File size: 13,894 Bytes
c301678 96f8ee3 c301678 2f5718d 1ec0ea0 2f5718d 6204318 b45a03e 2f5718d 1ec0ea0 2f5718d 4ceff8d 2f5718d 4d5178e 2f5718d 4ceff8d a6b32a7 d7d482b 4d5178e 4ceff8d 2f5718d 4ceff8d |
1 2 3 4 5 6 7 8 9 10 11 12 13 14 15 16 17 18 19 20 21 22 23 24 25 26 27 28 29 30 31 32 33 34 35 36 37 38 39 40 41 42 43 44 45 46 47 48 49 50 51 52 53 54 55 56 57 58 59 60 61 62 63 64 65 66 67 68 69 70 71 72 73 74 75 76 77 78 79 80 81 82 83 84 85 86 87 88 89 90 91 92 93 94 95 96 97 98 99 100 101 102 103 104 105 106 107 108 109 110 111 112 113 114 115 116 117 118 119 120 121 122 123 124 125 126 127 128 129 130 131 132 133 134 135 136 137 138 139 140 141 142 143 144 145 146 147 148 149 150 151 152 153 154 155 156 157 158 159 160 161 162 163 164 165 166 167 168 169 170 171 172 173 174 175 176 177 178 179 180 181 182 183 184 185 186 187 188 189 190 191 192 193 194 195 196 197 198 199 200 201 202 203 204 205 206 207 208 209 210 211 212 213 214 215 216 217 218 219 220 221 222 223 224 225 226 227 228 229 230 231 232 233 234 235 236 237 238 239 240 241 242 243 244 245 246 247 248 249 250 251 252 253 254 255 256 257 258 259 260 261 262 263 264 265 266 267 268 269 270 271 272 273 274 275 276 277 |
---
license: apache-2.0
datasets:
- cerebras/SlimPajama-627B
- EleutherAI/pile
language:
- en
---
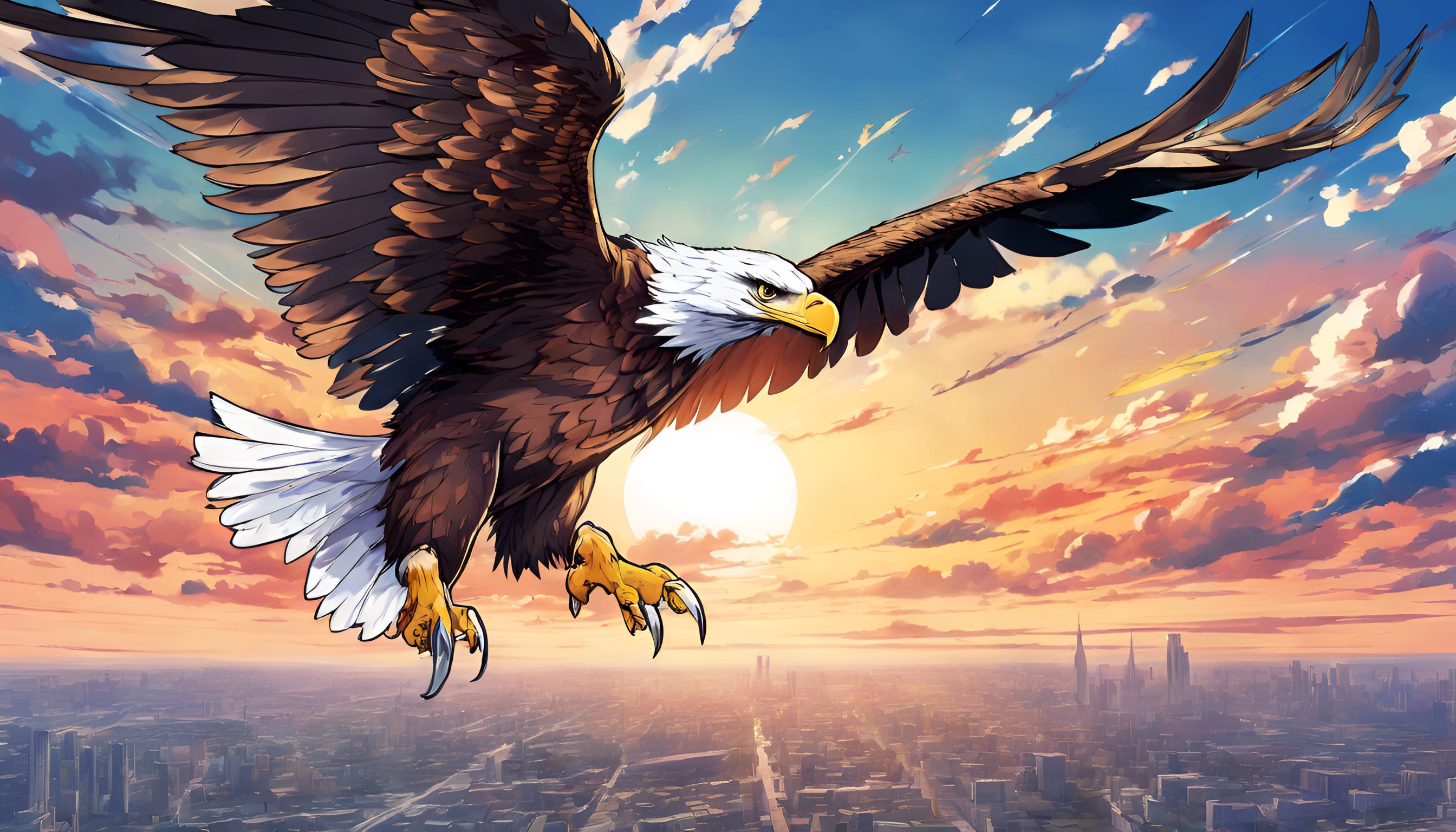
### Huggingface RWKV EagleX 7B v2 Model
> **! Important Note !**
>
> The following is the HF transformers implementation of the EagleX 7B 2.25T model. This is meant to be used with the huggingface transformers
>
> [For the full model weights on its own, to use with other RWKV libraries, refer to `RWKV/v5-EagleX-v2-7B-pth`](https://huggingface.co/RWKV/v5-EagleX-v2-7B-pth)
>
>
> This is not an instruct tune model! (soon...)
## Quickstart with the hugging face transformer library
```
model = AutoModelForCausalLM.from_pretrained("RWKV/v5-Eagle-7B-HF", trust_remote_code=True).to(torch.float32)
tokenizer = AutoTokenizer.from_pretrained("RWKV/v5-Eagle-7B-HF", trust_remote_code=True)
```
## Evaluation
The following shows the progression of the model from 1.1T trained to 2.25T trained.
|Model |Eagle-7B-HF|EagleX-7B-HF-v1|EagleX-7B-HF-v2|
|----------------------|-----------|---------------|---------------|
|Param Count |7.52 B |7.52 B |7.52 B |
|Tokens Trained |1.1 T |1.7 T |2.25 T |
|avg_acc |0.4822 |0.5391 |0.5495 |
|glue (acc) |0.5752 |0.7463 |0.7439 |
|anli (acc) |0.3594 |0.4847 |0.5097 |
|mnli (acc) |0.3802 |0.7928 |0.7884 |
|mnli_mismatch (acc) |0.3687 |0.7985 |0.784 |
|swag (acc) |0.568 |0.5814 |0.5905 |
|lambada_standard (acc)|0.685 |0.686 |0.7004 |
|lambada_openai (acc) |0.7425 |0.7522 |0.7502 |
|mmlu (acc) |0.3321 |0.4014 |0.438 |
|winogrande (acc) |0.674 |0.7206 |0.7332 |
|wnli (acc) |0.4225 |0.4648 |0.493 |
|truthfulqa (acc) |0.3303 |0.3268 |0.3401 |
|logiqa (acc) |0.2458 |0.2458 |0.2458 |
|logiqa2 (acc) |0.2494 |0.2595 |0.2621 |
|sciq (acc) |0.955 |0.96 |0.93 |
|piqa (acc) |0.7704 |0.7758 |0.7764 |
|arc_easy (acc) |0.7382 |0.7555 |0.7445 |
|arc_challenge (acc) |0.3951 |0.4087 |0.4155 |
|hellaswag (acc) |0.5264 |0.5411 |0.56 |
|openbookqa (acc) |0.302 |0.296 |0.304 |
|mathqa (acc) |0.26 |0.26 |0.2593 |
|arithmetic (acc) |0.245 |0.0634 |0.1703 |
Compared against other top performing models in the same weight class.
|Model |OLMo-7B |falcon-7b |Llama-2-7b-hf|EagleX-7B-HF-v2|Mistral-7B-v0.1|
|----------------------|---------------|----------------|-------------|---------------|---------------|
|Param Count |6.89 B |6.92 B |6.74 B |7.52 B |7.24 B |
|Tokens Trained |2.5 T |1.5 T |2 T |2.25 T |2 - 7 T? |
|avg_acc |0.4578 |0.4775 |0.5045 |0.5495 |0.5676 |
|glue (acc) |0.474 |0.4578 |0.4289 |0.7439 |0.515 |
|anli (acc) |0.3478 |0.3541 |0.3697 |0.5097 |0.3803 |
|mnli (acc) |0.3294 |0.3893 |0.4269 |0.7884 |0.4542 |
|mnli_mismatch (acc) |0.3348 |0.404 |0.4395 |0.784 |0.4632 |
|swag (acc) |0.5512 |0.5685 |0.5658 |0.5905 |0.5756 |
|lambada_standard (acc)|0.6396 |0.6868 |0.6808 |0.7004 |0.6944 |
|lambada_openai (acc) |0.6872 |0.746 |0.7353 |0.7502 |0.7553 |
|mmlu (acc) |0.2812 |0.2512 |0.4077 |0.438 |0.5964 |
|winogrande (acc) |0.6725 |0.6709 |0.6914 |0.7332 |0.7364 |
|wnli (acc) |0.5775 |0.4789 |0.4648 |0.493 |0.5775 |
|truthfulqa (acc) |0.3015 |0.2826 |0.3205 |0.3401 |0.3537 |
|logiqa (acc) |0.2335 |0.2151 |0.2535 |0.2458 |0.2427 |
|logiqa2 (acc) |0.2506 |0.2252 |0.2564 |0.2621 |0.3022 |
|sciq (acc) |0.927 |0.944 |0.939 |0.93 |0.959 |
|piqa (acc) |0.7878 |0.7949 |0.7807 |0.7764 |0.8052 |
|arc_easy (acc) |0.7353 |0.7479 |0.7643 |0.7445 |0.8081 |
|arc_challenge (acc) |0.3677 |0.4027 |0.4309 |0.4155 |0.5009 |
|hellaswag (acc) |0.5572 |0.5772 |0.5713 |0.56 |0.6131 |
|openbookqa (acc) |0.292 |0.306 |0.316 |0.304 |0.33 |
|mathqa (acc) |0.26 |0.2884 |0.2801 |0.2593 |0.3554 |
|arithmetic (acc) |0.0069 |0.2367 |0.4703 |0.1703 |0.9004 |
See the following, for the full details on this model: [https://blog.rwkv.com/p/eaglex-v2-soaring-past-llama2-7b](https://blog.rwkv.com/p/eaglex-v2-soaring-past-llama2-7b)
#### Running on CPU via HF transformers
```python
import torch
from transformers import AutoModelForCausalLM, AutoTokenizer
def generate_prompt(instruction, input=""):
instruction = instruction.strip().replace('\r\n','\n').replace('\n\n','\n')
input = input.strip().replace('\r\n','\n').replace('\n\n','\n')
if input:
return f"""Instruction: {instruction}
Input: {input}
Response:"""
else:
return f"""User: hi
Assistant: Hi. I am your assistant and I will provide expert full response in full details. Please feel free to ask any question and I will always answer it.
User: {instruction}
Assistant:"""
model = AutoModelForCausalLM.from_pretrained("RWKV/v5-Eagle-7B-HF", trust_remote_code=True).to(torch.float32)
tokenizer = AutoTokenizer.from_pretrained("RWKV/v5-Eagle-7B-HF", trust_remote_code=True)
text = "请介绍北京的旅游景点"
prompt = generate_prompt(text)
inputs = tokenizer(prompt, return_tensors="pt")
output = model.generate(inputs["input_ids"], max_new_tokens=333, do_sample=True, temperature=1.0, top_p=0.3, top_k=0, )
print(tokenizer.decode(output[0].tolist(), skip_special_tokens=True))
```
output:
```shell
User: hi
Assistant: Hi. I am your assistant and I will provide expert full response in full details. Please feel free to ask any question and I will always answer it.
User: 请介绍北京的旅游景点
Assistant: 北京是中国的首都,拥有众多的旅游景点,以下是其中一些著名的景点:
1. 故宫:位于北京市中心,是明清两代的皇宫,内有大量的文物和艺术品。
2. 天安门广场:是中国最著名的广场之一,是中国人民政治协商会议的旧址,也是中国人民政治协商会议的中心。
3. 颐和园:是中国古代皇家园林之一,有着悠久的历史和丰富的文化内涵。
4. 长城:是中国古代的一道长城,全长约万里,是中国最著名的旅游景点之一。
5. 北京大学:是中国著名的高等教育机构之一,有着悠久的历史和丰富的文化内涵。
6. 北京动物园:是中国最大的动物园之一,有着丰富的动物资源和丰富的文化内涵。
7. 故宫博物院:是中国最著名的博物馆之一,收藏了大量的文物和艺术品,是中国最重要的文化遗产之一。
8. 天坛:是中国古代皇家
```
#### Running on GPU via HF transformers
```python
import torch
from transformers import AutoModelForCausalLM, AutoTokenizer
def generate_prompt(instruction, input=""):
instruction = instruction.strip().replace('\r\n','\n').replace('\n\n','\n')
input = input.strip().replace('\r\n','\n').replace('\n\n','\n')
if input:
return f"""Instruction: {instruction}
Input: {input}
Response:"""
else:
return f"""User: hi
Assistant: Hi. I am your assistant and I will provide expert full response in full details. Please feel free to ask any question and I will always answer it.
User: {instruction}
Assistant:"""
model = AutoModelForCausalLM.from_pretrained("RWKV/v5-Eagle-7B-HF", trust_remote_code=True, torch_dtype=torch.float16).to(0)
tokenizer = AutoTokenizer.from_pretrained("RWKV/v5-Eagle-7B-HF", trust_remote_code=True)
text = "介绍一下大熊猫"
prompt = generate_prompt(text)
inputs = tokenizer(prompt, return_tensors="pt").to(0)
output = model.generate(inputs["input_ids"], max_new_tokens=128, do_sample=True, temperature=1.0, top_p=0.3, top_k=0, )
print(tokenizer.decode(output[0].tolist(), skip_special_tokens=True))
```
output:
```shell
User: hi
Assistant: Hi. I am your assistant and I will provide expert full response in full details. Please feel free to ask any question and I will always answer it.
User: 介绍一下大熊猫
Assistant: 大熊猫是一种中国特有的哺乳动物,也是中国的国宝之一。它们的外貌特征是圆形的黑白相间的身体,有着黑色的毛发和白色的耳朵。大熊猫的食物主要是竹子,它们会在竹林中寻找竹子,并且会将竹子放在竹笼中进行储存。大熊猫的寿命约为20至30年,但由于栖息地的丧失和人类活动的
```
#### Batch Inference
```python
import torch
from transformers import AutoModelForCausalLM, AutoTokenizer
def generate_prompt(instruction, input=""):
instruction = instruction.strip().replace('\r\n', '\n').replace('\n\n', '\n')
input = input.strip().replace('\r\n', '\n').replace('\n\n', '\n')
if input:
return f"""Instruction: {instruction}
Input: {input}
Response:"""
else:
return f"""User: hi
Assistant: Hi. I am your assistant and I will provide expert full response in full details. Please feel free to ask any question and I will always answer it.
User: {instruction}
Assistant:"""
model = AutoModelForCausalLM.from_pretrained("RWKV/v5-Eagle-7B-HF", trust_remote_code=True).to(torch.float32)
tokenizer = AutoTokenizer.from_pretrained("RWKV/v5-Eagle-7B-HF", trust_remote_code=True)
texts = ["请介绍北京的旅游景点", "介绍一下大熊猫", "乌兰察布"]
prompts = [generate_prompt(text) for text in texts]
inputs = tokenizer(prompts, return_tensors="pt", padding=True)
outputs = model.generate(inputs["input_ids"], max_new_tokens=128, do_sample=True, temperature=1.0, top_p=0.3, top_k=0, )
for output in outputs:
print(tokenizer.decode(output.tolist(), skip_special_tokens=True))
```
output:
```shell
User: hi
Assistant: Hi. I am your assistant and I will provide expert full response in full details. Please feel free to ask any question and I will always answer it.
User: 请介绍北京的旅游景点
Assistant: 北京是中国的首都,拥有丰富的旅游资源和历史文化遗产。以下是一些北京的旅游景点:
1. 故宫:位于北京市中心,是明清两代的皇宫,是中国最大的古代宫殿建筑群之一。
2. 天安门广场:位于北京市中心,是中国最著名的城市广场之一,也是中国最大的城市广场。
3. 颐和
User: hi
Assistant: Hi. I am your assistant and I will provide expert full response in full details. Please feel free to ask any question and I will always answer it.
User: 介绍一下大熊猫
Assistant: 大熊猫是一种生活在中国中部地区的哺乳动物,也是中国的国宝之一。它们的外貌特征是圆形的黑白相间的身体,有着黑色的毛发和圆圆的眼睛。大熊猫是一种濒危物种,目前只有在野外的几个保护区才能看到它们的身影。大熊猫的食物主要是竹子,它们会在竹子上寻找食物,并且可以通
User: hi
Assistant: Hi. I am your assistant and I will provide expert full response in full details. Please feel free to ask any question and I will always answer it.
User: 乌兰察布
Assistant: 乌兰察布是中国新疆维吾尔自治区的一个县级市,位于新疆维吾尔自治区中部,是新疆的第二大城市。乌兰察布市是新疆的第一大城市,也是新疆的重要城市之一。乌兰察布市是新疆的经济中心,也是新疆的重要交通枢纽之一。乌兰察布市的人口约为2.5万人,其中汉族占绝大多数。乌
```
## Links
- [Our wiki](https://wiki.rwkv.com)
- [Full eval data](https://docs.google.com/spreadsheets/d/1CBLU6yKkW-8FMvGD4INO3qjeHZ0qkKnZFcM6n6lWNOs/edit#gid=912381775)
- [Recursal.AI Cloud Platform](https://recursal.ai)
- [HF Gradio Demo](https://huggingface.co/spaces/RWKV/v5-EagleX-v2-7B-gradio)
- [Blog article, detailing our model launch](https://blog.rwkv.com/p/eaglex-v2-soaring-past-llama2-7b)
## Acknowledgement
We are grateful for the help and support from the following key groups:
- [Recursal.ai](https://recursal.ai) team for financing the GPU resources, and managing the training of this foundation model - you can run the Eagle line of RWKV models on their cloud / on-premise platform today.
- EleutherAI for their support, especially in the v5/v6 Eagle/Finch paper
- Linux Foundation AI & Data group for supporting and hosting the RWKV project |