Tried sadtalker , too much time consumption. D-ID is proprietary . Looking something from opensource. Tried wav2lip and also enhancing that with GFPGAN , output is good but i want something fast.
Akhil B
hakunamatata1997
AI & ML interests
Gen AI , NLP , Computer Vision , XAI
Organizations
hakunamatata1997's activity
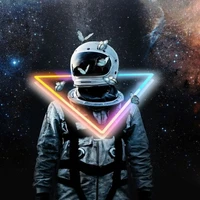
replied to
their
post
5 months ago
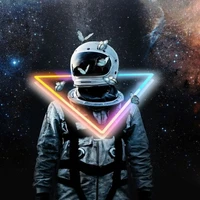
replied to
their
post
6 months ago
Yeah tried QwenVL , it's poor on understanding text, QwenVL-Plus and Max are good but not open sourced ๐ช
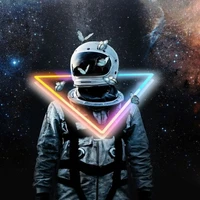
replied to
their
post
6 months ago
@merve more particularly if i say, something like understanding text good enough in images so the response are accurate enough from VLM
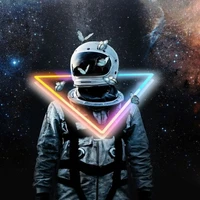
replied to
their
post
6 months ago
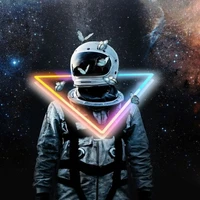
reacted to
akhaliq's
post with ๐ฅ
7 months ago
Post
4376
Leave No Context Behind
Efficient Infinite Context Transformers with Infini-attention
Leave No Context Behind: Efficient Infinite Context Transformers with Infini-attention (2404.07143)
This work introduces an efficient method to scale Transformer-based Large Language Models (LLMs) to infinitely long inputs with bounded memory and computation. A key component in our proposed approach is a new attention technique dubbed Infini-attention. The Infini-attention incorporates a compressive memory into the vanilla attention mechanism and builds in both masked local attention and long-term linear attention mechanisms in a single Transformer block. We demonstrate the effectiveness of our approach on long-context language modeling benchmarks, 1M sequence length passkey context block retrieval and 500K length book summarization tasks with 1B and 8B LLMs. Our approach introduces minimal bounded memory parameters and enables fast streaming inference for LLMs.
Efficient Infinite Context Transformers with Infini-attention
Leave No Context Behind: Efficient Infinite Context Transformers with Infini-attention (2404.07143)
This work introduces an efficient method to scale Transformer-based Large Language Models (LLMs) to infinitely long inputs with bounded memory and computation. A key component in our proposed approach is a new attention technique dubbed Infini-attention. The Infini-attention incorporates a compressive memory into the vanilla attention mechanism and builds in both masked local attention and long-term linear attention mechanisms in a single Transformer block. We demonstrate the effectiveness of our approach on long-context language modeling benchmarks, 1M sequence length passkey context block retrieval and 500K length book summarization tasks with 1B and 8B LLMs. Our approach introduces minimal bounded memory parameters and enables fast streaming inference for LLMs.
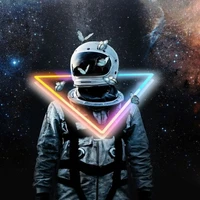
reacted to
lewtun's
post with โค๏ธ
7 months ago
Post
4672
Introducing Zephyr 141B-A35B ๐ช:
HuggingFaceH4/zephyr-orpo-141b-A35b-v0.1
Yesterday, Mistral released their latest base model (via magnet link of course ๐ ) and the community quickly converted it to transformers format and pushed it to the Hub: mistral-community/Mixtral-8x22B-v0.1
Early evals of this model looked extremely strong, so we teamed up with Argilla and KAIST AI to cook up a Zephyr recipe with a few new alignment techniques that came out recently:
๐งโ๐ณ Align the base model with Odds Ratio Preference Optimisation (ORPO). This novel algorithm developed by @JW17 and @nlee-208 and @j6mes and does not require an SFT step to achieve high performance and is thus much more computationally efficient than methods like DPO and PPO.
๐ฆซ Use a brand new dataset of 7k high-quality, multi-turn preferences that has been developed by our friends at Argilla. To create this dataset, they took the excellent Capybara SFT dataset from @LDJnr LDJnr/Capybara and converted it into a preference dataset by augmenting the final turn with responses from new LLMs that were then ranked by GPT-4.
What we find especially neat about this approach is that training on 7k samples only takes ~1.3h on 4 H100 nodes, yet produces a model that is very strong on chat benchmarks like IFEval and BBH.
Kudos to @alvarobartt @JW17 and @nlee-208 for this very nice and fast-paced collab!
For more details on the paper and dataset, checkout our collection: HuggingFaceH4/zephyr-orpo-6617eba2c5c0e2cc3c151524
HuggingFaceH4/zephyr-orpo-141b-A35b-v0.1
Yesterday, Mistral released their latest base model (via magnet link of course ๐ ) and the community quickly converted it to transformers format and pushed it to the Hub: mistral-community/Mixtral-8x22B-v0.1
Early evals of this model looked extremely strong, so we teamed up with Argilla and KAIST AI to cook up a Zephyr recipe with a few new alignment techniques that came out recently:
๐งโ๐ณ Align the base model with Odds Ratio Preference Optimisation (ORPO). This novel algorithm developed by @JW17 and @nlee-208 and @j6mes and does not require an SFT step to achieve high performance and is thus much more computationally efficient than methods like DPO and PPO.
๐ฆซ Use a brand new dataset of 7k high-quality, multi-turn preferences that has been developed by our friends at Argilla. To create this dataset, they took the excellent Capybara SFT dataset from @LDJnr LDJnr/Capybara and converted it into a preference dataset by augmenting the final turn with responses from new LLMs that were then ranked by GPT-4.
What we find especially neat about this approach is that training on 7k samples only takes ~1.3h on 4 H100 nodes, yet produces a model that is very strong on chat benchmarks like IFEval and BBH.
Kudos to @alvarobartt @JW17 and @nlee-208 for this very nice and fast-paced collab!
For more details on the paper and dataset, checkout our collection: HuggingFaceH4/zephyr-orpo-6617eba2c5c0e2cc3c151524
Did anyone research on frameworks or tools that are currently being used to make agents for production. I've been doing some research but most of them not suitable for production.