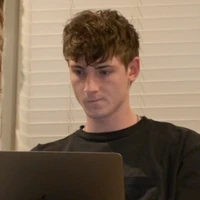
Datasets:
π° NewsMediaBias-Plus Dataset
π Overview
NewsMediaBias-Plus is a multimodal dataset designed for the analysis of media bias and disinformation through the combination of textual and visual data from news articles. This dataset aims to foster research and development in detecting, categorizing, and understanding the nuances of biased reporting and the dissemination of information in media outlets.
π Dataset Description
The NewsMediaBias-Plus dataset comprises news articles paired with relevant images, complete with annotations that reflect perceived biases and the reliability of the content. It extends existing datasets by adding a multimodal dimension, offering new opportunities for comprehensive bias detection in news media.
π Additional Resources
- π Dataset website
- πΌοΈ Full Version and Images Download
π Contents
unique_id
: Unique identifier for each news item. Eachunique_id
is associated with the image (top image) for the same news article.outlet
: Publisher of the news article.headline
: Headline of the news article.article_text
: Full text content of the news article.image_description
: Description of the image paired with the article.image
: File path of the image associated with the article.date_published
: Publication date of the news article.source_url
: Original URL of the news article.canonical_link
: Canonical URL of the news article, if different from the source URL.new_categories
: Categories assigned to the article.news_categories_confidence_scores
: Confidence scores for the assigned categories.
π·οΈ Annotation Labels
text_label
: Annotation for the textual content, indicating:'Likely'
: Likely to be disinformation.'Unlikely'
: Unlikely to be disinformation.
multimodal_label
: Annotation for the combined text snippet (first paragraph of the news story) and image content, assessing:'Likely'
: Likely to be disinformation.'Unlikely'
: Unlikely to be disinformation.
Benchmarking Results: Text-Based Models
Model | Configuration | Precision | Recall | F1 | Test Accuracy |
---|---|---|---|---|---|
Small Language Models | |||||
BERT-base-uncased | FT | 0.8887 | 0.8870 | 0.8878 | 0.8870 |
DistilBERT | FT | 0.8665 | 0.8554 | 0.8609 | 0.8710 |
RoBERTa-base | FT | 0.8940 | 0.8940 | 0.8940 | 0.8940 |
GPT2 | FT | 0.8762 | 0.8751 | 0.8756 | 0.8751 |
BART | FT | 0.8762 | 0.8760 | 0.8761 | 0.8760 |
Large Language Models | |||||
Llama 3.1-8B-instruct | 0-shot | 0.8280 | 0.6890 | 0.7521 | 0.7200 |
5-shot | 0.8400 | 0.7700 | 0.8035 | 0.7905 | |
IFT | 0.8019 | 0.8019 | 0.8019 | 0.8180 | |
Llama 3.1-8B (base) | FT | 0.8800 | 0.8600 | 0.8699 | 0.8320 |
Llama 3.2-3B-instruct | 0-shot | 0.7386 | 0.7550 | 0.7467 | 0.6897 |
5-shot | 0.7989 | 0.6840 | 0.7370 | 0.6133 | |
IFT | 0.8390 | 0.7984 | 0.8182 | 0.8084 | |
Llama 3.2-3B (base) | FT | 0.8400 | 0.8500 | 0.8450 | 0.8200 |
Mistral-v0.3 7B-instruct | 0-shot | 0.8153 | 0.5250 | 0.6387 | 0.6990 |
5-shot | 0.8319 | 0.8134 | 0.8225 | 0.7830 | |
IFT | 0.8890 | 0.9240 | 0.9062 | 0.7980 | |
Mistral-v0.3 7B (base) | FT | 0.8200 | 0.7400 | 0.7779 | 0.8014 |
Qwen2.5-7B | 0-shot | 0.8576 | 0.8576 | 0.8576 | 0.8576 |
5-shot | 0.8660 | 0.8790 | 0.8724 | 0.8900 | |
IFT | 0.8357 | 0.8474 | 0.8415 | 0.8474 |
Table 1: Performance metrics for various language models and configurations. Configuration types: 0-shot = No prior examples used for inference, 5-shot = Five examples provided for context before inference, FT = Fine-tuned on task-specific data, IFT = Instruction fine-tuned with targeted training.
Benchmarking Results: Text-Image Models
Model | Config. (Text-Image) | Precision | Recall | F1 | Test Accuracy |
---|---|---|---|---|---|
Small Language Models | |||||
SpotFake (XLNET + VGG-19) | FT | 0.7415 | 0.6790 | 0.7089 | 0.8151 |
BERT + ResNet-34 | FT | 0.8311 | 0.6277 | 0.7152 | 0.8248 |
FND-CLIP (BERT and CLIP) | FT | 0.6935 | 0.7151 | 0.7041 | 0.8971 |
Distill-RoBERTa and CLIP | FT | 0.7000 | 0.6600 | 0.6794 | 0.8600 |
Large Vision Language Models | |||||
Phi-3-vision-128k-instruct | 0-shot | 0.7400 | 0.6700 | 0.7033 | 0.7103 |
Phi-3-vision-128k-instruct | 5-shot | 0.7600 | 0.7200 | 0.7395 | 0.7024 |
Phi-3-vision-128k-instruct | IFT | 0.7800 | 0.8000 | 0.7899 | 0.7200 |
LLaVA-1.6 | 0-shot | 0.7531 | 0.6466 | 0.6958 | 0.6500 |
LLaVA-1.6 | 5-shot | 0.7102 | 0.6893 | 0.6996 | 0.6338 |
Llama-3.2-11B-Vision-Instruct | 0-shot | 0.6668 | 0.7233 | 0.6939 | 0.7060 |
Llama-3.2-11B-Vision-Instruct | 5-shot | 0.7570 | 0.7630 | 0.7600 | 0.7299 |
Llama-3.2-11B-Vision-Instruct | IFT | 0.7893 | 0.8838 | 0.8060 | 0.9040 |
Table 2: Performance metrics for various small and large language models in text-image configurations. Configuration types: 0-shot = No prior examples used for inference, 5-shot = Five examples provided for context before inference, FT = Fine-tuning, IFT = Instruction Fine-tuning.
π Getting Started
π Prerequisites
- Python 3.6 or later
- Pandas
- Datasets (from Hugging Face)
- Hugging Face Hub
π» Installation
pip install pandas datasets
π Usage
Load the full dataset into your Python environment:
from datasets import load_dataset
ds = load_dataset("vector-institute/newsmediabias-plus")
print(ds) # Displays structure and splits
print(ds['train'][0]) # Access the first element of the train split
print(ds['train'][:5]) # Access the first five elements of the train split
Load a few records:
from datasets import load_dataset
# Load the dataset in streaming mode
streamed_dataset = load_dataset("vector-institute/newsmediabias-plus", streaming=True)
# Get an iterable dataset
dataset_iterable = streamed_dataset['train'].take(5) # Change 'train' to the appropriate split if needed
# Print the records
for record in dataset_iterable:
print(record)
π€ Contributions
Contributions to this dataset are welcome. You can contribute in several ways:
- Data Contribution: Add more data points to enhance the datasetβs utility.
- Annotation Improvement: Help refine the annotations for better accuracy.
- Usage Examples: Contribute usage examples to help the community understand how to leverage this dataset effectively.
To contribute, please fork the repository and create a pull request with your proposed changes.
π License
This dataset is released under a specific license CC BY-NC: Attribution-NonCommercial 4.0 that allows for non-commercial use.
π Citation
If you use the NewsMediaBias-Plus dataset in your research, please cite it using the following BibTeX entry:
@misc{vector_institute_2024_newsmediabias_plus,
title={NewsMediaBias-Plus: A Multimodal Dataset for Analyzing Media Bias},
author={Vector Institute Research Team},
year={2024},
url={https://huggingface.co/datasets/vector-institute/newsmediabias-plus}
}
π§ Contact
For any questions or support related to this dataset, please contact shaina.raza@vectorinstitute.ai.
π₯ Dataset Creators
Creators: Shaina Raza*, Franklin Ogidi, Emrul Hasan, Caesar Saleh, Veronica Chatrath, Maximus Powers, Marcelo Lotif
Advisors: Arash Afkanpour, Aditya Jain, Gias uddin, Deval Pandya
β οΈ Disclaimer and Guidance for Users
Disclaimer: The classifications of 'Likely' and 'Unlikely' disinformation are based on LLM-based annotations and assessments by content experts and are intended for informational purposes only. They should not be seen as definitive or used to label entities definitively without further analysis.
Guidance for Users: This dataset is intended to encourage critical engagement with media content. Users are advised to use these annotations as a starting point for deeper analysis and to cross-reference findings with reliable sources. Please approach the data with an understanding of its intended use as a tool for research and awareness, not as a conclusive judgment.
- Downloads last month
- 155
Models trained or fine-tuned on vector-institute/newsmediabias-plus
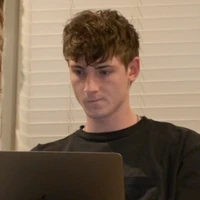