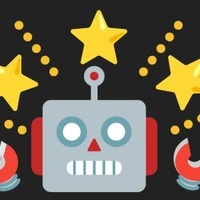
autoevaluator
HF staff
Add evaluation results on the sentiment config and test split of tweet_eval
e907b6b
metadata
language: en
datasets:
- tweet_eval
widget:
- text: Covid cases are increasing fast!
model-index:
- name: cardiffnlp/twitter-roberta-base-sentiment-latest
results:
- task:
type: text-classification
name: Text Classification
dataset:
name: tweet_eval
type: tweet_eval
config: sentiment
split: test
metrics:
- type: accuracy
value: 0.7219960924780202
name: Accuracy
verified: true
verifyToken: >-
eyJhbGciOiJFZERTQSIsInR5cCI6IkpXVCJ9.eyJoYXNoIjoiMGM1NzYxOTJjODg5MDllMzNkODk3NGE1NmNjNWJlOWViYWNmOGRjMGI3MTVlYjQyNDY3MzVjYzMyYmZiYzliMyIsInZlcnNpb24iOjF9.uWmmGJR83ee7_Fg5lG_atB8miVSheCmw7fhxZvJSdky1XcuHNSy9-SyRVg8kggNiMcL5vEBCsfFMrS7J134KBw
- type: f1
value: 0.7241871382174582
name: F1 Macro
verified: true
verifyToken: >-
eyJhbGciOiJFZERTQSIsInR5cCI6IkpXVCJ9.eyJoYXNoIjoiM2VmZDZkMTI4MDJlMDg5MGFhNDE3YTUxZTdlM2NmNjk5NDcwZDkwNjk4NDEzMzlkMDY5YWU5YTMyMTI3ZDlmNSIsInZlcnNpb24iOjF9.41oMX8kV6C9iICfZlNILOwLMODlYZQXr50sEHX88Eu8-Py2ZCR1raq_fWpTraRE56XBzdFZJQYIGEQxR6GAcCA
- type: f1
value: 0.7219960924780202
name: F1 Micro
verified: true
verifyToken: >-
eyJhbGciOiJFZERTQSIsInR5cCI6IkpXVCJ9.eyJoYXNoIjoiMzI4NDljZDcyNGIzMzYwZDJjOWMzNWZkZDZkNWJkYWRkNGEzNGJiNmJiMmJkNDEwMWVhNzM2NDIwNTBjZjdjZCIsInZlcnNpb24iOjF9.Quplp1xsiPIYPLHy7GivJhn9c7BZWI6HfxZ8KimWUuFulkLbZxV0iVCrahyVMzfjitJAOE3P7Tt2PqLkkJwADQ
- type: f1
value: 0.7208112218231548
name: F1 Weighted
verified: true
verifyToken: >-
eyJhbGciOiJFZERTQSIsInR5cCI6IkpXVCJ9.eyJoYXNoIjoiZDU5YTBjZmUzNjI3NGFhNDdkYTM2NWMxZGMwMzM4YmUwNzI1NmZkOGM4OWM1NmNmNzE0ZjAwNWM5Y2JkNTNjYyIsInZlcnNpb24iOjF9.W2yb9xfWNXgj-h4vXvvybT28eI2HNY5-rCLRVtKeZ7hjsgrXO6uhIkm4azSkX17IOcvz89XicjGg9HeAuTroBQ
- type: precision
value: 0.7188694819994699
name: Precision Macro
verified: true
verifyToken: >-
eyJhbGciOiJFZERTQSIsInR5cCI6IkpXVCJ9.eyJoYXNoIjoiMjJiYTJjNmE2MTlhZjZkMDQwZTM3NGFkZDM3ZGZjMzEwZGViNDg2ZTk3NzAwNDEzYTNmNWM5M2U3YWRjYTcyNiIsInZlcnNpb24iOjF9.bUL4gT0f_MJ11k0D6HtoOPkLsqwnaR22ym7u4oDCcWN81HUXHjNHRG-v416yQ1cbRaRg4PgkiynS5UBxk8EMBQ
- type: precision
value: 0.7219960924780202
name: Precision Micro
verified: true
verifyToken: >-
eyJhbGciOiJFZERTQSIsInR5cCI6IkpXVCJ9.eyJoYXNoIjoiMzcwOTQ5MzM0ZWZjMDk3MzNmN2IxNTJkYjI3ZDY1NGU2MDMyMDJjMTcyYWYwNmIxZmMwMWJiZDQyODE4ODA1YyIsInZlcnNpb24iOjF9.c2iXrDnKQ_fIX017v1WhCcisAuLOCTRkct9_wIg59c8Wt7heKvL3kg8phfuOmUv9vzZtTctdhzoeXCurQcRsBA
- type: precision
value: 0.7260700483940776
name: Precision Weighted
verified: true
verifyToken: >-
eyJhbGciOiJFZERTQSIsInR5cCI6IkpXVCJ9.eyJoYXNoIjoiMDI3ZmI3OGE4MTI1MmI1ZDM4ZGRmNGI1NDMxMzkwNzkwYjhiNWZjZGE2MjEzZDY0NDIwMWI4ZWNlNDc0ZmJiNyIsInZlcnNpb24iOjF9.aaYwzGJLwDsfALehisQKoEO8cx7yazGAq3oktqL-hC9o4J3YH1mke8_ab3PeOtYiVwYy-Ek_jvo2JAfeanRYCw
- type: recall
value: 0.7350898220292059
name: Recall Macro
verified: true
verifyToken: >-
eyJhbGciOiJFZERTQSIsInR5cCI6IkpXVCJ9.eyJoYXNoIjoiMjdiZDVjZTVhYzEyNjM1ZTMyZjVkOTljYjIwMTM0YmQxYjU5OGY3ZGE5NjYwZWRlOGEyMDg0NjNlODJiYTkzOCIsInZlcnNpb24iOjF9.zpUj26PoWaX8tgIv_PM1xAwGsezVF1sEAkpGY9YY98z3wec67765MVSWGFwk6mzdQQD5S0hLfvmgSyus1qJpCQ
- type: recall
value: 0.7219960924780202
name: Recall Micro
verified: true
verifyToken: >-
eyJhbGciOiJFZERTQSIsInR5cCI6IkpXVCJ9.eyJoYXNoIjoiMDliYTA5ZDQ0YjZjN2NjMmI0Y2NhYTQzMjM2MWYzYjUzMjg3NjkyOWQzYmU0NmVhYWZlYmJkNzdmMWJkZDJiMiIsInZlcnNpb24iOjF9.BLIIEbAnz72FSwxC7GaBGJp1T1kMb23rR1owVfJE7pcVHcALRpSH-ztdYHgs_dQw7_uZibYRXcoCtIfwHzaFBg
- type: recall
value: 0.7219960924780202
name: Recall Weighted
verified: true
verifyToken: >-
eyJhbGciOiJFZERTQSIsInR5cCI6IkpXVCJ9.eyJoYXNoIjoiYTllZWVhMzVjNjZlNWI5MzIyM2E4YjI4ZjFkZDgwNDAwNWYyYWY0ZTM0MzE5MTJhNmYyMjIwMTFiN2ExNzYxZSIsInZlcnNpb24iOjF9.9F7TUcFAWutxhWAEoJMz-ExjL8Zr-KPAYaUxYpQiGTDuhSfWAgIi580-S8QoS_pSsIoAOjD3J5tG8GDLC4-2Cw
- type: loss
value: 0.6139620542526245
name: loss
verified: true
verifyToken: >-
eyJhbGciOiJFZERTQSIsInR5cCI6IkpXVCJ9.eyJoYXNoIjoiYjA4MDcyNTA3ODRhMmZiNDBlMGU3YTk4MzBmY2NlYWYzM2YzYjRkZDEwNWJhOTM2M2VkZDQ1ZjdhOGFkMDAxNiIsInZlcnNpb24iOjF9.VuIi5ytIm14OrN1mrgEgYu1nu2GHhK6KWcrwfKEzzF_1CXmkXQnmOK_NIdstTvbHrqPnkwEwAqctbO37Tr-GDg
Twitter-roBERTa-base for Sentiment Analysis - UPDATED (2022)
This is a RoBERTa-base model trained on ~124M tweets from January 2018 to December 2021, and finetuned for sentiment analysis with the TweetEval benchmark. The original Twitter-based RoBERTa model can be found here and the original reference paper is TweetEval. This model is suitable for English.
- Reference Paper: TimeLMs paper.
- Git Repo: TimeLMs official repository.
Labels: 0 -> Negative; 1 -> Neutral; 2 -> Positive
This sentiment analysis model has been integrated into TweetNLP. You can access the demo here.
Example Pipeline
from transformers import pipeline
sentiment_task = pipeline("sentiment-analysis", model=model_path, tokenizer=model_path)
sentiment_task("Covid cases are increasing fast!")
[{'label': 'Negative', 'score': 0.7236}]
Full classification example
from transformers import AutoModelForSequenceClassification
from transformers import TFAutoModelForSequenceClassification
from transformers import AutoTokenizer, AutoConfig
import numpy as np
from scipy.special import softmax
# Preprocess text (username and link placeholders)
def preprocess(text):
new_text = []
for t in text.split(" "):
t = '@user' if t.startswith('@') and len(t) > 1 else t
t = 'http' if t.startswith('http') else t
new_text.append(t)
return " ".join(new_text)
MODEL = f"cardiffnlp/twitter-roberta-base-sentiment-latest"
tokenizer = AutoTokenizer.from_pretrained(MODEL)
config = AutoConfig.from_pretrained(MODEL)
# PT
model = AutoModelForSequenceClassification.from_pretrained(MODEL)
#model.save_pretrained(MODEL)
text = "Covid cases are increasing fast!"
text = preprocess(text)
encoded_input = tokenizer(text, return_tensors='pt')
output = model(**encoded_input)
scores = output[0][0].detach().numpy()
scores = softmax(scores)
# # TF
# model = TFAutoModelForSequenceClassification.from_pretrained(MODEL)
# model.save_pretrained(MODEL)
# text = "Covid cases are increasing fast!"
# encoded_input = tokenizer(text, return_tensors='tf')
# output = model(encoded_input)
# scores = output[0][0].numpy()
# scores = softmax(scores)
# Print labels and scores
ranking = np.argsort(scores)
ranking = ranking[::-1]
for i in range(scores.shape[0]):
l = config.id2label[ranking[i]]
s = scores[ranking[i]]
print(f"{i+1}) {l} {np.round(float(s), 4)}")
Output:
1) Negative 0.7236
2) Neutral 0.2287
3) Positive 0.0477
References
@inproceedings{camacho-collados-etal-2022-tweetnlp,
title = "{T}weet{NLP}: Cutting-Edge Natural Language Processing for Social Media",
author = "Camacho-collados, Jose and
Rezaee, Kiamehr and
Riahi, Talayeh and
Ushio, Asahi and
Loureiro, Daniel and
Antypas, Dimosthenis and
Boisson, Joanne and
Espinosa Anke, Luis and
Liu, Fangyu and
Mart{\'\i}nez C{\'a}mara, Eugenio" and others,
booktitle = "Proceedings of the 2022 Conference on Empirical Methods in Natural Language Processing: System Demonstrations",
month = dec,
year = "2022",
address = "Abu Dhabi, UAE",
publisher = "Association for Computational Linguistics",
url = "https://aclanthology.org/2022.emnlp-demos.5",
pages = "38--49"
}
@inproceedings{loureiro-etal-2022-timelms,
title = "{T}ime{LM}s: Diachronic Language Models from {T}witter",
author = "Loureiro, Daniel and
Barbieri, Francesco and
Neves, Leonardo and
Espinosa Anke, Luis and
Camacho-collados, Jose",
booktitle = "Proceedings of the 60th Annual Meeting of the Association for Computational Linguistics: System Demonstrations",
month = may,
year = "2022",
address = "Dublin, Ireland",
publisher = "Association for Computational Linguistics",
url = "https://aclanthology.org/2022.acl-demo.25",
doi = "10.18653/v1/2022.acl-demo.25",
pages = "251--260"
}