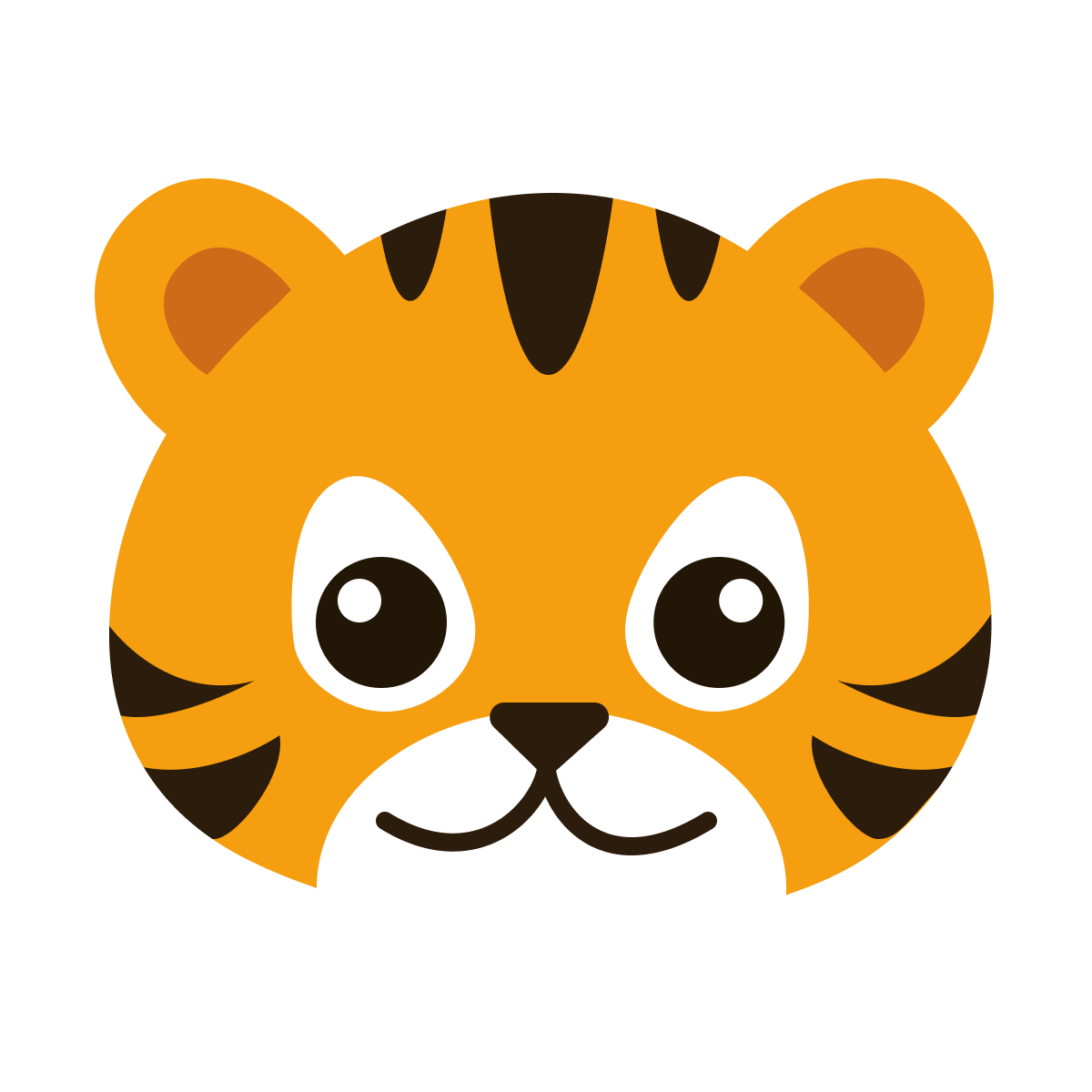
A cutting-edge foundation for your very own LLM.
๐ TigerBot โข ๐ค Hugging Face
Github
https://github.com/TigerResearch/TigerBot
Usage
from transformers import AutoTokenizer, AutoModelForCausalLM
from accelerate import infer_auto_device_map, dispatch_model
from accelerate.utils import get_balanced_memory
tokenizer = AutoTokenizer.from_pretrained("TigerResearch/tigerbot-180b-research")
model = AutoModelForCausalLM.from_pretrained("TigerResearch/tigerbot-180b-research")
max_memory = get_balanced_memory(model)
device_map = infer_auto_device_map(model, max_memory=max_memory, no_split_module_classes=["BloomBlock"])
model = dispatch_model(model, device_map=device_map, offload_buffers=True)
device = torch.cuda.current_device()
tok_ins = "\n\n### Instruction:\n"
tok_res = "\n\n### Response:\n"
prompt_input = tok_ins + "{instruction}" + tok_res
input_text = "What is the next number after this list: [1, 2, 3, 5, 8, 13, 21]"
input_text = prompt_input.format_map({'instruction': input_text})
max_input_length = 512
max_generate_length = 1024
generation_kwargs = {
"top_p": 0.95,
"temperature": 0.8,
"max_length": max_generate_length,
"eos_token_id": tokenizer.eos_token_id,
"pad_token_id": tokenizer.pad_token_id,
"early_stopping": True,
"no_repeat_ngram_size": 4,
}
inputs = tokenizer(input_text, return_tensors='pt', truncation=True, max_length=max_input_length)
inputs = {k: v.to(device) for k, v in inputs.items()}
output = model.generate(**inputs, **generation_kwargs)
answer = ''
for tok_id in output[0][inputs['input_ids'].shape[1]:]:
if tok_id != tokenizer.eos_token_id:
answer += tokenizer.decode(tok_id)
print(answer)
- Downloads last month
- 28
This model does not have enough activity to be deployed to Inference API (serverless) yet. Increase its social
visibility and check back later, or deploy to Inference Endpoints (dedicated)
instead.