Stephen Genusa PRO
AI & ML interests
Recent Activity
Organizations
StephenGenusa's activity
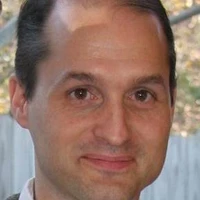
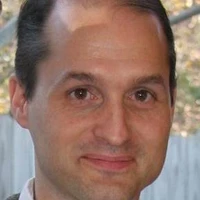
In this article, I share my latest Gen AI and LLM advances, featuring innovative approaches radically different from both standard AI and classical ML/NLP. The focus is on doing better with less, using efficient architectures, new algorithms and evaluation metrics. It originates from research that I started long ago. It gained significant momentum in the last two years. See background and history at https://mltblog.com/4g2sKTv.
OpenAI, Perplexity, Anthropic, Llama and others typically follow the trend and implement solutions very similar to mines within 3 to 6 months after I publish new milestones. For instance, multi-tokens, knowledge graph tokens, multi-indexes, real-time fine-tuning, mixtures of experts, LLM routers, small enterprise sub-LLMs, prompt distillation, relevancy scoring engine, deep contextual retrieval, optimum agentic chunking, and modern UI instead of the basic prompt box. I keep adding new features all the time, staying ahead of competition.
โก๏ธ Read full article with links to GitHub, at https://mltblog.com/3DsyZSq
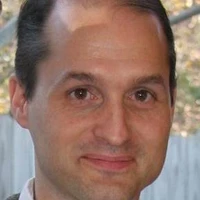
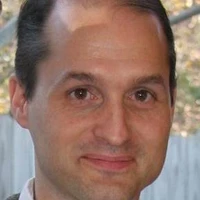
RAG systems are supposed to make your LLM's answer more trustworthy, by inserting in the prompt some supporting documents from a knowledge base : we say that we're "adding some context".
๐ But if you don't know which part of the answer has been generated based on which input tokens, it's hard to tell wether it was effectively grounded in the context knowledge or not!
๐ค I've been working on the question: is it possible to add notes to the answer linking to which part of the context they're generated from?
And I've found a great solution: a great technique called Layer-wise Relevance Propagation (LRP), showcased in a paper at ICML `24 by Reduan Achtibat et al allows, allows to precisely score how important each input token was in generating your output! They've made it into a library called LXT.
๐ For each generated output token, LXT gives you attribution scores for each input token.
โ๏ธ So I've worked a bit more on aggregating these scores into meaningful spans between successive input and output tokens, and I finally obtained my desired result: RAG with source highlighting!
Try the demo here ๐ m-ric/rag_highlights
Caveats:
- It slows down generation (for now quite a lot, could hopefully be reduced a lot)
- For now it supports only specific models: Llama models and Mixtral
If there's enough interest in this solution, I can improve it further and spin it off into a specific library for RAG! ๐
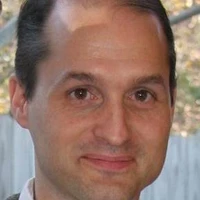
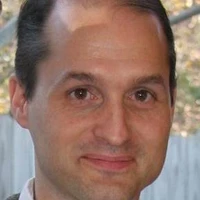
๐ฉ Report: Ethical issue(s)
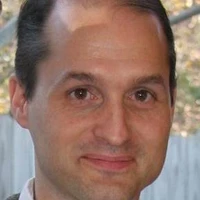
I think there will be a big breakthrough as well, but I'd be surprised if it happens soon. If it does, I'd be happy. While the architectures of LLMs continue to advance I don't see any evidence that significant progress is being made and I personally think the architectures are too primitive and inherently self-limiting. I am also a believer that bigger does not necessarily mean better. I think we've reached the limits or are near the point of reaching the limits of where size dictates how powerful the LLM is.
Therefore, I think, given the current architectural limitations, the external limits, namely those dictated by power availability, and the many resources/costs of building better LLMs, will slow AI development until a radical change comes along.
We've managed to survive without them and now that we have them, they are a great step forward and we'll continue using and improving what we have. There are many improvements that can be made around the LLM using NLP to improve what we expect from LLMs and that's where the focus will turn for the time being, such as xLLM. Better architectures are going to have to take into account the difference in statistical models of representations of the world and the way humans communicate through speech and writing.
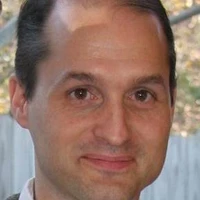
Vincent, thank you for your time, effort and especially for your willingness to share your expertise. I am really looking forward to this!
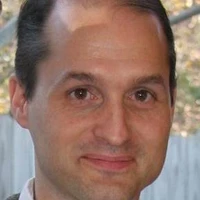
New additions to this ground-breaking system include multi-token distillation when processing prompts, agents to meet user intent, more NLP, and a command prompt menu accepting both standard prompts and various actions.
I also added several illustrations, featuring xLLM in action with a full session and sample commands to fine-tune in real-time. All the code, input sources (anonymized corporate corpus from fortune 100 company), contextual backend tables including embeddings, are on GitHub. My system has zero weight, no transformer, and no neural network. It relies on explainable AI, does not require training, is fully reproducible, and fits in memory. Yet your prompts can retrieve relevant full text entities from the corpus with no latency โ including URLs, categories, titles, email addresses, and so on โ thanks to well-designed architecture.
Read more, get the code, paper and everything for free, at https://mltblog.com/4dNPSnB
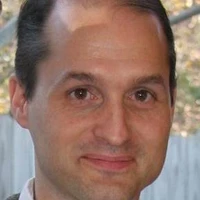
- Blogpost: https://huggingface.co/blog/falconmamba
- Link to collection: tiiuae/falconmamba-7b-66b9a580324dd1598b0f6d4a
- Link to playground: tiiuae/falcon-mamba-playground
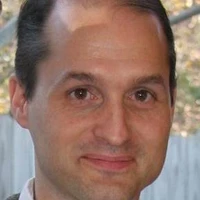
๐ Comprehensive Tutorial Video Link โถ๏ธ https://youtu.be/bupRePUOA18
FLUX represents a milestone in open source txt2img technology, delivering superior quality and more accurate prompt adherence than #Midjourney, Adobe Firefly, Leonardo Ai, Playground Ai, Stable Diffusion, SDXL, SD3, and Dall E3. #FLUX, a creation of Black Forest Labs, boasts a team largely comprised of #StableDiffusion's original developers, and its output quality is truly remarkable. This statement is not hyperbole; you'll witness its capabilities in the tutorial. This guide will demonstrate how to effortlessly install and utilize FLUX models on your personal computer and cloud platforms like Massed Compute, RunPod, and a complimentary Kaggle account.
๐ FLUX Setup Guide (publicly accessible) โคต๏ธ
โถ๏ธ https://www.patreon.com/posts/106135985
๐ FLUX Models One-Click Robust Automatic Downloader Scripts โคต๏ธ
โถ๏ธ https://www.patreon.com/posts/109289967
๐ Primary Windows SwarmUI Tutorial (Essential for Usage Instructions) โคต๏ธ
โถ๏ธ https://youtu.be/HKX8_F1Er_w
๐ Cloud-based SwarmUI Tutorial (Massed Compute - RunPod - Kaggle) โคต๏ธ
โถ๏ธ https://youtu.be/XFUZof6Skkw
๐ SECourses Discord Server for Comprehensive Support โคต๏ธ
โถ๏ธ https://discord.com/servers/software-engineering-courses-secourses-772774097734074388
๐ SECourses Reddit Community โคต๏ธ
โถ๏ธ https://www.reddit.com/r/SECourses/
๐ SECourses GitHub Repository โคต๏ธ
โถ๏ธ https://github.com/FurkanGozukara/Stable-Diffusion
๐ Official FLUX 1 Launch Announcement Blog Post โคต๏ธ
โถ๏ธ https://blackforestlabs.ai/announcing-black-forest-labs/
Video Segments
0:00 Introduction to the state-of-the-art open source txt2img model FLUX
5:01 Process for integrating FLUX model into SwarmUI
....