Deep RL Course documentation
Introduction
Introduction
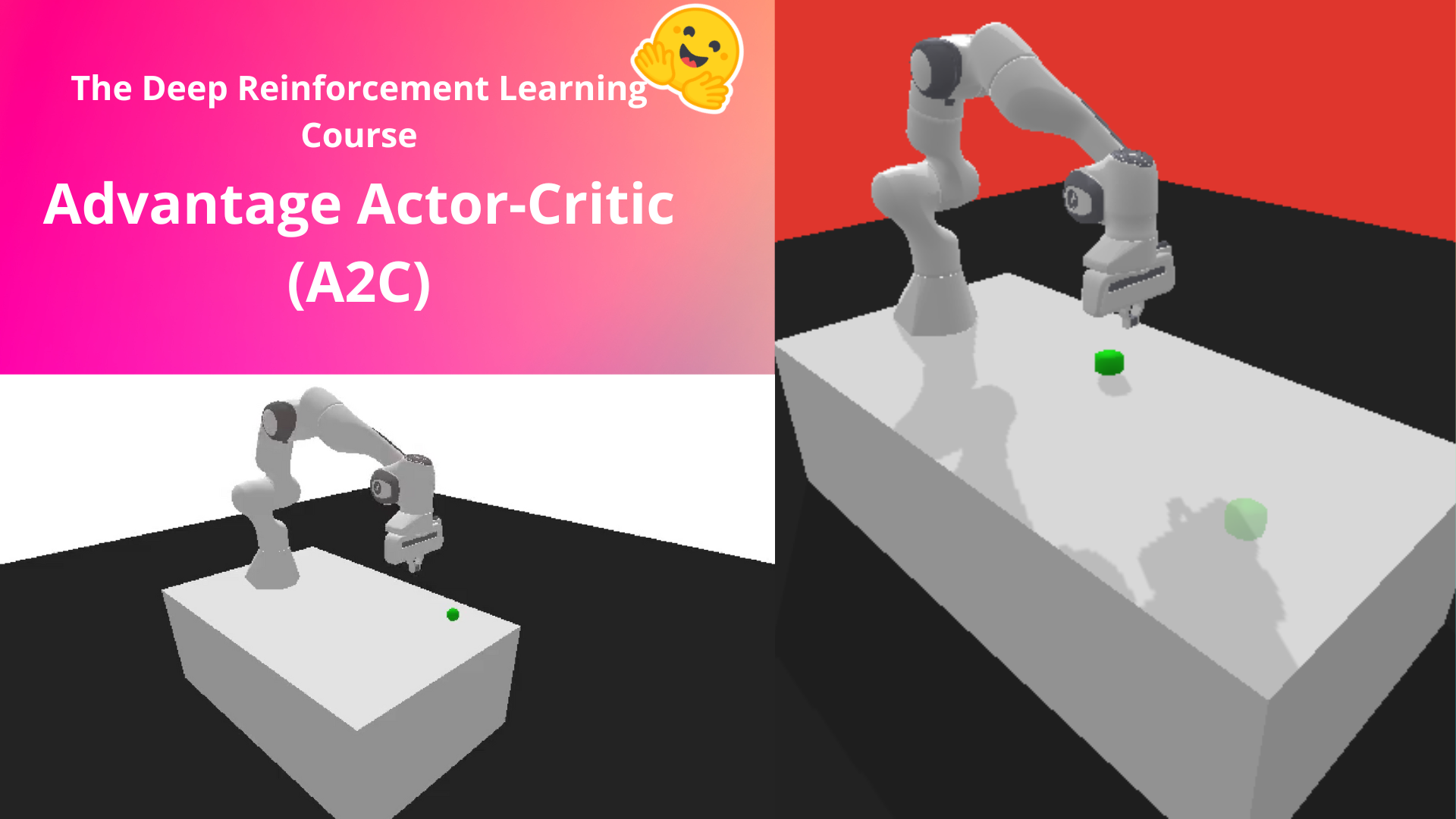
In unit 4, we learned about our first Policy-Based algorithm called Reinforce.
In Policy-Based methods, we aim to optimize the policy directly without using a value function. More precisely, Reinforce is part of a subclass of Policy-Based Methods called Policy-Gradient methods. This subclass optimizes the policy directly by estimating the weights of the optimal policy using Gradient Ascent.
We saw that Reinforce worked well. However, because we use Monte-Carlo sampling to estimate return (we use an entire episode to calculate the return), we have significant variance in policy gradient estimation.
Remember that the policy gradient estimation is the direction of the steepest increase in return. In other words, how to update our policy weights so that actions that lead to good returns have a higher probability of being taken. The Monte Carlo variance, which we will further study in this unit, leads to slower training since we need a lot of samples to mitigate it.
So today we’ll study Actor-Critic methods, a hybrid architecture combining value-based and Policy-Based methods that helps to stabilize the training by reducing the variance using:
- An Actor that controls how our agent behaves (Policy-Based method)
- A Critic that measures how good the taken action is (Value-Based method)
We’ll study one of these hybrid methods, Advantage Actor Critic (A2C), and train our agent using Stable-Baselines3 in robotic environments. We’ll train:
- A robotic arm 🦾 to move to the correct position.
Sound exciting? Let’s get started!
< > Update on GitHub