Spaces:
Runtime error
Runtime error
File size: 32,517 Bytes
47c361d |
1 2 3 4 5 6 7 8 9 10 11 12 13 14 15 16 17 18 19 20 21 22 23 24 25 26 27 28 29 30 31 32 33 34 35 36 37 38 39 40 41 42 43 44 45 46 47 48 49 50 51 52 53 54 55 56 57 58 59 60 61 62 63 64 65 66 67 68 69 70 71 72 73 74 75 76 77 78 79 80 81 82 83 84 85 86 87 88 89 90 91 92 93 94 95 96 97 98 99 100 101 102 103 104 105 106 107 108 109 110 111 112 113 114 115 116 117 118 119 120 121 122 123 124 125 126 127 128 129 130 131 132 133 134 135 136 137 138 139 140 141 142 143 144 145 146 147 148 149 150 151 152 153 154 155 156 157 158 159 160 161 162 163 164 165 166 167 168 169 170 171 172 173 174 175 176 177 178 179 180 181 182 183 184 185 186 187 188 189 190 191 192 193 194 195 196 197 198 199 200 201 202 203 204 205 206 207 208 209 210 211 212 213 214 215 216 217 218 219 220 221 222 223 224 225 226 227 228 229 230 231 232 233 234 235 236 237 238 239 240 241 242 243 244 245 246 247 248 249 250 251 252 253 254 255 256 257 258 259 260 261 262 263 264 265 266 267 268 269 270 271 272 273 274 275 276 277 278 279 280 281 282 283 284 285 286 287 288 289 290 291 292 293 294 295 296 297 298 299 300 301 302 303 304 305 306 307 308 309 310 311 312 313 314 315 316 317 318 319 320 321 322 323 324 325 326 327 328 329 330 331 332 333 334 335 336 337 338 339 340 341 342 343 344 345 346 347 348 349 350 351 352 353 354 355 356 357 358 359 360 361 362 363 364 365 366 367 368 369 370 371 372 373 374 375 376 377 378 379 380 381 382 383 384 385 386 387 388 389 390 391 392 393 394 395 396 397 398 399 400 401 402 403 404 405 406 407 408 409 410 411 412 413 414 415 416 417 418 419 420 421 422 423 424 425 426 427 428 429 430 431 432 433 434 435 436 437 438 439 440 441 442 443 444 445 446 447 448 449 450 451 452 453 454 455 456 457 458 459 460 461 462 |
# Parallel WaveGAN implementation with Pytorch
 [](https://pypi.org/project/parallel-wavegan/)   [](https://colab.research.google.com/github/espnet/notebook/blob/master/espnet2_tts_realtime_demo.ipynb)
This repository provides **UNOFFICIAL** pytorch implementations of the following models:
- [Parallel WaveGAN](https://arxiv.org/abs/1910.11480)
- [MelGAN](https://arxiv.org/abs/1910.06711)
- [Multiband-MelGAN](https://arxiv.org/abs/2005.05106)
- [HiFi-GAN](https://arxiv.org/abs/2010.05646)
- [StyleMelGAN](https://arxiv.org/abs/2011.01557)
You can combine these state-of-the-art non-autoregressive models to build your own great vocoder!
Please check our samples in [our demo HP](https://kan-bayashi.github.io/ParallelWaveGAN).
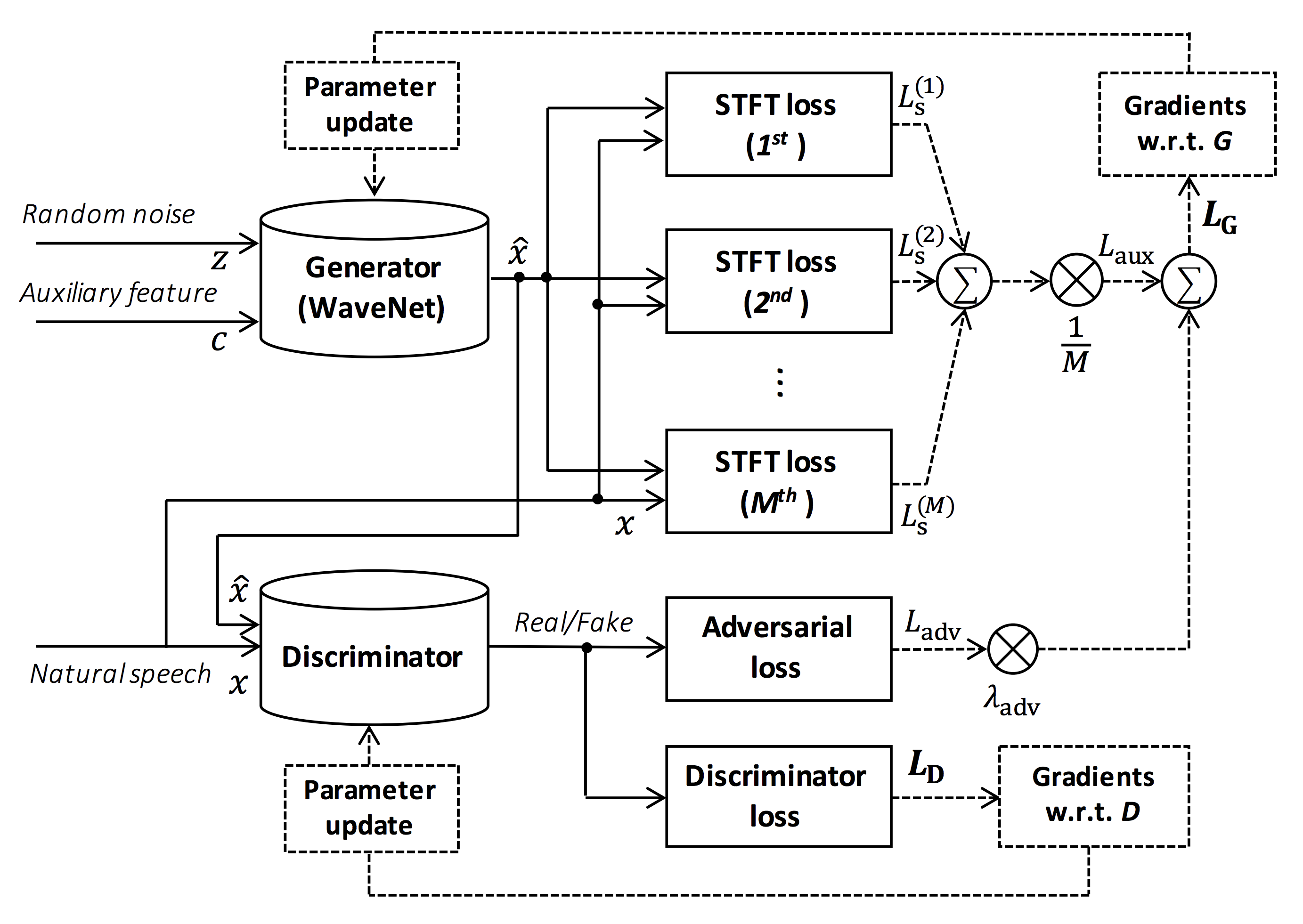
> Source of the figure: https://arxiv.org/pdf/1910.11480.pdf
The goal of this repository is to provide real-time neural vocoder, which is compatible with [ESPnet-TTS](https://github.com/espnet/espnet).
Also, this repository can be combined with [NVIDIA/tacotron2](https://github.com/NVIDIA/tacotron2)-based implementation (See [this comment](https://github.com/kan-bayashi/ParallelWaveGAN/issues/169#issuecomment-649320778)).
You can try the real-time end-to-end text-to-speech demonstration in Google Colab!
- Real-time demonstration with ESPnet2 [](https://colab.research.google.com/github/espnet/notebook/blob/master/espnet2_tts_realtime_demo.ipynb)
- Real-time demonstration with ESPnet1 [](https://colab.research.google.com/github/espnet/notebook/blob/master/tts_realtime_demo.ipynb)
## What's new
- 2021/08/24 Add more pretrained models of StyleMelGAN and HiFi-GAN.
- 2021/08/07 Add initial pretrained models of StyleMelGAN and HiFi-GAN.
- 2021/08/03 Support [StyleMelGAN](https://arxiv.org/abs/2011.01557) generator and discriminator!
- 2021/08/02 Support [HiFi-GAN](https://arxiv.org/abs/2010.05646) generator and discriminator!
- 2020/10/07 [JSSS](https://sites.google.com/site/shinnosuketakamichi/research-topics/jsss_corpus) recipe is available!
- 2020/08/19 [Real-time demo with ESPnet2](https://colab.research.google.com/github/espnet/notebook/blob/master/espnet2_tts_realtime_demo.ipynb) is available!
- 2020/05/29 [VCTK, JSUT, and CSMSC multi-band MelGAN pretrained model](#Results) is available!
- 2020/05/27 [New LJSpeech multi-band MelGAN pretrained model](#Results) is available!
- 2020/05/24 [LJSpeech full-band MelGAN pretrained model](#Results) is available!
- 2020/05/22 [LJSpeech multi-band MelGAN pretrained model](#Results) is available!
- 2020/05/16 [Multi-band MelGAN](https://arxiv.org/abs/2005.05106) is available!
- 2020/03/25 [LibriTTS pretrained models](#Results) are available!
- 2020/03/17 [Tensorflow conversion example notebook](https://github.com/kan-bayashi/ParallelWaveGAN/blob/master/notebooks/convert_melgan_from_pytorch_to_tensorflow.ipynb) is available (Thanks, [@dathudeptrai](https://github.com/dathudeptrai))!
- 2020/03/16 [LibriTTS recipe](https://github.com/kan-bayashi/ParallelWaveGAN/blob/master/egs/libritts/voc1) is available!
- 2020/03/12 [PWG G + MelGAN D + STFT-loss samples](#Results) are available!
- 2020/03/12 Multi-speaker English recipe [egs/vctk/voc1](https://github.com/kan-bayashi/ParallelWaveGAN/blob/master/egs/vctk/voc1) is available!
- 2020/02/22 [MelGAN G + MelGAN D + STFT-loss samples](#Results) are available!
- 2020/02/12 Support [MelGAN](https://arxiv.org/abs/1910.06711)'s discriminator!
- 2020/02/08 Support [MelGAN](https://arxiv.org/abs/1910.06711)'s generator!
## Requirements
This repository is tested on Ubuntu 20.04 with a GPU Titan V.
- Python 3.6+
- Cuda 10.0+
- CuDNN 7+
- NCCL 2+ (for distributed multi-gpu training)
- libsndfile (you can install via `sudo apt install libsndfile-dev` in ubuntu)
- jq (you can install via `sudo apt install jq` in ubuntu)
- sox (you can install via `sudo apt install sox` in ubuntu)
Different cuda version should be working but not explicitly tested.
All of the codes are tested on Pytorch 1.4, 1.5.1, 1.7.1, 1.8.1, and 1.9.
Pytorch 1.6 works but there are some issues in cpu mode (See #198).
## Setup
You can select the installation method from two alternatives.
### A. Use pip
```bash
$ git clone https://github.com/kan-bayashi/ParallelWaveGAN.git
$ cd ParallelWaveGAN
$ pip install -e .
# If you want to use distributed training, please install
# apex manually by following https://github.com/NVIDIA/apex
$ ...
```
Note that your cuda version must be exactly matched with the version used for the pytorch binary to install apex.
To install pytorch compiled with different cuda version, see `tools/Makefile`.
### B. Make virtualenv
```bash
$ git clone https://github.com/kan-bayashi/ParallelWaveGAN.git
$ cd ParallelWaveGAN/tools
$ make
# If you want to use distributed training, please run following
# command to install apex.
$ make apex
```
Note that we specify cuda version used to compile pytorch wheel.
If you want to use different cuda version, please check `tools/Makefile` to change the pytorch wheel to be installed.
## Recipe
This repository provides [Kaldi](https://github.com/kaldi-asr/kaldi)-style recipes, as the same as [ESPnet](https://github.com/espnet/espnet).
Currently, the following recipes are supported.
- [LJSpeech](https://keithito.com/LJ-Speech-Dataset/): English female speaker
- [JSUT](https://sites.google.com/site/shinnosuketakamichi/publication/jsut): Japanese female speaker
- [JSSS](https://sites.google.com/site/shinnosuketakamichi/research-topics/jsss_corpus): Japanese female speaker
- [CSMSC](https://www.data-baker.com/open_source.html): Mandarin female speaker
- [CMU Arctic](http://www.festvox.org/cmu_arctic/): English speakers
- [JNAS](http://research.nii.ac.jp/src/en/JNAS.html): Japanese multi-speaker
- [VCTK](https://homepages.inf.ed.ac.uk/jyamagis/page3/page58/page58.html): English multi-speaker
- [LibriTTS](https://arxiv.org/abs/1904.02882): English multi-speaker
- [YesNo](https://arxiv.org/abs/1904.02882): English speaker (For debugging)
To run the recipe, please follow the below instruction.
```bash
# Let us move on the recipe directory
$ cd egs/ljspeech/voc1
# Run the recipe from scratch
$ ./run.sh
# You can change config via command line
$ ./run.sh --conf <your_customized_yaml_config>
# You can select the stage to start and stop
$ ./run.sh --stage 2 --stop_stage 2
# If you want to specify the gpu
$ CUDA_VISIBLE_DEVICES=1 ./run.sh --stage 2
# If you want to resume training from 10000 steps checkpoint
$ ./run.sh --stage 2 --resume <path>/<to>/checkpoint-10000steps.pkl
```
See more info about the recipes in [this README](./egs/README.md).
## Speed
The decoding speed is RTF = 0.016 with TITAN V, much faster than the real-time.
```bash
[decode]: 100%|ββββββββββ| 250/250 [00:30<00:00, 8.31it/s, RTF=0.0156]
2019-11-03 09:07:40,480 (decode:127) INFO: finished generation of 250 utterances (RTF = 0.016).
```
Even on the CPU (Intel(R) Xeon(R) Gold 6154 CPU @ 3.00GHz 16 threads), it can generate less than the real-time.
```bash
[decode]: 100%|ββββββββββ| 250/250 [22:16<00:00, 5.35s/it, RTF=0.841]
2019-11-06 09:04:56,697 (decode:129) INFO: finished generation of 250 utterances (RTF = 0.734).
```
If you use MelGAN's generator, the decoding speed will be further faster.
```bash
# On CPU (Intel(R) Xeon(R) Gold 6154 CPU @ 3.00GHz 16 threads)
[decode]: 100%|ββββββββββ| 250/250 [04:00<00:00, 1.04it/s, RTF=0.0882]
2020-02-08 10:45:14,111 (decode:142) INFO: Finished generation of 250 utterances (RTF = 0.137).
# On GPU (TITAN V)
[decode]: 100%|ββββββββββ| 250/250 [00:06<00:00, 36.38it/s, RTF=0.00189]
2020-02-08 05:44:42,231 (decode:142) INFO: Finished generation of 250 utterances (RTF = 0.002).
```
If you use Multi-band MelGAN's generator, the decoding speed will be much further faster.
```bash
# On CPU (Intel(R) Xeon(R) Gold 6154 CPU @ 3.00GHz 16 threads)
[decode]: 100%|ββββββββββ| 250/250 [01:47<00:00, 2.95it/s, RTF=0.048]
2020-05-22 15:37:19,771 (decode:151) INFO: Finished generation of 250 utterances (RTF = 0.059).
# On GPU (TITAN V)
[decode]: 100%|ββββββββββ| 250/250 [00:05<00:00, 43.67it/s, RTF=0.000928]
2020-05-22 15:35:13,302 (decode:151) INFO: Finished generation of 250 utterances (RTF = 0.001).
```
If you want to accelerate the inference more, it is worthwhile to try the conversion from pytorch to tensorflow.
The example of the conversion is available in [the notebook](https://github.com/kan-bayashi/ParallelWaveGAN/blob/master/notebooks/convert_melgan_from_pytorch_to_tensorflow.ipynb) (Provided by [@dathudeptrai](https://github.com/dathudeptrai)).
## Results
Here the results are summarized in the table.
You can listen to the samples and download pretrained models from the link to our google drive.
| Model | Conf | Lang | Fs [Hz] | Mel range [Hz] | FFT / Hop / Win [pt] | # iters |
| :----------------------------------------------------------------------------------------------------------- | :-------------------------------------------------------------------------------------------------------------------------: | :---: | :-----: | :------------: | :------------------: | :-----: |
| [ljspeech_parallel_wavegan.v1](https://drive.google.com/open?id=1wdHr1a51TLeo4iKrGErVKHVFyq6D17TU) | [link](https://github.com/kan-bayashi/ParallelWaveGAN/blob/master/egs/ljspeech/voc1/conf/parallel_wavegan.v1.yaml) | EN | 22.05k | 80-7600 | 1024 / 256 / None | 400k |
| [ljspeech_parallel_wavegan.v1.long](https://drive.google.com/open?id=1XRn3s_wzPF2fdfGshLwuvNHrbgD0hqVS) | [link](https://github.com/kan-bayashi/ParallelWaveGAN/blob/master/egs/ljspeech/voc1/conf/parallel_wavegan.v1.long.yaml) | EN | 22.05k | 80-7600 | 1024 / 256 / None | 1M |
| [ljspeech_parallel_wavegan.v1.no_limit](https://drive.google.com/open?id=1NoD3TCmKIDHHtf74YsScX8s59aZFOFJA) | [link](https://github.com/kan-bayashi/ParallelWaveGAN/blob/master/egs/ljspeech/voc1/conf/parallel_wavegan.v1.no_limit.yaml) | EN | 22.05k | None | 1024 / 256 / None | 400k |
| [ljspeech_parallel_wavegan.v3](https://drive.google.com/open?id=1a5Q2KiJfUQkVFo5Bd1IoYPVicJGnm7EL) | [link](https://github.com/kan-bayashi/ParallelWaveGAN/blob/master/egs/ljspeech/voc1/conf/parallel_wavegan.v3.yaml) | EN | 22.05k | 80-7600 | 1024 / 256 / None | 3M |
| [ljspeech_melgan.v1](https://drive.google.com/open?id=1z0vO1UMFHyeCdCLAmd7Moewi4QgCb07S) | [link](https://github.com/kan-bayashi/ParallelWaveGAN/blob/master/egs/ljspeech/voc1/conf/melgan.v1.yaml) | EN | 22.05k | 80-7600 | 1024 / 256 / None | 400k |
| [ljspeech_melgan.v1.long](https://drive.google.com/open?id=1RqNGcFO7Geb6-4pJtMbC9-ph_WiWA14e) | [link](https://github.com/kan-bayashi/ParallelWaveGAN/blob/master/egs/ljspeech/voc1/conf/melgan.v1.long.yaml) | EN | 22.05k | 80-7600 | 1024 / 256 / None | 1M |
| [ljspeech_melgan_large.v1](https://drive.google.com/open?id=1KQt-gyxbG6iTZ4aVn9YjQuaGYjAleYs8) | [link](https://github.com/kan-bayashi/ParallelWaveGAN/blob/master/egs/ljspeech/voc1/conf/melgan_large.v1.yaml) | EN | 22.05k | 80-7600 | 1024 / 256 / None | 400k |
| [ljspeech_melgan_large.v1.long](https://drive.google.com/open?id=1ogEx-wiQS7HVtdU0_TmlENURIe4v2erC) | [link](https://github.com/kan-bayashi/ParallelWaveGAN/blob/master/egs/ljspeech/voc1/conf/melgan_large.v1.long.yaml) | EN | 22.05k | 80-7600 | 1024 / 256 / None | 1M |
| [ljspeech_melgan.v3](https://drive.google.com/open?id=1eXkm_Wf1YVlk5waP4Vgqd0GzMaJtW3y5) | [link](https://github.com/kan-bayashi/ParallelWaveGAN/blob/master/egs/ljspeech/voc1/conf/melgan.v3.yaml) | EN | 22.05k | 80-7600 | 1024 / 256 / None | 2M |
| [ljspeech_melgan.v3.long](https://drive.google.com/open?id=1u1w4RPefjByX8nfsL59OzU2KgEksBhL1) | [link](https://github.com/kan-bayashi/ParallelWaveGAN/blob/master/egs/ljspeech/voc1/conf/melgan.v3.long.yaml) | EN | 22.05k | 80-7600 | 1024 / 256 / None | 4M |
| [ljspeech_full_band_melgan.v1](https://drive.google.com/open?id=1RQqkbnoow0srTDYJNYA7RJ5cDRC5xB-t) | [link](https://github.com/kan-bayashi/ParallelWaveGAN/blob/master/egs/ljspeech/voc1/conf/full_band_melgan.v1.yaml) | EN | 22.05k | 80-7600 | 1024 / 256 / None | 1M |
| [ljspeech_full_band_melgan.v2](https://drive.google.com/open?id=1d9DWOzwOyxT1K5lPnyMqr2nED62vlHaX) | [link](https://github.com/kan-bayashi/ParallelWaveGAN/blob/master/egs/ljspeech/voc1/conf/full_band_melgan.v2.yaml) | EN | 22.05k | 80-7600 | 1024 / 256 / None | 1M |
| [ljspeech_multi_band_melgan.v1](https://drive.google.com/open?id=1ls_YxCccQD-v6ADbG6qXlZ8f30KrrhLT) | [link](https://github.com/kan-bayashi/ParallelWaveGAN/blob/master/egs/ljspeech/voc1/conf/multi_band_melgan.v1.yaml) | EN | 22.05k | 80-7600 | 1024 / 256 / None | 1M |
| [ljspeech_multi_band_melgan.v2](https://drive.google.com/open?id=1wevYP2HQ7ec2fSixTpZIX0sNBtYZJz_I) | [link](https://github.com/kan-bayashi/ParallelWaveGAN/blob/master/egs/ljspeech/voc1/conf/multi_band_melgan.v2.yaml) | EN | 22.05k | 80-7600 | 1024 / 256 / None | 1M |
| [ljspeech_hifigan.v1](https://drive.google.com/open?id=18_R5-pGHDIbIR1QvrtBZwVRHHpBy5xiZ) | [link](https://github.com/kan-bayashi/ParallelWaveGAN/blob/master/egs/ljspeech/voc1/conf/hifigan.v1.yaml) | EN | 22.05k | 80-7600 | 1024 / 256 / None | 2.5M |
| [ljspeech_style_melgan.v1](https://drive.google.com/open?id=1WFlVknhyeZhTT5R6HznVJCJ4fwXKtb3B) | [link](https://github.com/kan-bayashi/ParallelWaveGAN/blob/master/egs/ljspeech/voc1/conf/style_melgan.v1.yaml) | EN | 22.05k | 80-7600 | 1024 / 256 / None | 1.5M |
| [jsut_parallel_wavegan.v1](https://drive.google.com/open?id=1UDRL0JAovZ8XZhoH0wi9jj_zeCKb-AIA) | [link](https://github.com/kan-bayashi/ParallelWaveGAN/blob/master/egs/jsut/voc1/conf/parallel_wavegan.v1.yaml) | JP | 24k | 80-7600 | 2048 / 300 / 1200 | 400k |
| [jsut_multi_band_melgan.v2](https://drive.google.com/open?id=1E4fe0c5gMLtmSS0Hrzj-9nUbMwzke4PS) | [link](https://github.com/kan-bayashi/ParallelWaveGAN/blob/master/egs/jsut/voc1/conf/multi_band_melgan.v2.yaml) | JP | 24k | 80-7600 | 2048 / 300 / 1200 | 1M |
| [just_hifigan.v1](https://drive.google.com/open?id=1TY88141UWzQTAQXIPa8_g40QshuqVj6Y) | [link](https://github.com/kan-bayashi/ParallelWaveGAN/blob/master/egs/jsut/voc1/conf/hifigan.v1.yaml) | JP | 24k | 80-7600 | 2048 / 300 / 1200 | 2.5M |
| [just_style_melgan.v1](https://drive.google.com/open?id=1-qKAC0zLya6iKMngDERbSzBYD4JHmGdh) | [link](https://github.com/kan-bayashi/ParallelWaveGAN/blob/master/egs/jsut/voc1/conf/style_melgan.v1.yaml) | JP | 24k | 80-7600 | 2048 / 300 / 1200 | 1.5M |
| [csmsc_parallel_wavegan.v1](https://drive.google.com/open?id=1C2nu9nOFdKcEd-D9xGquQ0bCia0B2v_4) | [link](https://github.com/kan-bayashi/ParallelWaveGAN/blob/master/egs/csmsc/voc1/conf/parallel_wavegan.v1.yaml) | ZH | 24k | 80-7600 | 2048 / 300 / 1200 | 400k |
| [csmsc_multi_band_melgan.v2](https://drive.google.com/open?id=1F7FwxGbvSo1Rnb5kp0dhGwimRJstzCrz) | [link](https://github.com/kan-bayashi/ParallelWaveGAN/blob/master/egs/csmsc/voc1/conf/multi_band_melgan.v2.yaml) | ZH | 24k | 80-7600 | 2048 / 300 / 1200 | 1M |
| [csmsc_hifigan.v1](https://drive.google.com/open?id=1gTkVloMqteBfSRhTrZGdOBBBRsGd3qt8) | [link](https://github.com/kan-bayashi/ParallelWaveGAN/blob/master/egs/csmsc/voc1/conf/hifigan.v1.yaml) | ZH | 24k | 80-7600 | 2048 / 300 / 1200 | 2.5M |
| [csmsc_style_melgan.v1](https://drive.google.com/open?id=1gl4P5W_ST_nnv0vjurs7naVm5UJqkZIn) | [link](https://github.com/kan-bayashi/ParallelWaveGAN/blob/master/egs/csmsc/voc1/conf/style_melgan.v1.yaml) | ZH | 24k | 80-7600 | 2048 / 300 / 1200 | 1.5M |
| [arctic_slt_parallel_wavegan.v1](https://drive.google.com/open?id=1xG9CmSED2TzFdklD6fVxzf7kFV2kPQAJ) | [link](https://github.com/kan-bayashi/ParallelWaveGAN/blob/master/egs/arctic/voc1/conf/parallel_wavegan.v1.yaml) | EN | 16k | 80-7600 | 1024 / 256 / None | 400k |
| [jnas_parallel_wavegan.v1](https://drive.google.com/open?id=1n_hkxPxryVXbp6oHM1NFm08q0TcoDXz1) | [link](https://github.com/kan-bayashi/ParallelWaveGAN/blob/master/egs/jnas/voc1/conf/parallel_wavegan.v1.yaml) | JP | 16k | 80-7600 | 1024 / 256 / None | 400k |
| [vctk_parallel_wavegan.v1](https://drive.google.com/open?id=1dGTu-B7an2P5sEOepLPjpOaasgaSnLpi) | [link](https://github.com/kan-bayashi/ParallelWaveGAN/blob/master/egs/vctk/voc1/conf/parallel_wavegan.v1.yaml) | EN | 24k | 80-7600 | 2048 / 300 / 1200 | 400k |
| [vctk_parallel_wavegan.v1.long](https://drive.google.com/open?id=1qoocM-VQZpjbv5B-zVJpdraazGcPL0So) | [link](https://github.com/kan-bayashi/ParallelWaveGAN/blob/master/egs/vctk/voc1/conf/parallel_wavegan.v1.long.yaml) | EN | 24k | 80-7600 | 2048 / 300 / 1200 | 1M |
| [vctk_multi_band_melgan.v2](https://drive.google.com/open?id=17EkB4hSKUEDTYEne-dNHtJT724hdivn4) | [link](https://github.com/kan-bayashi/ParallelWaveGAN/blob/master/egs/vctk/voc1/conf/multi_band_melgan.v2.yaml) | EN | 24k | 80-7600 | 2048 / 300 / 1200 | 1M |
| [vctk_hifigan.v1](https://drive.google.com/open?id=17fu7ukS97m-8StXPc6ltW8a3hr0fsQBP) | [link](https://github.com/kan-bayashi/ParallelWaveGAN/blob/master/egs/vctk/voc1/conf/hifigan.v1.yaml) | EN | 24k | 80-7600 | 2048 / 300 / 1200 | 2.5M |
| [vctk_style_melgan.v1](https://drive.google.com/open?id=1kfJgzDgrOFYxTfVTNbTHcnyq--cc6plo) | [link](https://github.com/kan-bayashi/ParallelWaveGAN/blob/master/egs/vctk/voc1/conf/style_melgan.v1.yaml) | EN | 24k | 80-7600 | 2048 / 300 / 1200 | 1.5M |
| [libritts_parallel_wavegan.v1](https://drive.google.com/open?id=1pb18Nd2FCYWnXfStszBAEEIMe_EZUJV0) | [link](https://github.com/kan-bayashi/ParallelWaveGAN/blob/master/egs/libritts/voc1/conf/parallel_wavegan.v1.yaml) | EN | 24k | 80-7600 | 2048 / 300 / 1200 | 400k |
| [libritts_parallel_wavegan.v1.long](https://drive.google.com/open?id=15ibzv-uTeprVpwT946Hl1XUYDmg5Afwz) | [link](https://github.com/kan-bayashi/ParallelWaveGAN/blob/master/egs/libritts/voc1/conf/parallel_wavegan.v1.long.yaml) | EN | 24k | 80-7600 | 2048 / 300 / 1200 | 1M |
| [libritts_multi_band_melgan.v2](https://drive.google.com/open?id=1jfB15igea6tOQ0hZJGIvnpf3QyNhTLnq) | [link](https://github.com/kan-bayashi/ParallelWaveGAN/blob/master/egs/libritts/voc1/conf/multi_band_melgan.v2.yaml) | EN | 24k | 80-7600 | 2048 / 300 / 1200 | 1M |
| [libritts_hifigan.v1](https://drive.google.com/open?id=10jBLsjQT3LvR-3GgPZpRvWIWvpGjzDnM) | [link](https://github.com/kan-bayashi/ParallelWaveGAN/blob/master/egs/libritts/voc1/conf/hifigan.v1.yaml) | EN | 24k | 80-7600 | 2048 / 300 / 1200 | 2.5M |
| [libritts_style_melgan.v1](https://drive.google.com/open?id=1OPpYbrqYOJ_hHNGSQHzUxz_QZWWBwV9r) | [link](https://github.com/kan-bayashi/ParallelWaveGAN/blob/master/egs/libritts/voc1/conf/style_melgan.v1.yaml) | EN | 24k | 80-7600 | 2048 / 300 / 1200 | 1.5M |
| [kss_parallel_wavegan.v1](https://drive.google.com/open?id=1n5kitXZqPHUr-veoUKCyfJvb3p1g0VlY) | [link](https://github.com/kan-bayashi/ParallelWaveGAN/blob/master/egs/libritts/voc1/conf/parallel_wavegan.v1.yaml) | KO | 24k | 80-7600 | 2048 / 300 / 1200 | 400k |
| [hui_acg_hokuspokus_parallel_wavegan.v1](https://drive.google.com/open?id=1rwzpIwb65xbW5fFPsqPWdforsk4U-vDg) | [link](https://github.com/kan-bayashi/ParallelWaveGAN/blob/master/egs/libritts/voc1/conf/parallel_wavegan.v1.yaml) | DE | 24k | 80-7600 | 2048 / 300 / 1200 | 400k |
| [ruslan_parallel_wavegan.v1](https://drive.google.com/open?id=1QGuesaRKGful0bUTTaFZdbjqHNhy2LpE) | [link](https://github.com/kan-bayashi/ParallelWaveGAN/blob/master/egs/libritts/voc1/conf/parallel_wavegan.v1.yaml) | RU | 24k | 80-7600 | 2048 / 300 / 1200 | 400k |
Please access at [our google drive](https://drive.google.com/open?id=1sd_QzcUNnbiaWq7L0ykMP7Xmk-zOuxTi) to check more results.
## How-to-use pretrained models
### Analysis-synthesis
Here the minimal code is shown to perform analysis-synthesis using the pretrained model.
```bash
# Please make sure you installed `parallel_wavegan`
# If not, please install via pip
$ pip install parallel_wavegan
# You can download the pretrained model from terminal
$ python << EOF
from parallel_wavegan.utils import download_pretrained_model
download_pretrained_model("<pretrained_model_tag>", "pretrained_model")
EOF
# You can get all of available pretrained models as follows:
$ python << EOF
from parallel_wavegan.utils import PRETRAINED_MODEL_LIST
print(PRETRAINED_MODEL_LIST.keys())
EOF
# Now you can find downloaded pretrained model in `pretrained_model/<pretrain_model_tag>/`
$ ls pretrain_model/<pretrain_model_tag>
ο checkpoint-400000steps.pkl ο config.yml ο stats.h5
# These files can also be downloaded manually from the above results
# Please put an audio file in `sample` directory to perform analysis-synthesis
$ ls sample/
ο sample.wav
# Then perform feature extraction -> feature normalization -> synthesis
$ parallel-wavegan-preprocess \
--config pretrain_model/<pretrain_model_tag>/config.yml \
--rootdir sample \
--dumpdir dump/sample/raw
100%|ββββββββββββββββββββββββββββββββββββββββ| 1/1 [00:00<00:00, 914.19it/s]
$ parallel-wavegan-normalize \
--config pretrain_model/<pretrain_model_tag>/config.yml \
--rootdir dump/sample/raw \
--dumpdir dump/sample/norm \
--stats pretrain_model/<pretrain_model_tag>/stats.h5
2019-11-13 13:44:29,574 (normalize:87) INFO: the number of files = 1.
100%|ββββββββββββββββββββββββββββββββββββββββ| 1/1 [00:00<00:00, 513.13it/s]
$ parallel-wavegan-decode \
--checkpoint pretrain_model/<pretrain_model_tag>/checkpoint-400000steps.pkl \
--dumpdir dump/sample/norm \
--outdir sample
2019-11-13 13:44:31,229 (decode:91) INFO: the number of features to be decoded = 1.
[decode]: 100%|βββββββββββββββββββ| 1/1 [00:00<00:00, 18.33it/s, RTF=0.0146]
2019-11-13 13:44:37,132 (decode:129) INFO: finished generation of 1 utterances (RTF = 0.015).
# You can skip normalization step (on-the-fly normalization, feature extraction -> synthesis)
$ parallel-wavegan-preprocess \
--config pretrain_model/<pretrain_model_tag>/config.yml \
--rootdir sample \
--dumpdir dump/sample/raw
100%|ββββββββββββββββββββββββββββββββββββββββ| 1/1 [00:00<00:00, 914.19it/s]
$ parallel-wavegan-decode \
--checkpoint pretrain_model/<pretrain_model_tag>/checkpoint-400000steps.pkl \
--dumpdir dump/sample/raw \
--normalize-before \
--outdir sample
2019-11-13 13:44:31,229 (decode:91) INFO: the number of features to be decoded = 1.
[decode]: 100%|βββββββββββββββββββ| 1/1 [00:00<00:00, 18.33it/s, RTF=0.0146]
2019-11-13 13:44:37,132 (decode:129) INFO: finished generation of 1 utterances (RTF = 0.015).
# you can find the generated speech in `sample` directory
$ ls sample
ο sample.wav ο sample_gen.wav
```
### Decoding with ESPnet-TTS model's features
Here, I show the procedure to generate waveforms with features generated by [ESPnet-TTS](https://github.com/espnet/espnet) models.
```bash
# Make sure you already finished running the recipe of ESPnet-TTS.
# You must use the same feature settings for both Text2Mel and Mel2Wav models.
# Let us move on "ESPnet" recipe directory
$ cd /path/to/espnet/egs/<recipe_name>/tts1
$ pwd
/path/to/espnet/egs/<recipe_name>/tts1
# If you use ESPnet2, move on `egs2/`
$ cd /path/to/espnet/egs2/<recipe_name>/tts1
$ pwd
/path/to/espnet/egs2/<recipe_name>/tts1
# Please install this repository in ESPnet conda (or virtualenv) environment
$ . ./path.sh && pip install -U parallel_wavegan
# You can download the pretrained model from terminal
$ python << EOF
from parallel_wavegan.utils import download_pretrained_model
download_pretrained_model("<pretrained_model_tag>", "pretrained_model")
EOF
# You can get all of available pretrained models as follows:
$ python << EOF
from parallel_wavegan.utils import PRETRAINED_MODEL_LIST
print(PRETRAINED_MODEL_LIST.keys())
EOF
# You can find downloaded pretrained model in `pretrained_model/<pretrain_model_tag>/`
$ ls pretrain_model/<pretrain_model_tag>
ο checkpoint-400000steps.pkl ο config.yml ο stats.h5
# These files can also be downloaded manually from the above results
```
**Case 1**: If you use the same dataset for both Text2Mel and Mel2Wav
```bash
# In this case, you can directly use generated features for decoding.
# Please specify `feats.scp` path for `--feats-scp`, which is located in
# exp/<your_model_dir>/outputs_*_decode/<set_name>/feats.scp.
# Note that do not use outputs_*decode_denorm/<set_name>/feats.scp since
# it is de-normalized features (the input for PWG is normalized features).
$ parallel-wavegan-decode \
--checkpoint pretrain_model/<pretrain_model_tag>/checkpoint-400000steps.pkl \
--feats-scp exp/<your_model_dir>/outputs_*_decode/<set_name>/feats.scp \
--outdir <path_to_outdir>
# In the case of ESPnet2, the generated feature can be found in
# exp/<your_model_dir>/decode_*/<set_name>/norm/feats.scp.
$ parallel-wavegan-decode \
--checkpoint pretrain_model/<pretrain_model_tag>/checkpoint-400000steps.pkl \
--feats-scp exp/<your_model_dir>/decode_*/<set_name>/norm/feats.scp \
--outdir <path_to_outdir>
# You can find the generated waveforms in <path_to_outdir>/.
$ ls <path_to_outdir>
ο utt_id_1_gen.wav ο utt_id_2_gen.wav ... ο utt_id_N_gen.wav
```
**Case 2**: If you use different datasets for Text2Mel and Mel2Wav models
```bash
# In this case, you must provide `--normalize-before` option additionally.
# And use `feats.scp` of de-normalized generated features.
# ESPnet1 case
$ parallel-wavegan-decode \
--checkpoint pretrain_model/<pretrain_model_tag>/checkpoint-400000steps.pkl \
--feats-scp exp/<your_model_dir>/outputs_*_decode_denorm/<set_name>/feats.scp \
--outdir <path_to_outdir> \
--normalize-before
# ESPnet2 case
$ parallel-wavegan-decode \
--checkpoint pretrain_model/<pretrain_model_tag>/checkpoint-400000steps.pkl \
--feats-scp exp/<your_model_dir>/decode_*/<set_name>/denorm/feats.scp \
--outdir <path_to_outdir> \
--normalize-before
# You can find the generated waveforms in <path_to_outdir>/.
$ ls <path_to_outdir>
ο utt_id_1_gen.wav ο utt_id_2_gen.wav ... ο utt_id_N_gen.wav
```
If you want to combine these models in python, you can try the real-time demonstration in Google Colab!
- Real-time demonstration with ESPnet2 [](https://colab.research.google.com/github/espnet/notebook/blob/master/espnet2_tts_realtime_demo.ipynb)
- Real-time demonstration with ESPnet1 [](https://colab.research.google.com/github/espnet/notebook/blob/master/tts_realtime_demo.ipynb)
### Decoding with dumped npy files
Sometimes we want to decode with dumped npy files, which are mel-spectrogram generated by TTS models.
Please make sure you used the same feature extraction settings of the pretrained vocoder (`fs`, `fft_size`, `hop_size`, `win_length`, `fmin`, and `fmax`).
Only the difference of `log_base` can be changed with some post-processings (we use log 10 instead of natural log as a default).
See detail in [the comment](https://github.com/kan-bayashi/ParallelWaveGAN/issues/169#issuecomment-649320778).
```bash
# Generate dummy npy file of mel-spectrogram
$ ipython
[ins] In [1]: import numpy as np
[ins] In [2]: x = np.random.randn(512, 80) # (#frames, #mels)
[ins] In [3]: np.save("dummy_1.npy", x)
[ins] In [4]: y = np.random.randn(256, 80) # (#frames, #mels)
[ins] In [5]: np.save("dummy_2.npy", y)
[ins] In [6]: exit
# Make scp file (key-path format)
$ find -name "*.npy" | awk '{print "dummy_" NR " " $1}' > feats.scp
# Check (<utt_id> <path>)
$ cat feats.scp
dummy_1 ./dummy_1.npy
dummy_2 ./dummy_2.npy
# Decode without feature normalization
# This case assumes that the input mel-spectrogram is normalized with the same statistics of the pretrained model.
$ parallel-wavegan-decode \
--checkpoint /path/to/checkpoint-400000steps.pkl \
--feats-scp ./feats.scp \
--outdir wav
2021-08-10 09:13:07,624 (decode:140) INFO: The number of features to be decoded = 2.
[decode]: 100%|ββββββββββββββββββββββββββββββββββββββββ| 2/2 [00:00<00:00, 13.84it/s, RTF=0.00264]
2021-08-10 09:13:29,660 (decode:174) INFO: Finished generation of 2 utterances (RTF = 0.005).
# Decode with feature normalization
# This case assumes that the input mel-spectrogram is not normalized.
$ parallel-wavegan-decode \
--checkpoint /path/to/checkpoint-400000steps.pkl \
--feats-scp ./feats.scp \
--normalize-before \
--outdir wav
2021-08-10 09:13:07,624 (decode:140) INFO: The number of features to be decoded = 2.
[decode]: 100%|ββββββββββββββββββββββββββββββββββββββββ| 2/2 [00:00<00:00, 13.84it/s, RTF=0.00264]
2021-08-10 09:13:29,660 (decode:174) INFO: Finished generation of 2 utterances (RTF = 0.005).
```
## References
- [Parallel WaveGAN](https://arxiv.org/abs/1910.11480)
- [r9y9/wavenet_vocoder](https://github.com/r9y9/wavenet_vocoder)
- [LiyuanLucasLiu/RAdam](https://github.com/LiyuanLucasLiu/RAdam)
- [MelGAN](https://arxiv.org/abs/1910.06711)
- [descriptinc/melgan-neurips](https://github.com/descriptinc/melgan-neurips)
- [Multi-band MelGAN](https://arxiv.org/abs/2005.05106)
- [HiFi-GAN](https://arxiv.org/abs/2010.05646)
- [jik876/hifi-gan](https://github.com/jik876/hifi-gan)
- [StyleMelGAN](https://arxiv.org/abs/2011.01557)
## Acknowledgement
The author would like to thank Ryuichi Yamamoto ([@r9y9](https://github.com/r9y9)) for his great repository, paper, and valuable discussions.
## Author
Tomoki Hayashi ([@kan-bayashi](https://github.com/kan-bayashi))
E-mail: `hayashi.tomoki<at>g.sp.m.is.nagoya-u.ac.jp`
|