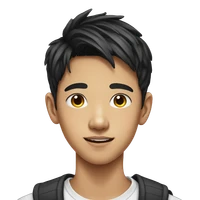
Datasets:
GUI Grounding Pre-training Data for OS-ATLAS
This document describes the acquisition of the pre-training data used by OS-ATLAS OS-ATLAS: A Foundation Action Model for Generalist GUI Agents.
Notes: In GUI grounding data, the position of the target element is recorded in the bbox
key, represented by [left, top, right, bottom]
.
Each value is a [0, 1] decimal number indicating the ratio of the corresponding position to the width or height of the image.
The data stored in this dataset consists of raw data containing only element grounding information. When training a model, you need to use the corresponding prompts to wrap these data.
The data we released is divided into three domains: mobile, desktop and web.
All annotation data is stored in JSON format and each sample contains:
img_filename
: the interface screenshot fileinstruction
: human instruction or referring expression extracted from ally tree or htmlbbox
: the bounding box of the target element corresponding to instruction
Some data also contains a data_type
, which records the type of an element in its structured information, if it can be obtained.
Mobile data
This part of data is stored under the mobile_domain directory. Our mobile grounding data consists of four parts.
AMEX
Android Multi-annotation EXpo (AMEX) is a comprehensive, large-scale dataset designed for generalist mobile GUI-control agents [1].
The annotation data is stored in
-amex_raw.json
Due to the single file size limitation of Hugging Face datasets, we stored the Amex images in zip format and split them into several sub-files.
amex_images_part_aa
amex_images_part_ab
amex_images_part_ac
You need to first merge these split files back into the original file and then extract the contents.
cat amex_images_part_* > amex_images.zip
7z x amex_images.zip -aoa -o/path/to/extract/folder
UIBert
UIBert [2] is a dataset extended from Rico dataset [3] for two tasks: similar UI component retrieval and referring expression component retrieval.
The annotation data is stored in
uibert_raw.json
The UIBert images are stored in
UIBert.zip
Widget Captioning and RICOSCA
Widget Captioning data are collected by [4].
RICOSCA is a dataset automatically labeled using Android VH in [5]
The annotation data is stored in
widget_captioning.json
ricosca.json
The rico images are stored in
rico_imgs.zip
Android_world_data
This part of data are sampled from a android environment for building and benchmarking autonomous computer control agents [6].
The annotation data is stored in
aw_mobile.json
The rico images are stored in
mobile_images.zip
Desktop data
This part of data is stored under the desktop_domain directory.
All of the desktop grounding data is collected from the real environments of personal computers running different operating systems. Each image is split into multiple sub-images to enhance data diversity.
Our desktop grounding data consists of three parts: Windows, Linux and MacOS.
The image and annotation data for each operating system are stored in corresponding zip and json files.
It is worth noting that, due to the large size of the Windows image data, the split files need to be merged before extraction.
cat windows_image_part_* > windows_images.zip
7z x windows_images.zip -aoa -o/path/to/extract/folder
Web data
This part of data is stored under the web_domain directory.
Our desktop grounding data consists of two parts.
Seeclick web data
The web data from SeeClick [7] was crawled from websites provided by Common Crawl, containing more than 270k webpage screenshots and over 3 million webpage elements.
The annotation data is stored in
seeclick_web.json
The images are stored into split files and need to be merged before extraction.
cat seeclick_web_image_part_* > seeclick_web_images.zip
7z x seeclick_web_images.zip -aoa -o/path/to/extract/folder
Fineweb_crawled_data
This part of data is crawled from web pages from the latest URLs obtained from FineWeb [8], a cleaned and deduplicated English dataset derived from Common Crawl.
Since this portion of the data contains at least 1.6 million images, we have compressed them into 10 zip files, from fineweb_3m_s11.zip
to fineweb_3m_s52.zip
.
Please extract them into the same directory.
As an example,
7z x fineweb_3m_s11.zip -aoa -o/same/path/to/extract/fineweb
The annotation data is stored in
fineweb_3m.json
Best practice
During the training of OS-Atlas-4B, we randomly sampled predefined prompts to wrap the grounding data. Additionally, we scaled the relative coordinates of each element (in the range [0, 1]) by multiplying them by 1000 before inputting them into the model for training.
Below is an example of a data entry:
{
"conversations": [
{
"from": "human",
"value": "<image>\nUsing the provided screenshot, I'll describe webpage elements for you to locate (with bbox).\n<ref>media-maniacs.org</ref>\n<ref>Home</ref>\n<ref>Sitemap</ref>\n<ref>shop you can have what you choose 2012</ref>"
},
{
"from": "gpt",
"value": "<ref>media-maniacs.org</ref><box>[[70,856,141,871]]</box>\n<ref>Home</ref><box>[[21,935,43,951]]</box>\n<ref>Sitemap</ref><box>[[21,919,52,934]]</box>\n<ref>shop you can have what you choose 2012</ref><box>[[368,839,523,855]]</box>"
}
]
}
OS-Atlas-7B
{
"conversations": [
{
"from": "human",
"value": "<image>\nUsing the provided screenshot, I'll describe webpage elements for you to locate (with bbox).\n<|object_ref_start|>Facebook<|object_ref_end|>\n<|object_ref_start|>Subscribe<|object_ref_end|>\n<|object_ref_start|>Twitter<|object_ref_end|>\n<|object_ref_start|>Read More<|object_ref_end|>\n<|object_ref_start|>Read More<|object_ref_end|>"
},
{
"from": "gpt",
"value": "<|object_ref_start|>Facebook<|object_ref_end|><|box_start|>(4,955),(36,970)<|box_end|>\n<|object_ref_start|>Subscribe<|object_ref_end|><|box_start|>(4,913),(43,932)<|box_end|>\n<|object_ref_start|>Twitter<|object_ref_end|><|box_start|>(39,955),(62,970)<|box_end|>\n<|object_ref_start|>Read More<|object_ref_end|><|box_start|>(30,138),(73,157)<|box_end|>\n<|object_ref_start|>Read More<|object_ref_end|><|box_start|>(30,139),(73,155)<|box_end|>"
}
]
}
The prompts we used are stored in prompts.json
.
The following are the open-source datasets we used as data sources. We welcome everyone to check the details and cite these sources accordingly!
[1] AMEX: Android Multi-annotation Expo Dataset for Mobile GUI Agents
[2] UIBert: Learning Generic Multimodal Representations for UI Understanding
[3] Rico: A mobile app dataset for building data-driven design applications
[4] Widget Captioning: Generating Natural Language Description for Mobile User Interface Elements
[5] Mapping Natural Language Instructions to Mobile UI Action Sequences
[6] ANDROIDWORLD: A Dynamic Benchmarking Environment for Autonomous Agents
[7] SeeClick: Harnessing GUI Grounding for Advanced Visual GUI Agents
[8] The fineweb datasets: Decanting the web for the finest text data at scale
- Downloads last month
- 1,871
Models trained or fine-tuned on OS-Copilot/OS-Atlas-data
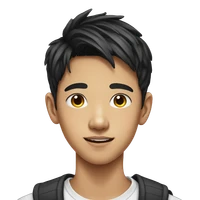