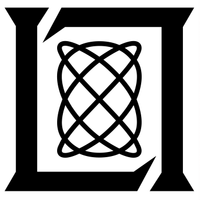
Datasets:
The dataset viewer is not available for this dataset.
Error code: DatasetModuleNotInstalledError Exception: ImportError Message: To be able to use MITLL/LADI-v2-dataset, you need to install the following dependency: cv2. Please install it using 'pip install cv2' for instance. Traceback: Traceback (most recent call last): File "/src/services/worker/src/worker/job_runners/dataset/config_names.py", line 73, in compute_config_names_response config_names = get_dataset_config_names( File "/src/services/worker/.venv/lib/python3.9/site-packages/datasets/inspect.py", line 347, in get_dataset_config_names dataset_module = dataset_module_factory( File "/src/services/worker/.venv/lib/python3.9/site-packages/datasets/load.py", line 1910, in dataset_module_factory raise e1 from None File "/src/services/worker/.venv/lib/python3.9/site-packages/datasets/load.py", line 1876, in dataset_module_factory return HubDatasetModuleFactoryWithScript( File "/src/services/worker/.venv/lib/python3.9/site-packages/datasets/load.py", line 1498, in get_module local_imports = _download_additional_modules( File "/src/services/worker/.venv/lib/python3.9/site-packages/datasets/load.py", line 353, in _download_additional_modules raise ImportError( ImportError: To be able to use MITLL/LADI-v2-dataset, you need to install the following dependency: cv2. Please install it using 'pip install cv2' for instance.
Need help to make the dataset viewer work? Open a discussion for direct support.
Dataset Card for LADI-v2-dataset
Dataset Summary : v2
The LADI-v2 dataset is a set of aerial disaster images captured and labeled by the Civil Air Patrol (CAP). The images are geotagged (in their EXIF metadata). Each image has been labeled in triplicate by CAP volunteers trained in the FEMA damage assessment process for multi-label classification; where volunteers disagreed about the presence of a class, a majority vote was taken. The classes are:
- bridges_any
- bridges_damage
- buildings_affected
- buildings_any
- buildings_destroyed
- buildings_major
- buildings_minor
- debris_any
- flooding_any
- flooding_structures
- roads_any
- roads_damage
- trees_any
- trees_damage
- water_any
The v2 dataset consists of approximately 10k images, split into a train set of 8k images, a validation set of 1k images, and a test test of 1k images. The train and validation sets are drawn from the same distribution (CAP images from federally-declared disasters 2015-2022), whereas the test set is drawn from events in 2023, which has a different distribution of event types and locations. This is done to simulate the distribution shift as new events occur each year.
Dataset v2a
The v2a
dataset presents the same images with a subset of the labels, where the damage categories for buildings have been compressed into two classes of buildings_affected_or_greater
and buildings_minor_or_greater
. We find that this task is easier and of similar practical value for triage purposes. The bridges_damage
label has also been removed due to the low number of positive examples in the dataset.
- bridges_any
- buildings_any
- buildings_affected_or_greater
- buildings_minor_or_greater
- debris_any
- flooding_any
- flooding_structures
- roads_any
- roads_damage
- trees_any
- trees_damage
- water_any
Dataset Summary: v1
This dataset code also supports loading a subset of the LADI v1 dataset, which consists of roughly 25k images, broken into two tasks, 'infrastructure' and 'damage'. The LADI v1 dataset was labeled by crowdsourced workers and the labels shouldn't be considered definitive. The data may be suitable for a pretraining task prior to fine-tuning on LADI v2.
The infrastructure task involves identifying infrastructure in images and has classes building
and road
. It is divided into a train set of 8.2k images and a test set of 2k images.
The damage task involves identifying damage and has classes flood
, rubble
, and misc_damage
. It is divided into a train set of 14.4k images and a test set of 3.6k images.
Supported Tasks
The images are labeled for multi-label classification, as any number of the elements listed above may be present in a single image.
Data Structure
A single example in the v2a dataset looks like this:
{
'image': <PIL.PngImagePlugin.PngImageFile image mode=RGB size=1800x1200 at ...>,
'bridges_any': False,
'buildings_any': False,
'buildings_affected_or_greater': False,
'buildings_minor_or_greater': False,
'debris_any': False,
'flooding_any': False,
'flooding_structures': False,
'roads_any': False,
'roads_damage': False,
'trees_any': True,
'trees_damage': True,
'water_any': True
}
Examples in the v1 datasets are analogous, with classes drawn from their respective tasks (infrastructure and damage).
Using the Dataset
Downloading the Dataset
You can download the dataset by loading it with download_ladi=True
, which fetches the compressed data from an s3 bucket and extracts it into your filesystem:
from datasets import load_dataset
ds = load_dataset("MITLL/LADI-v2-dataset", "v2a_resized",
streaming=True, download_ladi=True,
base_dir='./ladi_dataset', trust_remote_code=True)
You can browse the bucket here: https://ladi.s3.amazonaws.com/index.html. Note that the v2_resized
dataset is the same as the v2
dataset, but with lower-resolution images (1800x1200 px). We expect that these images are still more than large enough to support most tasks, and encourage you to use the v2_resized and v2a_resized datasets when possible as the download is about 45x smaller. We try not to download images you don't need, so this will only fetch the v2_resized images, leaving v1 and v2 alone.
We intend for this dataset to be used mostly in streaming mode from individual files. While you can convert it to a parquet table, we typically use the dataset with streaming=True
, which allows you to navigate, inspect, and alter the dataset on the filesystem. After the initial download, simply omitting the download_ladi
argument, or passing download_ladi=False
, will use the version of LADI already in base_dir
:
from datasets import load_dataset
ds = load_dataset("MITLL/LADI-v2-dataset", "v2a_resized",
streaming=True, base_dir='./ladi_dataset',
trust_remote_code=True)
As previously noted, LADI v1 does not have separate test and validation sets, so the 'val' and 'test' splits in LADI v1 data point to the same labels!
Dataset Information:
- Citation - BibTeX:
Paper forthcoming - watch this space for details
- Developed by: Jeff Liu, Sam Scheele
- Funded by: Department of the Air Force under Air Force Contract No. FA8702-15-D-0001
- License: MIT
DISTRIBUTION STATEMENT A. Approved for public release. Distribution is unlimited.
This material is based upon work supported by the Department of the Air Force under Air Force Contract No. FA8702-15-D-0001. Any opinions, findings, conclusions or recommendations expressed in this material are those of the author(s) and do not necessarily reflect the views of the Department of the Air Force.
© 2024 Massachusetts Institute of Technology.
The software/firmware is provided to you on an As-Is basis
Delivered to the U.S. Government with Unlimited Rights, as defined in DFARS Part 252.227-7013 or 7014 (Feb 2014). Notwithstanding any copyright notice, U.S. Government rights in this work are defined by DFARS 252.227-7013 or DFARS 252.227-7014 as detailed above. Use of this work other than as specifically authorized by the U.S. Government may violate any copyrights that exist in this work.
- Downloads last month
- 58