File size: 21,827 Bytes
18a1462 f024d1d a3a81b7 f024d1d 99e010d d9a0d0e d5cf678 d9a0d0e c470219 d9a0d0e d5cf678 98335ad d9a0d0e 8909940 d9a0d0e 124f469 99e010d d08596f 99e010d 124f469 f024d1d d9a0d0e 99e010d d08596f 7a2ccff 99e010d 5e0c76e 99e010d a4d3600 99e010d a4d3600 99e010d a4d3600 99e010d a4d3600 99e010d a4d3600 99e010d a4d3600 bf20fdb a4d3600 99e010d a4d3600 99e010d a4d3600 99e010d a4d3600 99e010d a4d3600 99e010d a4d3600 99e010d a4d3600 99e010d a4d3600 99e010d a4d3600 99e010d a4d3600 99e010d a4d3600 99e010d a4d3600 99e010d a4d3600 99e010d a4d3600 99e010d a4d3600 99e010d a4d3600 99e010d a4d3600 99e010d a4d3600 99e010d a4d3600 99e010d a4d3600 99e010d a4d3600 99e010d a4d3600 99e010d a4d3600 99e010d a4d3600 99e010d a4d3600 99e010d a4d3600 99e010d a4d3600 99e010d a4d3600 99e010d a4d3600 d9a0d0e 99e010d a4d3600 d9a0d0e 99e010d a4d3600 99e010d a4d3600 99e010d a4d3600 d9a0d0e a4d3600 99e010d a4d3600 99e010d a4d3600 99e010d a4d3600 99e010d a4d3600 99e010d a4d3600 99e010d a4d3600 99e010d a4d3600 99e010d a4d3600 99e010d a4d3600 99e010d a4d3600 99e010d a4d3600 99e010d a4d3600 99e010d a4d3600 99e010d a4d3600 99e010d a4d3600 99e010d a4d3600 99e010d a4d3600 99e010d a4d3600 99e010d a4d3600 99e010d a4d3600 99e010d a4d3600 99e010d a4d3600 99e010d a4d3600 99e010d a4d3600 99e010d a4d3600 99e010d d9a0d0e a4d3600 99e010d a4d3600 99e010d d5cf678 98335ad 99e010d a4d3600 99e010d a4d3600 99e010d a4d3600 99e010d d9a0d0e 99e010d a4d3600 99e010d d9a0d0e 99e010d a4d3600 99e010d d9a0d0e 99e010d d9a0d0e |
1 2 3 4 5 6 7 8 9 10 11 12 13 14 15 16 17 18 19 20 21 22 23 24 25 26 27 28 29 30 31 32 33 34 35 36 37 38 39 40 41 42 43 44 45 46 47 48 49 50 51 52 53 54 55 56 57 58 59 60 61 62 63 64 65 66 67 68 69 70 71 72 73 74 75 76 77 78 79 80 81 82 83 84 85 86 87 88 89 90 91 92 93 94 95 96 97 98 99 100 101 102 103 104 105 106 107 108 109 110 111 112 113 114 115 116 117 118 119 120 121 122 123 124 125 126 127 128 129 130 131 132 133 134 135 136 137 138 139 140 141 142 143 144 145 146 147 148 149 150 151 152 153 154 155 156 157 158 159 160 161 162 163 164 165 166 167 168 169 170 171 172 173 174 175 176 177 178 179 180 181 182 183 184 185 186 187 188 189 190 191 192 193 194 195 196 197 198 199 200 201 202 203 204 205 206 207 208 209 210 211 212 213 214 215 216 217 218 219 220 221 222 223 224 225 226 227 228 229 230 231 232 233 234 235 236 237 238 239 240 241 242 243 244 245 246 247 248 249 250 251 252 253 254 255 256 257 258 259 260 261 262 263 264 265 266 267 268 269 270 271 272 273 274 275 276 277 278 279 280 281 282 283 284 285 286 287 288 289 290 291 292 293 294 295 296 297 298 299 300 301 302 303 304 305 306 307 308 309 310 311 312 313 314 315 316 317 318 319 320 321 322 323 324 325 326 327 328 329 330 331 332 333 334 335 336 337 338 339 340 341 342 343 344 345 346 347 348 349 350 351 352 353 354 355 356 357 358 359 360 361 362 363 364 365 366 367 368 369 370 371 372 373 374 375 376 377 378 379 380 381 382 383 384 385 386 387 388 389 390 391 392 393 394 395 396 397 398 399 400 401 402 403 404 405 406 407 408 409 410 411 412 413 414 415 416 417 418 419 420 421 422 423 424 425 426 427 428 429 430 431 432 433 434 435 436 437 438 439 440 441 442 443 444 445 446 447 448 449 450 451 452 453 454 455 456 457 458 459 460 461 462 463 464 465 466 467 468 469 470 471 472 473 474 475 476 477 478 479 480 481 482 483 484 485 486 487 488 489 490 491 492 493 494 495 496 497 498 499 500 501 502 503 504 505 506 507 508 509 510 511 512 513 514 515 516 517 518 519 520 521 522 523 524 525 526 527 528 529 530 531 532 533 534 535 536 537 538 539 540 541 542 543 544 545 546 547 548 549 550 551 552 553 554 555 556 557 558 559 560 561 562 563 564 565 566 567 568 569 570 571 572 573 574 575 576 577 578 579 580 581 582 583 584 585 586 587 588 589 590 591 592 593 594 595 596 597 598 599 600 601 602 603 604 605 606 607 608 609 610 611 612 613 614 615 616 617 618 619 620 621 622 623 624 625 626 627 628 629 630 631 632 633 634 635 636 637 638 639 640 641 642 643 644 645 646 647 648 649 650 651 652 653 654 655 656 657 658 659 660 661 662 663 664 665 666 667 668 669 670 |
---
license: cc-by-nc-sa-4.0
language:
- en
- tr
tags:
- eeg
- brain
- deeplearning
- artificialintelligence
- ai
- model
- emotions
- neuroscience
- neura
- neuro
---
# bai Modelleri
## Model Detayları
#### bai Modelleri EEG verilerini okumak için eğitilmiştir. Bu modellerin eğitildiği veri setleri Neurazum tarafından gizli tutulmaktadır. Derin öğrenme yöntemleri ile eğitilir ve çok yüksek doğruluk oranları ile EEG üzerinde hassas bir şekilde çalışabilir. Elektrot sayısına bakılmaksızın her türlü EEG cihazı üzerinde çalışabilmektedir (Optimizasyon ve iyileştirmeler devam etmektedir). Nörobilim alanındaki geri kalmışlığa, ilkelliğe ve hata paylarına son vermeyi hedeflemektedir.
### Model Tanımı
- **Geliştirici:** _Neurazum_
- **Yayımcı:** _Eyüp İpler_
- **Model Tipi:** _EEG_
- **Lisans:** _CC-BY-NC-SA-4.0_
## Kullanımlar
**Bu modellerdeki amacımız;**
- _Kişinin duygusunu anlık olarak analiz etmek,_
- _Epilepsi ve MS gibi tehlikeli hastalıkları nöbet öncesi erken uyarmak ve gerekli önlemleri almak,_
- _Alzheimer hastaları için erken teşhis ve unutulan kelimeleri bai modeline aktararak hafızada tutmak,_
- _Günlük hayatta kullanılabilecek bir sesli yapay zeka asistanının geliştirilmesi,_
- _İnsan vücudunda bulunan 12 adet kraniyal sinir sayesinde hastalık teşhisinde hata payının azaltılması._
## Direkt Kullanımlar
**Klasik Kullanım:**
```python
import numpy as np
import pandas as pd
from sklearn.preprocessing import StandardScaler
from tensorflow.keras.models import load_model
import matplotlib.pyplot as plt
model_path = 'model-yolu'
model = load_model(model_path)
model_name = model_path.split('/')[-1].split('.')[0]
plt.figure(figsize=(10, 6))
plt.title(f'Duygu Tahmini ({model_name})')
plt.xlabel('Zaman')
plt.ylabel('Sınıf')
plt.legend(loc='upper right')
plt.grid(True)
plt.show()
model.summary()
```
**Tahmin Testi:**
```python
import numpy as np
import pandas as pd
from sklearn.preprocessing import StandardScaler
from tensorflow.keras.models import load_model
model_path = 'model-yolu'
model = load_model(model_path)
scaler = StandardScaler()
predictions = model.predict(X_new_reshaped)
predicted_labels = np.argmax(predictions, axis=1)
label_mapping = {'NEGATIVE': 0, 'NEUTRAL': 1, 'POSITIVE': 2}
label_mapping_reverse = {v: k for k, v in label_mapping.items()}
#new_input = np.array([[23, 465, 12, 9653] * 637])
new_input = np.random.rand(1, 2548) # 1 örnek ve 2548 özellik
new_input_scaled = scaler.fit_transform(new_input)
new_input_reshaped = new_input_scaled.reshape((new_input_scaled.shape[0], 1, new_input_scaled.shape[1]))
new_prediction = model.predict(new_input_reshaped)
predicted_label = np.argmax(new_prediction, axis=1)[0]
predicted_emotion = label_mapping_reverse[predicted_label]
# TR Lang
if predicted_emotion == 'NEGATIVE':
predicted_emotion = 'Negatif'
elif predicted_emotion == 'NEUTRAL':
predicted_emotion = 'Nötr'
elif predicted_emotion == 'POSITIVE':
predicted_emotion = 'Pozitif'
print(f'Girilen Veri: {new_input}')
print(f'Tahmin Edilen Duygu: {predicted_emotion}')
```
**Gerçek Zamanlı Kullanım (Modelsiz):**
```python
import sys
import pyaudio
import numpy as np
import matplotlib.pyplot as plt
from matplotlib.lines import Line2D
from PyQt5.QtWidgets import QApplication, QMainWindow, QPushButton, QVBoxLayout, QWidget
from PyQt5.QtCore import QTimer
from PyQt5.QtGui import QIcon
from matplotlib.backends.backend_qt5agg import FigureCanvasQTAgg as FigureCanvas
from matplotlib.backends.backend_qt5agg import NavigationToolbar2QT as NavigationToolbar
CHUNK = 1000 # Chunk size
FORMAT = pyaudio.paInt16 # Data type (16-bit PCM)
CHANNELS = 1 # (Mono)
RATE = 2000 # Sample rate (Hz)
p = pyaudio.PyAudio()
stream = p.open(format=FORMAT,
channels=CHANNELS,
rate=RATE,
input=True,
frames_per_buffer=CHUNK)
class MainWindow(QMainWindow):
def __init__(self):
super().__init__()
self.initUI()
self.timer = QTimer()
self.timer.timeout.connect(self.update_plot)
self.timer.start(1)
def initUI(self):
self.setWindowTitle('EEG Monitoring by Neurazum')
self.setWindowIcon(QIcon('/neurazumicon.ico'))
self.central_widget = QWidget()
self.setCentralWidget(self.central_widget)
self.layout = QVBoxLayout(self.central_widget)
self.fig, (self.ax1, self.ax2) = plt.subplots(2, 1, figsize=(12, 8), gridspec_kw={'height_ratios': [9, 1]})
self.fig.tight_layout()
self.canvas = FigureCanvas(self.fig)
self.layout.addWidget(self.canvas)
self.toolbar = NavigationToolbar(self.canvas, self)
self.layout.addWidget(self.toolbar)
self.x = np.arange(0, 2 * CHUNK, 2)
self.line1, = self.ax1.plot(self.x, np.random.rand(CHUNK))
self.line2, = self.ax2.plot(self.x, np.random.rand(CHUNK))
self.legend_elements = [
Line2D([0, 4], [0], color='yellow', lw=4, label='DELTA (0hz-4hz)'),
Line2D([4, 7], [0], color='blue', lw=4, label='TETA (4hz-7hz)'),
Line2D([8, 12], [0], color='green', lw=4, label='ALFA (8hz-12hz)'),
Line2D([12, 30], [0], color='red', lw=4, label='BETA (12hz-30hz)'),
Line2D([30, 100], [0], color='purple', lw=4, label='GAMA (30hz-100hz)')
]
def update_plot(self):
data = np.frombuffer(stream.read(CHUNK), dtype=np.int16)
data = np.abs(data)
voltage_data = data * (3.3 / 1024) # Voltajı "mV"'ye dönüştürme
frequency = voltage_data / (RATE * 1000) # Frekans hesaplama
self.line1.set_ydata(data)
self.line2.set_ydata(frequency)
for coll in self.ax1.collections:
coll.remove()
self.ax1.fill_between(self.x, data, where=((self.x >= 0) & (self.x <= 4)), color='yellow', alpha=1)
self.ax1.fill_between(self.x, data, where=((self.x >= 4) & (self.x <= 7)), color='blue', alpha=1)
self.ax1.fill_between(self.x, data, where=((self.x >= 8) & (self.x <= 12)), color='green', alpha=1)
self.ax1.fill_between(self.x, data, where=((self.x >= 12) & (self.x <= 30)), color='red', alpha=1)
self.ax1.fill_between(self.x, data, where=((self.x >= 30) & (self.x <= 100)), color='purple', alpha=1)
self.ax1.legend(handles=self.legend_elements, loc='upper right')
self.ax1.set_ylabel('Genlik (uV)')
self.ax1.set_xlabel('Frekans (Hz)')
self.ax1.set_title('Frekans ve Genlik Değerleri')
self.ax2.set_ylabel('Voltaj (mV)')
self.ax2.set_xlabel('Zaman')
self.canvas.draw()
def close_application(self):
self.timer.stop()
stream.stop_stream()
stream.close()
p.terminate()
sys.exit(app.exec_())
if __name__ == '__main__':
app = QApplication(sys.argv)
mainWin = MainWindow()
mainWin.show()
sys.exit(app.exec_())
```
**Duyguları İçeren Veri Seti Üzerinde Tahmin:**
```python
import numpy as np
import pandas as pd
from sklearn.preprocessing import StandardScaler
from tensorflow.keras.models import load_model
model_path = 'model-yolu'
new_data_path = 'veri-seti-yolu'
model = load_model(model_path)
new_data = pd.read_csv(new_data_path)
X_new = new_data.drop('label', axis=1)
y_new = new_data['label']
scaler = StandardScaler()
X_new_scaled = scaler.fit_transform(X_new)
X_new_reshaped = X_new_scaled.reshape((X_new_scaled.shape[0], 1, X_new_scaled.shape[1]))
predictions = model.predict(X_new_reshaped)
predicted_labels = np.argmax(predictions, axis=1)
label_mapping = {'NEGATIVE': 0, 'NEUTRAL': 1, 'POSITIVE': 2}
label_mapping_reverse = {v: k for k, v in label_mapping.items()}
actual_labels = y_new.replace(label_mapping).values
accuracy = np.mean(predicted_labels == actual_labels)
new_input = np.random.rand(2548, 2548) # 1 örnek ve 2548 özellik
new_input_scaled = scaler.transform(new_input)
new_input_reshaped = new_input_scaled.reshape((new_input_scaled.shape[0], 1, new_input_scaled.shape[1]))
new_prediction = model.predict(new_input_reshaped)
predicted_label = np.argmax(new_prediction, axis=1)[0]
predicted_emotion = label_mapping_reverse[predicted_label]
# TR Lang
if predicted_emotion == 'NEGATIVE':
predicted_emotion = 'Negatif'
elif predicted_emotion == 'NEUTRAL':
predicted_emotion = 'Nötr'
elif predicted_emotion == 'POSITIVE':
predicted_emotion = 'Pozitif'
print(f'Giriş Verisi: {new_input}')
print(f'Tahmin Edilen Duygu: {predicted_emotion}')
print(f'Doğruluk: %{accuracy * 100:.5f}')
```
## Önyargı, Riskler ve Kısıtlamalar
**bai Modelleri;**
- _En büyük riski yanlış tahmin etmesidir :),_
- _Herhangi bir kısıtlama bulunmamaktadır (şimdilik),_
- _Beyin sinyallerinden elde edilen veriler kişisel bilgi içermez (çünkü bunlar sadece mV değerleridir). Bu nedenle, bai tarafından yapılan her tahmin sadece bir "TAHMİN" dir._
### Öneriler
- _Çok fazla duygu durum değişikliği yaşamamaya çalışın,_
- _Çok fazla farklı nitelikte düşünce/karar almayın,_
- _Çok fazla hata yaptığında, yanlış cevap verdiğini düşünmeyin (doğru cevap verdiğini farz edin),_
**Not: Bu öğeler sadece modelin daha iyi çalışması için önerilerdir. Herhangi bir risk taşımazlar.**
## Modele Nasıl Başlanır
- Modelin içeriğindeki gerekli modülleri kurmak için;
- ```bash
pip install -r requirements.txt
```
- Örnek kullanımla modelin ve veri setinin yolunu yerleştirin.
- Ve dosyayı çalıştırın.
## Değerlendirme
- bai-2.0 => (Doğruluk oranı çok yüksek = %97,93621013133208) (DUYGUSAL SINIFLANDIRMA)
- bai-2.1 => (Doğruluk oranı çok yüksek = %97,93621013133208) (DUYGUSAL SINIFLANDIRMA)
- bai-2.2 => (Doğruluk oranı çok yüksek = %94,8874296435272) (DUYGUSAL SINIFLANDIRMA)
- bai-3.0 Emotion => (Doğruluk oranı çok yüksek = %97,79549718574108) (DUYGUSAL SINIFLANDIRMA)
- bai-3.0 Epilepsy => (Doğruluk oranı yüksek = %68,90829694323143) (EPİLEPSİ NÖBET TESPİTİ)
- bai-3.1 Emotion => (Doğruluk oranı çok yüksek = %95,1219512195122) (DUYGUSAL SINIFLANDIRMA)
### Sonuçlar
[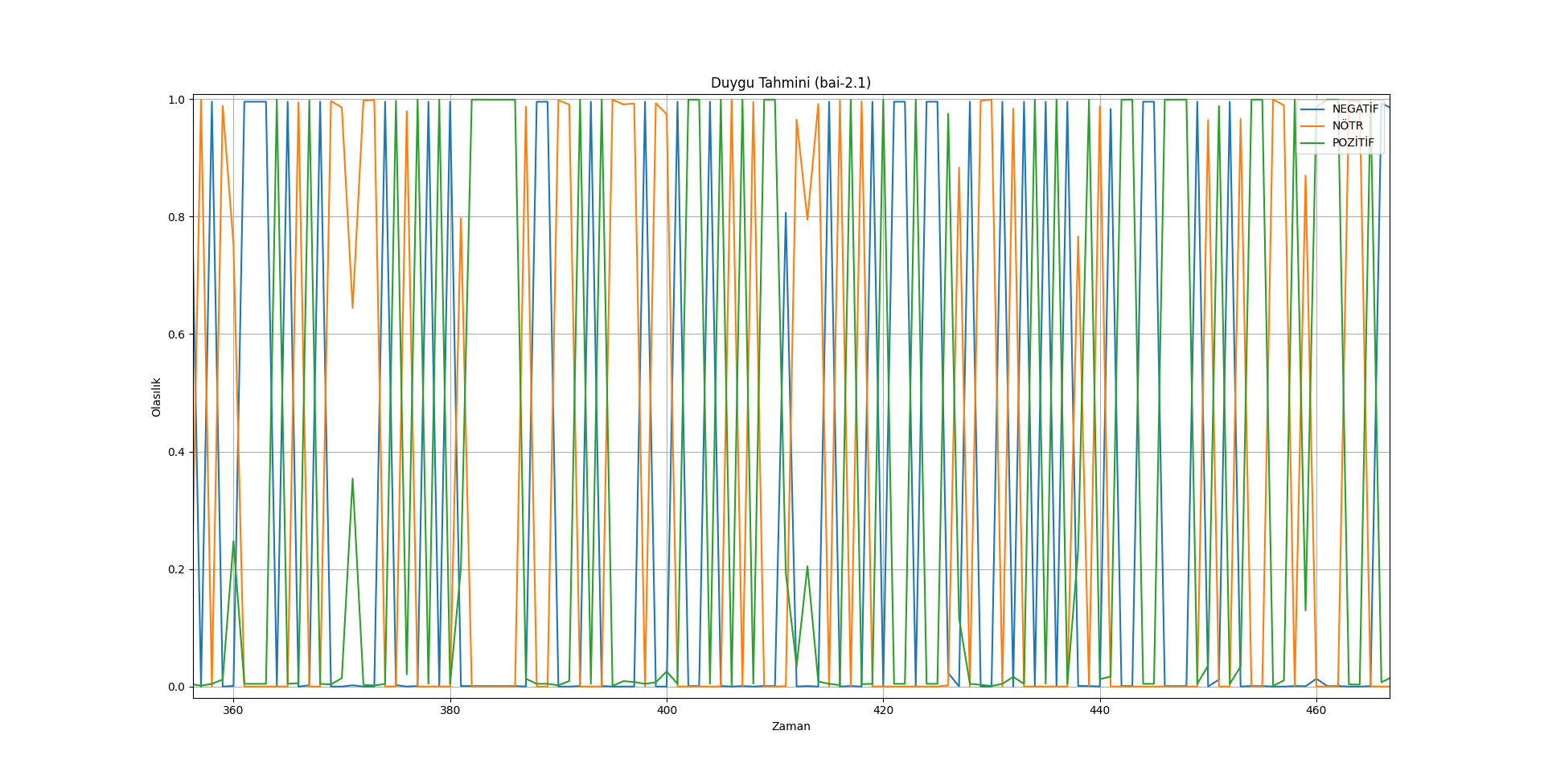](https://resimlink.com/O7GyMoQL)
[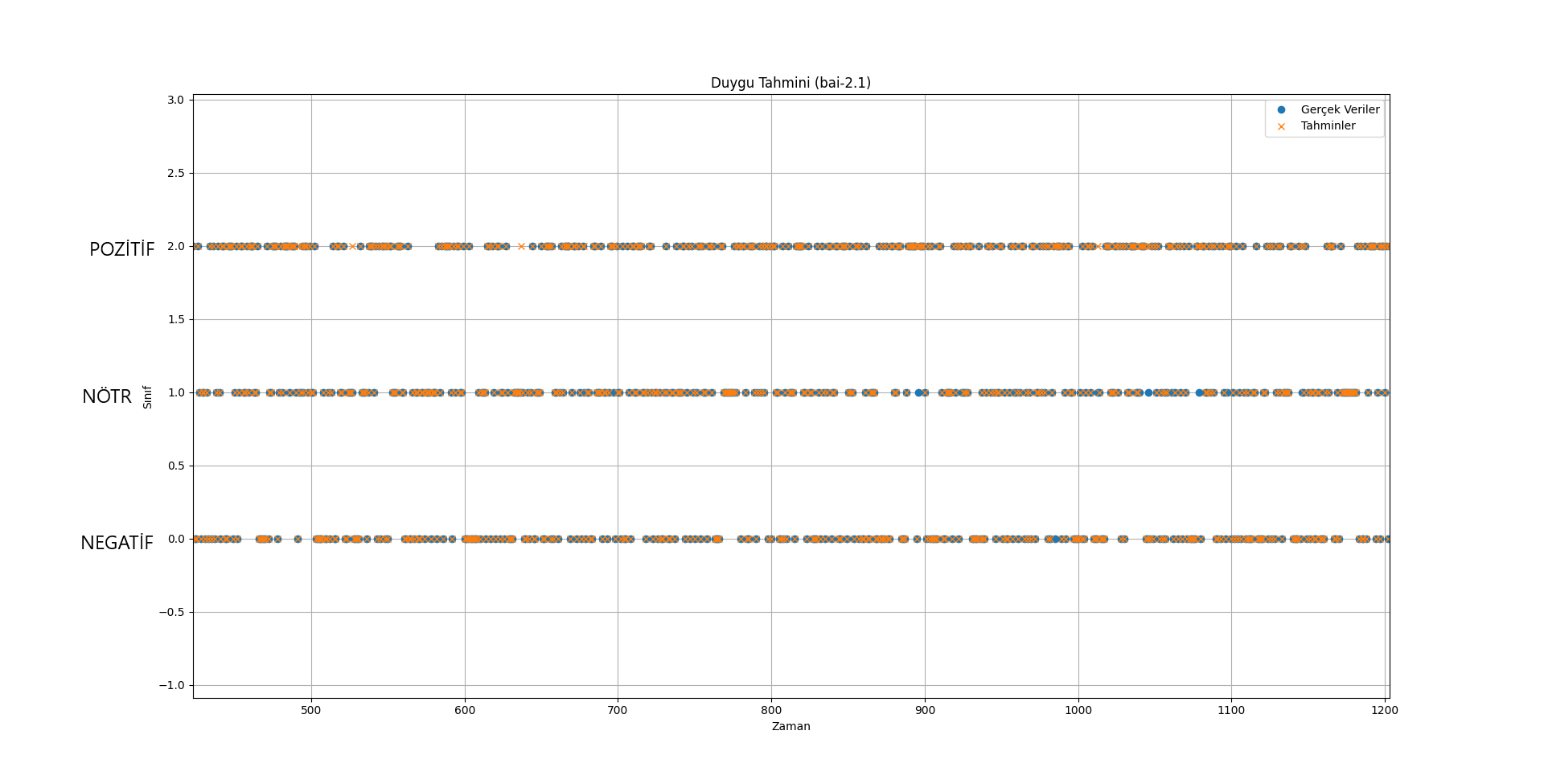](https://resimlink.com/gdyCW3RP)
[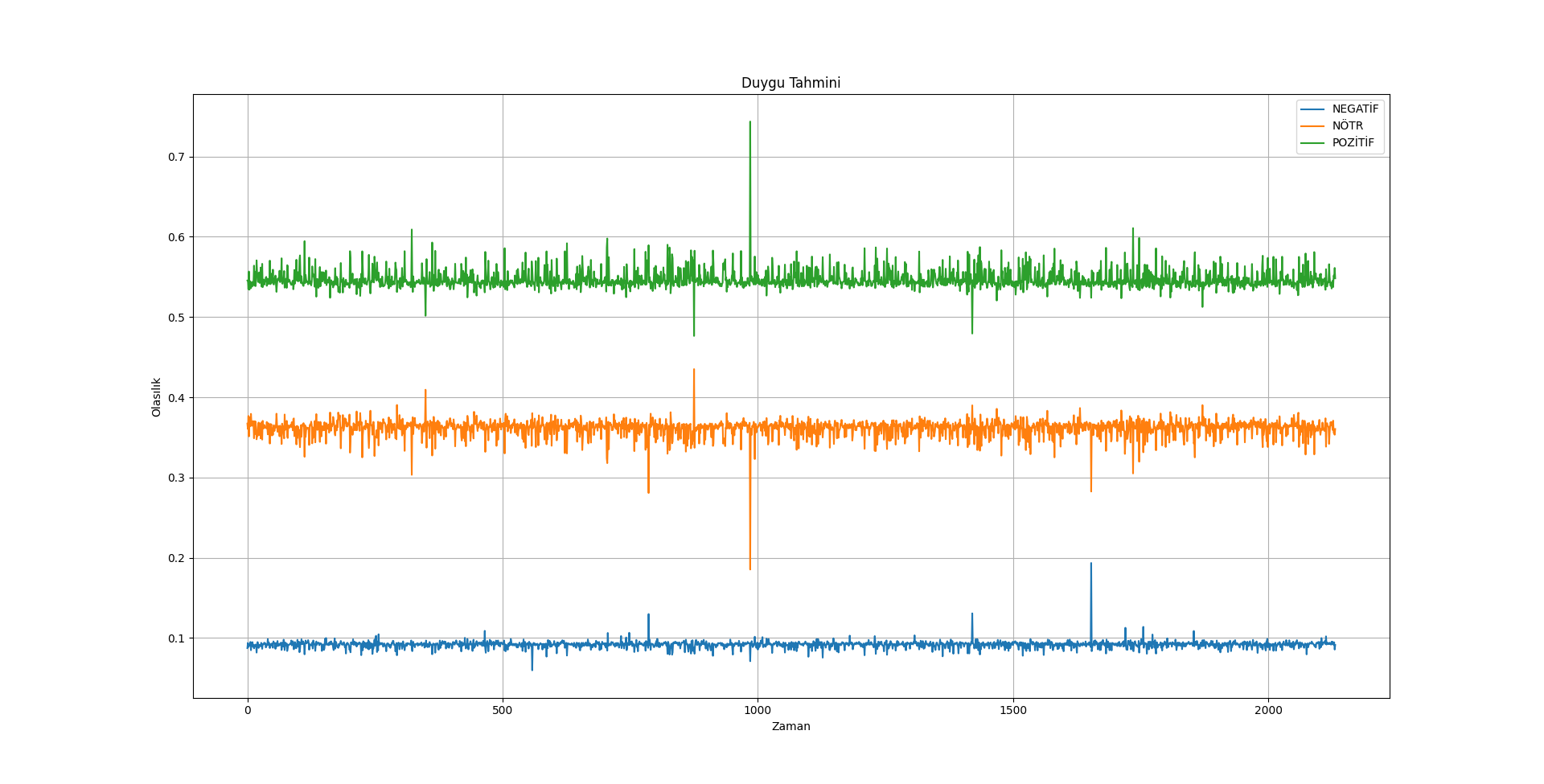](https://resimlink.com/MpH9XS_0E)
[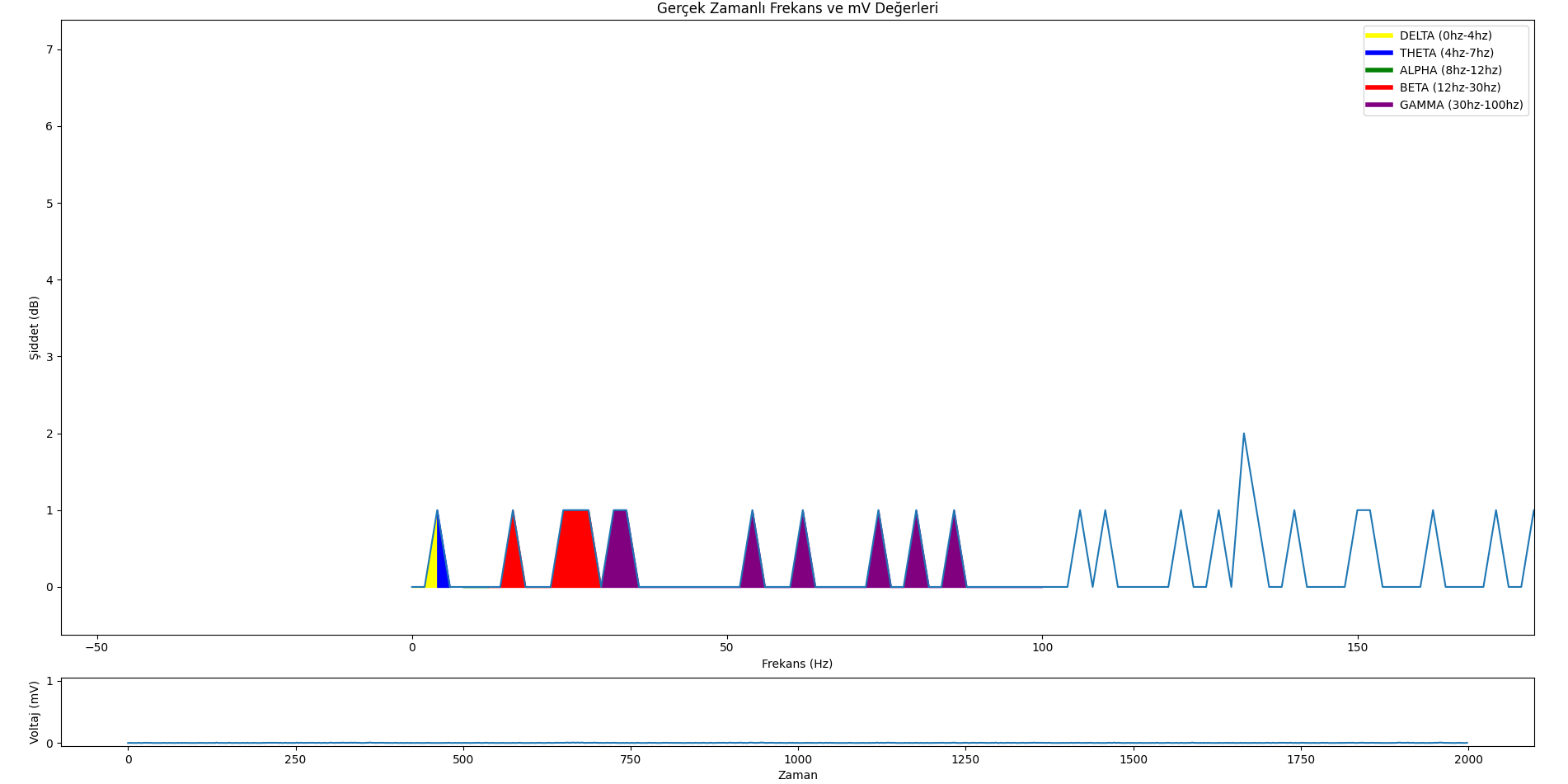](https://resimlink.com/vsyYqJnQ4k)
#### Özet
Özetle bai modelleri, kişinin düşüncelerini ve duygularını öğrenmek ve tahmin etmek için geliştirilmeye devam ediyor.
#### Donanım
Tek ihtiyacınız olan şey EEG!
#### Yazılım
Daha sonra bu EEG cihazını (şimdilik sadece ses girişi ile) yayınladığımız gerçek zamanlı veri izleme uygulamasıyla çalıştırabilirsiniz.
GitHub: https://github.com/neurazum/Realtime-EEG-Monitoring
## Daha Fazla
LinkedIn: https://www.linkedin.com/company/neurazum
### Yazar
Eyüp İpler - https://www.linkedin.com/in/eyupipler/
### İletişim
neurazum@gmail.com
# --------------------------------------
# bai Models
## Model Details
#### bai Models are trained to read EEG data. The data sets on which these models are trained are kept confidential by Neurazum. It is trained with deep learning methods and can work precisely on EEG with very high accuracy rates. It can work on all kinds of EEG devices regardless of the number of electrodes (Optimisation and improvements are ongoing). It aims to end the backwardness, primitiveness and error margins in the field of neuroscience.
### Model Description
- **Developed by:** _Neurazum_
- **Shared by:** _Eyüp İpler_
- **Model type:** _EEG_
- **License:** _CC-BY-NC-SA-4.0_
## Uses
**Our aim in these models;**
- _To analyse the person's emotion instantly,_
- _To warn dangerous patients such as epilepsy and MS early before the seizure and to take the necessary precautions,_
- _Early diagnosis for Alzheimer's patients and the bai model helps the person by memorising forgotten words,_
- _Development of a voice assistant that can be used in everyday life,_
- _Reducing the margin of error in disease diagnosis thanks to the 12 cranial nerves in the human body._
## Direct Uses
**Classical Use:**
```python
import numpy as np
import pandas as pd
from sklearn.preprocessing import StandardScaler
from tensorflow.keras.models import load_model
import matplotlib.pyplot as plt
model_path = 'model-path'
model = load_model(model_path)
model_name = model_path.split('/')[-1].split('.')[0]
plt.figure(figsize=(10, 6))
plt.title(f'Emotion Prediction ({model_name})')
plt.xlabel('Time')
plt.ylabel('Class')
plt.legend(loc='upper right')
plt.grid(True)
plt.show()
model.summary()
```
**Prediction Test:**
```python
import numpy as np
import pandas as pd
from sklearn.preprocessing import StandardScaler
from tensorflow.keras.models import load_model
model_path = 'model-path'
model = load_model(model_path)
scaler = StandardScaler()
predictions = model.predict(X_new_reshaped)
predicted_labels = np.argmax(predictions, axis=1)
label_mapping = {'NEGATIVE': 0, 'NEUTRAL': 1, 'POSITIVE': 2}
label_mapping_reverse = {v: k for k, v in label_mapping.items()}
#new_input = np.array([[23, 465, 12, 9653] * 637])
new_input = np.random.rand(1, 2548) # 1 sample and 2548 features
new_input_scaled = scaler.fit_transform(new_input)
new_input_reshaped = new_input_scaled.reshape((new_input_scaled.shape[0], 1, new_input_scaled.shape[1]))
new_prediction = model.predict(new_input_reshaped)
predicted_label = np.argmax(new_prediction, axis=1)[0]
predicted_emotion = label_mapping_reverse[predicted_label]
# TR Lang
if predicted_emotion == 'NEGATIVE':
predicted_emotion = 'Negatif'
elif predicted_emotion == 'NEUTRAL':
predicted_emotion = 'Nötr'
elif predicted_emotion == 'POSITIVE':
predicted_emotion = 'Pozitif'
print(f'Input Data: {new_input}')
print(f'Predicted Emotion: {predicted_emotion}')
```
**Realtime Use (EEG Monitoring without AI Model):**
```python
import sys
import pyaudio
import numpy as np
import matplotlib.pyplot as plt
from matplotlib.lines import Line2D
from PyQt5.QtWidgets import QApplication, QMainWindow, QPushButton, QVBoxLayout, QWidget
from PyQt5.QtCore import QTimer
from PyQt5.QtGui import QIcon
from matplotlib.backends.backend_qt5agg import FigureCanvasQTAgg as FigureCanvas
from matplotlib.backends.backend_qt5agg import NavigationToolbar2QT as NavigationToolbar
CHUNK = 1000 # Chunk size
FORMAT = pyaudio.paInt16 # Data type (16-bit PCM)
CHANNELS = 1 # (Mono)
RATE = 2000 # Sample rate (Hz)
p = pyaudio.PyAudio()
stream = p.open(format=FORMAT,
channels=CHANNELS,
rate=RATE,
input=True,
frames_per_buffer=CHUNK)
class MainWindow(QMainWindow):
def __init__(self):
super().__init__()
self.initUI()
self.timer = QTimer()
self.timer.timeout.connect(self.update_plot)
self.timer.start(1)
def initUI(self):
self.setWindowTitle('EEG Monitoring by Neurazum')
self.setWindowIcon(QIcon('/neurazumicon.ico'))
self.central_widget = QWidget()
self.setCentralWidget(self.central_widget)
self.layout = QVBoxLayout(self.central_widget)
self.fig, (self.ax1, self.ax2) = plt.subplots(2, 1, figsize=(12, 8), gridspec_kw={'height_ratios': [9, 1]})
self.fig.tight_layout()
self.canvas = FigureCanvas(self.fig)
self.layout.addWidget(self.canvas)
self.toolbar = NavigationToolbar(self.canvas, self)
self.layout.addWidget(self.toolbar)
self.x = np.arange(0, 2 * CHUNK, 2)
self.line1, = self.ax1.plot(self.x, np.random.rand(CHUNK))
self.line2, = self.ax2.plot(self.x, np.random.rand(CHUNK))
self.legend_elements = [
Line2D([0, 4], [0], color='yellow', lw=4, label='DELTA (0hz-4hz)'),
Line2D([4, 7], [0], color='blue', lw=4, label='THETA (4hz-7hz)'),
Line2D([8, 12], [0], color='green', lw=4, label='ALPHA (8hz-12hz)'),
Line2D([12, 30], [0], color='red', lw=4, label='BETA (12hz-30hz)'),
Line2D([30, 100], [0], color='purple', lw=4, label='GAMMA (30hz-100hz)')
]
def update_plot(self):
data = np.frombuffer(stream.read(CHUNK), dtype=np.int16)
data = np.abs(data)
voltage_data = data * (3.3 / 1024) # Voltage to "mV"
frequency = voltage_data / (RATE * 1000) # Calculate to frequency
self.line1.set_ydata(data)
self.line2.set_ydata(frequency)
for coll in self.ax1.collections:
coll.remove()
self.ax1.fill_between(self.x, data, where=((self.x >= 0) & (self.x <= 4)), color='yellow', alpha=1)
self.ax1.fill_between(self.x, data, where=((self.x >= 4) & (self.x <= 7)), color='blue', alpha=1)
self.ax1.fill_between(self.x, data, where=((self.x >= 8) & (self.x <= 12)), color='green', alpha=1)
self.ax1.fill_between(self.x, data, where=((self.x >= 12) & (self.x <= 30)), color='red', alpha=1)
self.ax1.fill_between(self.x, data, where=((self.x >= 30) & (self.x <= 100)), color='purple', alpha=1)
self.ax1.legend(handles=self.legend_elements, loc='upper right')
self.ax1.set_ylabel('Amplitude (uV)')
self.ax1.set_xlabel('Frequency (Hz)')
self.ax1.set_title('Frequency and mV')
self.ax2.set_ylabel('Voltage (mV)')
self.ax2.set_xlabel('Time')
self.canvas.draw()
def close_application(self):
self.timer.stop()
stream.stop_stream()
stream.close()
p.terminate()
sys.exit(app.exec_())
if __name__ == '__main__':
app = QApplication(sys.argv)
mainWin = MainWindow()
mainWin.show()
sys.exit(app.exec_())
```
**Emotion Dataset Prediction Use:**
```python
import numpy as np
import pandas as pd
from sklearn.preprocessing import StandardScaler
from tensorflow.keras.models import load_model
model_path = 'model-path'
new_data_path = 'dataset-path'
model = load_model(model_path)
new_data = pd.read_csv(new_data_path)
X_new = new_data.drop('label', axis=1)
y_new = new_data['label']
scaler = StandardScaler()
X_new_scaled = scaler.fit_transform(X_new)
X_new_reshaped = X_new_scaled.reshape((X_new_scaled.shape[0], 1, X_new_scaled.shape[1]))
predictions = model.predict(X_new_reshaped)
predicted_labels = np.argmax(predictions, axis=1)
label_mapping = {'NEGATIVE': 0, 'NEUTRAL': 1, 'POSITIVE': 2}
label_mapping_reverse = {v: k for k, v in label_mapping.items()}
actual_labels = y_new.replace(label_mapping).values
accuracy = np.mean(predicted_labels == actual_labels)
new_input = np.random.rand(2548, 2548) # 1 sample and 2548 features
new_input_scaled = scaler.transform(new_input)
new_input_reshaped = new_input_scaled.reshape((new_input_scaled.shape[0], 1, new_input_scaled.shape[1]))
new_prediction = model.predict(new_input_reshaped)
predicted_label = np.argmax(new_prediction, axis=1)[0]
predicted_emotion = label_mapping_reverse[predicted_label]
# TR Lang
if predicted_emotion == 'NEGATIVE':
predicted_emotion = 'Negatif'
elif predicted_emotion == 'NEUTRAL':
predicted_emotion = 'Nötr'
elif predicted_emotion == 'POSITIVE':
predicted_emotion = 'Pozitif'
print(f'Inputs: {new_input}')
print(f'Predicted Emotion: {predicted_emotion}')
print(f'Accuracy: %{accuracy * 100:.5f}')
```
## Bias, Risks, and Limitations
**bai Models;**
- _The biggest risk is wrong prediction :),_
- _It does not contain any restrictions in any area (for now),_
- _Data from brain signals do not contain personal information (because they are only mV values). Therefore, every guess made by bai is only a "GUESS"._
### Recommendations
- _Do not experience too many mood changes,_
- _Do not take thoughts/decisions with too many different qualities,_
- _When he/she makes a lot of mistakes, do not think that he/she gave the wrong answer (think of it as giving the correct answer),_
**Note: These items are only recommendations for better operation of the model. They do not carry any risk.**
## How to Get Started with the Model
- To install the necessary modules in the model;
- ```bash
pip install -r requirements.txt
```
- Place the path of the model in the example uses.
- And run the file.
## Evaluation
- bai-2.0 => (Accuracy very high = %97,93621013133208) (EMOTIONAL CLASSIFICATION)
- bai-2.1 => (Accuracy very high = %97,93621013133208) (EMOTIONAL CLASSIFICATION)
- bai-2.2 => (Accuracy very high = %94,8874296435272) (EMOTIONAL CLASSIFICATION)
- bai-3.0 Emotion => (Accuracy very high = %97,79549718574108) (EMOTIONAL CLASSIFICATION)
- bai-3.0 Epilepsy => (Accuracy high = %68,90829694323143) (SEIZURE DETECTION)
- bai-3.1 Emotion => (Accuracy very high = %95,1219512195122) (EMOTIONAL CLASSIFICATION)
### Results
[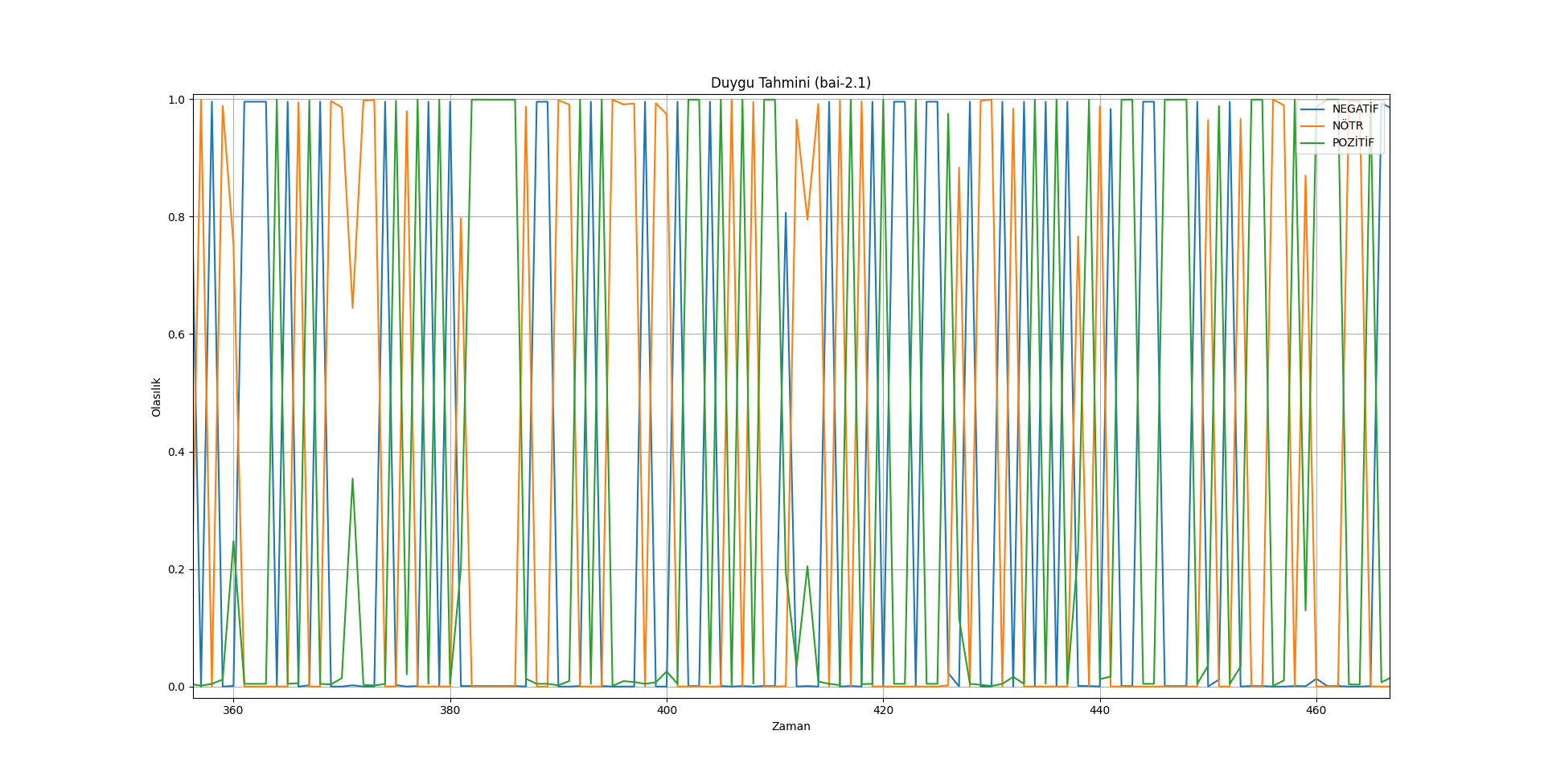](https://resimlink.com/O7GyMoQL)
[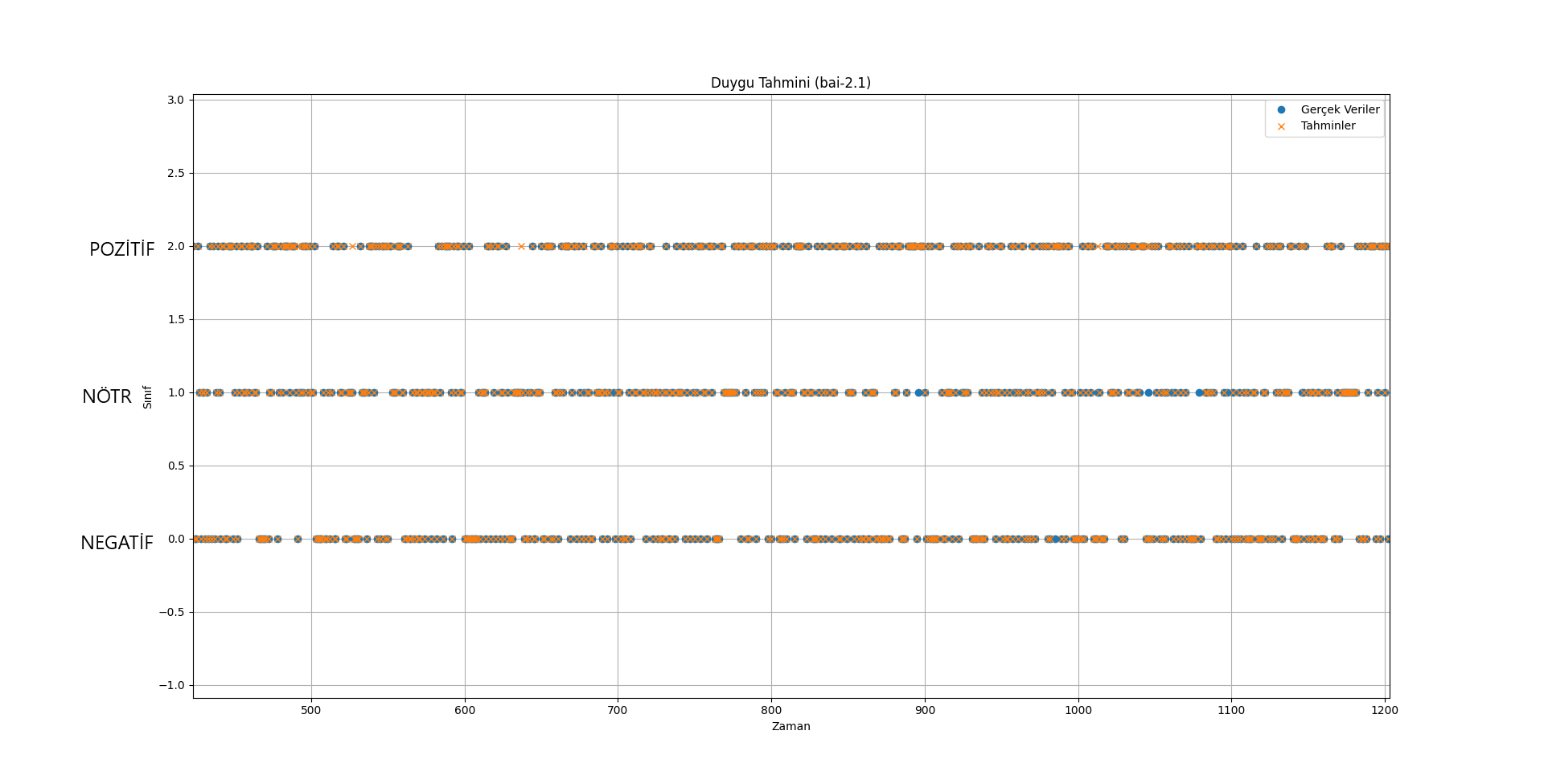](https://resimlink.com/gdyCW3RP)
[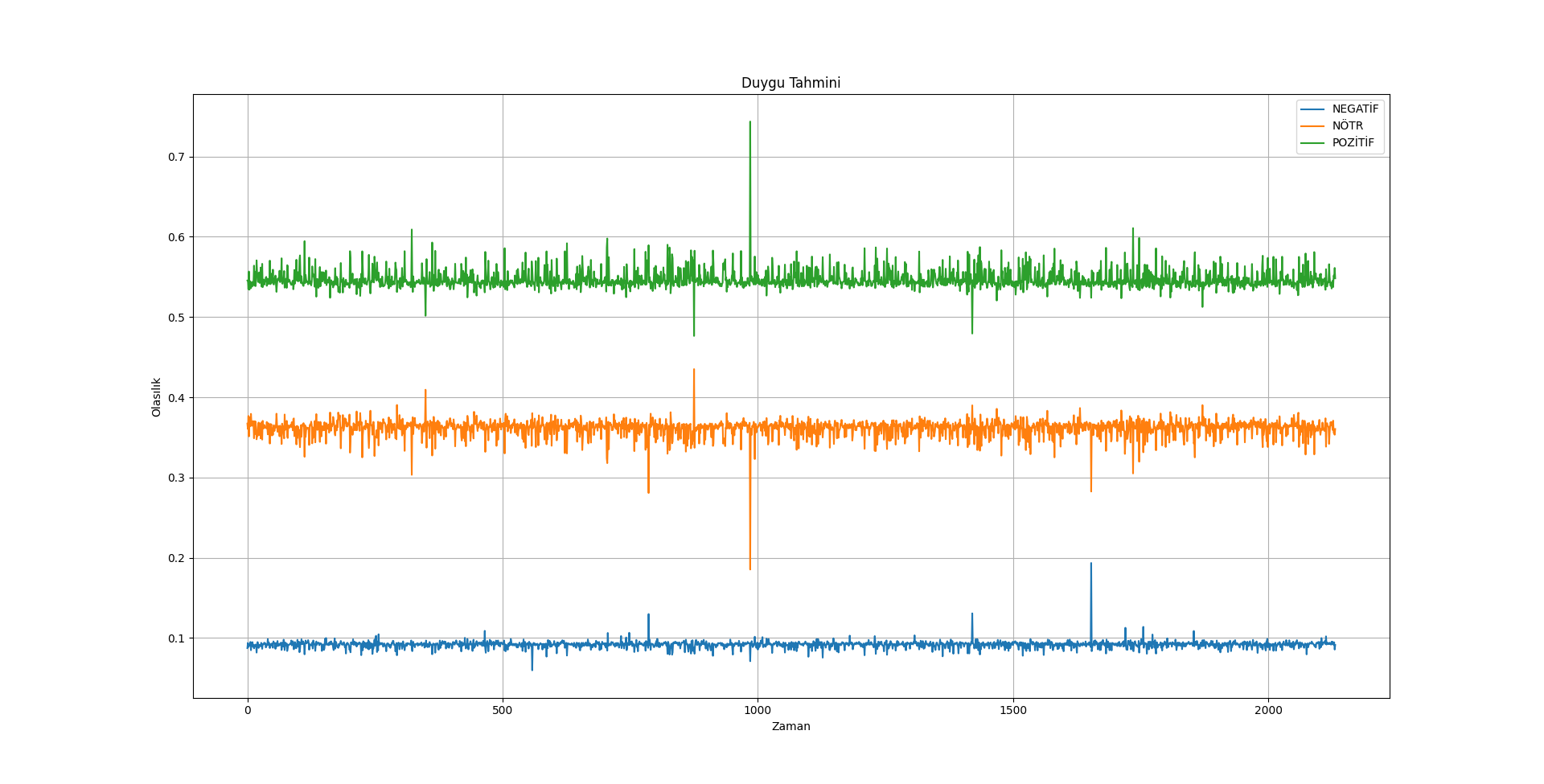](https://resimlink.com/MpH9XS_0E)
[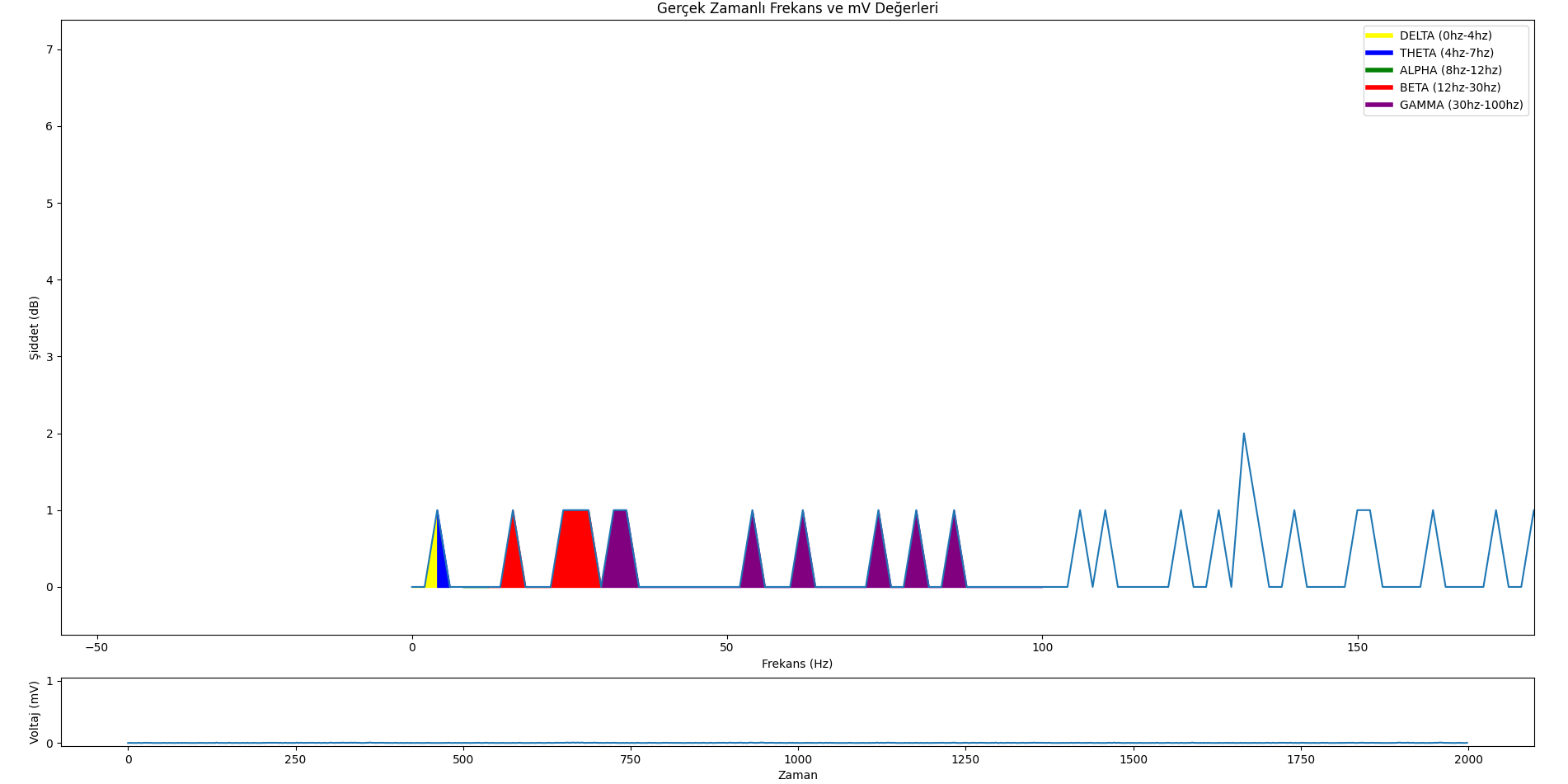](https://resimlink.com/vsyYqJnQ4k)
#### Summary
In summary, bai models continue to be developed to learn about and predict a person's thoughts and emotions.
#### Hardware
The EEG is the only hardware!
#### Software
You can then operate this EEG device (for the time being only with audio input) with the real-time data monitoring application we have published.
GitHub: https://github.com/neurazum/Realtime-EEG-Monitoring
## More
LinkedIn: https://www.linkedin.com/company/neurazum
### Author
Eyüp İpler - https://www.linkedin.com/in/eyupipler/
### Contact
neurazum@gmail.com
|