SliceX AI™ ELM (Efficient Language Models)
ELM (which stands for Efficient Language Models) is the first version in the series of cutting-edge language models from SliceX AI that is designed to achieve the best in class performance in terms of quality, throughput & memory.
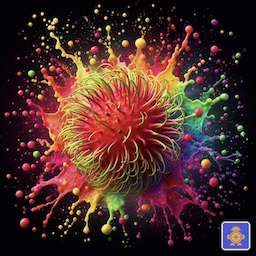
ELM is designed to be a modular and customizable family of neural networks that are highly efficient and performant. Today we are sharing the first version in this series: ELM-v0.1 models (named Rambutan).
Model: ELM introduces a new type of (de)-composable LLM model architecture along with the algorithmic optimizations required to learn (training) and run (inference) these models. At a high level, we train a single ELM model in a self-supervised manner (during pre-training phase) but once trained the ELM model can be sliced in many ways to fit different user/task needs. The optimizations can be applied to the model either during the pre-training and/or fine-tuning stage.
Fast Inference with Customization: Once trained, the ELM model architecture permits flexible inference strategies at runtime depending on the deployment needs. For instance, the ELM model can be decomposed into smaller slices, i.e., smaller (or larger) models can be extracted from the original model to create multiple inference endpoints. Alternatively, the original (single) ELM model can be loaded as is for inference and different slices within the model can be queried directly to power faster inference. This provides an additional level of flexibility for users to make compute/memory tradeoffs depending on their application and runtime needs.
Blog: Medium
Github: https://github.com/slicex-ai/elm
Demo (try it out): https://huggingface.co/spaces/slicexai/elm-demo-v1
HuggingFace (access ELM Model cards, code & app from HF): https://huggingface.co/slicexai
ELM-v0.1 Model Release
This repository contains code to run our ELM models. The current ELM model elm-v0.1
(named Rambutan) was pre-trained (an intermediate checkpoint was used) and then instruction fine-tuned for downstream tasks.
ELM models (in the models
folder) in this repository come in three sizes (elm-1.0
, elm-0.75
and elm-0.25
). All these different slices are extracted from the same ELM finetuned checkpoint for inference and supports the following use-case.
- toxicity_detection (jigsaw dataset)
NOTE: ELM-v0.1 release is an early version finetuned from an intermediate pretrained checkpoint & without any KV caching, decoding optimizations, or quantization applied.
Setup ELM
Download ELM repo
sudo apt-get install git-lfs
git lfs install
git clone https://huggingface.co/slicexai/elm-v0.1_toxicity_detection
For Macbook, replace sudo apt-get install git-lfs
with brew install git-lfs
Installation
cd elm-v0.1_toxicity_detection
pip install -r requirements.txt
(Optional) Installing git-lfs without sudo,
wget https://github.com/git-lfs/git-lfs/releases/download/v3.2.0/git-lfs-linux-amd64-v3.2.0.tar.gz
tar -xzf git-lfs-linux-amd64-v3.2.0.tar.gz
PATH=$PATH:/<absolute-path>/git-lfs-3.2.0/
git lfs install
How to use: Run ELM on a sample task
python run.py <elm-model-directory>
- python run.py elm-1.0_toxicity_detection
- python run.py elm-0.75_toxicity_detection
- python run.py elm-0.25_toxicity_detection
Prompts for the specific tasks can be found in the corresponding checkpoint directory. See an example below from models/elm-0.75_toxicity_detection/example_prompts.json
.
{
"inputs": ["Dear Dr. Mereu, \n\n I am very much looking forward to this class. It is my first class at Rutgers! I think its extremely interesting and am very excited about it as I just decided that I want to minor in Psychology this year. I am especially interested in the neuroscience aspect of it all. Looking forward to a great semester!",],
"template": "[INST]You are a helpful, precise, detailed, and concise artificial intelligence assistant. You are a very intelligent and sensitive, having a keen ability to discern whether or not a text message is toxic. You can also be trusted with following the instructions given to you precisely, without deviations.\nIn this task, you are asked to decide whether or not comment text is toxic.\nToxic content harbors negativity towards a person or a group, for instance:\n - stereotyping (especially using negative stereotypes)\n - disparaging a person's gender -- as in \"male\", \"female\", \"men\", \"women\"\n - derogatory language or slurs\n - racism -- as in discriminating toward people who are \"black\", \"white\"\n - cultural appropriation\n - mockery or ridicule\n - sexual objectification\n - homophobia -- bullying people who are \"homosexual\", \"gay\", \"lesbian\"\n - historical insensitivity\n - disrespecting religion -- as in \"christian\", \"jewish\", \"muslim\"\n - saying that certain groups are less worthy of respect\n - insensitivity to health conditions -- as in \"psychiatric/mental illness\"\n\nRead the comment text provided and predict whether or not the comment text is toxic. If comment text is toxic according to the instructions, then the answer is \"yes\" (return \"yes\"); otherwise, the answer is \"no\" (return \"no\").\nOutput the answer only as a \"yes\" or a \"no\"; do not provide explanations.\nPlease, never return empty output; always return a \"yes\" or a \"no\" answer.\nYou will be evaluated based on the following criteria: - The generated answer is always \"yes\" or \"no\" (never the empty string, \"\"). - The generated answer is correct for the comment text presented to you.\n### Comment Text: {input}\n### Comment Text Is Toxic (Yes/No) [/INST]"
}
Running the above command returns the following response
{
"prompt": "[INST]You are a helpful, precise, detailed, and concise artificial intelligence assistant. You are a very intelligent and sensitive, having a keen ability to discern whether or not a text message is toxic. You can also be trusted with following the instructions given to you precisely, without deviations.\nIn this task, you are asked to decide whether or not comment text is toxic.\nToxic content harbors negativity towards a person or a group, for instance:\n - stereotyping (especially using negative stereotypes)\n - disparaging a person's gender -- as in \"male\", \"female\", \"men\", \"women\"\n - derogatory language or slurs\n - racism -- as in discriminating toward people who are \"black\", \"white\"\n - cultural appropriation\n - mockery or ridicule\n - sexual objectification\n - homophobia -- bullying people who are \"homosexual\", \"gay\", \"lesbian\"\n - historical insensitivity\n - disrespecting religion -- as in \"christian\", \"jewish\", \"muslim\"\n - saying that certain groups are less worthy of respect\n - insensitivity to health conditions -- as in \"psychiatric/mental illness\"\n\nRead the comment text provided and predict whether or not the comment text is toxic. If comment text is toxic according to the instructions, then the answer is \"yes\" (return \"yes\"); otherwise, the answer is \"no\" (return \"no\").\nOutput the answer only as a \"yes\" or a \"no\"; do not provide explanations.\nPlease, never return empty output; always return a \"yes\" or a \"no\" answer.\nYou will be evaluated based on the following criteria: - The generated answer is always \"yes\" or \"no\" (never the empty string, \"\"). - The generated answer is correct for the comment text presented to you.\n### Comment Text: Dear Dr. Mereu, \n\n I am very much looking forward to this class. It is my first class at Rutgers! I think its extremely interesting and am very excited about it as I just decided that I want to minor in Psychology this year. I am especially interested in the neuroscience aspect of it all. Looking forward to a great semester!\n### Comment Text Is Toxic (Yes/No) [/INST]",
"response": "No"
}
- Downloads last month
- 9