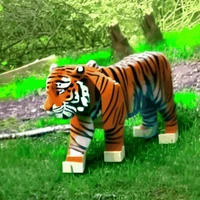
video-p2p-library
AI & ML interests
None defined yet.
Recent Activity
View all activity
video-p2p-library's activity
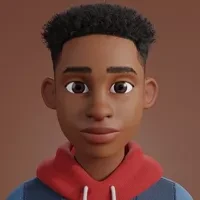
ameerazam08Β
posted
an
update
5 days ago
Post
1591
R1 is out! And with a lot of other R1 releated models...
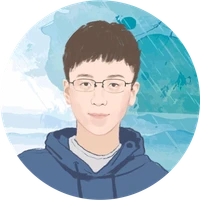
ShaldonΒ
authored
6
papers
20 days ago
Video-P2P: Video Editing with Cross-attention Control
Paper
β’
2303.04761
β’
Published
β’
2
Direct Inversion: Boosting Diffusion-based Editing with 3 Lines of Code
Paper
β’
2310.01506
β’
Published
RL-GPT: Integrating Reinforcement Learning and Code-as-policy
Paper
β’
2402.19299
β’
Published
Mini-Gemini: Mining the Potential of Multi-modality Vision Language Models
Paper
β’
2403.18814
β’
Published
β’
47
Multi-modal Cooking Workflow Construction for Food Recipes
Paper
β’
2008.09151
β’
Published
β’
1
Generative Video Propagation
Paper
β’
2412.19761
β’
Published
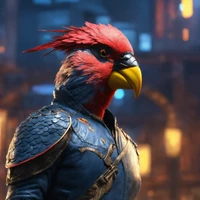
ehristoforuΒ
posted
an
update
about 1 month ago
Post
3176
βοΈ Ultraset - all-in-one dataset for SFT training in Alpaca format.
fluently-sets/ultraset
β Ultraset is a comprehensive dataset for training Large Language Models (LLMs) using the SFT (instruction-based Fine-Tuning) method. This dataset consists of over 785 thousand entries in eight languages, including English, Russian, French, Italian, Spanish, German, Chinese, and Korean.
π€― Ultraset solves the problem faced by users when selecting an appropriate dataset for LLM training. It combines various types of data required to enhance the model's skills in areas such as text writing and editing, mathematics, coding, biology, medicine, finance, and multilingualism.
π€ For effective use of the dataset, it is recommended to utilize only the "instruction," "input," and "output" columns and train the model for 1-3 epochs. The dataset does not include DPO or Instruct data, making it suitable for training various types of LLM models.
βοΈ Ultraset is an excellent tool to improve your language model's skills in diverse knowledge areas.
fluently-sets/ultraset
β Ultraset is a comprehensive dataset for training Large Language Models (LLMs) using the SFT (instruction-based Fine-Tuning) method. This dataset consists of over 785 thousand entries in eight languages, including English, Russian, French, Italian, Spanish, German, Chinese, and Korean.
π€― Ultraset solves the problem faced by users when selecting an appropriate dataset for LLM training. It combines various types of data required to enhance the model's skills in areas such as text writing and editing, mathematics, coding, biology, medicine, finance, and multilingualism.
π€ For effective use of the dataset, it is recommended to utilize only the "instruction," "input," and "output" columns and train the model for 1-3 epochs. The dataset does not include DPO or Instruct data, making it suitable for training various types of LLM models.
βοΈ Ultraset is an excellent tool to improve your language model's skills in diverse knowledge areas.
Post
7862
Google drops Gemini 2.0 Flash Thinking
a new experimental model that unlocks stronger reasoning capabilities and shows its thoughts. The model plans (with thoughts visible), can solve complex problems with Flash speeds, and more
now available in anychat, try it out: akhaliq/anychat
a new experimental model that unlocks stronger reasoning capabilities and shows its thoughts. The model plans (with thoughts visible), can solve complex problems with Flash speeds, and more
now available in anychat, try it out: akhaliq/anychat
Post
8847
QwQ-32B-Preview is now available in anychat
A reasoning model that is competitive with OpenAI o1-mini and o1-preview
try it out: akhaliq/anychat
A reasoning model that is competitive with OpenAI o1-mini and o1-preview
try it out: akhaliq/anychat
Post
3931
Post
2890
anychat
supports chatgpt, gemini, perplexity, claude, meta llama, grok all in one app
try it out there: akhaliq/anychat
supports chatgpt, gemini, perplexity, claude, meta llama, grok all in one app
try it out there: akhaliq/anychat
Post
2700
Plugins in NiansuhAI
Plugin Names:
1. WebSearch: Searches the web using search engines.
2. Calculator: Evaluates mathematical expressions, extending the base Tool class.
3. WebBrowser: Extracts and summarizes information from web pages.
4. Wikipedia: Retrieves information from Wikipedia using its API.
5. Arxiv: Searches and fetches article information from Arxiv.
6. WolframAlphaTool: Provides answers on math, science, technology, culture, society, and everyday life.
These plugins currently support the GPT-4O-2024-08-06 model, which also supports image analysis.
Try it now: https://huggingface.co/spaces/NiansuhAI/chat
Similar to: https://hf.co/chat
Plugin Names:
1. WebSearch: Searches the web using search engines.
2. Calculator: Evaluates mathematical expressions, extending the base Tool class.
3. WebBrowser: Extracts and summarizes information from web pages.
4. Wikipedia: Retrieves information from Wikipedia using its API.
5. Arxiv: Searches and fetches article information from Arxiv.
6. WolframAlphaTool: Provides answers on math, science, technology, culture, society, and everyday life.
These plugins currently support the GPT-4O-2024-08-06 model, which also supports image analysis.
Try it now: https://huggingface.co/spaces/NiansuhAI/chat
Similar to: https://hf.co/chat
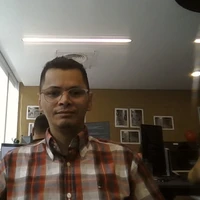
JoseRFJuniorΒ
posted
an
update
6 months ago
Post
1694
JoseRFJunior/TransNAR
https://github.com/JoseRFJuniorLLMs/TransNAR
https://arxiv.org/html/2406.09308v1
TransNAR hybrid architecture. Similar to Alayrac et al, we interleave existing Transformer layers with gated cross-attention layers which enable information to flow from the NAR to the Transformer. We generate queries from tokens while we obtain keys and values from nodes and edges of the graph. The node and edge embeddings are obtained by running the NAR on the graph version of the reasoning task to be solved. When experimenting with pre-trained Transformers, we initially close the cross-attention gate, in order to fully preserve the language modelβs internal knowledge at the beginning of training.
https://github.com/JoseRFJuniorLLMs/TransNAR
https://arxiv.org/html/2406.09308v1
TransNAR hybrid architecture. Similar to Alayrac et al, we interleave existing Transformer layers with gated cross-attention layers which enable information to flow from the NAR to the Transformer. We generate queries from tokens while we obtain keys and values from nodes and edges of the graph. The node and edge embeddings are obtained by running the NAR on the graph version of the reasoning task to be solved. When experimenting with pre-trained Transformers, we initially close the cross-attention gate, in order to fully preserve the language modelβs internal knowledge at the beginning of training.
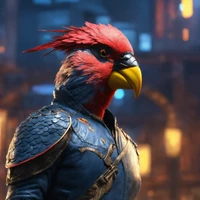
ehristoforuΒ
posted
an
update
6 months ago
Post
4319
π Hello from Project Fluently Team!
β¨ Finally we can give you some details about Supple Diffusion. We worked on it for a long time and we have little left, we apologize that we had to increase the work time.
π οΈ Some technical information. The first version will be the Small version (there will also be Medium, Large, Huge, possibly Tiny), it will be based on the SD1 architecture, that is, one text encoder, U-net, VAE. Now about each component, the first is a text encoder, it will be a CLIP model (perhaps not CLIP-L-path14), CLIP was specially retrained by us in order to achieve the universality of the model in understanding completely different styles and to simplify the prompt as much as possible. Next, we did U-net, U-net in a rather complicated way, first we trained different parts (types) of data with different U-nets, then we carried out merging using different methods, then we trained DPO and SPO using methods, and then we looked at the remaining shortcomings and further trained model, details will come later. We left VAE the same as in SD1 architecture.
π Compatibility. Another goal of the Supple model series is full compatibility with Auto1111 and ComfyUI already at the release stage, the model is fully supported by these interfaces and the diffusers library and does not require adaptation, your usual Sampling methods are also compatible, such as DPM++ 2M Karras, DPM++ SDE and others.
π§ Today, without demo images (there wasnβt much time), final work is underway on the model and we are already preparing to develop the Medium version, the release of the Small version will most likely be in mid-August or earlier.
π» Feel free to ask your questions in the comments below the post, we will be happy to answer them, have a nice day!
β¨ Finally we can give you some details about Supple Diffusion. We worked on it for a long time and we have little left, we apologize that we had to increase the work time.
π οΈ Some technical information. The first version will be the Small version (there will also be Medium, Large, Huge, possibly Tiny), it will be based on the SD1 architecture, that is, one text encoder, U-net, VAE. Now about each component, the first is a text encoder, it will be a CLIP model (perhaps not CLIP-L-path14), CLIP was specially retrained by us in order to achieve the universality of the model in understanding completely different styles and to simplify the prompt as much as possible. Next, we did U-net, U-net in a rather complicated way, first we trained different parts (types) of data with different U-nets, then we carried out merging using different methods, then we trained DPO and SPO using methods, and then we looked at the remaining shortcomings and further trained model, details will come later. We left VAE the same as in SD1 architecture.
π Compatibility. Another goal of the Supple model series is full compatibility with Auto1111 and ComfyUI already at the release stage, the model is fully supported by these interfaces and the diffusers library and does not require adaptation, your usual Sampling methods are also compatible, such as DPM++ 2M Karras, DPM++ SDE and others.
π§ Today, without demo images (there wasnβt much time), final work is underway on the model and we are already preparing to develop the Medium version, the release of the Small version will most likely be in mid-August or earlier.
π» Feel free to ask your questions in the comments below the post, we will be happy to answer them, have a nice day!
Post
2905
Introducing Plugins in NiansuhAI (on July 20, 2024)
Plugin Names:
1. WebSearch: Tool for searching the web using search engines.
2. Calculator: Helps evaluate mathematical expressions; extends the base Tool class.
3. WebBrowser: Interacts with web pages to extract information or summarize content.
4. Wikipedia: Retrieves data from Wikipedia using its API.
5. Arxiv: Searches and fetches article information from Arxiv.
6. WolframAlphaTool: Answers questions on Math, Science, Technology, Culture, Society, and Everyday Life.
Similar to https://hf.co/chat
Plugin Names:
1. WebSearch: Tool for searching the web using search engines.
2. Calculator: Helps evaluate mathematical expressions; extends the base Tool class.
3. WebBrowser: Interacts with web pages to extract information or summarize content.
4. Wikipedia: Retrieves data from Wikipedia using its API.
5. Arxiv: Searches and fetches article information from Arxiv.
6. WolframAlphaTool: Answers questions on Math, Science, Technology, Culture, Society, and Everyday Life.
Similar to https://hf.co/chat