i noticed the models and code are not out yet, but they said they will release them shortly
Jaward Sesay
Jaward
AI & ML interests
I like to train large deep neural nets too 🧠🤖💥 | First Paper (AutoAgents: A Framework for Automatic Agent Generation) Accepted @ IJCAI 2024 | Role Model Karpathy
Recent Activity
replied to
their
post
1 day ago
Amazing work👏
Introduces Dream 7B - a discrete diffusion reasoning model, fully opensourced with weights on 🤗
- it outperforms existing non-autoregressive models and matches or beats frontier autoregressive of similar size on reasoning tasks.
Models:
- base: https://huggingface.co/Dream-org/Dream-v0-Base-7B
- SFT: https://huggingface.co/Dream-org/Dream-v0-Instruct-7B
Code: https://github.com/HKUNLP/Dream
Project: https://hkunlp.github.io/blog/2025/dream/
posted
an
update
2 days ago
Amazing work👏
Introduces Dream 7B - a discrete diffusion reasoning model, fully opensourced with weights on 🤗
- it outperforms existing non-autoregressive models and matches or beats frontier autoregressive of similar size on reasoning tasks.
Models:
- base: https://huggingface.co/Dream-org/Dream-v0-Base-7B
- SFT: https://huggingface.co/Dream-org/Dream-v0-Instruct-7B
Code: https://github.com/HKUNLP/Dream
Project: https://hkunlp.github.io/blog/2025/dream/
posted
an
update
3 days ago
Implements from first-principle recently proposed dynamic tanh as alternative to layernorm. Specifically, we trained a nanoGPT (0.8 M params) on tiny shakespeare with conventional layernorm, RMSNorm and dynamic tanh, then compared performances. Observed performance seems to match or is stable for α = 0.5~ 1.5, might outperform if trained longer.
Code: https://github.com/Jaykef/ai-algorithms/blob/main/Dynamic_Tanh.ipynb
Background music by 周子珺
Organizations
Jaward's activity
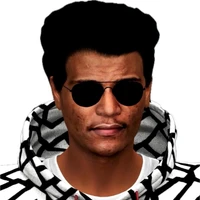
replied to
their
post
1 day ago
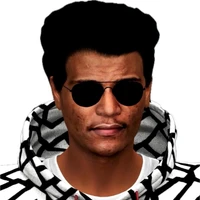
posted
an
update
2 days ago
Post
1715
Amazing work👏
Introduces Dream 7B - a discrete diffusion reasoning model, fully opensourced with weights on 🤗
- it outperforms existing non-autoregressive models and matches or beats frontier autoregressive of similar size on reasoning tasks.
Models:
- base: Dream-org/Dream-v0-Base-7B
- SFT: Dream-org/Dream-v0-Instruct-7B
Code: https://github.com/HKUNLP/Dream
Project: https://hkunlp.github.io/blog/2025/dream/
Introduces Dream 7B - a discrete diffusion reasoning model, fully opensourced with weights on 🤗
- it outperforms existing non-autoregressive models and matches or beats frontier autoregressive of similar size on reasoning tasks.
Models:
- base: Dream-org/Dream-v0-Base-7B
- SFT: Dream-org/Dream-v0-Instruct-7B
Code: https://github.com/HKUNLP/Dream
Project: https://hkunlp.github.io/blog/2025/dream/
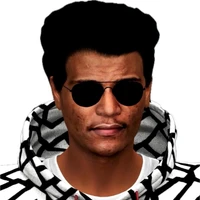
posted
an
update
3 days ago
Post
1810
Implements from first-principle recently proposed dynamic tanh as alternative to layernorm. Specifically, we trained a nanoGPT (0.8 M params) on tiny shakespeare with conventional layernorm, RMSNorm and dynamic tanh, then compared performances. Observed performance seems to match or is stable for α = 0.5~ 1.5, might outperform if trained longer.
Code: https://github.com/Jaykef/ai-algorithms/blob/main/Dynamic_Tanh.ipynb
Background music by 周子珺
Code: https://github.com/Jaykef/ai-algorithms/blob/main/Dynamic_Tanh.ipynb
Background music by 周子珺
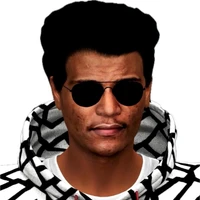
reacted to
clem's
post with 🚀
5 days ago
Post
3838
Before 2020, most of the AI field was open and collaborative. For me, that was the key factor that accelerated scientific progress and made the impossible possible—just look at the “T” in ChatGPT, which comes from the Transformer architecture openly shared by Google.
Then came the myth that AI was too dangerous to share, and companies started optimizing for short-term revenue. That led many major AI labs and researchers to stop sharing and collaborating.
With OAI and sama now saying they're willing to share open weights again, we have a real chance to return to a golden age of AI progress and democratization—powered by openness and collaboration, in the US and around the world.
This is incredibly exciting. Let’s go, open science and open-source AI!
Then came the myth that AI was too dangerous to share, and companies started optimizing for short-term revenue. That led many major AI labs and researchers to stop sharing and collaborating.
With OAI and sama now saying they're willing to share open weights again, we have a real chance to return to a golden age of AI progress and democratization—powered by openness and collaboration, in the US and around the world.
This is incredibly exciting. Let’s go, open science and open-source AI!
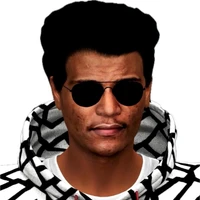
posted
an
update
13 days ago
Post
3617
Implemented a custom multimodal GRPO trainer that scales for Small VLMs, supports cpu and gpu with vllm + flash attention. Using SmolVLM-256M-Instruct reference & reward model, wasn’t trained for long btw, still got some sparks of “thinking”:)
Code: https://github.com/Jaykef/ai-algorithms/blob/main/grpo_multimodal_reasoner.ipynb
Code: https://github.com/Jaykef/ai-algorithms/blob/main/grpo_multimodal_reasoner.ipynb
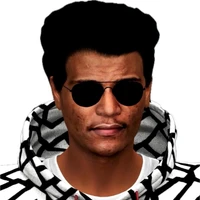
posted
an
update
16 days ago
Post
1747
Finally, the ground truth / AlexNet’s original source code is available to all.
Context: AlexNet had a historic win in the 2012 ImageNet Large Scale Visual Recognition Challenge (ILSVRC), reducing error rate from 26% (previous best) to 15.3%. It’s a deep CNN with 8 layers (5 convolutional + 3 fully connected), pioneering the use of ReLU activations for faster training, dropout for regularization, and GPU acceleration for large-scale learning. This moment marked the beginning of the deep learning revolution, inspiring architectures like VGG, ResNet, and modern transformers.
Code: https://github.com/computerhistory/AlexNet-Source-Code
Context: AlexNet had a historic win in the 2012 ImageNet Large Scale Visual Recognition Challenge (ILSVRC), reducing error rate from 26% (previous best) to 15.3%. It’s a deep CNN with 8 layers (5 convolutional + 3 fully connected), pioneering the use of ReLU activations for faster training, dropout for regularization, and GPU acceleration for large-scale learning. This moment marked the beginning of the deep learning revolution, inspiring architectures like VGG, ResNet, and modern transformers.
Code: https://github.com/computerhistory/AlexNet-Source-Code
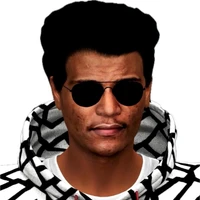
posted
an
update
17 days ago
Post
2101
Nvidia brings blue (from starwars droids) to life 🤯, supercute with flawless dexterity and droid voice. It's the result of their colab research with Google DeepMind and Disney, revealed as part of their new opensource physics engine for robotics simulation: NEWTON - which enables robots to learn how to complete complex tasks with greater precision.
ReadMore: https://developer.nvidia.com/blog/announcing-newton-an-open-source-physics-engine-for-robotics-simulation?ncid=so-twit-820797-vt48
ReadMore: https://developer.nvidia.com/blog/announcing-newton-an-open-source-physics-engine-for-robotics-simulation?ncid=so-twit-820797-vt48
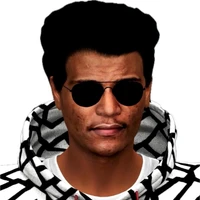
posted
an
update
24 days ago
Post
1912
This is the most exciting of this week’s release for me: Gemini Robotics - A SOTA generalist Vision-Language-Action model that brings intelligence to the physical world. It comes with a verifiable real-world knowledge Embodied Reasoning QA benchmark. Cool part is that the model can be specialized with fast adaptation to new tasks and have such adaptations transferred to new robot embodiment like humanoids. Looking forward to the model and data on hf, it’s about time I go full physical:)
Technical Report: https://storage.googleapis.com/deepmind-media/gemini-robotics/gemini_robotics_report.pdf
Technical Report: https://storage.googleapis.com/deepmind-media/gemini-robotics/gemini_robotics_report.pdf
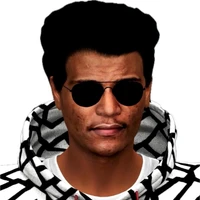
posted
an
update
26 days ago
Post
2014
Super Interesting Paper!
Proposes neural networks (CRNNs) that can learn to produce traveling waves in their hidden state in response to visual stimuli, thus enabling the transfer and integration of spatial information across neural connections. In other words they showed that neural networks have wave-like properties that blends and processes visual information over time, cool seeing a union of AI and physics in this way.
Paper: https://arxiv.org/pdf/2502.06034
Code: https://github.com/KempnerInstitute/traveling-waves-integrate
Proposes neural networks (CRNNs) that can learn to produce traveling waves in their hidden state in response to visual stimuli, thus enabling the transfer and integration of spatial information across neural connections. In other words they showed that neural networks have wave-like properties that blends and processes visual information over time, cool seeing a union of AI and physics in this way.
Paper: https://arxiv.org/pdf/2502.06034
Code: https://github.com/KempnerInstitute/traveling-waves-integrate
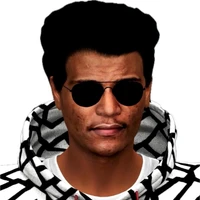
posted
an
update
27 days ago
Post
2582
Lightweight (nanoGPT) implementation of hybrid norm - an intuitive normalization method that combines the strength of both pre-norm (i.e QKV-norm in MHA) and post-norm in the feed-forward network.
Code: https://github.com/Jaykef/ai-algorithms/blob/main/hybrid_normalization.ipynb
Code: https://github.com/Jaykef/ai-algorithms/blob/main/hybrid_normalization.ipynb
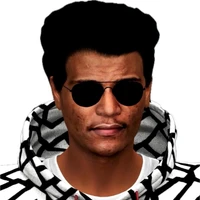
posted
an
update
about 1 month ago
Post
4964
made a few improvements on custom grpo trainer:
- added sequence similarity reward (seems to work)
- improved vllm support (5x inference speed)
- adjusted reward scores (this helped with format/accuracy)
- can now push to hf hub (already pushed mine lol: Jaward/smollm2_360m_grpo_gsm8k_reasoner)
Code: https://github.com/Jaykef/ai-algorithms/blob/main/smollm2_360M_135M_grpo_gsm8k.ipynb
- added sequence similarity reward (seems to work)
- improved vllm support (5x inference speed)
- adjusted reward scores (this helped with format/accuracy)
- can now push to hf hub (already pushed mine lol: Jaward/smollm2_360m_grpo_gsm8k_reasoner)
Code: https://github.com/Jaykef/ai-algorithms/blob/main/smollm2_360M_135M_grpo_gsm8k.ipynb
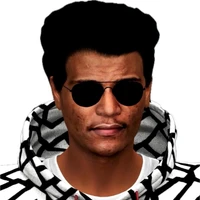
replied to
their
post
about 2 months ago
bro if you had read the repo you would see that this implementation is for educational purpose, it's not done because it's easy. Not to mention unsloth is using trl's GRPO trainer which is super slow on cpu and does not scale for models under 500M params, I tried it both on cpu and gpu. This custom implementation cuts most of the heavy lifting allowing you to train and scale faster even on cpu, plus a bunch of custom configs with a simplified GRPO trainer in under 500 lines of code. There's a lot one can learn from it.
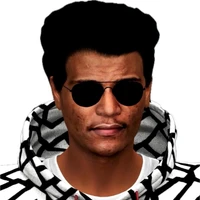
posted
an
update
about 2 months ago
Post
3896
Finally here it is: a faster, custom, scalable GRPO trainer for smaller models with < 500M params, can train on 8gb ram cpu, also supports gpu for sanity sake (includes support for vllm + flash attention). Using smolLM2-135M/360M-instructs as ref & base models. Experience your own “aha” moment 🐳 on 8gb ram.
Code: https://github.com/Jaykef/ai-algorithms/blob/main/smollm2_360M_135M_grpo_gsm8k.ipynb
Code: https://github.com/Jaykef/ai-algorithms/blob/main/smollm2_360M_135M_grpo_gsm8k.ipynb
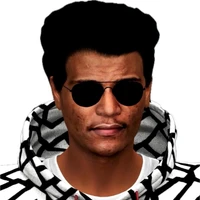
posted
an
update
2 months ago
Post
3553
ByteDance drops OmniHuman🔥
This is peak SOTA performance - flawless natural gestures with perfect lip sync and facial expressions. This is the second time they've released SOTA level talking-heads only this time with hands and body motion.
Project: https://omnihuman-lab.github.io/
This is peak SOTA performance - flawless natural gestures with perfect lip sync and facial expressions. This is the second time they've released SOTA level talking-heads only this time with hands and body motion.
Project: https://omnihuman-lab.github.io/
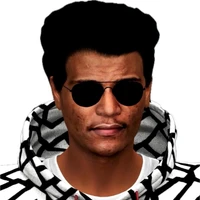
posted
an
update
2 months ago
Post
1526
The beauty in GRPO is the fact that it doesn’t care if the rewards are rule-based or learned, the hack: let the data self-normalize— trajectories in a batch compete against their mean, no value model, no extra params, just clean, efficient RL that cuts memory usage by 50%, while maintaining SOTA performance. btw it was introduced 9months prior to R1: arxiv.org/pdf/2402.03300
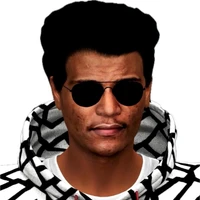
reacted to
mlabonne's
post with 🧠
3 months ago
Post
6305
🆕 LLM Course 2025 edition!
I updated the LLM Scientist roadmap and added a ton of new information and references. It covers training, datasets, evaluation, quantization, and new trends like test-time compute scaling.
The LLM Course has been incredibly popular (41.3k stars!) and I've been touched to receive many, many messages about how it helped people in their careers.
I know how difficult this stuff can be, so I'm super proud of the impact it had. I want to keep updating it in 2025, especially with the LLM Engineer roadmap.
Thanks everyone, hope you'll enjoy it!
💻 LLM Course: https://huggingface.co/blog/mlabonne/llm-course
I updated the LLM Scientist roadmap and added a ton of new information and references. It covers training, datasets, evaluation, quantization, and new trends like test-time compute scaling.
The LLM Course has been incredibly popular (41.3k stars!) and I've been touched to receive many, many messages about how it helped people in their careers.
I know how difficult this stuff can be, so I'm super proud of the impact it had. I want to keep updating it in 2025, especially with the LLM Engineer roadmap.
Thanks everyone, hope you'll enjoy it!
💻 LLM Course: https://huggingface.co/blog/mlabonne/llm-course
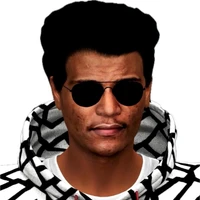
posted
an
update
3 months ago
Post
1890
minimal single script implementation of knowledge distillation in LLMs. In this implementation, we use GPT-2 (124M) as student model and GPT-2 Medium (340M) as teacher via reverse Kullback-Leibler (KL) divergence, trained on a small chunk of openwebtext.
Code: https://github.com/Jaykef/ai-algorithms/blob/main/llm_knowledge_distillation.ipynb
Code: https://github.com/Jaykef/ai-algorithms/blob/main/llm_knowledge_distillation.ipynb
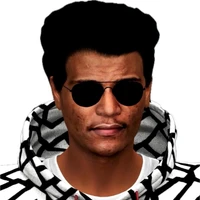
posted
an
update
3 months ago
Post
1383
Huge AI win in medicine👏
"Large language of life model" just dropped!!
Full paper: https://www.nature.com/articles/s41586-024-08391-z
"Large language of life model" just dropped!!
Full paper: https://www.nature.com/articles/s41586-024-08391-z
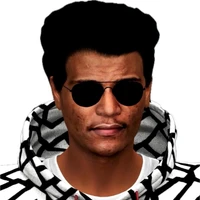
posted
an
update
3 months ago
Post
2341
damn I love nvidia's bullish stance on taking AI to the edge - from being the overlord of compute to cutting-edge physical AI with SOTA multiverse simulation engines that brings the scaling laws under your control!!
My favorite: Cosmos - fully opensourced, open-weight physics based video gen platform, what an incredible way to start off the year✨
Code: https://github.com/NVIDIA/Cosmos
Models: nvidia/cosmos-6751e884dc10e013a0a0d8e6
Paper: https://d1qx31qr3h6wln.cloudfront.net/publications/NVIDIA%20Cosmos_2.pdf
My favorite: Cosmos - fully opensourced, open-weight physics based video gen platform, what an incredible way to start off the year✨
Code: https://github.com/NVIDIA/Cosmos
Models: nvidia/cosmos-6751e884dc10e013a0a0d8e6
Paper: https://d1qx31qr3h6wln.cloudfront.net/publications/NVIDIA%20Cosmos_2.pdf
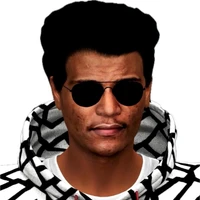
posted
an
update
3 months ago
Post
3118
nanoBLT: Simplified lightweight implementation of a character-level Byte Latent Transformer model (under 500 lines of code). The model is 2x4x2 (n_layers_encoder, n_layers_latent, n_layers_decoder) layer deep trained on ~1M bytes of tiny Shakespeare with a patch size of 4.
Code: https://github.com/Jaykef/ai-algorithms/blob/main/byte_latent_transformer.ipynb
Code: https://github.com/Jaykef/ai-algorithms/blob/main/byte_latent_transformer.ipynb