Model Card for Model ID
Fine-Tuning Gemma Model with qLora and Supervised Fine-Tuning
This repository contains a comprehensive tutorial and notebook for fine-tuning the gemma-7b-it
model using qLora and Supervised Fine-Tuning (SFT). The tutorial demonstrates the process from setting up the environment to fine-tuning the model on a code generation dataset.
Overview
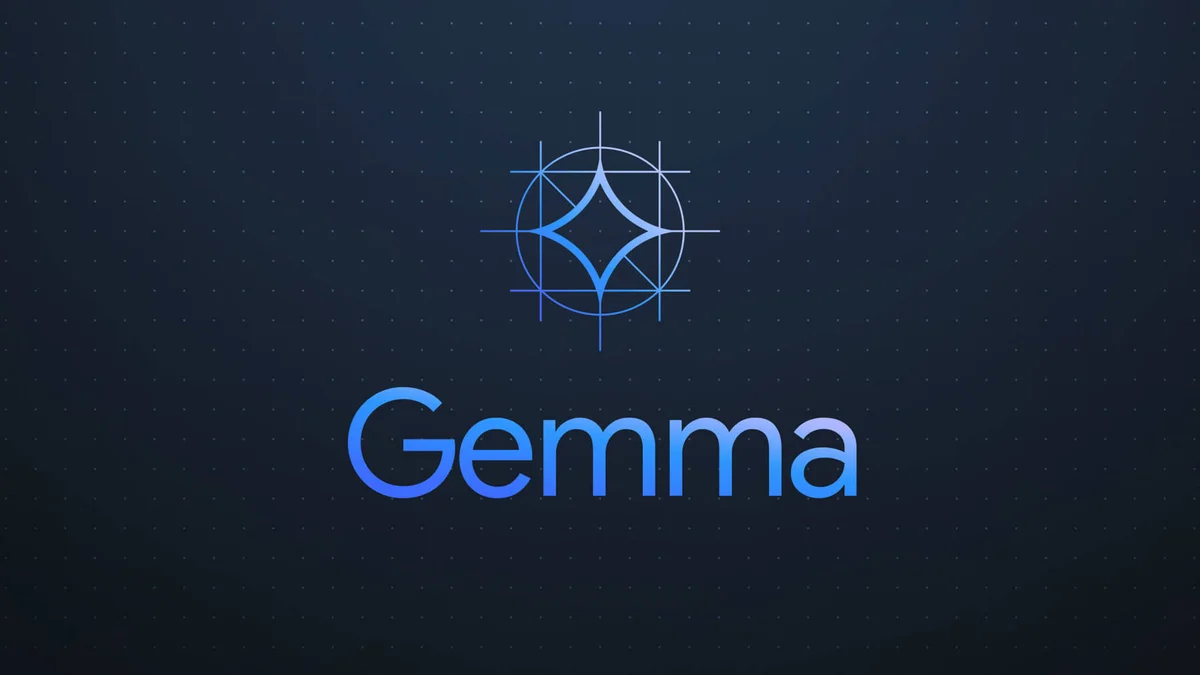
This notebook provides an end-to-end guide on how to fine-tune the gemma-7b-it
model. The fine-tuning process includes:
- Setting up the environment and prerequisites
- Loading and configuring the model with QLoRA quantization
- Preparing and formatting the dataset
- Applying LoRA for efficient fine-tuning
- Running the fine-tuning process
- Testing the fine-tuned model
Prerequisites
Ensure that you have the following prerequisites before running the notebook:
- GPU: A T4 (for
gemma-2b
) or an A100 GPU (forgemma-7b
). - Python Packages: Install the necessary Python packages using the commands provided in the notebook.
Model Details
Use the following Python code snippet to generate text using the model:
from transformers import AutoTokenizer, AutoModelForCausalLM import torch tokenizer = AutoTokenizer.from_pretrained("madhan2301/gemma-Instruct-Finetune-on-alpaca") model = AutoModelForCausalLM.from_pretrained( "madhan2301/gemma-Instruct-Finetune-on-alpaca", device_map="auto", torch_dtype=torch.bfloat16 ) input_text = "Write me a poem about Machine Learning." input_ids = tokenizer(input_text, return_tensors="pt").to("cuda") outputs = model.generate(**input_ids) print(tokenizer.decode(outputs[0]))
Model Description
This is the model card of a 🤗 transformers model that has been pushed on the Hub. This model card has been automatically generated.
- Developed by: [https://huggingface.co/madhan2301]
- Model type: [Instruct-Finetune-on-alpaca]
- Language(s) (NLP): [More Information Needed]
- License: [apache]
- Finetuned from model [optional]: [More Information Needed]
Model Sources [optional]
- Repository: [huggingface.co/madhan2301/gemma-Instruct-Finetune-on-alpaca]
- Paper [optional]: [More Information Needed]
- Demo [optional]: [More Information Needed]
Uses
Direct Use
[More Information Needed]
Downstream Use [optional]
[More Information Needed]
Out-of-Scope Use
[More Information Needed]
Bias, Risks, and Limitations
[More Information Needed]
Recommendations
Users (both direct and downstream) should be made aware of the risks, biases and limitations of the model. More information needed for further recommendations.
How to Get Started with the Model
Use the code below to get started with the model.
[More Information Needed]
Training Details
Training Data
[More Information Needed]
Training Procedure
Preprocessing [optional]
[More Information Needed]
Training Hyperparameters
- Training regime: [More Information Needed]
Speeds, Sizes, Times [optional]
[More Information Needed]
Evaluation
Testing Data, Factors & Metrics
Testing Data
[More Information Needed]
Factors
[More Information Needed]
Metrics
[More Information Needed]
Results
[More Information Needed]
Summary
Model Examination [optional]
[More Information Needed]
Environmental Impact
Carbon emissions can be estimated using the Machine Learning Impact calculator presented in Lacoste et al. (2019).
- Hardware Type: [More Information Needed]
- Hours used: [More Information Needed]
- Cloud Provider: [More Information Needed]
- Compute Region: [More Information Needed]
- Carbon Emitted: [More Information Needed]
Technical Specifications [optional]
Model Architecture and Objective
[More Information Needed]
Compute Infrastructure
[More Information Needed]
Hardware
[More Information Needed]
Software
[More Information Needed]
Citation [optional]
BibTeX:
[More Information Needed]
APA:
[More Information Needed]
Glossary [optional]
[More Information Needed]
More Information [optional]
[More Information Needed]
Model Card Authors [optional]
[More Information Needed]
Model Card Contact
[More Information Needed]
- Downloads last month
- 4