
The primary code back-end is stored in `main.py`, which runs the application on the HuggingFace space UI. The application uses **Streamlit** to render UI elements on the screen. All models run off of Transformers and Tokenizers from **HuggingFace**.
The application has two features: Sentiment Analysis (for Milestone #2) and USPTO Patent Acceptance Prediction (Milestone #3). Both run on `main.py`. Sentiment Analysis relies on pre-trained [models](https://huggingface.co/models) from HuggingFace's public [datasets](https://huggingface.co/datasets) - particularly 4 models:
- [cardiffnlp/twitter-roberta-base-sentiment](https://huggingface.co/cardiffnlp/twitter-roberta-base-sentiment)
- [finiteautomata/beto-sentiment-analysis](https://huggingface.co/finiteautomata/beto-sentiment-analysis)
- [bhadresh-savani/distilbert-base-uncased-emotion](https://huggingface.co/bhadresh-savani/distilbert-base-uncased-emotion)
- [siebert/sentiment-roberta-large-english](https://huggingface.co/siebert/sentiment-roberta-large-english)
The Patent Acceptance Prediction uses two fine-tuned models, which are built off of a pre-existing model named [distilbert-base-uncased](https://huggingface.co/distilbert-base-uncased) and fine-tuned off of the USPTO dataset. The tokenizer used to parse text uses the same [distilbert-base-uncased](https://huggingface.co/distilbert-base-uncased) model but is left unmodified.
### **Shared Code**
In this section, I describe some code back-end used universally across both the Sentiment Analysis and Patent Accceptance Prediction functions.
Starting from `main.py`, we load in the necessary tokenizers and pipelines needed to run the model.
````python
import streamlit as st
from transformers import TextClassificationPipeline, pipeline
from transformers import AutoTokenizer, AutoModelForSequenceClassification, DistilBertTokenizerFast, DistilBertForSequenceClassification
````
These transformers are crucial to the functionality of both Sentiment Analysis and Patent Acceptance Prediction. Particularly, Sentiment Analysis uses `AutoModelForSequenceClassification` as our model class, `AutoTokenizer` as our string pre-processor, and `pipeline` to combine the two. Patent Acceptance Prediction uses `DistilBertForSequenceClassification` as our model class, `DistilBertTokenizerFast` as our string pre-processor, and `TextClassificationPipeline` to combine the two.
Because everything runs on one `main.py` page and both the Sentiment Analysis and Patent Acceptance Prediction function similarly, I developed a custom python class called `ModelImplementation` that's used by both and allows for switching between different models:
````python
class ModelImplementation(object):
def __init__(
self,
transformer_model_name,
model_transformer,
tokenizer_model_name,
tokenizer_func,
pipeline_func,
parser_func,
classifier_args={},
placeholders=[""]
):
self.transformer_model_name = transformer_model_name
self.tokenizer_model_name = tokenizer_model_name
self.placeholders = placeholders
self.model = model_transformer.from_pretrained(self.transformer_model_name)
self.tokenizer = tokenizer_func.from_pretrained(self.tokenizer_model_name)
self.classifier = pipeline_func(model=self.model, tokenizer=self.tokenizer, padding=True, truncation=True, **classifier_args)
self.parser = parser_func
self.history = []
def predict(self, val):
result = self.classifier(val)
return self.parser(self, result)
````
The main idea is that for every model that's needed, we create a new instance of this class. In each case, we can store a reference to the tokenizer, model, and pipeline; the model will then use that tokenizer, model, and pipeline in the `predict()` call. If the output of a model needs to be curated in some way (ex. we need to post-process the output of a model so that it's more human-readable), we can also pass a custom method alongside the other parameters too. This is useful when we are switching between models in the Sentiment Analysis page or between the Sentiment Analysis and Patent Acceptance Prediction page - we merely have to create or modify an instance of the `ModelImplementation` class with the proper tokenizer, model, pipeline, and post-process method (if needed). Placeholder text for any inputs can also be stored as well in an array.
The Sentiment Analysis and Patent Acceptance Prediction pages are both stored on one interface, with a tab menu allowing a user to switch between the two.
````python
# Title
st.title("CSGY-6613 Project")
# Subtitle
st.markdown("_**Ryan Kim (rk2546)**_")
sentimentTab, patentTab = st.tabs([
"Emotion Analysis [Milestone #2]",
"Patent Prediction [Milestone #3]"
])
with sentimentTab:
st.subheader("Sentiment Analysis")
// ...
with patentTab:
st.subheader("USPTO Patent Evaluation")
// ...
````
### **Sentiment Analysis**
Sentiment Analysis is relatively simple. It uses the `ModelImplementation` class detailed above to switch between four pre-existing HuggingFace models for the sentiment analysis:
- [cardiffnlp/twitter-roberta-base-sentiment](https://huggingface.co/cardiffnlp/twitter-roberta-base-sentiment)
- [finiteautomata/beto-sentiment-analysis](https://huggingface.co/finiteautomata/beto-sentiment-analysis)
- [bhadresh-savani/distilbert-base-uncased-emotion](https://huggingface.co/bhadresh-savani/distilbert-base-uncased-emotion)
- [siebert/sentiment-roberta-large-english](https://huggingface.co/siebert/sentiment-roberta-large-english)
A method called `ParseEmotionOutput()` is used to process labels outputted by the [cardiffnlp/twitter-roberta-base-sentiment](https://huggingface.co/cardiffnlp/twitter-roberta-base-sentiment) model in particular. Upon loading, if a model hasn't been instantiated yet, the page will create a new model with a pre-set model name and cache it for later use:
````python
def emotion_model_change():
st.session_state.emotion_model = ModelImplementation(
st.session_state.emotion_model_name,
AutoModelForSequenceClassification,
st.session_state.emotion_model_name,
AutoTokenizer,
pipeline,
ParseEmotionOutput,
classifier_args={ "task" : "sentiment-analysis" },
placeholders=["@AmericanAir just landed - 3hours Late Flight - and now we need to wait TWENTY MORE MINUTES for a gate! I have patience but none for incompetence."]
)
if "emotion_model_name" not in st.session_state:
st.session_state.emotion_model_name = "cardiffnlp/twitter-roberta-base-sentiment"
emotion_model_change()
````
The method `emotion_model_change()` can be called to switch between different models, based on the session-saved `emotion_model_name` value. By default, the [cardiffnlp/twitter-roberta-base-sentiment](https://huggingface.co/cardiffnlp/twitter-roberta-base-sentiment) model is used. To switch between models, we use a **Streamlit** `selectbox` module:
````python
model_option = st.selectbox(
"What sentiment analysis model do you want to use? NOTE: Lag may occur when loading a new model!",
emotion_model_names,
on_change=emotion_model_change,
key="emotion_model_name"
)
form = st.form(key='sentiment-analysis-form')
text_input = form.text_area(
"Enter some text for sentiment analysis! If you just want to test it out without entering anything, just press the \"Submit\" button and the model will look at the placeholder.",
placeholder=st.session_state.emotion_model.placeholders[0]
)
submit = form.form_submit_button('Submit')
````
When the page loads, a placeholder from the current model is printed inside a **Streamlit** `text_area` module. When the user clicks on the `form_submit_button` button, the app will use whatever model is currently cached to generate output predictions. If no user input was provided, the placeholder value is passed as the input instead.
````python
if submit:
if text_input is None or len(text_input.strip()) == 0:
to_eval = st.session_state.emotion_model.placeholders[0]
else:
to_eval = text_input.strip()
label, score, output_func = st.session_state.emotion_model.predict(to_eval)
output_func("**{}**: {}".format(label,score))
````
### **USPTO Patent Acceptance Prediction**
The back-end for the USPTO Patent Acceptance Prediction is similar to that of **Sentiment Analysis**, but with some major differences.
Instead of switching between for pre-trained HuggingFace models, we use two fine-tuned models. These models are available online:
- [rk2546/uspto-patents-abstracts](https://huggingface.co/rk2546/uspto-patents-abstracts)
- [rk2546/uspto-patents-claims](https://huggingface.co/rk2546/uspto-patents-claims)
and are built off of a pre-existing model named [distilbert-base-uncased](https://huggingface.co/distilbert-base-uncased). They are fine-tuned off of the USPTO dataset. The tokenizer used to parse text uses the same [distilbert-base-uncased](https://huggingface.co/distilbert-base-uncased) model but is left unmodified.
````python
if "patent_data" not in st.session_state:
f = open('./data/val.json')
valData = json.load(f)
f.close()
patent_data = {}
for num, label, abstract, claim in zip(valData["patent_numbers"],valData["labels"], valData["abstracts"], valData["claims"]):
patent_data[num] = {"patent_number":num,"label":label,"abstract":abstract,"claim":claim}
st.session_state.patent_data = patent_data
st.session_state.patent_num = list(patent_data.keys())[0]
st.session_state.weight = 0.5
st.session_state.patent_abstract_model = ModelImplementation(
'rk2546/uspto-patents-abstracts',
DistilBertForSequenceClassification,
'distilbert-base-uncased',
DistilBertTokenizerFast,
TextClassificationPipeline,
ParsePatentOutput,
classifier_args={"return_all_scores":True},
)
print("Patent abstracts model initialized")
st.session_state.patent_claim_model = ModelImplementation(
'rk2546/uspto-patents-claims',
DistilBertForSequenceClassification,
'distilbert-base-uncased',
DistilBertTokenizerFast,
TextClassificationPipeline,
ParsePatentOutput,
classifier_args={"return_all_scores":True},
)
print("Patent claims model initialized")
````
In addition, instead of the user selecting a particular model to use, instead the interface allows for two separate inputs - one for an Abstract draft and another for a Claims draft. These fields can be pre-populated using an `st.selectbox` that, when selected, pre-fills the two inputs with examples from `val.json`:
````python
patent_select_list = list(st.session_state.patent_data.keys())
patent_index_option = st.selectbox(
"Want to pre-populate with an existing patent? Select the index number of below.",
patent_select_list,
key="patent_num",
)
//...
with st.form(key='patent-form'):
col1, col2 = st.columns(2)
with col1:
abstract_input = st.text_area(
"Enter the abstract of the patent below",
placeholder=st.session_state.patent_data[st.session_state.patent_num]["abstract"],
height=400
)
with col2:
claim_input = st.text_area(
"Enter the claims of the patent below",
placeholder=st.session_state.patent_data[st.session_state.patent_num]["claim"],
height=400
)
weight_val = st.slider(
"How much do the abstract and claims weight when aggregating a total softmax score?",
min_value=-1.0,
max_value=1.0,
value=0.5,
)
submit = st.form_submit_button('Submit')
````
One unique addition is a slider `st.slider` that lets the user tune how much the two models' softmax outputs are combined. This way, some customizability is allowed - if the user wants only an Abstract to be used, then the weight can be adjusted to bias the Abstract model's output completely.
### **Training the USPTO Patent Acceptance Predictor**
While the Sentiment Analysis function does not need to be trained, the model for the patent prediction needs to be fine-tuned from an existing model. The scripts that do so are available in both `src/train.py` and `src/patent_train.ipynb`, with the latter being a more user-readable option. **NOTE:** the `src/train.py` implementation is a tad outdated. Please rely on `src/patent_train.ipynb` if you want to try for yourself.
The general idea was to divide the training into steps:
1. Parse only the necessary data out from the raw USPTO data,
2. Separate the data into training and validation data
3. Fine-tune an existing HuggingFace model into two separate models, each of which separately perform predictions onto abstracts and claims respectively.
4. Publish the two models onto HuggingFace to make the models easily accessible.
The only data columns parsed from the original USPTO data were:
- "patent_number" <- Unique identifier
- "abstract" <- Input #1
- "claims" <- Input #2
- "decision" <- Output Labels
We also filter rows such that the values in the "decision" column only consist of "ACCEPTED" or "REJECTED"; these values are then coded into `1` and `0` respectively for easier data processing. Beyond splitting the data into training and validation data, we also split each into two sub-datasets - one consisting of Abstracts, and another for Claims. No pre-processing was performed further.
During fine-tuning, we use a custom class that will be used by HuggingFace's various transformers:
````python
class USPTODataset(Dataset):
def __init__(self, encodings, labels):
self.encodings = encodings
self.labels = labels
def __getitem__(self, idx):
item = {key: torch.tensor(val[idx]) for key, val in self.encodings.items()}
item['labels'] = torch.tensor(self.labels[idx])
return item
def __len__(self):
return len(self.labels)
````
The training data is fitted into an instance of this class, and that instance is fed into the training algorithm. First, all input strings are tokenized. The tokenized data is then fed into instances of `USPTODataset`.
````python
train_abstracts_encodings = tokenizer(trainData["abstracts"], truncation=True, padding=True)
train_claims_encodings = tokenizer(trainData["claims"], truncation=True, padding=True)
train_abstracts_dataset = USPTODataset(train_abstracts_encodings, trainData["labels"])
train_claims_dataset = USPTODataset(train_claims_encodings, trainData["labels"])
````
When initializing the model that we'll fine-tune, we also add an additional step to run the training on the GPU rather than the CPU. This is a time optimization measure.
````python
device = torch.device('cuda') if torch.cuda.is_available() else torch.device('cpu')
model = DistilBertForSequenceClassification.from_pretrained(model_name)
model.to(device)
model.train()
````
Finally, we load the datasets into wrapper classes that are used during the training. We then train for `10` epochs.
````python
train_abstracts_loader = DataLoader(train_abstracts_dataset, batch_size=32, shuffle=True)
train_claims_loader = DataLoader(train_claims_dataset, batch_size=32, shuffle=True)
optim = AdamW(model.parameters(), lr=5e-5)
def Train(loader, save_path, num_train_epochs=2):
batch_num = len(loader)
for epoch in range(num_train_epochs):
print(f'\t- Training epoch {epoch+1}/{num_train_epochs}')
batch_count = 0
for batch in loader:
print(f'{batch_count}|{batch_num} - {round((batch_count/batch_num)*100)}%', end="")
#print('\t\t- optim zero grad')
optim.zero_grad()
#print('\t\t- input_ids')
input_ids = batch['input_ids'].to(device)
#print('\t\t- attention_mask')
attention_mask = batch['attention_mask'].to(device)
#print('\t\t- labels0')
labels = batch['labels'].to(device)
#print('\t\t- outputs')
outputs = model(input_ids, attention_mask=attention_mask, labels=labels)
#print('\t\t- loss')
loss = outputs[0]
#print('\t\t- backwards')
loss.backward()
#print('\t\t- step')
optim.step()
batch_count += 1
print("\r", end="")
model.save_pretrained(save_path, from_pt=True)
print(f'Saved model in {save_path}!\n')
print("=== TRAINING ABSTRACTS ===")
Train(train_abstracts_loader,upsto_abstracts_model_path, num_train_epochs=10)
print("----")
print("=== TRAINING CLAIMS ===")
Train(train_claims_loader,upsto_claims_model_path, num_train_epochs=10)
````
### Evaluating the Models
#### Sentiment Analysis
There isn't an effective way to validate the sentiment analysis models, as they are publicly available models and it is unknown what data they were explicitly trained on. Therefore, evaluation will rely on anecdotal testing.
The sentiment models that appear to work the best are the [cardiffnlp/twitter-roberta-base-sentiment](https://huggingface.co/cardiffnlp/twitter-roberta-base-sentiment) and [siebert/sentiment-roberta-large-english](https://huggingface.co/siebert/sentiment-roberta-large-english) models, with few caveats. These two models generally perform very well at detecting sentiment in mid to long expressions. However the [siebert/sentiment-roberta-large-english](https://huggingface.co/siebert/sentiment-roberta-large-english) model tends to suffer when expressions are shorter and less complex in lexicon. Even the [cardiffnlp/twitter-roberta-base-sentiment](https://huggingface.co/cardiffnlp/twitter-roberta-base-sentiment) model suffers from time to time if not enough context has been provided.
The model that performed the worst is the [finiteautomata/beto-sentiment-analysis](https://huggingface.co/finiteautomata/beto-sentiment-analysis). This model seems to have the worst time trying to interpret meaning from sentences, even with strongly worded language such as "hate". For example, the expression "I hate you" returns a **NEUTRAL** response with 99.6% confidence, which differs from the [cardiffnlp/twitter-roberta-base-sentiment](https://huggingface.co/cardiffnlp/twitter-roberta-base-sentiment) and [siebert/sentiment-roberta-large-english](https://huggingface.co/siebert/sentiment-roberta-large-english) models (**NEGATIVE**: ~96.5% - ~99.9% accuracy respectively). It appears that the [finiteautomata/beto-sentiment-analysis](https://huggingface.co/finiteautomata/beto-sentiment-analysis) gets confused when not enough context is provided. The expression "I hate you because you hurt my family" manages to return a **NEGATIVE** label, but with a mere 87.7% confidence. **NOTE**: _These expressions are purely meant for experimentation purposes, to convey model inaccuracies!_
The unique model is the [bhadresh-savani/distilbert-base-uncased-emotion](https://huggingface.co/bhadresh-savani/distilbert-base-uncased-emotion) model, which instead gives 6 general emotions as opposed to a binary **NEGATIVE** or **POSITIVE** rating:
* Sadness
* Joy
* Love
* Anger
* Fear
* Surprise
This one offers more nuance to each evaluation, but while the general distinction between "positive" and "negative" emotions is often accurate, the specific emotion applied to the statement is sometimes confusing. For example, the expression "I love you because you saved my family" is labeled as **JOY** instead of **LOVE**.
Overall, all models are able to perform to some level of success, but the context of each input needs to be made clear in the wording for the models to produce accurate responses. These models seem to suffer when not enough context is provided, sometimes blatantly giving incorrect responses to situations that are too simple.
#### Patent Acceptance Prediction
With access to labeled validation data, the fine-tuned models can be ranked in accuracy. Sample code that does so is provided in `src/val.ipynb` and evaluates 1000 random data samples out of 4000+ samples.
Overall, both the fine-tuned Abstract model and the Claims model seem to perform very similarly, producing accuracy rates of 72.89% and 72.8% accuracy respectively out of 1000 random samples. The aggregated softmax labeling, assuming equal weighting between the two models, produces 76.2% accuracy. Depending on who you ask, this performance can be discouraging or encouraging.
The 76% accuracy rating being higher than the individual fine-tuned models is interesting, as it implies that the accuracy between the two models is not consistent; there are times when one of the models might outperform the other in certain situations. If the accuracy remained the same across all 1000 random samples, then the aggregated prediction accuracy rate would be within the same range as the individual fine-tuned models.
---
## Milestone 3
Click the link to access the HuggingFace Streamlit application:
[Project webpage](https://huggingface.co/spaces/rk2546/csgy-6613-project-rk2546).
_The HuggingFace page includes the ability to check Milestone #2 as well._
The fine-tuned models have been uploaded as HuggingFace models, public and open for anyone to use. You can find and use them here:
- [https://huggingface.co/rk2546/uspto-patents-abstracts](https://huggingface.co/rk2546/uspto-patents-abstracts)
- Purpose: Primary purpose is to evaluate whether a patent will be accepted or rejected. Trained from patent abstracts from the USPTO database.
- Model Name: `rk2546/uspto-patents-abstracts`
- [https://huggingface.co/rk2546/uspto-patents-claims/tree/main](https://huggingface.co/rk2546/uspto-patents-claims/tree/main)
- Purpose: Primary purpose is to evaluate whether a patent will be accepted or rejected. Trained from patent claims from the USPTO database.
- Model Name: `rk2546/uspto-patents-claims`
Milestone #3's implementation performs a softmax evaluation using both models, then combines the responses based on a weight parameter. The weight parameter is adjustable in the HuggingFace application interface. By default, the output from the Claims model is weighed heavier (75%) over the Abstracts model (25%).
---
## Milestone 2
Click the link to access the HuggingFace Streamlit application:
[Project webpage](https://huggingface.co/spaces/rk2546/csgy-6613-project-rk2546)
---
## Milestone 1
### Installing Necessary Prerequisites
I already have Docker installed on my system from prior projects. I recall following these specific steps:
1. Install Docker Desktop, provided via the official [Docker Website](https://www.docker.com/products/docker-desktop)
2. Installed WSL2. Unknown source.
3. Restarted PC to initialize and complete install of WSL2.
Proof of installs for both are provided in the following screenshot:

Visual Studio Code is already installed on my PC. The VS Code version was provided via the [official website](https://code.visualstudio.com/). VS Code has the recommended extensions for Docker installed for ease-of-use:

There's another list of extensions I added to my VS Code:
- **Remote - SSH**
- **Remote Development**
- **Dev Containers** <-- The REAL important one
- **Remote - Tunnels**
The third one, **Dev Containers**, will be useful for opening up folders inside the container.
The steps to get the Docker instance ready for this project follow the attached video, provided in the instructions of the milestone writeup on the course website.
[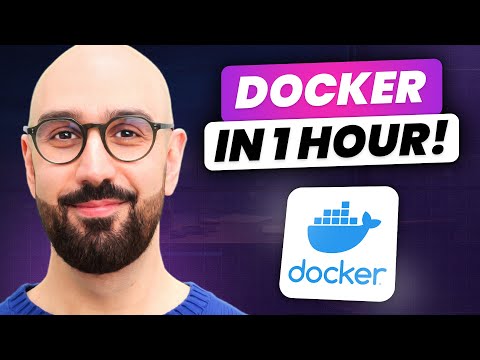](https://www.youtube.com/watch?v=pTFZFxd4hOI "Docker Tutorial for Beginners")
### Example: Creating a Docker Image
While this isn't necessarily related to setting up Docker for python development, this is here for posterity and to show how to create customized Docker images that can be used later. This example is creating a Node.JS-based image that executes a simple
The steps are as follows:
1. I created a `misc_example/` directory that will contain a sample application to run. Inside, I created an `example.js` file that has some basic code to run (ex. `console.log("Hellow World")`)
2. I created a `Dockerfile` file with the following code:
```docker
FROM node:alpine
COPY . /misc_example
WORKDIR /misc_example
CMD node example.js
```
The code above uses the "apline" linux distribution and node. It tells to copy everything in this root directory over to the `misc_example` folder, changes the working directory to the `misc_example` folder, then executes the command to run `example.js`.
To buld the docker image, we execute the following command in the CMD:
```
docker build -t csgy6613proj-rk2546 ./misc_example
```
To prove that the build was created successfully, the following command was used to list all images that Docker has access to:
```
docker image ls
```
To see if it works, we execute the following command:
```
docker run csgy6613proj-rk2546
```
We can see that the very simple console logs we wrote in `example.js` were successfully printed. Refer to the screenshot below for a full understanding of the commands executed and logs created:

### Initializing a Docker Development Environment for Python
A part of this milestone will probably involve a lot of dev work in python and Docker. We will save time by having our project code open in a pre-existing Docker Container specifically designed for python work.
To that end, I have a basic `src` directory that will be used to store all dev work in the repo. Inside is a `app.py` file that does some basic operations and prints them to console. We will open this repo inside of a python-specific Docker container and see if we can work from there.
Here are the steps to follow:
1. Generate a `requirements.txt` file. We will palce this inside of the root of this repo. I generated it using the following a single command (shown below). This was inspired by [this article](https://learnpython.com/blog/python-requirements-file/) that describes how to generate the new `requirements.txt` file. This file contains all important packages that are key for python development in modern use cases.
```
pip freeze > requirements.txt
```
2. Have the project open in VS Code.
3. Make sure that the **Dev Containers** extension is installed.
4. In the bottom-left corner, there is a `><` button. Click it, and select the option `Reopen in Container`.
5. In the popup window, type `Python` and select `Python 3`, whichever version you want (I go with the default, 3.11).
6. No need to add any additional features. Simply click "OK".
You will have VS Code reopen a new window, this time with the folder opened inside a new `Python 3` container. You can confirm this by looking at the bottom-left corner of the window and checking if it says "Dev Container: Python 3". You should also see an additional new `.devcontainer` directory that was added. This is a very convenient step that actually makes the process simpler the next go around - instead of designating which container to open the folder in and all that, this directory caches our selections and simply skips a lot of the above steps.
One last step is to install some key packages that will be helpful for dev work in Python. These are recorded in `requirements.txt`, and we can install them all using a one-line command in the terminal:
```
pip install -r requirements.txt
```
### Example: Hello World Python Test
For example, we'll create a new `src` folder. The new `src` folder will contain a simple python script `app.py` that spits out some lists of numbers of varying dimensionalities.
We can simply run this command by clicking the "Play" button on the top-right corner of the window, assuming you have `src/app.py` open in the window.
An example of the output is provided below as proof that we have this working:

The main benefit is that any changes we make to these files will be automatically saved to our local files as well, speeding up any logistics behind how the Docker container is interacting with our original files. |