The advantages and disadvantages of policy-gradient methods
At this point, you might ask, “but Deep Q-Learning is excellent! Why use policy-gradient methods?“. To answer this question, let’s study the advantages and disadvantages of policy-gradient methods.
Advantages
There are multiple advantages over value-based methods. Let’s see some of them:
The simplicity of integration
We can estimate the policy directly without storing additional data (action values).
Policy-gradient methods can learn a stochastic policy
Policy-gradient methods can learn a stochastic policy while value functions can’t.
This has two consequences:
We don’t need to implement an exploration/exploitation trade-off by hand. Since we output a probability distribution over actions, the agent explores the state space without always taking the same trajectory.
We also get rid of the problem of perceptual aliasing. Perceptual aliasing is when two states seem (or are) the same but need different actions.
Let’s take an example: we have an intelligent vacuum cleaner whose goal is to suck the dust and avoid killing the hamsters.
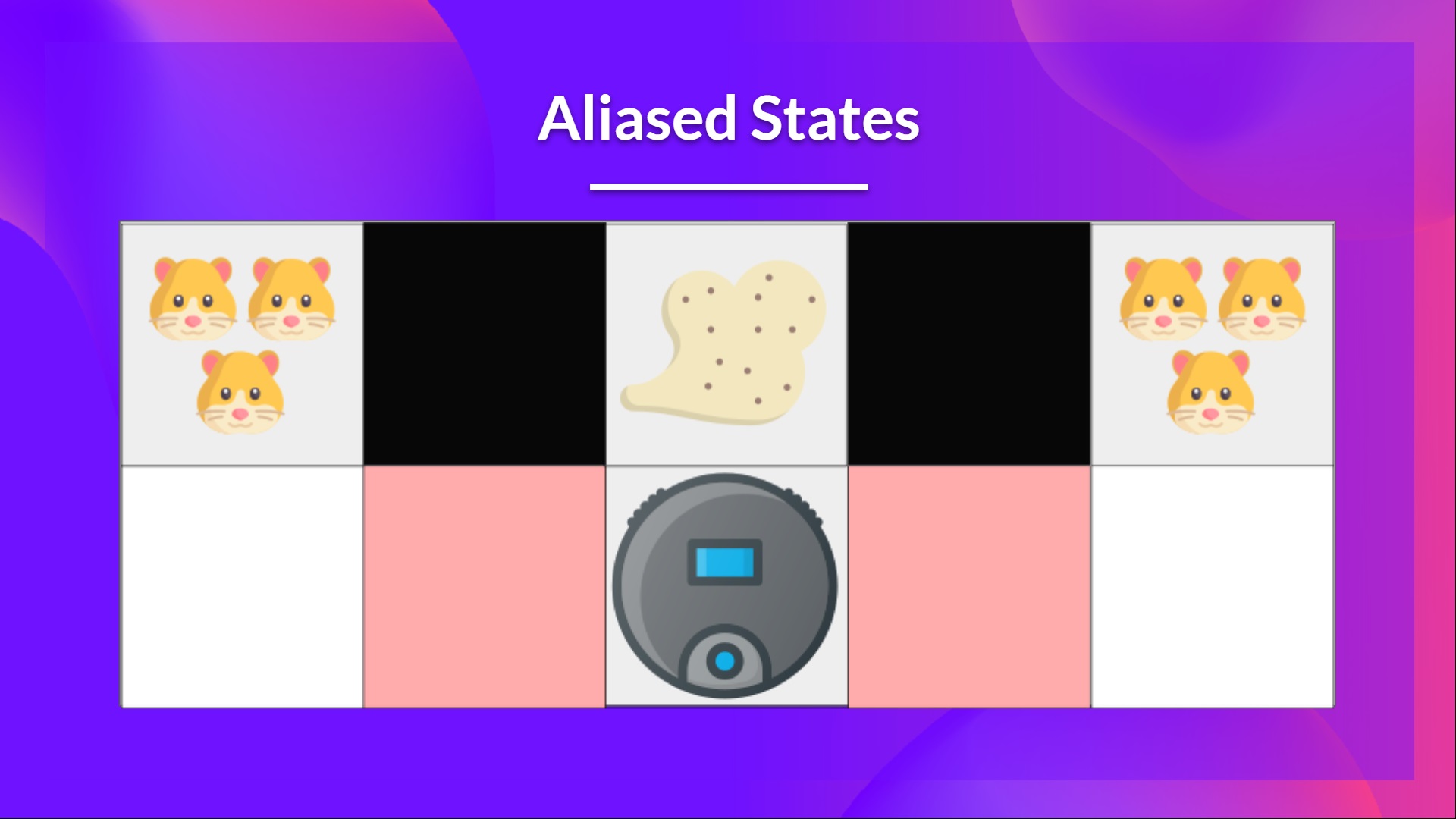
Our vacuum cleaner can only perceive where the walls are.
The problem is that the two red (colored) states are aliased states because the agent perceives an upper and lower wall for each.
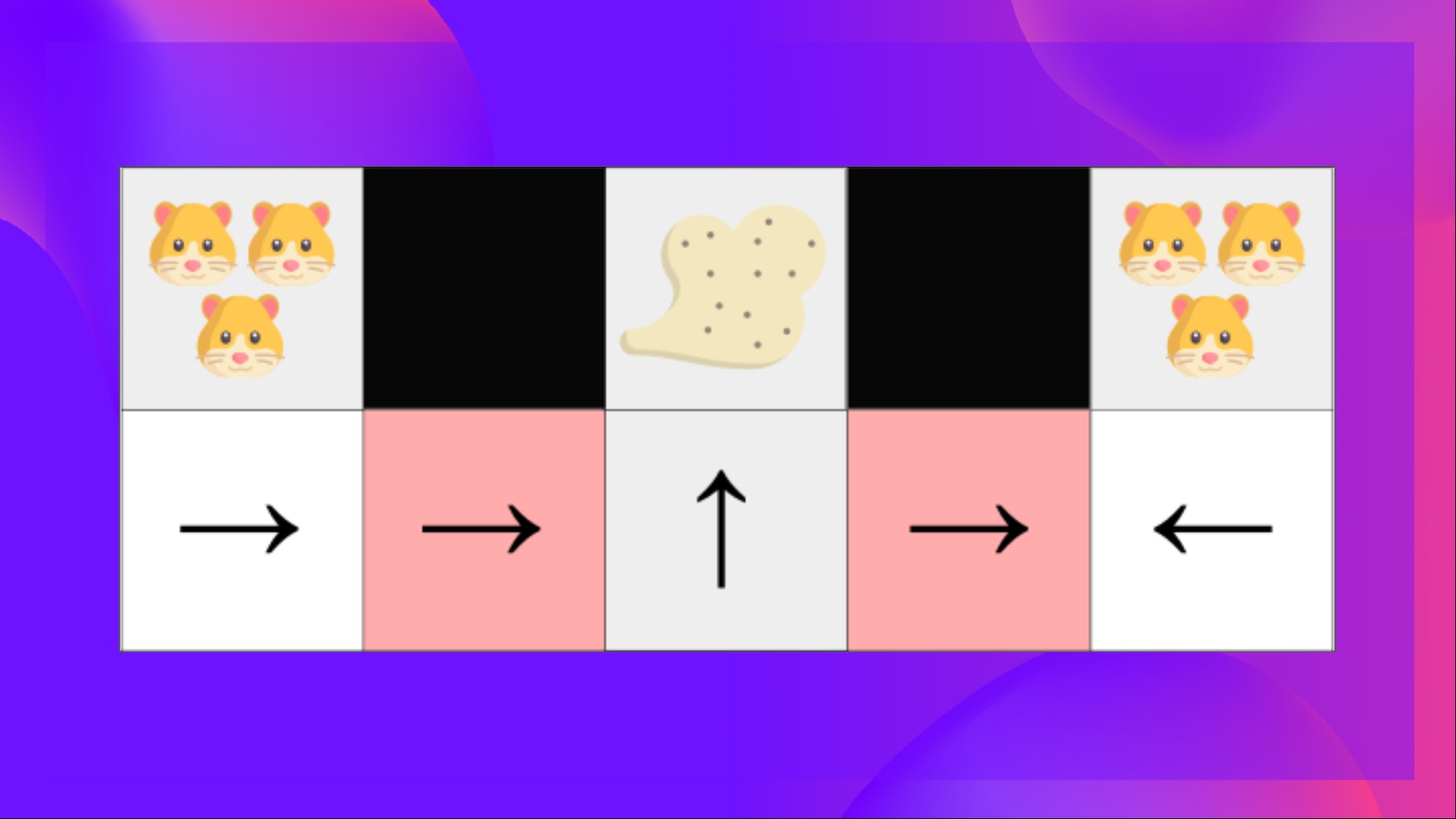
Under a deterministic policy, the policy will either always move right when in a red state or always move left. Either case will cause our agent to get stuck and never suck the dust.
Under a value-based Reinforcement learning algorithm, we learn a quasi-deterministic policy (“greedy epsilon strategy”). Consequently, our agent can spend a lot of time before finding the dust.
On the other hand, an optimal stochastic policy will randomly move left or right in red (colored) states. Consequently, it will not be stuck and will reach the goal state with a high probability.
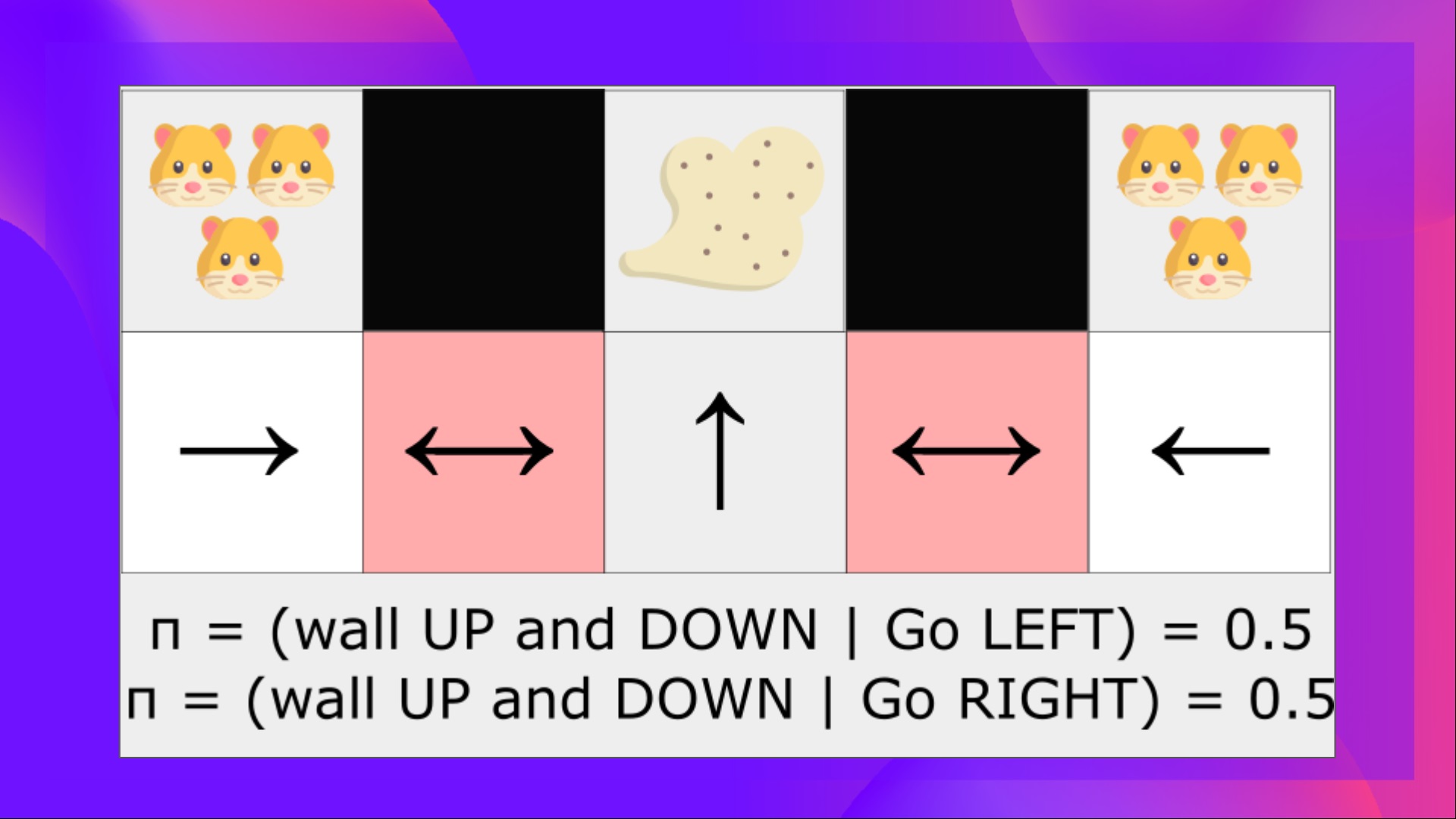
Policy-gradient methods are more effective in high-dimensional action spaces and continuous actions spaces
The problem with Deep Q-learning is that their predictions assign a score (maximum expected future reward) for each possible action, at each time step, given the current state.
But what if we have an infinite possibility of actions?
For instance, with a self-driving car, at each state, you can have a (near) infinite choice of actions (turning the wheel at 15°, 17.2°, 19,4°, honking, etc.). We’ll need to output a Q-value for each possible action! And taking the max action of a continuous output is an optimization problem itself!
Instead, with policy-gradient methods, we output a probability distribution over actions.
Policy-gradient methods have better convergence properties
In value-based methods, we use an aggressive operator to change the value function: we take the maximum over Q-estimates. Consequently, the action probabilities may change dramatically for an arbitrarily small change in the estimated action values if that change results in a different action having the maximal value.
For instance, if during the training, the best action was left (with a Q-value of 0.22) and the training step after it’s right (since the right Q-value becomes 0.23), we dramatically changed the policy since now the policy will take most of the time right instead of left.
On the other hand, in policy-gradient methods, stochastic policy action preferences (probability of taking action) change smoothly over time.
Disadvantages
Naturally, policy-gradient methods also have some disadvantages:
- Frequently, policy-gradient methods converges to a local maximum instead of a global optimum.
- Policy-gradient goes slower, step by step: it can take longer to train (inefficient).
- Policy-gradient can have high variance. We’ll see in the actor-critic unit why, and how we can solve this problem.
👉 If you want to go deeper into the advantages and disadvantages of policy-gradient methods, you can check this video.
< > Update on GitHub