File size: 40,503 Bytes
3df17b8 |
1 2 3 4 5 6 7 8 9 10 11 12 13 14 15 16 17 18 19 20 21 22 23 24 25 26 27 28 29 30 31 32 33 34 35 36 37 38 39 40 41 42 43 44 45 46 47 48 49 50 51 52 53 54 55 56 57 58 59 60 61 62 63 64 65 66 67 68 69 70 71 72 73 74 75 76 77 78 79 80 81 82 83 84 85 86 87 88 89 90 91 92 93 94 95 96 97 98 99 100 101 102 103 104 105 106 107 108 109 110 111 112 113 114 115 116 117 118 119 120 121 122 123 124 125 126 127 128 129 130 131 132 133 134 135 136 137 138 139 140 141 142 143 144 145 146 147 148 149 150 151 152 153 154 155 156 157 158 159 160 161 162 163 164 165 166 167 168 169 170 171 172 173 174 175 176 177 178 179 180 181 182 183 184 185 186 187 188 189 190 191 192 193 194 195 196 197 198 199 200 201 202 203 204 205 206 207 208 209 210 211 212 213 214 215 216 217 218 219 220 221 222 223 224 225 226 227 228 229 230 231 232 233 234 235 236 237 238 239 240 241 242 243 244 245 246 247 248 249 250 251 252 253 254 255 256 257 258 259 260 261 262 263 264 265 266 267 268 269 270 271 272 273 274 275 276 277 278 279 280 281 282 283 284 285 286 287 288 289 290 291 292 293 294 295 296 297 298 299 300 301 302 303 304 305 306 307 308 309 310 311 312 313 314 315 316 317 318 319 320 321 322 323 324 325 326 327 328 329 330 331 332 333 334 335 336 337 338 339 340 341 342 343 344 345 346 347 348 349 350 351 352 353 354 355 356 357 358 359 360 361 362 363 364 365 366 367 368 369 370 371 372 373 374 375 376 377 378 379 380 381 382 383 384 385 386 387 388 389 390 391 392 393 394 395 396 397 398 399 400 401 402 403 404 405 406 407 408 409 410 411 412 413 414 415 416 417 418 419 420 421 422 423 424 425 426 427 428 429 430 431 432 433 434 435 436 437 438 439 440 441 442 443 444 445 446 447 448 449 450 451 452 453 454 455 456 457 458 459 460 461 462 463 464 465 466 467 468 469 470 471 472 473 474 475 476 477 478 479 480 481 482 483 484 485 486 487 488 489 490 491 492 493 494 495 496 497 498 499 500 501 502 503 504 505 506 507 508 509 510 511 512 513 514 515 516 517 518 519 520 521 522 523 524 525 526 527 528 529 530 531 532 533 534 535 536 537 538 539 540 541 542 543 544 545 546 547 548 549 550 551 552 553 554 555 556 557 558 559 560 561 562 563 564 565 566 567 568 569 570 571 572 573 574 575 576 577 578 579 580 581 582 583 584 585 586 587 588 589 590 591 592 593 594 595 596 597 598 599 600 601 602 603 604 605 606 607 608 609 610 611 612 613 614 615 616 617 618 619 620 621 622 623 624 625 626 627 628 629 630 631 632 633 634 635 636 637 638 639 640 641 642 643 644 645 646 647 648 649 650 651 652 653 654 655 656 657 658 659 660 661 662 663 664 665 666 667 668 669 670 671 672 673 674 675 676 677 678 679 680 681 682 683 684 685 686 687 688 689 690 691 692 693 694 695 696 697 698 699 700 701 702 703 704 705 706 707 708 709 710 711 712 713 714 715 716 717 718 719 720 721 722 723 724 725 726 727 728 729 730 731 732 733 734 735 736 737 738 739 740 741 742 743 744 745 746 747 748 749 750 751 752 753 754 755 756 757 758 759 760 761 762 763 764 765 766 767 768 769 770 771 772 773 774 775 776 777 |
# Community Examples
> **For more information about community pipelines, please have a look at [this issue](https://github.com/huggingface/diffusers/issues/841).**
**Community** examples consist of both inference and training examples that have been added by the community.
Please have a look at the following table to get an overview of all community examples. Click on the **Code Example** to get a copy-and-paste ready code example that you can try out.
If a community doesn't work as expected, please open an issue and ping the author on it.
| Example | Description | Code Example | Colab | Author |
|:---------------------------------------|:---------------------------------------------------------------------------------------------------------------------------------------------------------------------------------------------------------------------------------------------------------------------------------------------------------------------------------------------------------------------------------------------------------------------------------------------------------------------------------------------------------|:------------------------------------------------------------------|:-------------------------------------------------------------------------------------------------------------------------------------------------------------------------------------------------------------------|-----------------------------------------------------------:|
| CLIP Guided Stable Diffusion | Doing CLIP guidance for text to image generation with Stable Diffusion | [CLIP Guided Stable Diffusion](#clip-guided-stable-diffusion) | [](https://colab.research.google.com/github/huggingface/notebooks/blob/main/diffusers/CLIP_Guided_Stable_diffusion_with_diffusers.ipynb) | [Suraj Patil](https://github.com/patil-suraj/) |
| One Step U-Net (Dummy) | Example showcasing of how to use Community Pipelines (see https://github.com/huggingface/diffusers/issues/841) | [One Step U-Net](#one-step-unet) | - | [Patrick von Platen](https://github.com/patrickvonplaten/) |
| Stable Diffusion Interpolation | Interpolate the latent space of Stable Diffusion between different prompts/seeds | [Stable Diffusion Interpolation](#stable-diffusion-interpolation) | - | [Nate Raw](https://github.com/nateraw/) |
| Stable Diffusion Mega | **One** Stable Diffusion Pipeline with all functionalities of [Text2Image](https://github.com/huggingface/diffusers/blob/main/src/diffusers/pipelines/stable_diffusion/pipeline_stable_diffusion.py), [Image2Image](https://github.com/huggingface/diffusers/blob/main/src/diffusers/pipelines/stable_diffusion/pipeline_stable_diffusion_img2img.py) and [Inpainting](https://github.com/huggingface/diffusers/blob/main/src/diffusers/pipelines/stable_diffusion/pipeline_stable_diffusion_inpaint.py) | [Stable Diffusion Mega](#stable-diffusion-mega) | - | [Patrick von Platen](https://github.com/patrickvonplaten/) |
| Long Prompt Weighting Stable Diffusion | **One** Stable Diffusion Pipeline without tokens length limit, and support parsing weighting in prompt. | [Long Prompt Weighting Stable Diffusion](#long-prompt-weighting-stable-diffusion) | - | [SkyTNT](https://github.com/SkyTNT) |
| Speech to Image | Using automatic-speech-recognition to transcribe text and Stable Diffusion to generate images | [Speech to Image](#speech-to-image) | - | [Mikail Duzenli](https://github.com/MikailINTech)
| Wild Card Stable Diffusion | Stable Diffusion Pipeline that supports prompts that contain wildcard terms (indicated by surrounding double underscores), with values instantiated randomly from a corresponding txt file or a dictionary of possible values | [Wildcard Stable Diffusion](#wildcard-stable-diffusion) | - | [Shyam Sudhakaran](https://github.com/shyamsn97) |
| [Composable Stable Diffusion](https://energy-based-model.github.io/Compositional-Visual-Generation-with-Composable-Diffusion-Models/) | Stable Diffusion Pipeline that supports prompts that contain "|" in prompts (as an AND condition) and weights (separated by "|" as well) to positively / negatively weight prompts. | [Composable Stable Diffusion](#composable-stable-diffusion) | - | [Mark Rich](https://github.com/MarkRich) |
| Seed Resizing Stable Diffusion| Stable Diffusion Pipeline that supports resizing an image and retaining the concepts of the 512 by 512 generation. | [Seed Resizing](#seed-resizing) | - | [Mark Rich](https://github.com/MarkRich) |
| Imagic Stable Diffusion | Stable Diffusion Pipeline that enables writing a text prompt to edit an existing image| [Imagic Stable Diffusion](#imagic-stable-diffusion) | - | [Mark Rich](https://github.com/MarkRich) |
| Multilingual Stable Diffusion| Stable Diffusion Pipeline that supports prompts in 50 different languages. | [Multilingual Stable Diffusion](#multilingual-stable-diffusion-pipeline) | - | [Juan Carlos Piñeros](https://github.com/juancopi81) |
| Image to Image Inpainting Stable Diffusion | Stable Diffusion Pipeline that enables the overlaying of two images and subsequent inpainting| [Image to Image Inpainting Stable Diffusion](#image-to-image-inpainting-stable-diffusion) | - | [Alex McKinney](https://github.com/vvvm23) |
| Text Based Inpainting Stable Diffusion | Stable Diffusion Inpainting Pipeline that enables passing a text prompt to generate the mask for inpainting| [Text Based Inpainting Stable Diffusion](#image-to-image-inpainting-stable-diffusion) | - | [Dhruv Karan](https://github.com/unography) |
| Bit Diffusion | Diffusion on discrete data | [Bit Diffusion](#bit-diffusion) | - |[Stuti R.](https://github.com/kingstut) |
| K-Diffusion Stable Diffusion | Run Stable Diffusion with any of [K-Diffusion's samplers](https://github.com/crowsonkb/k-diffusion/blob/master/k_diffusion/sampling.py) | [Stable Diffusion with K Diffusion](#stable-diffusion-with-k-diffusion) | - | [Patrick von Platen](https://github.com/patrickvonplaten/) |
| Checkpoint Merger Pipeline | Diffusion Pipeline that enables merging of saved model checkpoints | [Checkpoint Merger Pipeline](#checkpoint-merger-pipeline) | - | [Naga Sai Abhinay Devarinti](https://github.com/Abhinay1997/) |
To load a custom pipeline you just need to pass the `custom_pipeline` argument to `DiffusionPipeline`, as one of the files in `diffusers/examples/community`. Feel free to send a PR with your own pipelines, we will merge them quickly.
```py
pipe = DiffusionPipeline.from_pretrained("runwayml/stable-diffusion-v1-5", custom_pipeline="filename_in_the_community_folder")
```
## Example usages
### CLIP Guided Stable Diffusion
CLIP guided stable diffusion can help to generate more realistic images
by guiding stable diffusion at every denoising step with an additional CLIP model.
The following code requires roughly 12GB of GPU RAM.
```python
from diffusers import DiffusionPipeline
from transformers import CLIPFeatureExtractor, CLIPModel
import torch
feature_extractor = CLIPFeatureExtractor.from_pretrained("laion/CLIP-ViT-B-32-laion2B-s34B-b79K")
clip_model = CLIPModel.from_pretrained("laion/CLIP-ViT-B-32-laion2B-s34B-b79K", torch_dtype=torch.float16)
guided_pipeline = DiffusionPipeline.from_pretrained(
"runwayml/stable-diffusion-v1-5",
custom_pipeline="clip_guided_stable_diffusion",
clip_model=clip_model,
feature_extractor=feature_extractor,
revision="fp16",
torch_dtype=torch.float16,
)
guided_pipeline.enable_attention_slicing()
guided_pipeline = guided_pipeline.to("cuda")
prompt = "fantasy book cover, full moon, fantasy forest landscape, golden vector elements, fantasy magic, dark light night, intricate, elegant, sharp focus, illustration, highly detailed, digital painting, concept art, matte, art by WLOP and Artgerm and Albert Bierstadt, masterpiece"
generator = torch.Generator(device="cuda").manual_seed(0)
images = []
for i in range(4):
image = guided_pipeline(
prompt,
num_inference_steps=50,
guidance_scale=7.5,
clip_guidance_scale=100,
num_cutouts=4,
use_cutouts=False,
generator=generator,
).images[0]
images.append(image)
# save images locally
for i, img in enumerate(images):
img.save(f"./clip_guided_sd/image_{i}.png")
```
The `images` list contains a list of PIL images that can be saved locally or displayed directly in a google colab.
Generated images tend to be of higher qualtiy than natively using stable diffusion. E.g. the above script generates the following images:
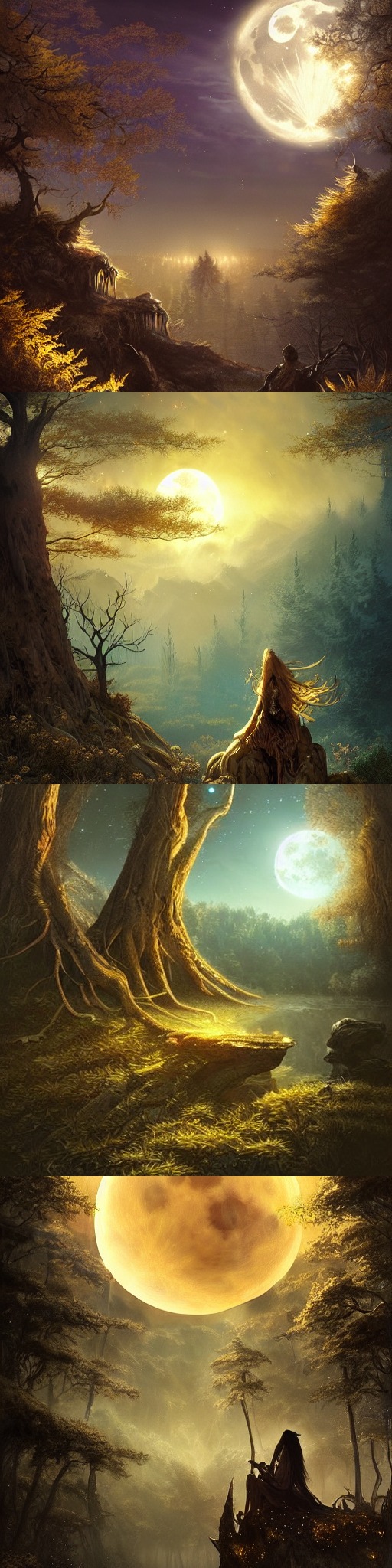.
### One Step Unet
The dummy "one-step-unet" can be run as follows:
```python
from diffusers import DiffusionPipeline
pipe = DiffusionPipeline.from_pretrained("google/ddpm-cifar10-32", custom_pipeline="one_step_unet")
pipe()
```
**Note**: This community pipeline is not useful as a feature, but rather just serves as an example of how community pipelines can be added (see https://github.com/huggingface/diffusers/issues/841).
### Stable Diffusion Interpolation
The following code can be run on a GPU of at least 8GB VRAM and should take approximately 5 minutes.
```python
from diffusers import DiffusionPipeline
import torch
pipe = DiffusionPipeline.from_pretrained(
"CompVis/stable-diffusion-v1-4",
revision='fp16',
torch_dtype=torch.float16,
safety_checker=None, # Very important for videos...lots of false positives while interpolating
custom_pipeline="interpolate_stable_diffusion",
).to('cuda')
pipe.enable_attention_slicing()
frame_filepaths = pipe.walk(
prompts=['a dog', 'a cat', 'a horse'],
seeds=[42, 1337, 1234],
num_interpolation_steps=16,
output_dir='./dreams',
batch_size=4,
height=512,
width=512,
guidance_scale=8.5,
num_inference_steps=50,
)
```
The output of the `walk(...)` function returns a list of images saved under the folder as defined in `output_dir`. You can use these images to create videos of stable diffusion.
> **Please have a look at https://github.com/nateraw/stable-diffusion-videos for more in-detail information on how to create videos using stable diffusion as well as more feature-complete functionality.**
### Stable Diffusion Mega
The Stable Diffusion Mega Pipeline lets you use the main use cases of the stable diffusion pipeline in a single class.
```python
#!/usr/bin/env python3
from diffusers import DiffusionPipeline
import PIL
import requests
from io import BytesIO
import torch
def download_image(url):
response = requests.get(url)
return PIL.Image.open(BytesIO(response.content)).convert("RGB")
pipe = DiffusionPipeline.from_pretrained("runwayml/stable-diffusion-v1-5", custom_pipeline="stable_diffusion_mega", torch_dtype=torch.float16, revision="fp16")
pipe.to("cuda")
pipe.enable_attention_slicing()
### Text-to-Image
images = pipe.text2img("An astronaut riding a horse").images
### Image-to-Image
init_image = download_image("https://raw.githubusercontent.com/CompVis/stable-diffusion/main/assets/stable-samples/img2img/sketch-mountains-input.jpg")
prompt = "A fantasy landscape, trending on artstation"
images = pipe.img2img(prompt=prompt, image=init_image, strength=0.75, guidance_scale=7.5).images
### Inpainting
img_url = "https://raw.githubusercontent.com/CompVis/latent-diffusion/main/data/inpainting_examples/overture-creations-5sI6fQgYIuo.png"
mask_url = "https://raw.githubusercontent.com/CompVis/latent-diffusion/main/data/inpainting_examples/overture-creations-5sI6fQgYIuo_mask.png"
init_image = download_image(img_url).resize((512, 512))
mask_image = download_image(mask_url).resize((512, 512))
prompt = "a cat sitting on a bench"
images = pipe.inpaint(prompt=prompt, image=init_image, mask_image=mask_image, strength=0.75).images
```
As shown above this one pipeline can run all both "text-to-image", "image-to-image", and "inpainting" in one pipeline.
### Long Prompt Weighting Stable Diffusion
Features of this custom pipeline:
- Input a prompt without the 77 token length limit.
- Includes tx2img, img2img. and inpainting pipelines.
- Emphasize/weigh part of your prompt with parentheses as so: `a baby deer with (big eyes)`
- De-emphasize part of your prompt as so: `a [baby] deer with big eyes`
- Precisely weigh part of your prompt as so: `a baby deer with (big eyes:1.3)`
Prompt weighting equivalents:
- `a baby deer with` == `(a baby deer with:1.0)`
- `(big eyes)` == `(big eyes:1.1)`
- `((big eyes))` == `(big eyes:1.21)`
- `[big eyes]` == `(big eyes:0.91)`
You can run this custom pipeline as so:
#### pytorch
```python
from diffusers import DiffusionPipeline
import torch
pipe = DiffusionPipeline.from_pretrained(
'hakurei/waifu-diffusion',
custom_pipeline="lpw_stable_diffusion",
revision="fp16",
torch_dtype=torch.float16
)
pipe=pipe.to("cuda")
prompt = "best_quality (1girl:1.3) bow bride brown_hair closed_mouth frilled_bow frilled_hair_tubes frills (full_body:1.3) fox_ear hair_bow hair_tubes happy hood japanese_clothes kimono long_sleeves red_bow smile solo tabi uchikake white_kimono wide_sleeves cherry_blossoms"
neg_prompt = "lowres, bad_anatomy, error_body, error_hair, error_arm, error_hands, bad_hands, error_fingers, bad_fingers, missing_fingers, error_legs, bad_legs, multiple_legs, missing_legs, error_lighting, error_shadow, error_reflection, text, error, extra_digit, fewer_digits, cropped, worst_quality, low_quality, normal_quality, jpeg_artifacts, signature, watermark, username, blurry"
pipe.text2img(prompt, negative_prompt=neg_prompt, width=512,height=512,max_embeddings_multiples=3).images[0]
```
#### onnxruntime
```python
from diffusers import DiffusionPipeline
import torch
pipe = DiffusionPipeline.from_pretrained(
'CompVis/stable-diffusion-v1-4',
custom_pipeline="lpw_stable_diffusion_onnx",
revision="onnx",
provider="CUDAExecutionProvider"
)
prompt = "a photo of an astronaut riding a horse on mars, best quality"
neg_prompt = "lowres, bad anatomy, error body, error hair, error arm, error hands, bad hands, error fingers, bad fingers, missing fingers, error legs, bad legs, multiple legs, missing legs, error lighting, error shadow, error reflection, text, error, extra digit, fewer digits, cropped, worst quality, low quality, normal quality, jpeg artifacts, signature, watermark, username, blurry"
pipe.text2img(prompt,negative_prompt=neg_prompt, width=512, height=512, max_embeddings_multiples=3).images[0]
```
if you see `Token indices sequence length is longer than the specified maximum sequence length for this model ( *** > 77 ) . Running this sequence through the model will result in indexing errors`. Do not worry, it is normal.
### Speech to Image
The following code can generate an image from an audio sample using pre-trained OpenAI whisper-small and Stable Diffusion.
```Python
import torch
import matplotlib.pyplot as plt
from datasets import load_dataset
from diffusers import DiffusionPipeline
from transformers import (
WhisperForConditionalGeneration,
WhisperProcessor,
)
device = "cuda" if torch.cuda.is_available() else "cpu"
ds = load_dataset("hf-internal-testing/librispeech_asr_dummy", "clean", split="validation")
audio_sample = ds[3]
text = audio_sample["text"].lower()
speech_data = audio_sample["audio"]["array"]
model = WhisperForConditionalGeneration.from_pretrained("openai/whisper-small").to(device)
processor = WhisperProcessor.from_pretrained("openai/whisper-small")
diffuser_pipeline = DiffusionPipeline.from_pretrained(
"CompVis/stable-diffusion-v1-4",
custom_pipeline="speech_to_image_diffusion",
speech_model=model,
speech_processor=processor,
revision="fp16",
torch_dtype=torch.float16,
)
diffuser_pipeline.enable_attention_slicing()
diffuser_pipeline = diffuser_pipeline.to(device)
output = diffuser_pipeline(speech_data)
plt.imshow(output.images[0])
```
This example produces the following image:
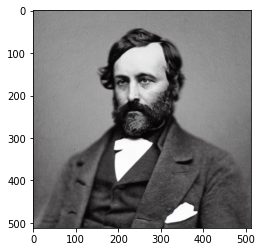
### Wildcard Stable Diffusion
Following the great examples from https://github.com/jtkelm2/stable-diffusion-webui-1/blob/master/scripts/wildcards.py and https://github.com/AUTOMATIC1111/stable-diffusion-webui/wiki/Custom-Scripts#wildcards, here's a minimal implementation that allows for users to add "wildcards", denoted by `__wildcard__` to prompts that are used as placeholders for randomly sampled values given by either a dictionary or a `.txt` file. For example:
Say we have a prompt:
```
prompt = "__animal__ sitting on a __object__ wearing a __clothing__"
```
We can then define possible values to be sampled for `animal`, `object`, and `clothing`. These can either be from a `.txt` with the same name as the category.
The possible values can also be defined / combined by using a dictionary like: `{"animal":["dog", "cat", mouse"]}`.
The actual pipeline works just like `StableDiffusionPipeline`, except the `__call__` method takes in:
`wildcard_files`: list of file paths for wild card replacement
`wildcard_option_dict`: dict with key as `wildcard` and values as a list of possible replacements
`num_prompt_samples`: number of prompts to sample, uniformly sampling wildcards
A full example:
create `animal.txt`, with contents like:
```
dog
cat
mouse
```
create `object.txt`, with contents like:
```
chair
sofa
bench
```
```python
from diffusers import DiffusionPipeline
import torch
pipe = DiffusionPipeline.from_pretrained(
"CompVis/stable-diffusion-v1-4",
custom_pipeline="wildcard_stable_diffusion",
revision="fp16",
torch_dtype=torch.float16,
)
prompt = "__animal__ sitting on a __object__ wearing a __clothing__"
out = pipe(
prompt,
wildcard_option_dict={
"clothing":["hat", "shirt", "scarf", "beret"]
},
wildcard_files=["object.txt", "animal.txt"],
num_prompt_samples=1
)
```
### Composable Stable diffusion
[Composable Stable Diffusion](https://energy-based-model.github.io/Compositional-Visual-Generation-with-Composable-Diffusion-Models/) proposes conjunction and negation (negative prompts) operators for compositional generation with conditional diffusion models.
```python
import torch as th
import numpy as np
import torchvision.utils as tvu
from diffusers import DiffusionPipeline
has_cuda = th.cuda.is_available()
device = th.device('cpu' if not has_cuda else 'cuda')
pipe = DiffusionPipeline.from_pretrained(
"CompVis/stable-diffusion-v1-4",
use_auth_token=True,
custom_pipeline="composable_stable_diffusion",
).to(device)
def dummy(images, **kwargs):
return images, False
pipe.safety_checker = dummy
images = []
generator = torch.Generator("cuda").manual_seed(0)
seed = 0
prompt = "a forest | a camel"
weights = " 1 | 1" # Equal weight to each prompt. Can be negative
images = []
for i in range(4):
res = pipe(
prompt,
guidance_scale=7.5,
num_inference_steps=50,
weights=weights,
generator=generator)
image = res.images[0]
images.append(image)
for i, img in enumerate(images):
img.save(f"./composable_diffusion/image_{i}.png")
```
### Imagic Stable Diffusion
Allows you to edit an image using stable diffusion.
```python
import requests
from PIL import Image
from io import BytesIO
import torch
import os
from diffusers import DiffusionPipeline, DDIMScheduler
has_cuda = torch.cuda.is_available()
device = torch.device('cpu' if not has_cuda else 'cuda')
pipe = DiffusionPipeline.from_pretrained(
"CompVis/stable-diffusion-v1-4",
safety_checker=None,
use_auth_token=True,
custom_pipeline="imagic_stable_diffusion",
scheduler = DDIMScheduler(beta_start=0.00085, beta_end=0.012, beta_schedule="scaled_linear", clip_sample=False, set_alpha_to_one=False)
).to(device)
generator = torch.Generator("cuda").manual_seed(0)
seed = 0
prompt = "A photo of Barack Obama smiling with a big grin"
url = 'https://www.dropbox.com/s/6tlwzr73jd1r9yk/obama.png?dl=1'
response = requests.get(url)
init_image = Image.open(BytesIO(response.content)).convert("RGB")
init_image = init_image.resize((512, 512))
res = pipe.train(
prompt,
image=init_image,
generator=generator)
res = pipe(alpha=1, guidance_scale=7.5, num_inference_steps=50)
os.makedirs("imagic", exist_ok=True)
image = res.images[0]
image.save('./imagic/imagic_image_alpha_1.png')
res = pipe(alpha=1.5, guidance_scale=7.5, num_inference_steps=50)
image = res.images[0]
image.save('./imagic/imagic_image_alpha_1_5.png')
res = pipe(alpha=2, guidance_scale=7.5, num_inference_steps=50)
image = res.images[0]
image.save('./imagic/imagic_image_alpha_2.png')
```
### Seed Resizing
Test seed resizing. Originally generate an image in 512 by 512, then generate image with same seed at 512 by 592 using seed resizing. Finally, generate 512 by 592 using original stable diffusion pipeline.
```python
import torch as th
import numpy as np
from diffusers import DiffusionPipeline
has_cuda = th.cuda.is_available()
device = th.device('cpu' if not has_cuda else 'cuda')
pipe = DiffusionPipeline.from_pretrained(
"CompVis/stable-diffusion-v1-4",
use_auth_token=True,
custom_pipeline="seed_resize_stable_diffusion"
).to(device)
def dummy(images, **kwargs):
return images, False
pipe.safety_checker = dummy
images = []
th.manual_seed(0)
generator = th.Generator("cuda").manual_seed(0)
seed = 0
prompt = "A painting of a futuristic cop"
width = 512
height = 512
res = pipe(
prompt,
guidance_scale=7.5,
num_inference_steps=50,
height=height,
width=width,
generator=generator)
image = res.images[0]
image.save('./seed_resize/seed_resize_{w}_{h}_image.png'.format(w=width, h=height))
th.manual_seed(0)
generator = th.Generator("cuda").manual_seed(0)
pipe = DiffusionPipeline.from_pretrained(
"CompVis/stable-diffusion-v1-4",
use_auth_token=True,
custom_pipeline="/home/mark/open_source/diffusers/examples/community/"
).to(device)
width = 512
height = 592
res = pipe(
prompt,
guidance_scale=7.5,
num_inference_steps=50,
height=height,
width=width,
generator=generator)
image = res.images[0]
image.save('./seed_resize/seed_resize_{w}_{h}_image.png'.format(w=width, h=height))
pipe_compare = DiffusionPipeline.from_pretrained(
"CompVis/stable-diffusion-v1-4",
use_auth_token=True,
custom_pipeline="/home/mark/open_source/diffusers/examples/community/"
).to(device)
res = pipe_compare(
prompt,
guidance_scale=7.5,
num_inference_steps=50,
height=height,
width=width,
generator=generator
)
image = res.images[0]
image.save('./seed_resize/seed_resize_{w}_{h}_image_compare.png'.format(w=width, h=height))
```
### Multilingual Stable Diffusion Pipeline
The following code can generate an images from texts in different languages using the pre-trained [mBART-50 many-to-one multilingual machine translation model](https://huggingface.co/facebook/mbart-large-50-many-to-one-mmt) and Stable Diffusion.
```python
from PIL import Image
import torch
from diffusers import DiffusionPipeline
from transformers import (
pipeline,
MBart50TokenizerFast,
MBartForConditionalGeneration,
)
device = "cuda" if torch.cuda.is_available() else "cpu"
device_dict = {"cuda": 0, "cpu": -1}
# helper function taken from: https://huggingface.co/blog/stable_diffusion
def image_grid(imgs, rows, cols):
assert len(imgs) == rows*cols
w, h = imgs[0].size
grid = Image.new('RGB', size=(cols*w, rows*h))
grid_w, grid_h = grid.size
for i, img in enumerate(imgs):
grid.paste(img, box=(i%cols*w, i//cols*h))
return grid
# Add language detection pipeline
language_detection_model_ckpt = "papluca/xlm-roberta-base-language-detection"
language_detection_pipeline = pipeline("text-classification",
model=language_detection_model_ckpt,
device=device_dict[device])
# Add model for language translation
trans_tokenizer = MBart50TokenizerFast.from_pretrained("facebook/mbart-large-50-many-to-one-mmt")
trans_model = MBartForConditionalGeneration.from_pretrained("facebook/mbart-large-50-many-to-one-mmt").to(device)
diffuser_pipeline = DiffusionPipeline.from_pretrained(
"CompVis/stable-diffusion-v1-4",
custom_pipeline="multilingual_stable_diffusion",
detection_pipeline=language_detection_pipeline,
translation_model=trans_model,
translation_tokenizer=trans_tokenizer,
revision="fp16",
torch_dtype=torch.float16,
)
diffuser_pipeline.enable_attention_slicing()
diffuser_pipeline = diffuser_pipeline.to(device)
prompt = ["a photograph of an astronaut riding a horse",
"Una casa en la playa",
"Ein Hund, der Orange isst",
"Un restaurant parisien"]
output = diffuser_pipeline(prompt)
images = output.images
grid = image_grid(images, rows=2, cols=2)
```
This example produces the following images:
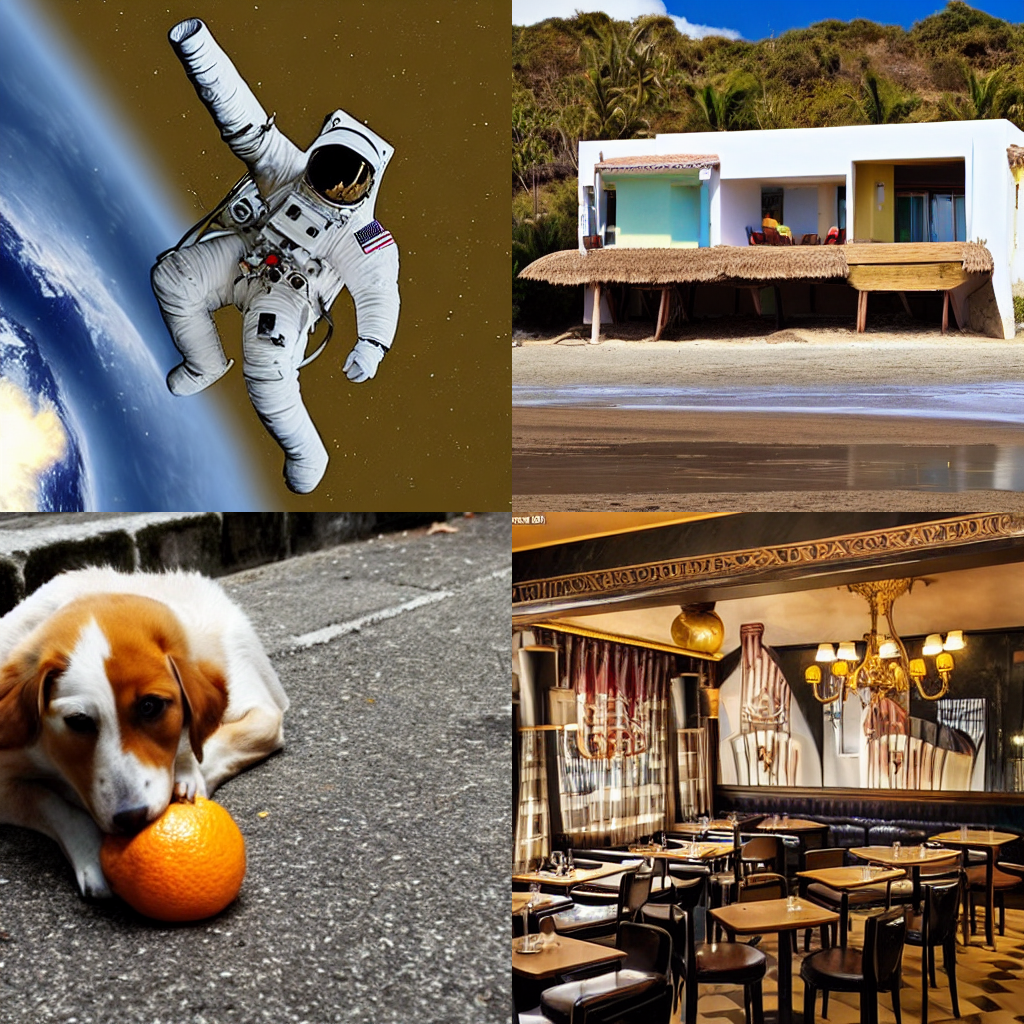
### Image to Image Inpainting Stable Diffusion
Similar to the standard stable diffusion inpainting example, except with the addition of an `inner_image` argument.
`image`, `inner_image`, and `mask` should have the same dimensions. `inner_image` should have an alpha (transparency) channel.
The aim is to overlay two images, then mask out the boundary between `image` and `inner_image` to allow stable diffusion to make the connection more seamless.
For example, this could be used to place a logo on a shirt and make it blend seamlessly.
```python
import PIL
import torch
from diffusers import DiffusionPipeline
image_path = "./path-to-image.png"
inner_image_path = "./path-to-inner-image.png"
mask_path = "./path-to-mask.png"
init_image = PIL.Image.open(image_path).convert("RGB").resize((512, 512))
inner_image = PIL.Image.open(inner_image_path).convert("RGBA").resize((512, 512))
mask_image = PIL.Image.open(mask_path).convert("RGB").resize((512, 512))
pipe = DiffusionPipeline.from_pretrained(
"runwayml/stable-diffusion-inpainting",
custom_pipeline="img2img_inpainting",
revision="fp16",
torch_dtype=torch.float16
)
pipe = pipe.to("cuda")
prompt = "Your prompt here!"
image = pipe(prompt=prompt, image=init_image, inner_image=inner_image, mask_image=mask_image).images[0]
```
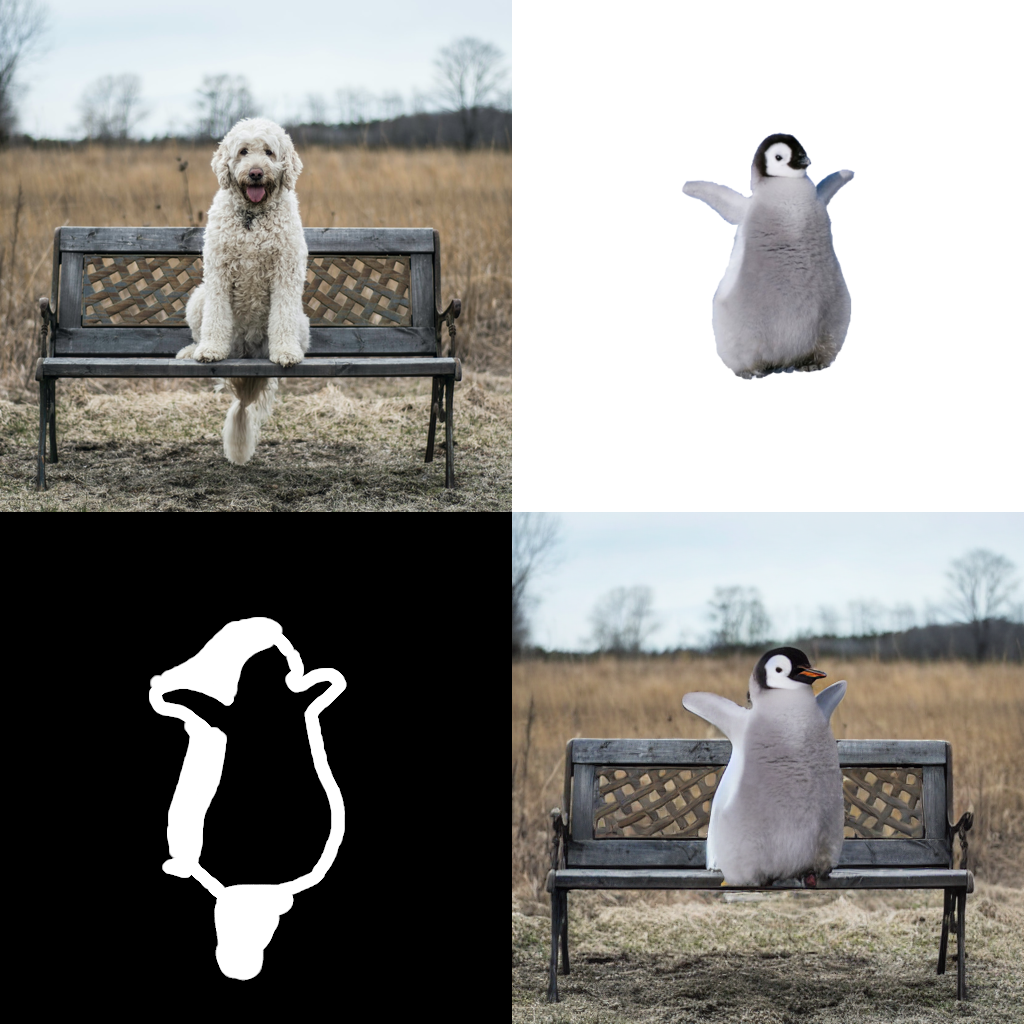
### Text Based Inpainting Stable Diffusion
Use a text prompt to generate the mask for the area to be inpainted.
Currently uses the CLIPSeg model for mask generation, then calls the standard Stable Diffusion Inpainting pipeline to perform the inpainting.
```python
from transformers import CLIPSegProcessor, CLIPSegForImageSegmentation
from diffusers import DiffusionPipeline
from PIL import Image
import requests
from torch import autocast
processor = CLIPSegProcessor.from_pretrained("CIDAS/clipseg-rd64-refined")
model = CLIPSegForImageSegmentation.from_pretrained("CIDAS/clipseg-rd64-refined")
pipe = DiffusionPipeline.from_pretrained(
"runwayml/stable-diffusion-inpainting",
custom_pipeline="text_inpainting",
segmentation_model=model,
segmentation_processor=processor
)
pipe = pipe.to("cuda")
url = "https://github.com/timojl/clipseg/blob/master/example_image.jpg?raw=true"
image = Image.open(requests.get(url, stream=True).raw).resize((512, 512))
text = "a glass" # will mask out this text
prompt = "a cup" # the masked out region will be replaced with this
with autocast("cuda"):
image = pipe(image=image, text=text, prompt=prompt).images[0]
```
### Bit Diffusion
Based https://arxiv.org/abs/2208.04202, this is used for diffusion on discrete data - eg, discreate image data, DNA sequence data. An unconditional discreate image can be generated like this:
```python
from diffusers import DiffusionPipeline
pipe = DiffusionPipeline.from_pretrained("google/ddpm-cifar10-32", custom_pipeline="bit_diffusion")
image = pipe().images[0]
```
### Stable Diffusion with K Diffusion
Make sure you have @crowsonkb's https://github.com/crowsonkb/k-diffusion installed:
```
pip install k-diffusion
```
You can use the community pipeline as follows:
```python
from diffusers import DiffusionPipeline
pipe = DiffusionPipeline.from_pretrained("CompVis/stable-diffusion-v1-4", custom_pipeline="sd_text2img_k_diffusion")
pipe = pipe.to("cuda")
prompt = "an astronaut riding a horse on mars"
pipe.set_scheduler("sample_heun")
generator = torch.Generator(device="cuda").manual_seed(seed)
image = pipe(prompt, generator=generator, num_inference_steps=20).images[0]
image.save("./astronaut_heun_k_diffusion.png")
```
To make sure that K Diffusion and `diffusers` yield the same results:
**Diffusers**:
```python
from diffusers import DiffusionPipeline, EulerDiscreteScheduler
seed = 33
pipe = DiffusionPipeline.from_pretrained("CompVis/stable-diffusion-v1-4")
pipe.scheduler = EulerDiscreteScheduler.from_config(pipe.scheduler.config)
pipe = pipe.to("cuda")
generator = torch.Generator(device="cuda").manual_seed(seed)
image = pipe(prompt, generator=generator, num_inference_steps=50).images[0]
```

**K Diffusion**:
```python
from diffusers import DiffusionPipeline, EulerDiscreteScheduler
seed = 33
pipe = DiffusionPipeline.from_pretrained("CompVis/stable-diffusion-v1-4", custom_pipeline="sd_text2img_k_diffusion")
pipe.scheduler = EulerDiscreteScheduler.from_config(pipe.scheduler.config)
pipe = pipe.to("cuda")
pipe.set_scheduler("sample_euler")
generator = torch.Generator(device="cuda").manual_seed(seed)
image = pipe(prompt, generator=generator, num_inference_steps=50).images[0]
```

### Checkpoint Merger Pipeline
Based on the AUTOMATIC1111/webui for checkpoint merging. This is a custom pipeline that merges upto 3 pretrained model checkpoints as long as they are in the HuggingFace model_index.json format.
The checkpoint merging is currently memory intensive as it modifies the weights of a DiffusionPipeline object in place. Expect atleast 13GB RAM Usage on Kaggle GPU kernels and
on colab you might run out of the 12GB memory even while merging two checkpoints.
Usage:-
```python
from diffusers import DiffusionPipeline
#Return a CheckpointMergerPipeline class that allows you to merge checkpoints.
#The checkpoint passed here is ignored. But still pass one of the checkpoints you plan to
#merge for convenience
pipe = DiffusionPipeline.from_pretrained("CompVis/stable-diffusion-v1-4", custom_pipeline="checkpoint_merger")
#There are multiple possible scenarios:
#The pipeline with the merged checkpoints is returned in all the scenarios
#Compatible checkpoints a.k.a matched model_index.json files. Ignores the meta attributes in model_index.json during comparision.( attrs with _ as prefix )
merged_pipe = pipe.merge(["CompVis/stable-diffusion-v1-4","CompVis/stable-diffusion-v1-2"], interp = "sigmoid", alpha = 0.4)
#Incompatible checkpoints in model_index.json but merge might be possible. Use force = True to ignore model_index.json compatibility
merged_pipe_1 = pipe.merge(["CompVis/stable-diffusion-v1-4","hakurei/waifu-diffusion"], force = True, interp = "sigmoid", alpha = 0.4)
#Three checkpoint merging. Only "add_difference" method actually works on all three checkpoints. Using any other options will ignore the 3rd checkpoint.
merged_pipe_2 = pipe.merge(["CompVis/stable-diffusion-v1-4","hakurei/waifu-diffusion","prompthero/openjourney"], force = True, interp = "add_difference", alpha = 0.4)
prompt = "An astronaut riding a horse on Mars"
image = merged_pipe(prompt).images[0]
```
Some examples along with the merge details:
1. "CompVis/stable-diffusion-v1-4" + "hakurei/waifu-diffusion" ; Sigmoid interpolation; alpha = 0.8

2. "hakurei/waifu-diffusion" + "prompthero/openjourney" ; Inverse Sigmoid interpolation; alpha = 0.8

3. "CompVis/stable-diffusion-v1-4" + "hakurei/waifu-diffusion" + "prompthero/openjourney"; Add Difference interpolation; alpha = 0.5

|