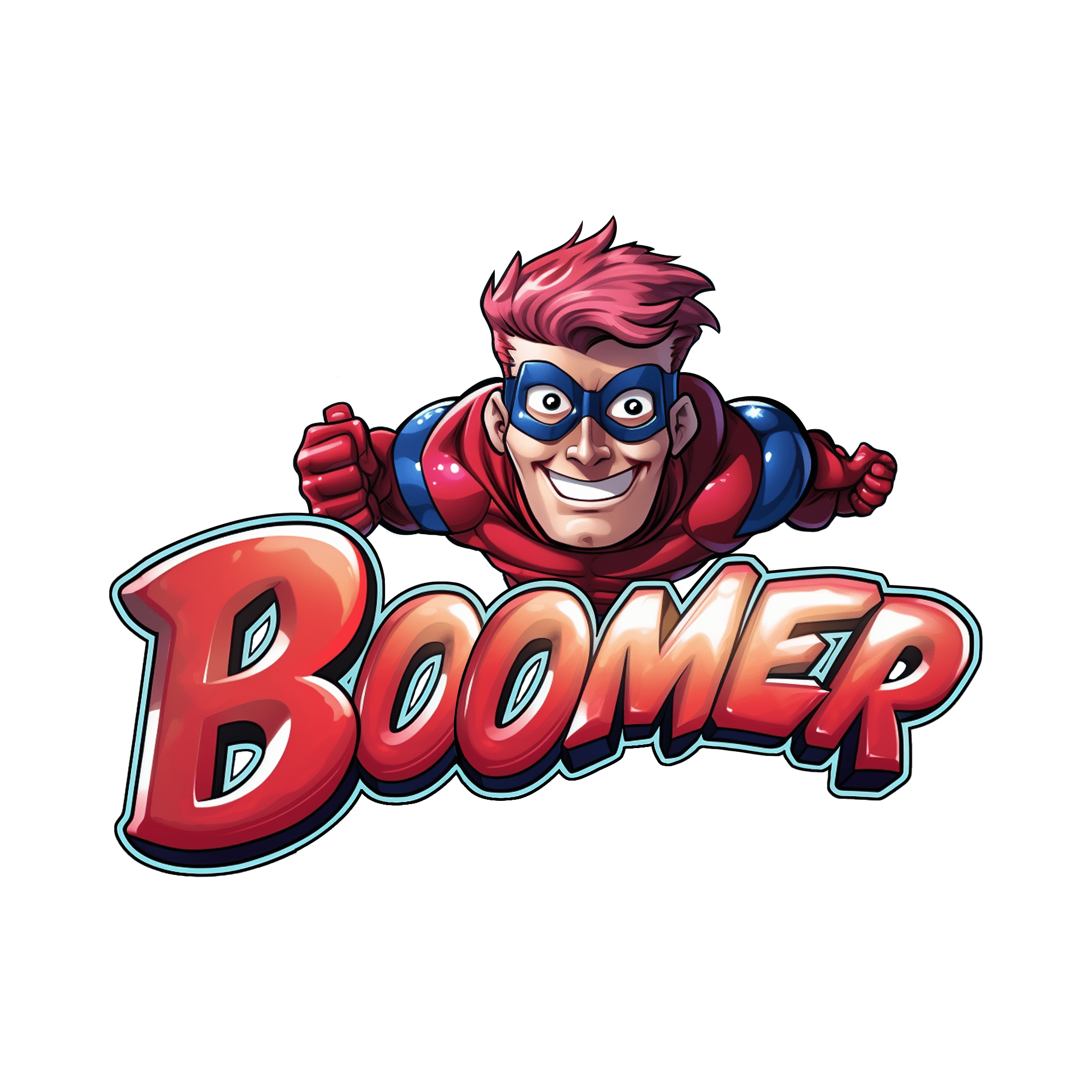
Democratizing access to LLMs for the open-source community.
Let's advance AI, together.
Introduction π
We are open-sourcing one of our early experiments of pretraining with custom architecture and datasets. This 1.1B parameter model is pre-trained from scratch using a custom-curated dataset of 41B tokens. The model's architecture experiments contain the addition of flash attention and a higher intermediate dimension of the MLP layer. The dataset is a combination of wiki, stories, arxiv, math and code. The model is available on huggingface Boomer1B
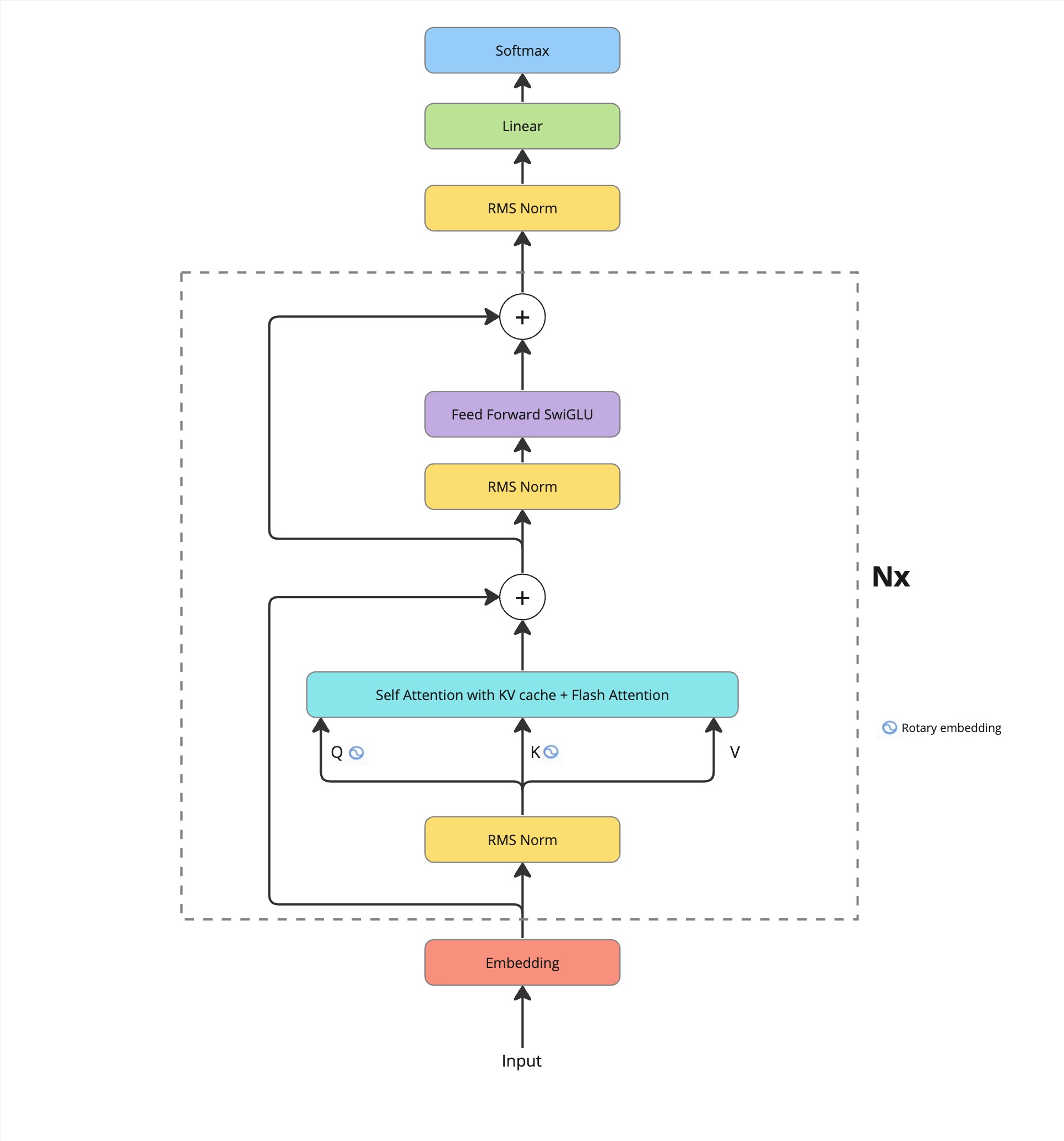
Getting Started on GitHub π»
Ready to dive in? Here's how you can get started with our models on GitHub.
Install the necessary dependencies with the following command:
pip install -r requirements.txt
Generate responses
Now that your model is fine-tuned, you're ready to generate responses. You can do this using our generate.py script, which runs inference from the Hugging Face model hub and inference on a specified input. Here's an example of usage:
python generate.py --base_model 'budecosystem/boomer-1b' --prompt="the president of India is"
Fine-tuning π―
It's time to upgrade the model by fine-tuning the model. You can do this using our provided finetune.py script. Here's an example command:
torchrun --nproc_per_node 4 train.py \
--base_model budecosystem/boomer-1b \
--data_path dataset.json \
--output_dir output \
--per_device_train_batch_size 2 \
--gradient_accumulation_steps 2 \
--num_train_epochs 1 \
--learning_rate 2e-5 \
--fp16 True \
--logging_steps 10 \
--deepspeed ds_config.json
Model details
Parameters | Value |
---|---|
n_layers | 4 |
n_heads | 32 |
d_model | 4096 |
vocab size | 32000 |
sequence length | 4096 |
Intermediate size | 11008 |
Tokenizer
We used the SentencePiece tokenizer during the fine-tuning process. This tokenizer is known for its capability to handle open-vocabulary language tasks efficiently.
Training details
The model is trained of 4 A100 80GB for approximately 250hrs.
Hyperparameters | Value |
---|---|
per_device_train_batch_size | 2 |
gradient_accumulation_steps | 2 |
learning_rate | 2e-4 |
optimizer | adamw |
beta | 0.9, 0.95 |
fp16 | True |
GPU | 4 A100 80GB |
Evaluations
We have evaluated the pre-trained model on few of the benchmarks
Model Name | ARC | MMLU | Human Eval | Hellaswag | BBH | DROP | GSM8K |
---|---|---|---|---|---|---|---|
Boomer1B | 22.35 | 25.92 | 6.1 | 31.66 | 28.65 | 6.13 | 1.5 |
Why use BOOMER?
- Retrieval augmentation
- Inference at the edge
- Language modeling use cases
Final thought on Boomer!
This isn't the end. It's just the beginning of a journey towards creating more advanced, more efficient, and more accessible language models. We invite you to join us on this exciting journey.
Aknowledgements
We'd like to thank the open-source community and the researchers whose foundational work laid the path for BOOMER. Special shoutout to our dedicated team who have worked relentlessly to curate the dataset and fine-tune the model to perfection.
- Downloads last month
- 2,085