BLIP-2
Overview
BLIP-2 モデルは、BLIP-2: Bootstrapping Language-Image Pre-training with Frozen Image Encoders and Large Language Models で提案されました。 Junnan Li, Dongxu Li, Silvio Savarese, Steven Hoi.・サバレーゼ、スティーブン・ホイ。 BLIP-2 は、軽量の 12 層 Transformer をトレーニングすることで、フリーズされた事前トレーニング済み画像エンコーダーと大規模言語モデル (LLM) を活用します。 それらの間にエンコーダーを配置し、さまざまな視覚言語タスクで最先端のパフォーマンスを実現します。最も注目すべき点は、BLIP-2 が 800 億パラメータ モデルである Flamingo を 8.7% 改善していることです。 ゼロショット VQAv2 ではトレーニング可能なパラメーターが 54 分の 1 に減少します。
論文の要約は次のとおりです。
大規模モデルのエンドツーエンドのトレーニングにより、視覚と言語の事前トレーニングのコストはますます法外なものになってきています。この論文では、市販の凍結済み事前トレーニング画像エンコーダと凍結された大規模言語モデルから視覚言語の事前トレーニングをブートストラップする、汎用的で効率的な事前トレーニング戦略である BLIP-2 を提案します。 BLIP-2 は、2 段階で事前トレーニングされた軽量の Querying Transformer でモダリティのギャップを橋渡しします。最初のステージでは、フリーズされた画像エンコーダーから学習する視覚言語表現をブートストラップします。第 2 段階では、凍結された言語モデルから視覚から言語への生成学習をブートストラップします。 BLIP-2 は、既存の方法よりもトレーニング可能なパラメーターが大幅に少ないにもかかわらず、さまざまな視覚言語タスクで最先端のパフォーマンスを実現します。たとえば、私たちのモデルは、トレーニング可能なパラメーターが 54 分の 1 少ないゼロショット VQAv2 で、Flamingo80B を 8.7% 上回っています。また、自然言語の命令に従うことができる、ゼロショット画像からテキストへの生成というモデルの新しい機能も実証します
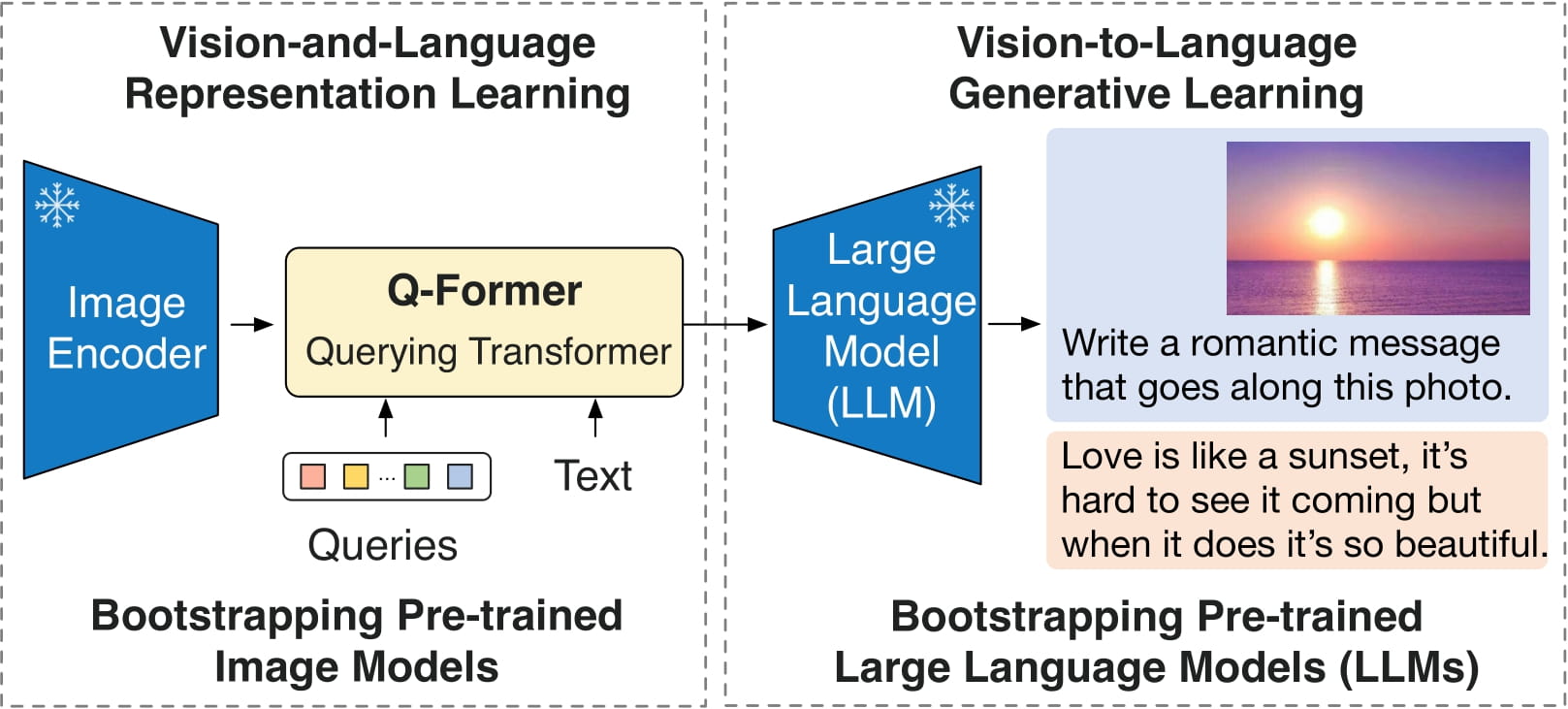
このモデルは、nielsr によって提供されました。 元のコードは ここ にあります。
Usage tips
- BLIP-2 は、画像とオプションのテキスト プロンプトを指定して条件付きテキストを生成するために使用できます。推論時には、
generate
メソッドを使用することをお勧めします。 - Blip2Processor を使用してモデル用の画像を準備し、予測されたトークン ID をデコードしてテキストに戻すことができます。
Resources
BLIP-2 の使用を開始するのに役立つ公式 Hugging Face およびコミュニティ (🌎 で示されている) リソースのリスト。
- 画像キャプション、ビジュアル質問応答 (VQA)、およびチャットのような会話のための BLIP-2 のデモ ノートブックは、こちら にあります。
ここに含めるリソースの送信に興味がある場合は、お気軽にプル リクエストを開いてください。審査させていただきます。リソースは、既存のリソースを複製するのではなく、何か新しいものを示すことが理想的です。
Blip2Config
class transformers.Blip2Config
< source >( vision_config = None qformer_config = None text_config = None num_query_tokens = 32 **kwargs )
Parameters
- vision_config (
dict
, optional) — Dictionary of configuration options used to initialize Blip2VisionConfig. - qformer_config (
dict
, optional) — Dictionary of configuration options used to initialize Blip2QFormerConfig. - text_config (
dict
, optional) — Dictionary of configuration options used to initialize any PretrainedConfig. - num_query_tokens (
int
, optional, defaults to 32) — The number of query tokens passed through the Transformer. - kwargs (optional) — Dictionary of keyword arguments.
Blip2Config is the configuration class to store the configuration of a Blip2ForConditionalGeneration. It is used to instantiate a BLIP-2 model according to the specified arguments, defining the vision model, Q-Former model and language model configs. Instantiating a configuration with the defaults will yield a similar configuration to that of the BLIP-2 Salesforce/blip2-opt-2.7b architecture.
Configuration objects inherit from PretrainedConfig and can be used to control the model outputs. Read the documentation from PretrainedConfig for more information.
Example:
>>> from transformers import (
... Blip2VisionConfig,
... Blip2QFormerConfig,
... OPTConfig,
... Blip2Config,
... Blip2ForConditionalGeneration,
... )
>>> # Initializing a Blip2Config with Salesforce/blip2-opt-2.7b style configuration
>>> configuration = Blip2Config()
>>> # Initializing a Blip2ForConditionalGeneration (with random weights) from the Salesforce/blip2-opt-2.7b style configuration
>>> model = Blip2ForConditionalGeneration(configuration)
>>> # Accessing the model configuration
>>> configuration = model.config
>>> # We can also initialize a Blip2Config from a Blip2VisionConfig, Blip2QFormerConfig and any PretrainedConfig
>>> # Initializing BLIP-2 vision, BLIP-2 Q-Former and language model configurations
>>> vision_config = Blip2VisionConfig()
>>> qformer_config = Blip2QFormerConfig()
>>> text_config = OPTConfig()
>>> config = Blip2Config.from_text_vision_configs(vision_config, qformer_config, text_config)
from_vision_qformer_text_configs
< source >( vision_config: Blip2VisionConfig qformer_config: Blip2QFormerConfig text_config: PretrainedConfig **kwargs ) → Blip2Config
Instantiate a Blip2Config (or a derived class) from a BLIP-2 vision model, Q-Former and language model configurations.
Blip2VisionConfig
class transformers.Blip2VisionConfig
< source >( hidden_size = 1408 intermediate_size = 6144 num_hidden_layers = 39 num_attention_heads = 16 image_size = 224 patch_size = 14 hidden_act = 'gelu' layer_norm_eps = 1e-06 attention_dropout = 0.0 initializer_range = 1e-10 qkv_bias = True **kwargs )
Parameters
- hidden_size (
int
, optional, defaults to 1408) — Dimensionality of the encoder layers and the pooler layer. - intermediate_size (
int
, optional, defaults to 6144) — Dimensionality of the “intermediate” (i.e., feed-forward) layer in the Transformer encoder. - num_hidden_layers (
int
, optional, defaults to 39) — Number of hidden layers in the Transformer encoder. - num_attention_heads (
int
, optional, defaults to 16) — Number of attention heads for each attention layer in the Transformer encoder. - image_size (
int
, optional, defaults to 224) — The size (resolution) of each image. - patch_size (
int
, optional, defaults to 14) — The size (resolution) of each patch. - hidden_act (
str
orfunction
, optional, defaults to"gelu"
) — The non-linear activation function (function or string) in the encoder and pooler. If string,"gelu"
,"relu"
,"selu"
and"gelu_new"
`"gelu"
are supported. layer_norm_eps (float
, optional, defaults to 1e-5): The epsilon used by the layer normalization layers. - attention_dropout (
float
, optional, defaults to 0.0) — The dropout ratio for the attention probabilities. - initializer_range (
float
, optional, defaults to 0.02) — The standard deviation of the truncated_normal_initializer for initializing all weight matrices. - qkv_bias (
bool
, optional, defaults toTrue
) — Whether to add a bias to the queries and values in the self-attention layers.
This is the configuration class to store the configuration of a Blip2VisionModel. It is used to instantiate a BLIP-2 vision encoder according to the specified arguments, defining the model architecture. Instantiating a configuration defaults will yield a similar configuration to that of the BLIP-2 Salesforce/blip2-opt-2.7b architecture.
Configuration objects inherit from PretrainedConfig and can be used to control the model outputs. Read the documentation from PretrainedConfig for more information.
Example:
>>> from transformers import Blip2VisionConfig, Blip2VisionModel
>>> # Initializing a Blip2VisionConfig with Salesforce/blip2-opt-2.7b style configuration
>>> configuration = Blip2VisionConfig()
>>> # Initializing a Blip2VisionModel (with random weights) from the Salesforce/blip2-opt-2.7b style configuration
>>> model = Blip2VisionModel(configuration)
>>> # Accessing the model configuration
>>> configuration = model.config
Blip2QFormerConfig
class transformers.Blip2QFormerConfig
< source >( vocab_size = 30522 hidden_size = 768 num_hidden_layers = 12 num_attention_heads = 12 intermediate_size = 3072 hidden_act = 'gelu' hidden_dropout_prob = 0.1 attention_probs_dropout_prob = 0.1 max_position_embeddings = 512 initializer_range = 0.02 layer_norm_eps = 1e-12 pad_token_id = 0 position_embedding_type = 'absolute' cross_attention_frequency = 2 encoder_hidden_size = 1408 **kwargs )
Parameters
- vocab_size (
int
, optional, defaults to 30522) — Vocabulary size of the Q-Former model. Defines the number of different tokens that can be represented by theinputs_ids
passed when calling the model. - hidden_size (
int
, optional, defaults to 768) — Dimensionality of the encoder layers and the pooler layer. - num_hidden_layers (
int
, optional, defaults to 12) — Number of hidden layers in the Transformer encoder. - num_attention_heads (
int
, optional, defaults to 12) — Number of attention heads for each attention layer in the Transformer encoder. - intermediate_size (
int
, optional, defaults to 3072) — Dimensionality of the “intermediate” (often named feed-forward) layer in the Transformer encoder. - hidden_act (
str
orCallable
, optional, defaults to"gelu"
) — The non-linear activation function (function or string) in the encoder and pooler. If string,"gelu"
,"relu"
,"silu"
and"gelu_new"
are supported. - hidden_dropout_prob (
float
, optional, defaults to 0.1) — The dropout probability for all fully connected layers in the embeddings, encoder, and pooler. - attention_probs_dropout_prob (
float
, optional, defaults to 0.1) — The dropout ratio for the attention probabilities. - max_position_embeddings (
int
, optional, defaults to 512) — The maximum sequence length that this model might ever be used with. Typically set this to something large just in case (e.g., 512 or 1024 or 2048). - initializer_range (
float
, optional, defaults to 0.02) — The standard deviation of the truncated_normal_initializer for initializing all weight matrices. - layer_norm_eps (
float
, optional, defaults to 1e-12) — The epsilon used by the layer normalization layers. - position_embedding_type (
str
, optional, defaults to"absolute"
) — Type of position embedding. Choose one of"absolute"
,"relative_key"
,"relative_key_query"
. For positional embeddings use"absolute"
. For more information on"relative_key"
, please refer to Self-Attention with Relative Position Representations (Shaw et al.). For more information on"relative_key_query"
, please refer to Method 4 in Improve Transformer Models with Better Relative Position Embeddings (Huang et al.). - cross_attention_frequency (
int
, optional, defaults to 2) — The frequency of adding cross-attention to the Transformer layers. - encoder_hidden_size (
int
, optional, defaults to 1408) — The hidden size of the hidden states for cross-attention.
This is the configuration class to store the configuration of a Blip2QFormerModel. It is used to instantiate a BLIP-2 Querying Transformer (Q-Former) model according to the specified arguments, defining the model architecture. Instantiating a configuration with the defaults will yield a similar configuration to that of the BLIP-2 Salesforce/blip2-opt-2.7b architecture. Configuration objects inherit from PretrainedConfig and can be used to control the model outputs. Read the documentation from PretrainedConfig for more information.
Note that Blip2QFormerModel is very similar to BertLMHeadModel with interleaved cross-attention.
Examples:
>>> from transformers import Blip2QFormerConfig, Blip2QFormerModel
>>> # Initializing a BLIP-2 Salesforce/blip2-opt-2.7b style configuration
>>> configuration = Blip2QFormerConfig()
>>> # Initializing a model (with random weights) from the Salesforce/blip2-opt-2.7b style configuration
>>> model = Blip2QFormerModel(configuration)
>>> # Accessing the model configuration
>>> configuration = model.config
Blip2Processor
class transformers.Blip2Processor
< source >( image_processor tokenizer )
Parameters
- image_processor (
BlipImageProcessor
) — An instance of BlipImageProcessor. The image processor is a required input. - tokenizer (
AutoTokenizer
) — An instance of [‘PreTrainedTokenizer`]. The tokenizer is a required input.
Constructs a BLIP-2 processor which wraps a BLIP image processor and an OPT/T5 tokenizer into a single processor.
BlipProcessor offers all the functionalities of BlipImageProcessor and AutoTokenizer. See the docstring
of __call__()
and decode() for more information.
This method forwards all its arguments to PreTrainedTokenizer’s batch_decode(). Please refer to the docstring of this method for more information.
This method forwards all its arguments to PreTrainedTokenizer’s decode(). Please refer to the docstring of this method for more information.
Blip2VisionModel
forward
< source >( pixel_values: Optional = None output_attentions: Optional = None output_hidden_states: Optional = None return_dict: Optional = None ) → transformers.modeling_outputs.BaseModelOutputWithPooling or tuple(torch.FloatTensor)
Parameters
- pixel_values (
torch.FloatTensor
of shape(batch_size, num_channels, height, width)
) — Pixel values. Pixel values can be obtained using Blip2Processor. SeeBlip2Processor.__call__()
for details. - output_attentions (
bool
, optional) — Whether or not to return the attentions tensors of all attention layers. Seeattentions
under returned tensors for more detail. - output_hidden_states (
bool
, optional) — Whether or not to return the hidden states of all layers. Seehidden_states
under returned tensors for more detail. - return_dict (
bool
, optional) — Whether or not to return a ModelOutput instead of a plain tuple.
Returns
transformers.modeling_outputs.BaseModelOutputWithPooling or tuple(torch.FloatTensor)
A transformers.modeling_outputs.BaseModelOutputWithPooling or a tuple of
torch.FloatTensor
(if return_dict=False
is passed or when config.return_dict=False
) comprising various
elements depending on the configuration (<class 'transformers.models.blip_2.configuration_blip_2.Blip2VisionConfig'>
) and inputs.
-
last_hidden_state (
torch.FloatTensor
of shape(batch_size, sequence_length, hidden_size)
) — Sequence of hidden-states at the output of the last layer of the model. -
pooler_output (
torch.FloatTensor
of shape(batch_size, hidden_size)
) — Last layer hidden-state of the first token of the sequence (classification token) after further processing through the layers used for the auxiliary pretraining task. E.g. for BERT-family of models, this returns the classification token after processing through a linear layer and a tanh activation function. The linear layer weights are trained from the next sentence prediction (classification) objective during pretraining. -
hidden_states (
tuple(torch.FloatTensor)
, optional, returned whenoutput_hidden_states=True
is passed or whenconfig.output_hidden_states=True
) — Tuple oftorch.FloatTensor
(one for the output of the embeddings, if the model has an embedding layer, + one for the output of each layer) of shape(batch_size, sequence_length, hidden_size)
.Hidden-states of the model at the output of each layer plus the optional initial embedding outputs.
-
attentions (
tuple(torch.FloatTensor)
, optional, returned whenoutput_attentions=True
is passed or whenconfig.output_attentions=True
) — Tuple oftorch.FloatTensor
(one for each layer) of shape(batch_size, num_heads, sequence_length, sequence_length)
.Attentions weights after the attention softmax, used to compute the weighted average in the self-attention heads.
The Blip2VisionModel forward method, overrides the __call__
special method.
Although the recipe for forward pass needs to be defined within this function, one should call the Module
instance afterwards instead of this since the former takes care of running the pre and post processing steps while
the latter silently ignores them.
Blip2QFormerModel
Querying Transformer (Q-Former), used in BLIP-2.
forward
< source >( query_embeds: FloatTensor attention_mask: Optional = None head_mask: Optional = None encoder_hidden_states: Optional = None encoder_attention_mask: Optional = None past_key_values: Optional = None use_cache: Optional = None output_attentions: Optional = None output_hidden_states: Optional = None return_dict: Optional = None )
encoder_hidden_states (torch.FloatTensor
of shape (batch_size, sequence_length, hidden_size)
, optional
):
Sequence of hidden-states at the output of the last layer of the encoder. Used in the cross-attention if
the model is configured as a decoder.
encoder_attention_mask (torch.FloatTensor
of shape (batch_size, sequence_length)
, optional
):
Mask to avoid performing attention on the padding token indices of the encoder input. This mask is used in
the cross-attention if the model is configured as a decoder. Mask values selected in [0, 1]
:
- 1 for tokens that are not masked,
- 0 for tokens that are masked.
past_key_values (
tuple(tuple(torch.FloatTensor))
of lengthconfig.n_layers
with each tuple having 4 tensors of: shape(batch_size, num_heads, sequence_length - 1, embed_size_per_head)
): Contains precomputed key and value hidden states of the attention blocks. Can be used to speed up decoding. Ifpast_key_values
are used, the user can optionally input only the lastdecoder_input_ids
(those that don’t have their past key value states given to this model) of shape(batch_size, 1)
instead of alldecoder_input_ids
of shape(batch_size, sequence_length)
. use_cache (bool
,optional
): If set toTrue
,past_key_values
key value states are returned and can be used to speed up decoding (seepast_key_values
).
Blip2Model
class transformers.Blip2Model
< source >( config: Blip2Config )
Parameters
- config (Blip2Config) — Model configuration class with all the parameters of the model. Initializing with a config file does not load the weights associated with the model, only the configuration. Check out the from_pretrained() method to load the model weights.
BLIP-2 Model for generating text and image features. The model consists of a vision encoder, Querying Transformer (Q-Former) and a language model.
This model inherits from PreTrainedModel. Check the superclass documentation for the generic methods the library implements for all its model (such as downloading or saving, resizing the input embeddings, pruning heads etc.)
This model is also a PyTorch torch.nn.Module subclass. Use it as a regular PyTorch Module and refer to the PyTorch documentation for all matter related to general usage and behavior.
forward
< source >( pixel_values: FloatTensor input_ids: FloatTensor attention_mask: Optional = None decoder_input_ids: Optional = None decoder_attention_mask: Optional = None output_attentions: Optional = None output_hidden_states: Optional = None labels: Optional = None return_dict: Optional = None ) → transformers.models.blip_2.modeling_blip_2.Blip2ForConditionalGenerationModelOutput
or tuple(torch.FloatTensor)
Parameters
- pixel_values (
torch.FloatTensor
of shape(batch_size, num_channels, height, width)
) — Pixel values. Pixel values can be obtained using Blip2Processor. SeeBlip2Processor.__call__()
for details. - input_ids (
torch.LongTensor
of shape(batch_size, sequence_length)
, optional) — Indices of input sequence tokens in the vocabulary of the language model. Input tokens can optionally be provided to serve as text prompt, which the language model can continue.Indices can be obtained using Blip2Processor. See
Blip2Processor.__call__()
for details. - attention_mask (
torch.Tensor
of shape(batch_size, sequence_length)
, optional) — Mask to avoid performing attention on padding token indices. Mask values selected in[0, 1]
:- 1 for tokens that are not masked,
- 0 for tokens that are masked.
- decoder_input_ids (
torch.LongTensor
of shape(batch_size, target_sequence_length)
, optional) — Indices of decoder input sequence tokens in the vocabulary of the language model. Only relevant in case an encoder-decoder language model (like T5) is used.Indices can be obtained using AutoTokenizer. See PreTrainedTokenizer.encode() and PreTrainedTokenizer.call() for details. What are decoder input IDs?
- decoder_attention_mask (
torch.BoolTensor
of shape(batch_size, target_sequence_length)
, optional) — Default behavior: generate a tensor that ignores pad tokens indecoder_input_ids
. Causal mask will also be used by default.Only relevant in case an encoder-decoder language model (like T5) is used.
- output_attentions (
bool
, optional) — Whether or not to return the attentions tensors of all attention layers. Seeattentions
under returned tensors for more detail. - output_hidden_states (
bool
, optional) — Whether or not to return the hidden states of all layers. Seehidden_states
under returned tensors for more detail. - return_dict (
bool
, optional) — Whether or not to return a ModelOutput instead of a plain tuple.
Returns
transformers.models.blip_2.modeling_blip_2.Blip2ForConditionalGenerationModelOutput
or tuple(torch.FloatTensor)
A transformers.models.blip_2.modeling_blip_2.Blip2ForConditionalGenerationModelOutput
or a tuple of
torch.FloatTensor
(if return_dict=False
is passed or when config.return_dict=False
) comprising various
elements depending on the configuration (<class 'transformers.models.blip_2.configuration_blip_2.Blip2VisionConfig'>
) and inputs.
- loss (
torch.FloatTensor
, optional, returned whenlabels
is provided,torch.FloatTensor
of shape(1,)
) — Language modeling loss from the language model. - logits (
torch.FloatTensor
of shape(batch_size, sequence_length, config.vocab_size)
) — Prediction scores of the language modeling head of the language model. - vision_outputs (
BaseModelOutputWithPooling
) — Outputs of the vision encoder. - qformer_outputs (
BaseModelOutputWithPoolingAndCrossAttentions
) — Outputs of the Q-Former (Querying Transformer). - language_model_outputs (
CausalLMOutputWithPast
orSeq2SeqLMOutput
) — Outputs of the language model.
The Blip2Model forward method, overrides the __call__
special method.
Although the recipe for forward pass needs to be defined within this function, one should call the Module
instance afterwards instead of this since the former takes care of running the pre and post processing steps while
the latter silently ignores them.
Examples:
>>> from PIL import Image
>>> import requests
>>> from transformers import Blip2Processor, Blip2Model
>>> import torch
>>> device = "cuda" if torch.cuda.is_available() else "cpu"
>>> processor = Blip2Processor.from_pretrained("Salesforce/blip2-opt-2.7b")
>>> model = Blip2Model.from_pretrained("Salesforce/blip2-opt-2.7b", torch_dtype=torch.float16)
>>> model.to(device)
>>> url = "http://images.cocodataset.org/val2017/000000039769.jpg"
>>> image = Image.open(requests.get(url, stream=True).raw)
>>> prompt = "Question: how many cats are there? Answer:"
>>> inputs = processor(images=image, text=prompt, return_tensors="pt").to(device, torch.float16)
>>> outputs = model(**inputs)
get_text_features
< source >( input_ids: Optional = None attention_mask: Optional = None decoder_input_ids: Optional = None decoder_attention_mask: Optional = None labels: Optional = None output_attentions: Optional = None output_hidden_states: Optional = None return_dict: Optional = None ) → text_outputs (CausalLMOutputWithPast
, or tuple(torch.FloatTensor)
if return_dict=False
)
Parameters
- input_ids (
torch.LongTensor
of shape(batch_size, sequence_length)
) — Indices of input sequence tokens in the vocabulary. Padding will be ignored by default should you provide it. Indices can be obtained using AutoTokenizer. See PreTrainedTokenizer.encode() and PreTrainedTokenizer.call() for details. What are input IDs? - attention_mask (
torch.Tensor
of shape(batch_size, sequence_length)
, optional) — Mask to avoid performing attention on padding token indices. Mask values selected in[0, 1]
:- 1 for tokens that are not masked,
- 0 for tokens that are masked. What are attention masks?
- decoder_input_ids (
torch.LongTensor
of shape(batch_size, target_sequence_length)
, optional) — Indices of decoder input sequence tokens in the vocabulary.Indices can be obtained using AutoTokenizer. See PreTrainedTokenizer.encode() and PreTrainedTokenizer.call() for details.
T5 uses the
pad_token_id
as the starting token fordecoder_input_ids
generation. Ifpast_key_values
is used, optionally only the lastdecoder_input_ids
have to be input (seepast_key_values
).To know more on how to prepare
decoder_input_ids
for pretraining take a look at T5 Training. - decoder_attention_mask (
torch.BoolTensor
of shape(batch_size, target_sequence_length)
, optional) — Default behavior: generate a tensor that ignores pad tokens indecoder_input_ids
. Causal mask will also be used by default. - output_attentions (
bool
, optional) — Whether or not to return the attentions tensors of all attention layers. Seeattentions
under returned tensors for more detail. - output_hidden_states (
bool
, optional) — Whether or not to return the hidden states of all layers. Seehidden_states
under returned tensors for more detail. - return_dict (
bool
, optional) — Whether or not to return a ModelOutput instead of a plain tuple.
Returns
text_outputs (CausalLMOutputWithPast
, or tuple(torch.FloatTensor)
if return_dict=False
)
The language model outputs. If return_dict=True
, the output is a CausalLMOutputWithPast
that
contains the language model logits, the past key values and the hidden states if
output_hidden_states=True
.
The Blip2Model forward method, overrides the __call__
special method.
Although the recipe for forward pass needs to be defined within this function, one should call the Module
instance afterwards instead of this since the former takes care of running the pre and post processing steps while
the latter silently ignores them.
Examples:
>>> import torch
>>> from transformers import AutoTokenizer, Blip2Model
>>> model = Blip2Model.from_pretrained("Salesforce/blip2-opt-2.7b")
>>> tokenizer = AutoTokenizer.from_pretrained("Salesforce/blip2-opt-2.7b")
>>> inputs = tokenizer(["a photo of a cat"], padding=True, return_tensors="pt")
>>> text_features = model.get_text_features(**inputs)
get_image_features
< source >( pixel_values: Optional = None output_attentions: Optional = None output_hidden_states: Optional = None return_dict: Optional = None ) → vision_outputs (BaseModelOutputWithPooling
or tuple of torch.FloatTensor
)
Parameters
- pixel_values (
torch.FloatTensor
of shape(batch_size, num_channels, height, width)
) — Pixel values. Pixel values can be obtained using Blip2Processor. SeeBlip2Processor.__call__()
for details. - output_attentions (
bool
, optional) — Whether or not to return the attentions tensors of all attention layers. Seeattentions
under returned tensors for more detail. - output_hidden_states (
bool
, optional) — Whether or not to return the hidden states of all layers. Seehidden_states
under returned tensors for more detail. - return_dict (
bool
, optional) — Whether or not to return a ModelOutput instead of a plain tuple.
Returns
vision_outputs (BaseModelOutputWithPooling
or tuple of torch.FloatTensor
)
The vision model outputs. If return_dict=True
, the output is a BaseModelOutputWithPooling
that
contains the image features, the pooled image features and the hidden states if
output_hidden_states=True
.
The Blip2Model forward method, overrides the __call__
special method.
Although the recipe for forward pass needs to be defined within this function, one should call the Module
instance afterwards instead of this since the former takes care of running the pre and post processing steps while
the latter silently ignores them.
Examples:
>>> import torch
>>> from PIL import Image
>>> import requests
>>> from transformers import AutoProcessor, Blip2Model
>>> model = Blip2Model.from_pretrained("Salesforce/blip2-opt-2.7b")
>>> processor = AutoProcessor.from_pretrained("Salesforce/blip2-opt-2.7b")
>>> url = "http://images.cocodataset.org/val2017/000000039769.jpg"
>>> image = Image.open(requests.get(url, stream=True).raw)
>>> inputs = processor(images=image, return_tensors="pt")
>>> image_outputs = model.get_image_features(**inputs)
get_qformer_features
< source >( pixel_values: Optional = None output_attentions: Optional = None output_hidden_states: Optional = None return_dict: Optional = None ) → vision_outputs (BaseModelOutputWithPooling
or tuple of torch.FloatTensor
)
Parameters
- pixel_values (
torch.FloatTensor
of shape(batch_size, num_channels, height, width)
) — Pixel values. Pixel values can be obtained using Blip2Processor. SeeBlip2Processor.__call__()
for details. - input_ids (
torch.LongTensor
of shape(batch_size, sequence_length)
, optional) — Indices of input sequence tokens in the vocabulary of the language model. Input tokens can optionally be provided to serve as text prompt, which the language model can continue.Indices can be obtained using Blip2Processor. See
Blip2Processor.__call__()
for details. - attention_mask (
torch.Tensor
of shape(batch_size, sequence_length)
, optional) — Mask to avoid performing attention on padding token indices. Mask values selected in[0, 1]
:- 1 for tokens that are not masked,
- 0 for tokens that are masked.
- decoder_input_ids (
torch.LongTensor
of shape(batch_size, target_sequence_length)
, optional) — Indices of decoder input sequence tokens in the vocabulary of the language model. Only relevant in case an encoder-decoder language model (like T5) is used.Indices can be obtained using AutoTokenizer. See PreTrainedTokenizer.encode() and PreTrainedTokenizer.call() for details. What are decoder input IDs?
- decoder_attention_mask (
torch.BoolTensor
of shape(batch_size, target_sequence_length)
, optional) — Default behavior: generate a tensor that ignores pad tokens indecoder_input_ids
. Causal mask will also be used by default.Only relevant in case an encoder-decoder language model (like T5) is used.
- output_attentions (
bool
, optional) — Whether or not to return the attentions tensors of all attention layers. Seeattentions
under returned tensors for more detail. - output_hidden_states (
bool
, optional) — Whether or not to return the hidden states of all layers. Seehidden_states
under returned tensors for more detail. - return_dict (
bool
, optional) — Whether or not to return a ModelOutput instead of a plain tuple.
Returns
vision_outputs (BaseModelOutputWithPooling
or tuple of torch.FloatTensor
)
The vision model outputs. If return_dict=True
, the output is a BaseModelOutputWithPooling
that
contains the image features, the pooled image features and the hidden states if
output_hidden_states=True
.
The Blip2Model forward method, overrides the __call__
special method.
Although the recipe for forward pass needs to be defined within this function, one should call the Module
instance afterwards instead of this since the former takes care of running the pre and post processing steps while
the latter silently ignores them.
Examples:
>>> import torch
>>> from PIL import Image
>>> import requests
>>> from transformers import Blip2Processor, Blip2Model
>>> processor = Blip2Processor.from_pretrained("Salesforce/blip2-opt-2.7b")
>>> model = Blip2Model.from_pretrained("Salesforce/blip2-opt-2.7b")
>>> url = "http://images.cocodataset.org/val2017/000000039769.jpg"
>>> image = Image.open(requests.get(url, stream=True).raw)
>>> inputs = processor(images=image, return_tensors="pt")
>>> qformer_outputs = model.get_qformer_features(**inputs)
Blip2ForConditionalGeneration
class transformers.Blip2ForConditionalGeneration
< source >( config: Blip2Config )
Parameters
- config (Blip2Config) — Model configuration class with all the parameters of the model. Initializing with a config file does not load the weights associated with the model, only the configuration. Check out the from_pretrained() method to load the model weights.
BLIP-2 Model for generating text given an image and an optional text prompt. The model consists of a vision encoder, Querying Transformer (Q-Former) and a language model.
One can optionally pass input_ids
to the model, which serve as a text prompt, to make the language model continue
the prompt. Otherwise, the language model starts generating text from the [BOS] (beginning-of-sequence) token.
Note that Flan-T5 checkpoints cannot be cast to float16. They are pre-trained using bfloat16.
This model inherits from PreTrainedModel. Check the superclass documentation for the generic methods the library implements for all its model (such as downloading or saving, resizing the input embeddings, pruning heads etc.)
This model is also a PyTorch torch.nn.Module subclass. Use it as a regular PyTorch Module and refer to the PyTorch documentation for all matter related to general usage and behavior.
forward
< source >( pixel_values: FloatTensor input_ids: FloatTensor attention_mask: Optional = None decoder_input_ids: Optional = None decoder_attention_mask: Optional = None output_attentions: Optional = None output_hidden_states: Optional = None labels: Optional = None return_dict: Optional = None ) → transformers.models.blip_2.modeling_blip_2.Blip2ForConditionalGenerationModelOutput
or tuple(torch.FloatTensor)
Parameters
- pixel_values (
torch.FloatTensor
of shape(batch_size, num_channels, height, width)
) — Pixel values. Pixel values can be obtained using Blip2Processor. SeeBlip2Processor.__call__()
for details. - input_ids (
torch.LongTensor
of shape(batch_size, sequence_length)
, optional) — Indices of input sequence tokens in the vocabulary of the language model. Input tokens can optionally be provided to serve as text prompt, which the language model can continue.Indices can be obtained using Blip2Processor. See
Blip2Processor.__call__()
for details. - attention_mask (
torch.Tensor
of shape(batch_size, sequence_length)
, optional) — Mask to avoid performing attention on padding token indices. Mask values selected in[0, 1]
:- 1 for tokens that are not masked,
- 0 for tokens that are masked.
- decoder_input_ids (
torch.LongTensor
of shape(batch_size, target_sequence_length)
, optional) — Indices of decoder input sequence tokens in the vocabulary of the language model. Only relevant in case an encoder-decoder language model (like T5) is used.Indices can be obtained using AutoTokenizer. See PreTrainedTokenizer.encode() and PreTrainedTokenizer.call() for details. What are decoder input IDs?
- decoder_attention_mask (
torch.BoolTensor
of shape(batch_size, target_sequence_length)
, optional) — Default behavior: generate a tensor that ignores pad tokens indecoder_input_ids
. Causal mask will also be used by default.Only relevant in case an encoder-decoder language model (like T5) is used.
- output_attentions (
bool
, optional) — Whether or not to return the attentions tensors of all attention layers. Seeattentions
under returned tensors for more detail. - output_hidden_states (
bool
, optional) — Whether or not to return the hidden states of all layers. Seehidden_states
under returned tensors for more detail. - return_dict (
bool
, optional) — Whether or not to return a ModelOutput instead of a plain tuple.
Returns
transformers.models.blip_2.modeling_blip_2.Blip2ForConditionalGenerationModelOutput
or tuple(torch.FloatTensor)
A transformers.models.blip_2.modeling_blip_2.Blip2ForConditionalGenerationModelOutput
or a tuple of
torch.FloatTensor
(if return_dict=False
is passed or when config.return_dict=False
) comprising various
elements depending on the configuration (<class 'transformers.models.blip_2.configuration_blip_2.Blip2VisionConfig'>
) and inputs.
- loss (
torch.FloatTensor
, optional, returned whenlabels
is provided,torch.FloatTensor
of shape(1,)
) — Language modeling loss from the language model. - logits (
torch.FloatTensor
of shape(batch_size, sequence_length, config.vocab_size)
) — Prediction scores of the language modeling head of the language model. - vision_outputs (
BaseModelOutputWithPooling
) — Outputs of the vision encoder. - qformer_outputs (
BaseModelOutputWithPoolingAndCrossAttentions
) — Outputs of the Q-Former (Querying Transformer). - language_model_outputs (
CausalLMOutputWithPast
orSeq2SeqLMOutput
) — Outputs of the language model.
The Blip2ForConditionalGeneration forward method, overrides the __call__
special method.
Although the recipe for forward pass needs to be defined within this function, one should call the Module
instance afterwards instead of this since the former takes care of running the pre and post processing steps while
the latter silently ignores them.
Examples:
Prepare processor, model and image input
>>> from PIL import Image
>>> import requests
>>> from transformers import Blip2Processor, Blip2ForConditionalGeneration
>>> import torch
>>> device = "cuda" if torch.cuda.is_available() else "cpu"
>>> processor = Blip2Processor.from_pretrained("Salesforce/blip2-opt-2.7b")
>>> model = Blip2ForConditionalGeneration.from_pretrained(
... "Salesforce/blip2-opt-2.7b", load_in_8bit=True, device_map={"": 0}, torch_dtype=torch.float16
... ) # doctest: +IGNORE_RESULT
>>> url = "http://images.cocodataset.org/val2017/000000039769.jpg"
>>> image = Image.open(requests.get(url, stream=True).raw)
Image captioning (without providing a text prompt):
>>> inputs = processor(images=image, return_tensors="pt").to(device, torch.float16)
>>> generated_ids = model.generate(**inputs)
>>> generated_text = processor.batch_decode(generated_ids, skip_special_tokens=True)[0].strip()
>>> print(generated_text)
two cats laying on a couch
Visual question answering (prompt = question):
>>> prompt = "Question: how many cats are there? Answer:"
>>> inputs = processor(images=image, text=prompt, return_tensors="pt").to(device="cuda", dtype=torch.float16)
>>> generated_ids = model.generate(**inputs)
>>> generated_text = processor.batch_decode(generated_ids, skip_special_tokens=True)[0].strip()
>>> print(generated_text)
two
Note that int8 inference is also supported through bitsandbytes. This greatly reduces the amount of memory used by the model while maintaining the same performance.
>>> model = Blip2ForConditionalGeneration.from_pretrained(
... "Salesforce/blip2-opt-2.7b", load_in_8bit=True, device_map={"": 0}, torch_dtype=torch.bfloat16
... ) # doctest: +IGNORE_RESULT
>>> inputs = processor(images=image, text=prompt, return_tensors="pt").to(device="cuda", dtype=torch.bfloat16)
>>> generated_ids = model.generate(**inputs)
>>> generated_text = processor.batch_decode(generated_ids, skip_special_tokens=True)[0].strip()
>>> print(generated_text)
two
generate
< source >( pixel_values: FloatTensor input_ids: Optional = None attention_mask: Optional = None **generate_kwargs ) → captions (list)
Parameters
- pixel_values (
torch.FloatTensor
of shape (batch_size, num_channels, height, width)) — Input images to be processed. - input_ids (
torch.LongTensor
of shape (batch_size, sequence_length), optional) — The sequence used as a prompt for the generation. - attention_mask (
torch.LongTensor
of shape (batch_size, sequence_length), optional) — Mask to avoid performing attention on padding token indices
Returns
captions (list)
A list of strings of length batch_size * num_captions.
Overrides generate
function to be able to use the model as a conditional generator.