File size: 6,586 Bytes
0772425 cc3f8cb 0772425 cc3f8cb 0772425 cc3f8cb 0772425 ab70036 27b2af9 cc3f8cb 27b2af9 0772425 |
1 2 3 4 5 6 7 8 9 10 11 12 13 14 15 16 17 18 19 20 21 22 23 24 25 26 27 28 29 30 31 32 33 34 35 36 37 38 39 40 41 42 43 44 45 46 47 48 49 50 51 52 53 54 55 56 57 58 59 60 61 62 63 64 65 66 67 68 69 70 71 72 73 74 75 76 77 78 79 80 81 82 83 84 85 86 87 88 89 90 91 92 93 94 95 96 97 98 99 100 101 102 103 104 105 106 107 108 109 110 111 112 113 114 115 116 117 118 119 120 121 122 123 124 125 126 127 128 129 130 131 132 133 134 135 136 137 138 139 140 141 142 143 144 145 146 147 148 149 150 151 152 153 154 155 156 157 158 159 160 161 162 163 164 165 166 167 168 169 170 171 172 173 174 175 176 177 178 179 180 181 182 183 184 185 |
---
language:
- en
library_name: transformers
license: cc-by-4.0
tags:
- kl3m
- kl3m-002
- patent
- all the patents
- slm
date: '2024-03-12T00:00:00.000Z'
pipeline_tag: text-generation
widget:
- text: "# Title\n"
- temperature: 0.3
- do_sample: True
---
# All the Patents 170m Model
`kl3m-002-170m-patent` is a a (very) small language model (SLM) model fine-tuned from `kl3m-002-170m` to
generate "realistic" patent text. For more information about the base model,
please see [its model page](https://huggingface.co/alea-institute/kl3m-002-170m).
# All the Patents
## Why?
#### If a GPT2-sized model can generate a valid set of claims, should anyone be able to monopolize the invention?
At their heart, patents are a temporary, sanctioned monopoly on an invention through a license to sue. This monopoly
is justified by the public good created by encouraging innovation and the long-term impact of that innovation being
shared in the public domain.
Unfortunately, this worthy policy goal has been lost in the chaos and misuse of the patent system.
One of the most common sources of frustration is the granting of "obvious" patents. While some inventions are clearly novel
and non-obvious, many are not - but still slip through the examination process. These obvious but granted patents then
loom large over the market, creating a "thicket" that discourages use or subsequent invention in the area of the granted
patent. "Undoing" the grant of a patent is a costly and time-consuming process with possible negative consequences, and
so many of these patents simply sit as prior art on the books, even if the patentholder knows they could never enforce them.
Congress and various stakeholders have discussed and proposed changes over time, including most recently the
America Invents Act (AIA), but the problem of obvious patents persists.
But what if someone were to generate all the obvious inventions and make them public?
What if we shared the means of producing these obvious inventions so that everyone could help generate them on a normal CPU or consumer GPU?
And what if we could then make those obvious inventions easily searchable for anyone, including PTO examiners themselves, to use?
## How it Works
We start with a small, GPT2-sized large language model - [kl3m-170](https://273ventures.com/kl3m-the-first-legal-large-language-model/) - which was trained on a clean, copyright-free dataset.
This helps us ensure that generations do not include copyrighted text, which would allow third-parties to interfere with the project
via DMCA takedown requests.
Next, we fine-tune this model on two simultaneous tasks:
1. **Top-down drafting**: We start from the most abstract parts of the patent - the title and abstract - and then generate the detailed claims. This is a traditional next-token prediction order.
```text
# Patent
## Title
{title}
## Abstract
{abstract}
## Claims
1. {claim 1}
2. {claim 2}
...
```
2. **Bottom-up**: We start from the most detailed part of the patent - the claims - and then generate the abstract and title. This reversed order can be thought of as similar to traditional extractive/abstractive summarization tasks.
```text
# Patent
## Claims
1. {claim 1}
2. {claim 2}
...
## Abstract
{abstract}
## Title
{title}
```
Once this fine-tuning is complete, we can then generate new patents using either technique by prompting the model as follows:
1. **Top-down prompt**: `"# Patent\n\n## Title"`
2. **Bottom-up prompt**: `"# Patent\n\n## Claims"`
It's critical that generation occurs with sufficient randomness and diversity to ensure that the generated patents are not
simply reproductions of the training data. This is a key area of ongoing research and development.
**Much like the real process of invention, most of the "ideas" generated by this process will be either nonsense or
unpatentable otherwise. Our goal is to estimate the "hit rate" of the model and continue to improve the efficiency and
accessibility of the generation process so that the "cost per obvious invention" is as low as possible.**
## Current Status
This project is still in its infancy. We're doing R&D to develop prototype tools to demonstrate the possibility and
cost of generating and sharing these obvious inventions. This R&D is currently focused on data collection,
data curation, model training, and model evaluation.
## Generation
You can generate your own examples as follows. For a "complete" patent, you'll want to extend the `max_new_tokens` value to the biggest number you can fit in your available VRAM.
```python
import json
from transformers import pipeline
# Load the model and tokenizer on CPU
p = pipeline('text-generation', 'alea-institute/kl3m-002-170m-patent', device='cpu')
# Example usage on CPU
text = "# Patent\n\n## Title"
print(
json.dumps(
[
r.get("generated_text")
for r in p(text, do_sample=True, temperature=0.5, num_return_sequences=3, max_new_tokens=32)
],
indent=2
)
)
```
```json
[
"# Patent\n\n## Title\nMethod for manufacturing a temperature-controllable polyurethane composition and method",
"# Patent\n\n## Title\nElectronic device\n\n## Abstract\nAn electronic device includes a display panel and a",
"# Patent\n\n## Title\nMethods and devices for tissue repair using a neural network\n\n## Abstract"
]
```
### Related Material
* https://www.federalregister.gov/documents/2024/02/27/2024-03967/updated-guidance-for-making-a-proper-determination-of-obviousness
## License
This model was originally developed by 273 Ventures and has been donated to the ALEA Institute.
The model weights are released under the CC-BY 4.0 License.
## Contact
The KL3M model family is now maintained by the [ALEA Institute](https://aleainstitute.ai). For technical support, collaboration opportunities, or general inquiries:
- GitHub: https://github.com/alea-institute/kl3m-model-research
- Email: hello@aleainstitute.ai
- Website: https://aleainstitute.ai
## Acknowledgments
Special thanks to 273 Ventures for developing and donating this model to the open-source community through the Alea Institute.
## Citation
Tokenizer, dataset, and model publications are pending.
## Contact
For any questions, please contact [ALEA Institute](https://aleainstitute.ai) at [hello@aleainstitute.ai](mailto:hello@aleainstitute.ai) or
create an issue on this repository or [GitHub](https://github.com/alea-institute/kl3m-model-research).
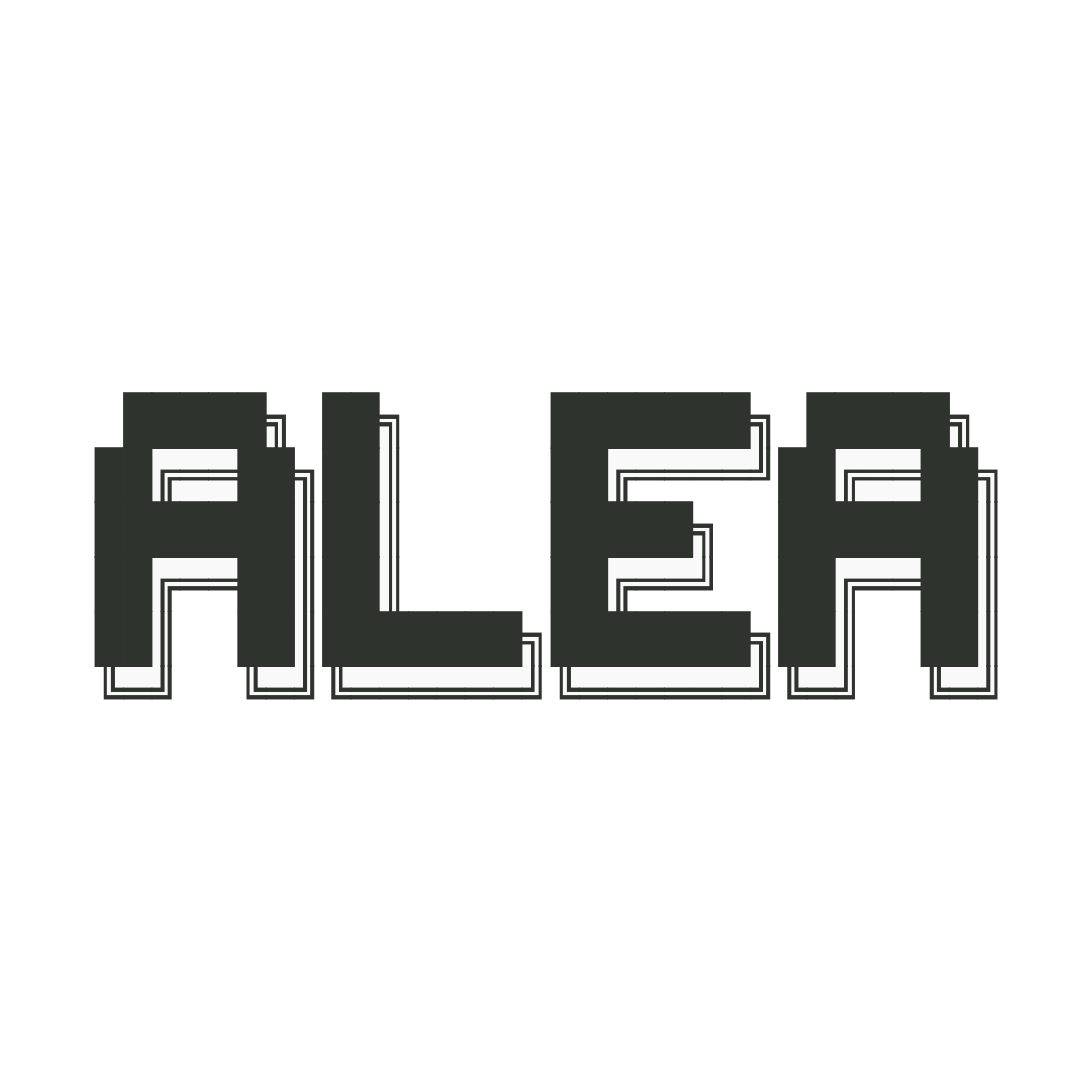 |