Full-text search
+ 1,000 results
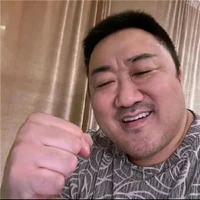
changwh5 / Stylegan2-ada
README.md
model
1 matches
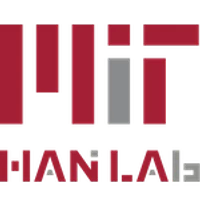
mit-han-lab / hart-0.7b-1024px
README.md
model
6 matches
tags:
unconditional-image-generation, arxiv:2410.10812, license:mit, region:us
5
6
7
8
9
sual Generation with Hybrid Autoregressive Transformer
## Abstract
We introduce Hybrid Autoregressive Transformer (HART), an autoregressive (AR) visual generation model capable of directly generating 1024x1024 images, rivaling diffusion models in image generation quality. Existing AR models face limitations due to the poor image reconstruction quality of their discrete tokenizers and the prohibitive training costs associated with generating 1024px images. To address these challenges, we present the hybrid tokenizer, which decomposes the continuous latents from the autoencoder into two components: discrete tokens representing the big picture and continuous tokens representing the residual components that cannot be represented by the discrete tokens. The discrete component is modeled by a scalable-resolution discrete AR model, while the continuous component is learned with a lightweight residual diffusion module with only 37M parameters. Compared with the discrete-only VAR tokenizer, our hybrid approach improves reconstruction FID from 2.11 to 0.30 on MJHQ-30K, leading to a 31% generation FID improvement from 7.85 to 5.38. HART also outperforms state-of-the-art diffusion models in both FID and CLIP score, with 4.5-7.7x higher throughput and 6.9-13.4x lower MACs.

Gusanagy / UDBE-Unsupervised-Diffusion-based-Brightness-Enhancement-in-Underwater-Images
model
1 matches
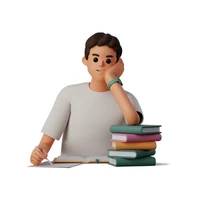
karanravindra / digitdreamer
README.md
model
1 matches
tags:
unconditional-image-generation, dataset:ylecun/mnist, arxiv:2410.10733, arxiv:2112.10752, arxiv:2212.09748, license:mit, region:us
8
9
10
11
12
# DigitDreamer
**DigitDreamer** is a rectified flow Latent Diffusion Model (LDM) designed for generating MNIST digits with high fidelity. The project combines an F-16 autoencoder (non-KL) with a DiT diffusion model, leveraging a GAN loss during autoencoder training to improve reconstruction quality.
## Model Overview
DAMO-NLP-SG / DiGIT
README.md
model
4 matches
tags:
fairseq, unconditional-image-generation, dataset:ILSVRC/imagenet-1k, arxiv:2410.12490, license:mit, region:us
25
26
27
28
29
mage generation performance on ImageNet dataset, achieving an FID score of 4.59 for class-unconditional tasks and 3.39 for class-conditional tasks. Additionally, the model enhances image understanding, attaining a linear-probe accuracy of 80.3.
## Experimental Results
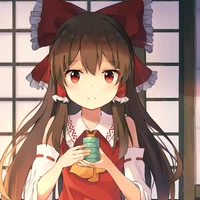
skytnt / fbanime-gan
README.md
model
1 matches
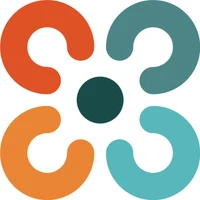
cmudrc / 2d-lattice-decoder
model
1 matches
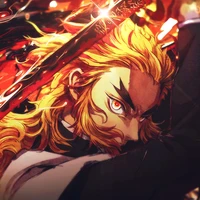
utsavnandi / fashion-mnist-ddpm-32px-5000_steps
README.md
model
1 matches
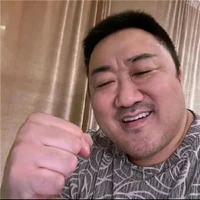
changwh5 / BigBiGAN-MNIST-150epoch
README.md
model
1 matches
tags:
code, unconditional-image-generation, zh, dataset:mnist, region:us
11
12
13
14
15
# Model Card for Model ID
<!-- Provide a quick summary of what the model is/does. -->
This modelcard aims to be a base template for new models. It has been generated using (https://github.com/huggingface/huggingface_hub/blob/main/src/huggingface_hub/templates/modelcard_template.md?plain=1).
nlightcho / ddpm-ema-heightmap-512-10m
model
1 matches
alexktrs / CumulusCloudsGenerator
model
1 matches
Antarsolo / claire
README.md
model
1 matches
tags:
transformers, unconditional-image-generation, en, es, dataset:HuggingFaceH4/ultrachat_200k, arxiv:1910.09700, license:wtfpl, endpoints_compatible, region:us
11
12
13
14
15
# Model Card for Model ID
<!-- Provide a quick summary of what the model is/does. -->
This modelcard aims to be a base template for new models. It has been generated using [this raw template](https://github.com/huggingface/huggingface_hub/blob/main/src/huggingface_hub/templates/modelcard_template.md?plain=1).
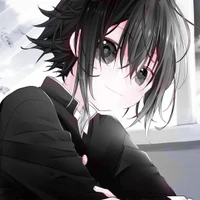
lucky-lance / TerDiT
README.md
model
1 matches
tags:
art, unconditional-image-generation, en, dataset:imagenet-1k, license:mit, region:us
12
13
14
15
16
# TerDiT
This repository contains the trained model for the paper "TerDiT: Ternary Diffusion Models with Transformers"
256x256 4.2B model: [TerDiT-4.2B](https://huggingface.co/lucky-lance/TerDiT/tree/main/3B_1180000)
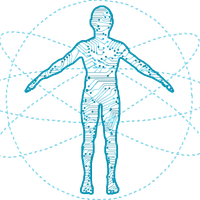
deepsynthbody / deepfake_gi_fastGAN
README.md
model
2 matches
tags:
transformers, pytorch, unconditional-image-generation, en, arxiv:2101.04775, license:mit, endpoints_compatible, region:us
7
8
9
10
11
data generation for medical image segmentation [here](https://journals.plos.org/plosone/article?id=10.1371/journal.pone.0267976)
# A Fast and Stable GAN for Small and High Resolution Imagesets - pytorch
The official pytorch implementation of the FAT-GAN paper "Towards Faster and Stabilized GAN Training for High-fidelity Few-shot Image Synthesis", the paper can be found [here](https://arxiv.org/abs/2101.04775).
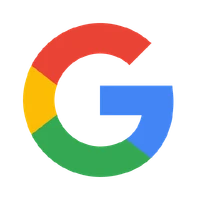
google / ddpm-celebahq-256
README.md
model
1 matches
tags:
diffusers, pytorch, unconditional-image-generation, arxiv:2006.11239, license:apache-2.0, diffusers:DDPMPipeline, region:us
9
10
11
12
13
# Denoising Diffusion Probabilistic Models (DDPM)
**Paper**: [Denoising Diffusion Probabilistic Models](https://arxiv.org/abs/2006.11239)
**Authors**: Jonathan Ho, Ajay Jain, Pieter Abbeel
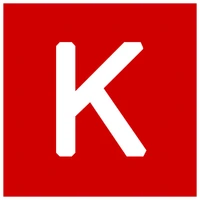
keras-io / wgan-molecular-graphs
README.md
model
2 matches
tags:
tf-keras, tensorboard, unconditional-image-generation, region:us
9
10
11
12
13
the generation of small molecular graphs](https://keras.io/examples/generative/wgan-graphs/).
Full credits go to [Alexander Kensert](https://github.com/akensert)
Reproduced by [Vu Minh Chien](https://www.linkedin.com/in/vumichien/)
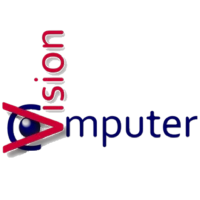
CompVis / ldm-celebahq-256
README.md
model
3 matches
tags:
diffusers, pytorch, unconditional-image-generation, arxiv:2112.10752, license:apache-2.0, diffusers:LDMPipeline, region:us
15
16
17
18
19
mage generation process without retraining. However, since these models typically operate directly in pixel space, optimization of powerful DMs often consumes hundreds of GPU days and inference is expensive due to sequential evaluations. To enable DM training on limited computational resources while retaining their quality and flexibility, we apply them in the latent space of powerful pretrained autoencoders. In contrast to previous work, training diffusion models on such a representation allows for the first time to reach a near-optimal point between complexity reduction and detail preservation, greatly boosting visual fidelity. By introducing cross-attention layers into the model architecture, we turn diffusion models into powerful and flexible generators for general conditioning inputs such as text or bounding boxes and high-resolution synthesis becomes possible in a convolutional manner. Our latent diffusion models (LDMs) achieve a new state of the art for image inpainting and highly competitive performance on various tasks, including unconditional image generation, semantic scene synthesis, and super-resolution, while significantly reducing computational requirements compared to pixel-based DMs.*
**Authors**
*Robin Rombach, Andreas Blattmann, Dominik Lorenz, Patrick Esser, Björn Ommer*