Michael Conrad
m-conrad-202
·
AI & ML interests
Cherokee Language, NLP, TTS
Recent Activity
replied to
singhsidhukuldeep's
post
about 2 months ago
Good folks from @Microsoft Research have just released bitnet.cpp, a game-changing inference framework that achieves remarkable performance gains.
Key Technical Highlights:
- Achieves speedups of up to 6.17x on x86 CPUs and 5.07x on ARM CPUs
- Reduces energy consumption by 55.4–82.2%
- Enables running 100B parameter models at human reading speed (5–7 tokens/second) on a single CPU
Features Three Optimized Kernels:
1. I2_S: Uses 2-bit weight representation
2. TL1: Implements 4-bit index lookup tables for every two weights
3. TL2: Employs 5-bit compression for every three weights
Performance Metrics:
- Lossless inference with 100% accuracy compared to full-precision models
- Tested across model sizes from 125M to 100B parameters
- Evaluated on both Apple M2 Ultra and Intel i7-13700H processors
This breakthrough makes running large language models locally more accessible than ever, opening new possibilities for edge computing and resource-constrained environments.
replied to
singhsidhukuldeep's
post
about 2 months ago
Good folks from @Microsoft Research have just released bitnet.cpp, a game-changing inference framework that achieves remarkable performance gains.
Key Technical Highlights:
- Achieves speedups of up to 6.17x on x86 CPUs and 5.07x on ARM CPUs
- Reduces energy consumption by 55.4–82.2%
- Enables running 100B parameter models at human reading speed (5–7 tokens/second) on a single CPU
Features Three Optimized Kernels:
1. I2_S: Uses 2-bit weight representation
2. TL1: Implements 4-bit index lookup tables for every two weights
3. TL2: Employs 5-bit compression for every three weights
Performance Metrics:
- Lossless inference with 100% accuracy compared to full-precision models
- Tested across model sizes from 125M to 100B parameters
- Evaluated on both Apple M2 Ultra and Intel i7-13700H processors
This breakthrough makes running large language models locally more accessible than ever, opening new possibilities for edge computing and resource-constrained environments.
Organizations
None yet
m-conrad-202's activity
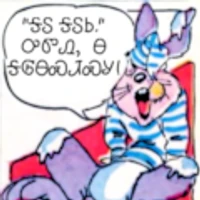
replied to
singhsidhukuldeep's
post
about 2 months ago
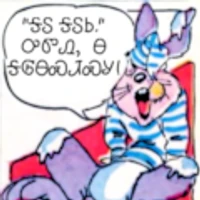
replied to
singhsidhukuldeep's
post
about 2 months ago
A proper link to the Microsoft page would be appreciated.
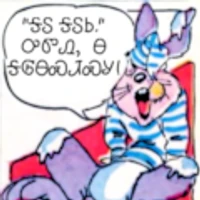
reacted to
DmitryRyumin's
post with 🔥
6 months ago
Post
3638
🚀🎭🌟 New Research Alert - Portrait4D-v2 (Avatars Collection)! 🌟🎭🚀
📄 Title: Portrait4D-v2: Pseudo Multi-View Data Creates Better 4D Head Synthesizer 🔝
📝 Description: Portrait4D-v2 is a novel method for one-shot 4D head avatar synthesis using pseudo multi-view videos and a vision transformer backbone, achieving superior performance without relying on 3DMM reconstruction.
👥 Authors: Yu Deng, Duomin Wang, and Baoyuan Wang
📄 Paper: Portrait4D-v2: Pseudo Multi-View Data Creates Better 4D Head Synthesizer (2403.13570)
🌐 GitHub Page: https://yudeng.github.io/Portrait4D-v2/
📁 Repository: https://github.com/YuDeng/Portrait-4D
📺 Video: https://www.youtube.com/watch?v=5YJY6-wcOJo
🚀 CVPR-2023-24-Papers: https://github.com/DmitryRyumin/CVPR-2023-24-Papers
📚 More Papers: more cutting-edge research presented at other conferences in the DmitryRyumin/NewEraAI-Papers curated by @DmitryRyumin
🚀 Added to the Avatars Collection: DmitryRyumin/avatars-65df37cdf81fec13d4dbac36
🔍 Keywords: Portrait4D #4DAvatar #HeadSynthesis #3DModeling #TechInnovation #DeepLearning #ComputerGraphics #ComputerVision #Innovation
📄 Title: Portrait4D-v2: Pseudo Multi-View Data Creates Better 4D Head Synthesizer 🔝
📝 Description: Portrait4D-v2 is a novel method for one-shot 4D head avatar synthesis using pseudo multi-view videos and a vision transformer backbone, achieving superior performance without relying on 3DMM reconstruction.
👥 Authors: Yu Deng, Duomin Wang, and Baoyuan Wang
📄 Paper: Portrait4D-v2: Pseudo Multi-View Data Creates Better 4D Head Synthesizer (2403.13570)
🌐 GitHub Page: https://yudeng.github.io/Portrait4D-v2/
📁 Repository: https://github.com/YuDeng/Portrait-4D
📺 Video: https://www.youtube.com/watch?v=5YJY6-wcOJo
🚀 CVPR-2023-24-Papers: https://github.com/DmitryRyumin/CVPR-2023-24-Papers
📚 More Papers: more cutting-edge research presented at other conferences in the DmitryRyumin/NewEraAI-Papers curated by @DmitryRyumin
🚀 Added to the Avatars Collection: DmitryRyumin/avatars-65df37cdf81fec13d4dbac36
🔍 Keywords: Portrait4D #4DAvatar #HeadSynthesis #3DModeling #TechInnovation #DeepLearning #ComputerGraphics #ComputerVision #Innovation
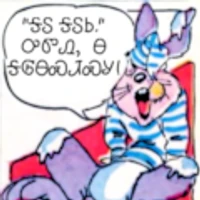
reacted to
Fizzarolli's
post with 👍
7 months ago
Post
2330
Is anyone looking into some sort of decentralized/federated dataset generation or classification by humans instead of synthetically?
From my experience with trying models, a *lot* of modern finetunes are trained on what amounts to, in essence, GPT-4 generated slop that makes everything sound like a rip-off GPT-4 (refer to i.e. the Dolphin finetunes). I have a feeling that this is a lot of the reason people haven't been quite as successful as Meta's instruct tunes of Llama 3.
From my experience with trying models, a *lot* of modern finetunes are trained on what amounts to, in essence, GPT-4 generated slop that makes everything sound like a rip-off GPT-4 (refer to i.e. the Dolphin finetunes). I have a feeling that this is a lot of the reason people haven't been quite as successful as Meta's instruct tunes of Llama 3.
Is there a way to use IPA phonetics?
#13 opened 8 months ago
by
m-conrad-202
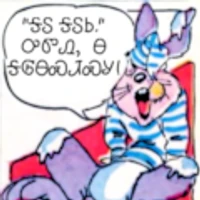
Can IPA phonetics be used with this model?
#8 opened 8 months ago
by
m-conrad-202
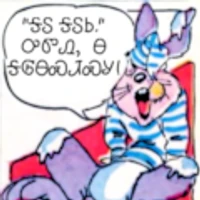
Is there a way to use IPA symbols?
#42 opened 8 months ago
by
m-conrad-202
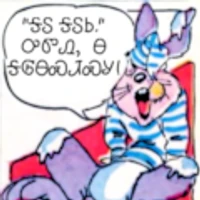
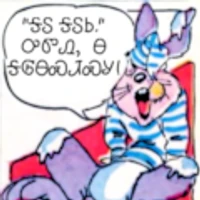
reacted to
abidlabs's
post with ❤️
8 months ago
Post
3617
Open Models vs. Closed APIs for Software Engineers
-----------------------------------------------------------------------
If you're an ML researcher / scientist, you probably don't need much convincing to use open models instead of closed APIs -- open models give you reproducibility and let you deeply investigate the model's behavior.
But what if you are a software engineer building products on top of LLMs? I'd argue that open models are a much better option even if you are using them as APIs. For at least 3 reasons:
1) The most obvious reason is reliability of your product. Relying on a closed API means that your product has a single point-of-failure. On the other hand, there are at least 7 different API providers that offer Llama3 70B already. As well as libraries that abstract on top of these API providers so that you can make a single request that goes to different API providers depending on availability / latency.
2) Another benefit is eventual consistency going local. If your product takes off, it will be more economical and lower latency to have a dedicated inference endpoint running on your VPC than to call external APIs. If you've started with an open-source model, you can always deploy the same model locally. You don't need to modify prompts or change any surrounding logic to get consistent behavior. Minimize your technical debt from the beginning.
3) Finally, open models give you much more flexibility. Even if you keep using APIs, you might want to tradeoff latency vs. cost, or use APIs that support batches of inputs, etc. Because different API providers have different infrastructure, you can use the API provider that makes the most sense for your product -- or you can even use multiple API providers for different users (free vs. paid) or different parts of your product (priority features vs. nice-to-haves)
-----------------------------------------------------------------------
If you're an ML researcher / scientist, you probably don't need much convincing to use open models instead of closed APIs -- open models give you reproducibility and let you deeply investigate the model's behavior.
But what if you are a software engineer building products on top of LLMs? I'd argue that open models are a much better option even if you are using them as APIs. For at least 3 reasons:
1) The most obvious reason is reliability of your product. Relying on a closed API means that your product has a single point-of-failure. On the other hand, there are at least 7 different API providers that offer Llama3 70B already. As well as libraries that abstract on top of these API providers so that you can make a single request that goes to different API providers depending on availability / latency.
2) Another benefit is eventual consistency going local. If your product takes off, it will be more economical and lower latency to have a dedicated inference endpoint running on your VPC than to call external APIs. If you've started with an open-source model, you can always deploy the same model locally. You don't need to modify prompts or change any surrounding logic to get consistent behavior. Minimize your technical debt from the beginning.
3) Finally, open models give you much more flexibility. Even if you keep using APIs, you might want to tradeoff latency vs. cost, or use APIs that support batches of inputs, etc. Because different API providers have different infrastructure, you can use the API provider that makes the most sense for your product -- or you can even use multiple API providers for different users (free vs. paid) or different parts of your product (priority features vs. nice-to-haves)
Instruct format?
3
#44 opened 8 months ago
by
m-conrad-202
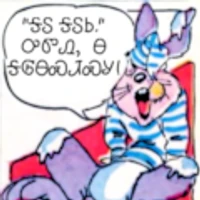
Hardware Requirements?
4
#10 opened over 1 year ago
by
cameronraygun
What about inference speed ?
2
#35 opened over 1 year ago
by
Pitchboy
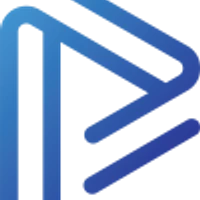