roberta-base-squad2 for QA on COVID-19
Overview
Language model: deepset/roberta-base-squad2
Language: English
Downstream-task: Extractive QA
Training data: SQuAD-style CORD-19 annotations from 23rd April
Code: See an example QA pipeline on Haystack
Infrastructure: Tesla v100
Hyperparameters
batch_size = 24
n_epochs = 3
base_LM_model = "deepset/roberta-base-squad2"
max_seq_len = 384
learning_rate = 3e-5
lr_schedule = LinearWarmup
warmup_proportion = 0.1
doc_stride = 128
xval_folds = 5
dev_split = 0
no_ans_boost = -100
license: cc-by-4.0
Performance
5-fold cross-validation on the data set led to the following results:
Single EM-Scores: [0.222, 0.123, 0.234, 0.159, 0.158]
Single F1-Scores: [0.476, 0.493, 0.599, 0.461, 0.465]
Single top\_3\_recall Scores: [0.827, 0.776, 0.860, 0.771, 0.777]
XVAL EM: 0.17890995260663506
XVAL f1: 0.49925444207319924
XVAL top\_3\_recall: 0.8021327014218009
This model is the model obtained from the third fold of the cross-validation.
Usage
In Haystack
For doing QA at scale (i.e. many docs instead of single paragraph), you can load the model also in haystack:
reader = FARMReader(model_name_or_path="deepset/roberta-base-squad2-covid")
# or
reader = TransformersReader(model="deepset/roberta-base-squad2",tokenizer="deepset/roberta-base-squad2-covid")
In Transformers
from transformers import AutoModelForQuestionAnswering, AutoTokenizer, pipeline
model_name = "deepset/roberta-base-squad2-covid"
# a) Get predictions
nlp = pipeline('question-answering', model=model_name, tokenizer=model_name)
QA_input = {
'question': 'Why is model conversion important?',
'context': 'The option to convert models between FARM and transformers gives freedom to the user and let people easily switch between frameworks.'
}
res = nlp(QA_input)
# b) Load model & tokenizer
model = AutoModelForQuestionAnswering.from_pretrained(model_name)
tokenizer = AutoTokenizer.from_pretrained(model_name)
Authors
Branden Chan: branden.chan@deepset.ai
Timo Möller: timo.moeller@deepset.ai
Malte Pietsch: malte.pietsch@deepset.ai
Tanay Soni: tanay.soni@deepset.ai
Bogdan Kostić: bogdan.kostic@deepset.ai
About us
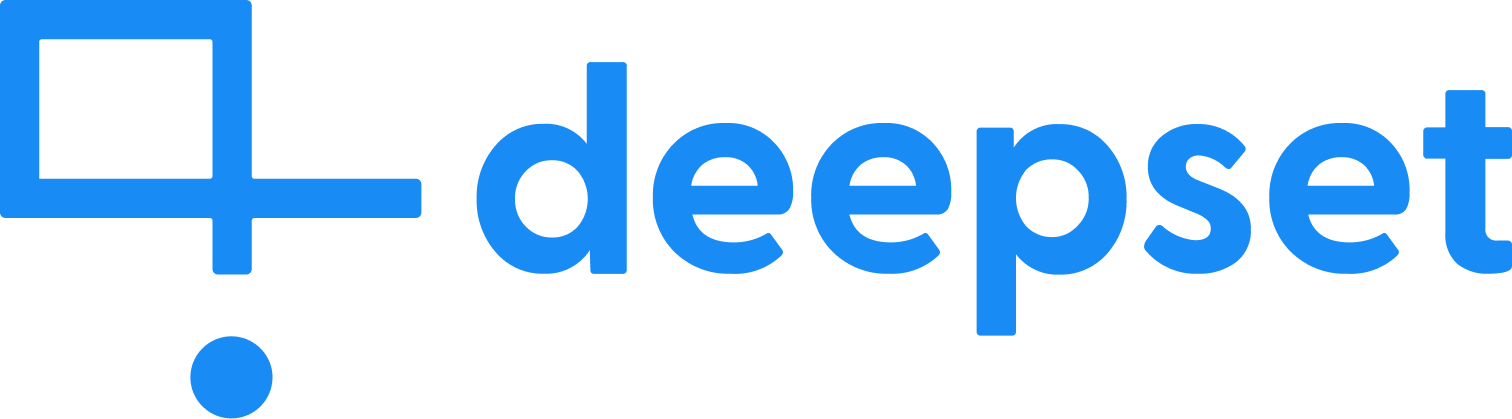
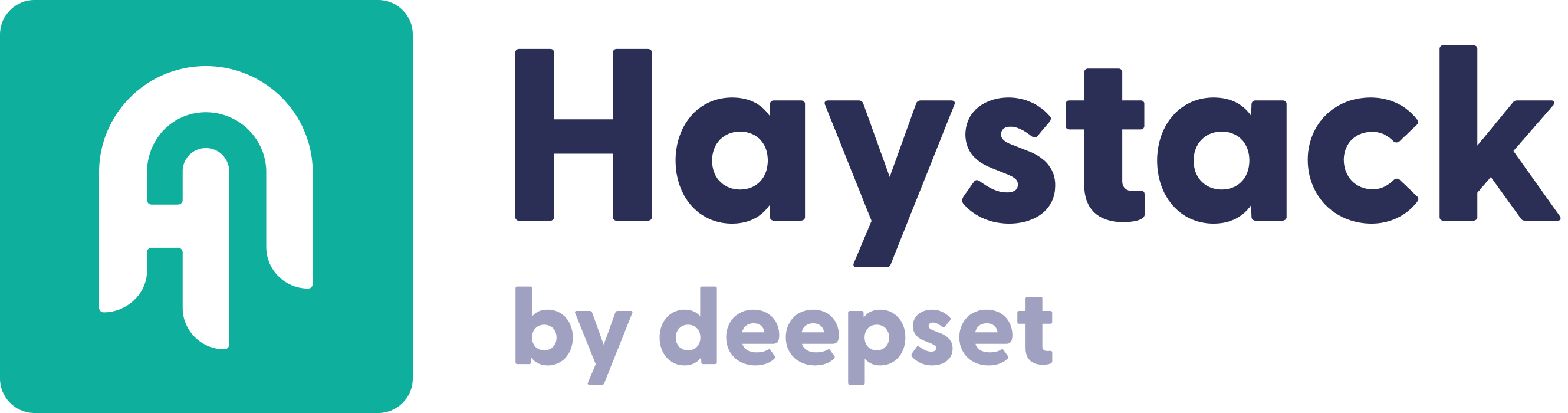
deepset is the company behind the open-source NLP framework Haystack which is designed to help you build production ready NLP systems that use: Question answering, summarization, ranking etc.
Some of our other work:
- Distilled roberta-base-squad2 (aka "tinyroberta-squad2")
- German BERT (aka "bert-base-german-cased")
- GermanQuAD and GermanDPR datasets and models (aka "gelectra-base-germanquad", "gbert-base-germandpr")
Get in touch and join the Haystack community
For more info on Haystack, visit our GitHub repo and Documentation.
We also have a Discord community open to everyone!
Twitter | LinkedIn | Discord | GitHub Discussions | Website
By the way: we're hiring!
- Downloads last month
- 70