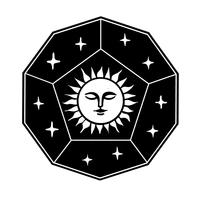
Timaeus
AI & ML interests
Singular Learning Theory & Developmental Interpretability
Recent Activity
Timaeus
About Us
Timaeus is a non-profit research organization founded in October 2023, focused on applying singular learning theory (SLT) to AI alignment. Our mission is to make fundamental breakthroughs in technical AI alignment using deep ideas from mathematics and the sciences.
Our Research Focus
We concentrate on understanding the relationship between internal structure in neural networks and the geometry of the loss landscape, as revealed by singular learning theory. This connection provides a basis for developing scalable tools for interpretability, mechanistic anomaly detection, and beyond.
Key Research Areas:
Developmental Interpretability (DevInterp): Applying SLT to interpret the development of structure in neural networks, aiming to identify when, where, and what circuits form.
Structural Generalization (StrucGen): Using SLT to study out-of-distribution generalization, with the goal of building tools to predict how circuits will "break".
Geometry of Program Synthesis (GPS): Applying SLT to study inductive biases, advancing our understanding of how to predict and measure alignment-relevant risks.
Resources
Connect With Us
For collaboration inquiries or more information about our research, please contact us at contact@timaeus.co