File size: 6,985 Bytes
73b5627 071d017 73b5627 071d017 73b5627 071d017 73b5627 |
1 2 3 4 5 6 7 8 9 10 11 12 13 14 15 16 17 18 19 20 21 22 23 24 25 26 27 28 29 30 31 32 33 34 35 36 37 38 39 40 41 42 43 44 45 46 47 48 49 50 51 52 53 54 55 56 57 58 59 60 61 62 63 64 65 66 67 68 69 70 71 72 73 74 75 76 77 78 79 80 81 82 83 84 85 86 87 88 89 90 91 92 93 94 95 96 97 98 99 100 101 102 103 104 105 106 107 108 109 110 111 112 113 114 115 116 117 118 119 120 121 122 123 124 125 126 127 128 129 130 131 132 |
From:
https://huggingface.co/MediaTek-Research/Breeze-7B-32k-Instruct-v1_0
---
pipeline_tag: text-generation
license: apache-2.0
language:
- zh
- en
---
# Model Card for MediaTek Research Breeze-7B-32k-Instruct-v1_0
MediaTek Research Breeze-7B (hereinafter referred to as Breeze-7B) is a language model family that builds on top of [Mistral-7B](https://huggingface.co/mistralai/Mistral-7B-v0.1), specifically intended for Traditional Chinese use.
[Breeze-7B-Base](https://huggingface.co/MediaTek-Research/Breeze-7B-Base-v1_0) is the base model for the Breeze-7B series.
It is suitable for use if you have substantial fine-tuning data to tune it for your specific use case.
[Breeze-7B-Instruct](https://huggingface.co/MediaTek-Research/Breeze-7B-Instruct-v1_0) derives from the base model Breeze-7B-Base, making the resulting model amenable to be used as-is for commonly seen tasks.
[Breeze-7B-32k-Base](https://huggingface.co/MediaTek-Research/Breeze-7B-32k-Base-v1_0) is extended from the base model with more data, base change, and the disabling of the sliding window.
Roughly speaking, that is equivalent to 44k Traditional Chinese characters.
[Breeze-7B-32k-Instruct](https://huggingface.co/MediaTek-Research/Breeze-7B-32k-Instruct-v1_0) derives from the base model Breeze-7B-32k-Base, making the resulting model amenable to be used as-is for commonly seen tasks.
Practicality-wise:
- Breeze-7B-Base expands the original vocabulary with additional 30,000 Traditional Chinese tokens. With the expanded vocabulary, everything else being equal, Breeze-7B operates at twice the inference speed for Traditional Chinese to Mistral-7B and Llama 7B. [See [Inference Performance](#inference-performance).]
- Breeze-7B-Instruct can be used as is for common tasks such as Q&A, RAG, multi-round chat, and summarization.
- Breeze-7B-32k-Instruct can perform tasks at a document level (For Chinese, 20 ~ 40 pages).
*A project by the members (in alphabetical order): Chan-Jan Hsu 許湛然, Feng-Ting Liao 廖峰挺, Po-Chun Hsu 許博竣, Yi-Chang Chen 陳宜昌, and the supervisor Da-Shan Shiu 許大山.*
## Features
- Breeze-7B-32k-Base-v1_0
- Expanding the vocabulary dictionary size from 32k to 62k to better support Traditional Chinese
- 32k-token context length
- Breeze-7B-32k-Instruct-v1_0
- Expanding the vocabulary dictionary size from 32k to 62k to better support Traditional Chinese
- 32k-token context length
- Multi-turn dialogue (without special handling for harmfulness)
## Model Details
- Breeze-7B-32k-Base-v1_0
- Pretrained from: [Breeze-7B-Base](https://huggingface.co/MediaTek-Research/Breeze-7B-Base-v1_0)
- Model type: Causal decoder-only transformer language model
- Language: English and Traditional Chinese (zh-tw)
- Breeze-7B-32k-Instruct-v1_0
- Finetuned from: [Breeze-7B-32k-Base](https://huggingface.co/MediaTek-Research/Breeze-7B-32k-Base-v1_0)
- Model type: Causal decoder-only transformer language model
- Language: English and Traditional Chinese (zh-tw)
## Long-context Performance
#### Needle-in-a-haystack Performance
We use the passkey retrieval task to test the model's ability to attend to different various depths in a given sequence.
A key in placed within a long context distracting document for the model to retrieve.
The key position is binned into 16 bins, and there are 20 testcases for each bin.
Breeze-7B-32k-Base clears the tasks with 90+% accuracy, shown in the figure below.
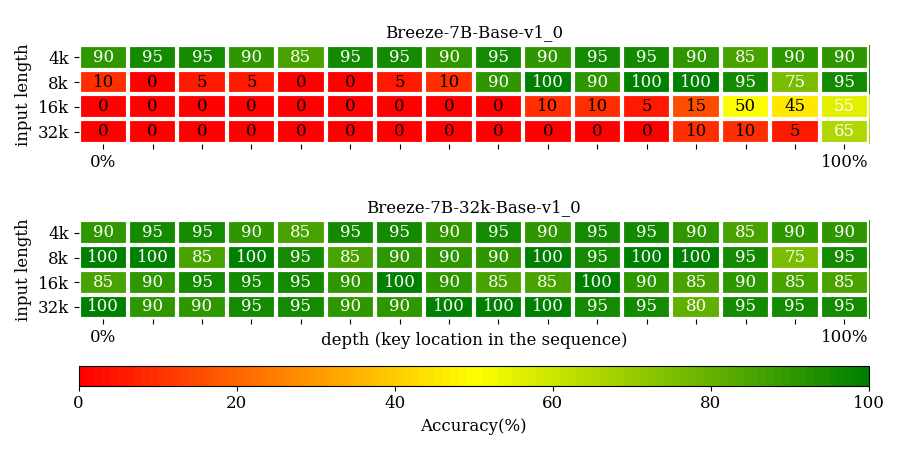
#### Long-DRCD Performance
| **Model/Performance(EM)** | **DRCD** | **DRCD-16k** | **DRCD-32k** |
|---------------------------|----------|--------------|--------------|
| **Breeze-7B-32k-Instruct-v1\_0** | 76.9 | 54.82 | 44.26 |
| **Breeze-7B-32k-Base-v1\_0** | 79.73 | 69.68 | 61.55 |
| **Breeze-7B-Base-v1\_0** | 80.61 | 21.79 | 15.29 |
#### Short-Benchmark Performance
| **Model/Performance(EM)** | **TMMLU+** | **MMLU** | **TABLE** | **MT-Bench-tw** | **MT-Bench** |
|---------------------------|----------|--------------|--------------|-----|-----|
| **Breeze-7B-32k-Instruct-v1\_0** | 41.37 | 61.34 | 34 | 5.8 | 7.4 |
| **Breeze-7B-Instruct-v1\_0** | 42.67 | 62.73 | 39.58 | 6.0 | 7.4 |
## Use in Transformers
First, install direct dependencies:
```
pip install transformers torch accelerate
```
<p style="color:red;">Flash-attention2 is strongly recommended for long context scenarios.</p>
```bash
pip install packaging ninja
pip install flash-attn
```
Then load the model in transformers:
```python
>>> from transformers import AutoModelForCausalLM, AutoTokenizer
>>> tokenizer = AutoTokenizer.from_pretrained("MediaTek-Research/Breeze-7B-32k-Instruct-v1_0/")
>>> model = AutoModelForCausalLM.from_pretrained(
>>> "MediaTek-Research/Breeze-7B-32k-Instruct-v1_0",
... device_map="auto",
... torch_dtype=torch.bfloat16,
... attn_implementation="flash_attention_2"
... )
>>> chat = [
... {"role": "user", "content": "你好,請問你可以完成什麼任務?"},
... {"role": "assistant", "content": "你好,我可以幫助您解決各種問題、提供資訊和協助您完成許多不同的任務。例如:回答技術問題、提供建議、翻譯文字、尋找資料或協助您安排行程等。請告訴我如何能幫助您。"},
... {"role": "user", "content": "太棒了!"},
... ]
>>> tokenizer.apply_chat_template(chat, tokenize=False)
"<s>You are a helpful AI assistant built by MediaTek Research. The user you are helping speaks Traditional Chinese and comes from Taiwan. [INST] 你好,請問你可以完成什麼任務? [/INST] 你好,我可以幫助您解決各種問題、提供資訊和協助您完成許多不同的任務。例如:回答技術問題、提供建議、翻譯文字、尋找資料或協助您安排行程等。請告訴我如何能幫助您。 [INST] 太棒了! [/INST] "
# Tokenized results
# ['▁', '你好', ',', '請問', '你', '可以', '完成', '什麼', '任務', '?']
# ['▁', '你好', ',', '我', '可以', '幫助', '您', '解決', '各種', '問題', '、', '提供', '資訊', '和', '協助', '您', '完成', '許多', '不同', '的', '任務', '。', '例如', ':', '回答', '技術', '問題', '、', '提供', '建議', '、', '翻譯', '文字', '、', '尋找', '資料', '或', '協助', '您', '安排', '行程', '等', '。', '請', '告訴', '我', '如何', '能', '幫助', '您', '。']
# ['▁', '太', '棒', '了', '!']
```
## Citation
```
@article{MediaTek-Research2024breeze7b,
title={Breeze-7B Technical Report},
author={Chan-Jan Hsu and Chang-Le Liu and Feng-Ting Liao and Po-Chun Hsu and Yi-Chang Chen and Da-Shan Shiu},
year={2024},
eprint={2403.02712},
archivePrefix={arXiv},
primaryClass={cs.CL}
}
``` |