File size: 19,152 Bytes
43b7e92 |
1 2 3 4 5 6 7 8 9 10 11 12 13 14 15 16 17 18 19 20 21 22 23 24 25 26 27 28 29 30 31 32 33 34 35 36 37 38 39 40 41 42 43 44 45 46 47 48 49 50 51 52 53 54 55 56 57 58 59 60 61 62 63 64 65 66 67 68 69 70 71 72 73 74 75 76 77 78 79 80 81 82 83 84 85 86 87 88 89 90 91 92 93 94 95 96 97 98 99 100 101 102 103 104 105 106 107 108 109 110 111 112 113 114 115 116 117 118 119 120 121 122 123 124 125 126 127 128 129 130 131 132 133 134 135 136 137 138 139 140 141 142 143 144 145 146 147 148 149 150 151 152 153 154 155 156 157 158 159 160 161 162 163 164 165 166 167 168 169 170 171 172 173 174 175 176 177 178 179 180 181 182 183 184 185 186 187 188 189 190 191 192 193 194 195 196 197 198 199 200 201 202 203 204 205 206 207 208 209 210 211 212 213 214 215 216 217 218 219 220 221 222 223 224 225 226 227 228 229 230 231 232 233 234 235 236 237 238 239 240 241 242 243 244 245 246 247 248 249 250 251 252 253 254 255 256 257 258 259 260 261 262 263 264 265 266 267 268 269 270 271 272 273 274 275 276 277 278 279 280 281 282 283 284 285 286 287 288 289 290 291 292 293 294 295 296 297 298 299 300 301 302 303 304 305 306 307 308 309 310 311 312 313 314 315 316 317 318 319 320 321 322 323 324 325 326 327 328 329 330 331 332 333 334 335 336 337 338 339 340 341 342 343 344 345 346 347 348 349 350 351 352 353 354 355 356 357 358 359 360 361 362 363 364 365 366 367 368 369 370 371 372 373 374 375 376 377 378 379 380 381 382 383 384 385 386 387 388 389 390 391 392 393 394 395 396 397 398 399 400 401 402 403 404 405 406 407 408 409 410 411 412 413 414 415 416 417 418 419 420 421 422 |
<!--Copyright 2024 The HuggingFace Team. All rights reserved.
Licensed under the Apache License, Version 2.0 (the "License"); you may not use this file except in compliance with
the License. You may obtain a copy of the License at
http://www.apache.org/licenses/LICENSE-2.0
Unless required by applicable law or agreed to in writing, software distributed under the License is distributed on
an "AS IS" BASIS, WITHOUT WARRANTIES OR CONDITIONS OF ANY KIND, either express or implied. See the License for the
specific language governing permissions and limitations under the License.
-->
# PyTorch 2.0
π€ Diffusers supports the latest optimizations from [PyTorch 2.0](https://pytorch.org/get-started/pytorch-2.0/) which include:
1. A memory-efficient attention implementation, scaled dot product attention, without requiring any extra dependencies such as xFormers.
2. [`torch.compile`](https://pytorch.org/tutorials/intermediate/torch_compile_tutorial.html), a just-in-time (JIT) compiler to provide an extra performance boost when individual models are compiled.
Both of these optimizations require PyTorch 2.0 or later and π€ Diffusers > 0.13.0.
```bash
pip install --upgrade torch diffusers
```
## Scaled dot product attention
[`torch.nn.functional.scaled_dot_product_attention`](https://pytorch.org/docs/master/generated/torch.nn.functional.scaled_dot_product_attention) (SDPA) is an optimized and memory-efficient attention (similar to xFormers) that automatically enables several other optimizations depending on the model inputs and GPU type. SDPA is enabled by default if you're using PyTorch 2.0 and the latest version of π€ Diffusers, so you don't need to add anything to your code.
However, if you want to explicitly enable it, you can set a [`DiffusionPipeline`] to use [`~models.attention_processor.AttnProcessor2_0`]:
```diff
import torch
from diffusers import DiffusionPipeline
+ from diffusers.models.attention_processor import AttnProcessor2_0
pipe = DiffusionPipeline.from_pretrained("runwayml/stable-diffusion-v1-5", torch_dtype=torch.float16, use_safetensors=True).to("cuda")
+ pipe.unet.set_attn_processor(AttnProcessor2_0())
prompt = "a photo of an astronaut riding a horse on mars"
image = pipe(prompt).images[0]
```
SDPA should be as fast and memory efficient as `xFormers`; check the [benchmark](#benchmark) for more details.
In some cases - such as making the pipeline more deterministic or converting it to other formats - it may be helpful to use the vanilla attention processor, [`~models.attention_processor.AttnProcessor`]. To revert to [`~models.attention_processor.AttnProcessor`], call the [`~UNet2DConditionModel.set_default_attn_processor`] function on the pipeline:
```diff
import torch
from diffusers import DiffusionPipeline
pipe = DiffusionPipeline.from_pretrained("runwayml/stable-diffusion-v1-5", torch_dtype=torch.float16, use_safetensors=True).to("cuda")
+ pipe.unet.set_default_attn_processor()
prompt = "a photo of an astronaut riding a horse on mars"
image = pipe(prompt).images[0]
```
## torch.compile
The `torch.compile` function can often provide an additional speed-up to your PyTorch code. In π€ Diffusers, it is usually best to wrap the UNet with `torch.compile` because it does most of the heavy lifting in the pipeline.
```python
from diffusers import DiffusionPipeline
import torch
pipe = DiffusionPipeline.from_pretrained("runwayml/stable-diffusion-v1-5", torch_dtype=torch.float16, use_safetensors=True).to("cuda")
pipe.unet = torch.compile(pipe.unet, mode="reduce-overhead", fullgraph=True)
images = pipe(prompt, num_inference_steps=steps, num_images_per_prompt=batch_size).images[0]
```
Depending on GPU type, `torch.compile` can provide an *additional speed-up* of **5-300x** on top of SDPA! If you're using more recent GPU architectures such as Ampere (A100, 3090), Ada (4090), and Hopper (H100), `torch.compile` is able to squeeze even more performance out of these GPUs.
Compilation requires some time to complete, so it is best suited for situations where you prepare your pipeline once and then perform the same type of inference operations multiple times. For example, calling the compiled pipeline on a different image size triggers compilation again which can be expensive.
For more information and different options about `torch.compile`, refer to the [`torch_compile`](https://pytorch.org/tutorials/intermediate/torch_compile_tutorial.html) tutorial.
> [!TIP]
> Learn more about other ways PyTorch 2.0 can help optimize your model in the [Accelerate inference of text-to-image diffusion models](../tutorials/fast_diffusion) tutorial.
## Benchmark
We conducted a comprehensive benchmark with PyTorch 2.0's efficient attention implementation and `torch.compile` across different GPUs and batch sizes for five of our most used pipelines. The code is benchmarked on π€ Diffusers v0.17.0.dev0 to optimize `torch.compile` usage (see [here](https://github.com/huggingface/diffusers/pull/3313) for more details).
Expand the dropdown below to find the code used to benchmark each pipeline:
<details>
### Stable Diffusion text-to-image
```python
from diffusers import DiffusionPipeline
import torch
path = "runwayml/stable-diffusion-v1-5"
run_compile = True # Set True / False
pipe = DiffusionPipeline.from_pretrained(path, torch_dtype=torch.float16, use_safetensors=True)
pipe = pipe.to("cuda")
pipe.unet.to(memory_format=torch.channels_last)
if run_compile:
print("Run torch compile")
pipe.unet = torch.compile(pipe.unet, mode="reduce-overhead", fullgraph=True)
prompt = "ghibli style, a fantasy landscape with castles"
for _ in range(3):
images = pipe(prompt=prompt).images
```
### Stable Diffusion image-to-image
```python
from diffusers import StableDiffusionImg2ImgPipeline
from diffusers.utils import load_image
import torch
url = "https://raw.githubusercontent.com/CompVis/stable-diffusion/main/assets/stable-samples/img2img/sketch-mountains-input.jpg"
init_image = load_image(url)
init_image = init_image.resize((512, 512))
path = "runwayml/stable-diffusion-v1-5"
run_compile = True # Set True / False
pipe = StableDiffusionImg2ImgPipeline.from_pretrained(path, torch_dtype=torch.float16, use_safetensors=True)
pipe = pipe.to("cuda")
pipe.unet.to(memory_format=torch.channels_last)
if run_compile:
print("Run torch compile")
pipe.unet = torch.compile(pipe.unet, mode="reduce-overhead", fullgraph=True)
prompt = "ghibli style, a fantasy landscape with castles"
for _ in range(3):
image = pipe(prompt=prompt, image=init_image).images[0]
```
### Stable Diffusion inpainting
```python
from diffusers import StableDiffusionInpaintPipeline
from diffusers.utils import load_image
import torch
img_url = "https://raw.githubusercontent.com/CompVis/latent-diffusion/main/data/inpainting_examples/overture-creations-5sI6fQgYIuo.png"
mask_url = "https://raw.githubusercontent.com/CompVis/latent-diffusion/main/data/inpainting_examples/overture-creations-5sI6fQgYIuo_mask.png"
init_image = load_image(img_url).resize((512, 512))
mask_image = load_image(mask_url).resize((512, 512))
path = "runwayml/stable-diffusion-inpainting"
run_compile = True # Set True / False
pipe = StableDiffusionInpaintPipeline.from_pretrained(path, torch_dtype=torch.float16, use_safetensors=True)
pipe = pipe.to("cuda")
pipe.unet.to(memory_format=torch.channels_last)
if run_compile:
print("Run torch compile")
pipe.unet = torch.compile(pipe.unet, mode="reduce-overhead", fullgraph=True)
prompt = "ghibli style, a fantasy landscape with castles"
for _ in range(3):
image = pipe(prompt=prompt, image=init_image, mask_image=mask_image).images[0]
```
### ControlNet
```python
from diffusers import StableDiffusionControlNetPipeline, ControlNetModel
from diffusers.utils import load_image
import torch
url = "https://raw.githubusercontent.com/CompVis/stable-diffusion/main/assets/stable-samples/img2img/sketch-mountains-input.jpg"
init_image = load_image(url)
init_image = init_image.resize((512, 512))
path = "runwayml/stable-diffusion-v1-5"
run_compile = True # Set True / False
controlnet = ControlNetModel.from_pretrained("lllyasviel/sd-controlnet-canny", torch_dtype=torch.float16, use_safetensors=True)
pipe = StableDiffusionControlNetPipeline.from_pretrained(
path, controlnet=controlnet, torch_dtype=torch.float16, use_safetensors=True
)
pipe = pipe.to("cuda")
pipe.unet.to(memory_format=torch.channels_last)
pipe.controlnet.to(memory_format=torch.channels_last)
if run_compile:
print("Run torch compile")
pipe.unet = torch.compile(pipe.unet, mode="reduce-overhead", fullgraph=True)
pipe.controlnet = torch.compile(pipe.controlnet, mode="reduce-overhead", fullgraph=True)
prompt = "ghibli style, a fantasy landscape with castles"
for _ in range(3):
image = pipe(prompt=prompt, image=init_image).images[0]
```
### DeepFloyd IF text-to-image + upscaling
```python
from diffusers import DiffusionPipeline
import torch
run_compile = True # Set True / False
pipe_1 = DiffusionPipeline.from_pretrained("DeepFloyd/IF-I-M-v1.0", variant="fp16", text_encoder=None, torch_dtype=torch.float16, use_safetensors=True)
pipe_1.to("cuda")
pipe_2 = DiffusionPipeline.from_pretrained("DeepFloyd/IF-II-M-v1.0", variant="fp16", text_encoder=None, torch_dtype=torch.float16, use_safetensors=True)
pipe_2.to("cuda")
pipe_3 = DiffusionPipeline.from_pretrained("stabilityai/stable-diffusion-x4-upscaler", torch_dtype=torch.float16, use_safetensors=True)
pipe_3.to("cuda")
pipe_1.unet.to(memory_format=torch.channels_last)
pipe_2.unet.to(memory_format=torch.channels_last)
pipe_3.unet.to(memory_format=torch.channels_last)
if run_compile:
pipe_1.unet = torch.compile(pipe_1.unet, mode="reduce-overhead", fullgraph=True)
pipe_2.unet = torch.compile(pipe_2.unet, mode="reduce-overhead", fullgraph=True)
pipe_3.unet = torch.compile(pipe_3.unet, mode="reduce-overhead", fullgraph=True)
prompt = "the blue hulk"
prompt_embeds = torch.randn((1, 2, 4096), dtype=torch.float16)
neg_prompt_embeds = torch.randn((1, 2, 4096), dtype=torch.float16)
for _ in range(3):
image_1 = pipe_1(prompt_embeds=prompt_embeds, negative_prompt_embeds=neg_prompt_embeds, output_type="pt").images
image_2 = pipe_2(image=image_1, prompt_embeds=prompt_embeds, negative_prompt_embeds=neg_prompt_embeds, output_type="pt").images
image_3 = pipe_3(prompt=prompt, image=image_1, noise_level=100).images
```
</details>
The graph below highlights the relative speed-ups for the [`StableDiffusionPipeline`] across five GPU families with PyTorch 2.0 and `torch.compile` enabled. The benchmarks for the following graphs are measured in *number of iterations/second*.
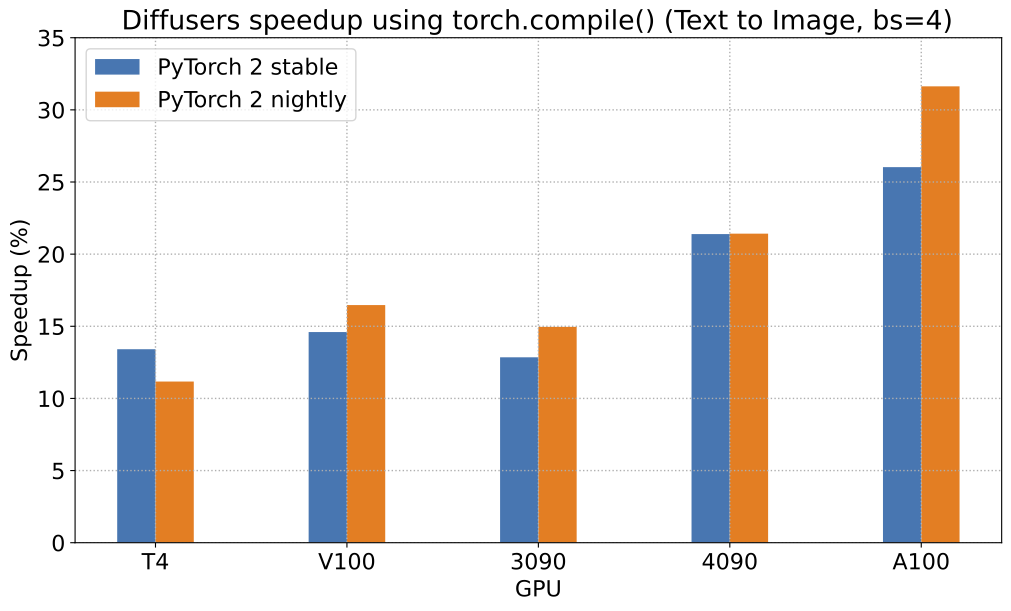
To give you an even better idea of how this speed-up holds for the other pipelines, consider the following
graph for an A100 with PyTorch 2.0 and `torch.compile`:
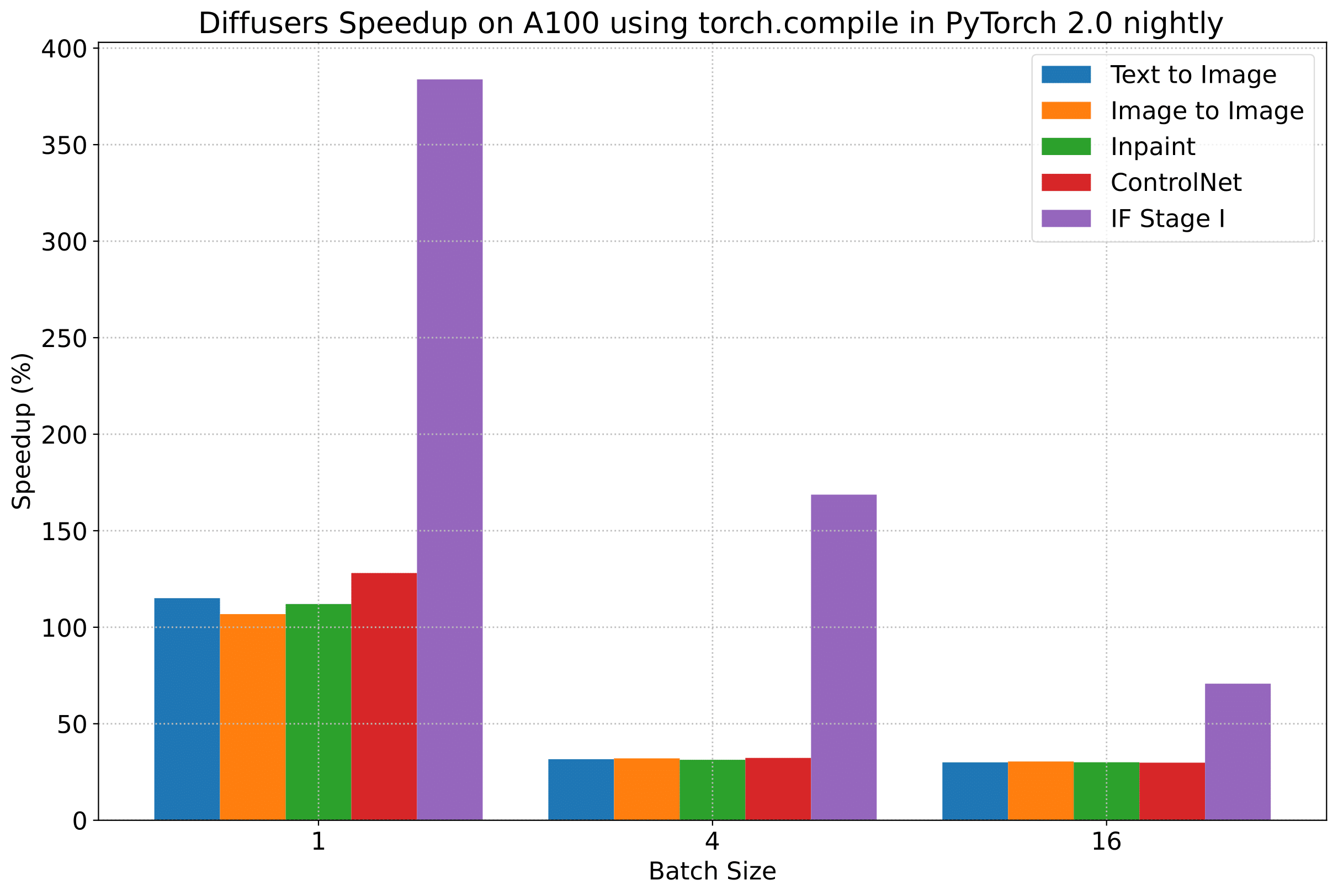
In the following tables, we report our findings in terms of the *number of iterations/second*.
### A100 (batch size: 1)
| **Pipeline** | **torch 2.0 - <br>no compile** | **torch nightly - <br>no compile** | **torch 2.0 - <br>compile** | **torch nightly - <br>compile** |
|:---:|:---:|:---:|:---:|:---:|
| SD - txt2img | 21.66 | 23.13 | 44.03 | 49.74 |
| SD - img2img | 21.81 | 22.40 | 43.92 | 46.32 |
| SD - inpaint | 22.24 | 23.23 | 43.76 | 49.25 |
| SD - controlnet | 15.02 | 15.82 | 32.13 | 36.08 |
| IF | 20.21 / <br>13.84 / <br>24.00 | 20.12 / <br>13.70 / <br>24.03 | β | 97.34 / <br>27.23 / <br>111.66 |
| SDXL - txt2img | 8.64 | 9.9 | - | - |
### A100 (batch size: 4)
| **Pipeline** | **torch 2.0 - <br>no compile** | **torch nightly - <br>no compile** | **torch 2.0 - <br>compile** | **torch nightly - <br>compile** |
|:---:|:---:|:---:|:---:|:---:|
| SD - txt2img | 11.6 | 13.12 | 14.62 | 17.27 |
| SD - img2img | 11.47 | 13.06 | 14.66 | 17.25 |
| SD - inpaint | 11.67 | 13.31 | 14.88 | 17.48 |
| SD - controlnet | 8.28 | 9.38 | 10.51 | 12.41 |
| IF | 25.02 | 18.04 | β | 48.47 |
| SDXL - txt2img | 2.44 | 2.74 | - | - |
### A100 (batch size: 16)
| **Pipeline** | **torch 2.0 - <br>no compile** | **torch nightly - <br>no compile** | **torch 2.0 - <br>compile** | **torch nightly - <br>compile** |
|:---:|:---:|:---:|:---:|:---:|
| SD - txt2img | 3.04 | 3.6 | 3.83 | 4.68 |
| SD - img2img | 2.98 | 3.58 | 3.83 | 4.67 |
| SD - inpaint | 3.04 | 3.66 | 3.9 | 4.76 |
| SD - controlnet | 2.15 | 2.58 | 2.74 | 3.35 |
| IF | 8.78 | 9.82 | β | 16.77 |
| SDXL - txt2img | 0.64 | 0.72 | - | - |
### V100 (batch size: 1)
| **Pipeline** | **torch 2.0 - <br>no compile** | **torch nightly - <br>no compile** | **torch 2.0 - <br>compile** | **torch nightly - <br>compile** |
|:---:|:---:|:---:|:---:|:---:|
| SD - txt2img | 18.99 | 19.14 | 20.95 | 22.17 |
| SD - img2img | 18.56 | 19.18 | 20.95 | 22.11 |
| SD - inpaint | 19.14 | 19.06 | 21.08 | 22.20 |
| SD - controlnet | 13.48 | 13.93 | 15.18 | 15.88 |
| IF | 20.01 / <br>9.08 / <br>23.34 | 19.79 / <br>8.98 / <br>24.10 | β | 55.75 / <br>11.57 / <br>57.67 |
### V100 (batch size: 4)
| **Pipeline** | **torch 2.0 - <br>no compile** | **torch nightly - <br>no compile** | **torch 2.0 - <br>compile** | **torch nightly - <br>compile** |
|:---:|:---:|:---:|:---:|:---:|
| SD - txt2img | 5.96 | 5.89 | 6.83 | 6.86 |
| SD - img2img | 5.90 | 5.91 | 6.81 | 6.82 |
| SD - inpaint | 5.99 | 6.03 | 6.93 | 6.95 |
| SD - controlnet | 4.26 | 4.29 | 4.92 | 4.93 |
| IF | 15.41 | 14.76 | β | 22.95 |
### V100 (batch size: 16)
| **Pipeline** | **torch 2.0 - <br>no compile** | **torch nightly - <br>no compile** | **torch 2.0 - <br>compile** | **torch nightly - <br>compile** |
|:---:|:---:|:---:|:---:|:---:|
| SD - txt2img | 1.66 | 1.66 | 1.92 | 1.90 |
| SD - img2img | 1.65 | 1.65 | 1.91 | 1.89 |
| SD - inpaint | 1.69 | 1.69 | 1.95 | 1.93 |
| SD - controlnet | 1.19 | 1.19 | OOM after warmup | 1.36 |
| IF | 5.43 | 5.29 | β | 7.06 |
### T4 (batch size: 1)
| **Pipeline** | **torch 2.0 - <br>no compile** | **torch nightly - <br>no compile** | **torch 2.0 - <br>compile** | **torch nightly - <br>compile** |
|:---:|:---:|:---:|:---:|:---:|
| SD - txt2img | 6.9 | 6.95 | 7.3 | 7.56 |
| SD - img2img | 6.84 | 6.99 | 7.04 | 7.55 |
| SD - inpaint | 6.91 | 6.7 | 7.01 | 7.37 |
| SD - controlnet | 4.89 | 4.86 | 5.35 | 5.48 |
| IF | 17.42 / <br>2.47 / <br>18.52 | 16.96 / <br>2.45 / <br>18.69 | β | 24.63 / <br>2.47 / <br>23.39 |
| SDXL - txt2img | 1.15 | 1.16 | - | - |
### T4 (batch size: 4)
| **Pipeline** | **torch 2.0 - <br>no compile** | **torch nightly - <br>no compile** | **torch 2.0 - <br>compile** | **torch nightly - <br>compile** |
|:---:|:---:|:---:|:---:|:---:|
| SD - txt2img | 1.79 | 1.79 | 2.03 | 1.99 |
| SD - img2img | 1.77 | 1.77 | 2.05 | 2.04 |
| SD - inpaint | 1.81 | 1.82 | 2.09 | 2.09 |
| SD - controlnet | 1.34 | 1.27 | 1.47 | 1.46 |
| IF | 5.79 | 5.61 | β | 7.39 |
| SDXL - txt2img | 0.288 | 0.289 | - | - |
### T4 (batch size: 16)
| **Pipeline** | **torch 2.0 - <br>no compile** | **torch nightly - <br>no compile** | **torch 2.0 - <br>compile** | **torch nightly - <br>compile** |
|:---:|:---:|:---:|:---:|:---:|
| SD - txt2img | 2.34s | 2.30s | OOM after 2nd iteration | 1.99s |
| SD - img2img | 2.35s | 2.31s | OOM after warmup | 2.00s |
| SD - inpaint | 2.30s | 2.26s | OOM after 2nd iteration | 1.95s |
| SD - controlnet | OOM after 2nd iteration | OOM after 2nd iteration | OOM after warmup | OOM after warmup |
| IF * | 1.44 | 1.44 | β | 1.94 |
| SDXL - txt2img | OOM | OOM | - | - |
### RTX 3090 (batch size: 1)
| **Pipeline** | **torch 2.0 - <br>no compile** | **torch nightly - <br>no compile** | **torch 2.0 - <br>compile** | **torch nightly - <br>compile** |
|:---:|:---:|:---:|:---:|:---:|
| SD - txt2img | 22.56 | 22.84 | 23.84 | 25.69 |
| SD - img2img | 22.25 | 22.61 | 24.1 | 25.83 |
| SD - inpaint | 22.22 | 22.54 | 24.26 | 26.02 |
| SD - controlnet | 16.03 | 16.33 | 17.38 | 18.56 |
| IF | 27.08 / <br>9.07 / <br>31.23 | 26.75 / <br>8.92 / <br>31.47 | β | 68.08 / <br>11.16 / <br>65.29 |
### RTX 3090 (batch size: 4)
| **Pipeline** | **torch 2.0 - <br>no compile** | **torch nightly - <br>no compile** | **torch 2.0 - <br>compile** | **torch nightly - <br>compile** |
|:---:|:---:|:---:|:---:|:---:|
| SD - txt2img | 6.46 | 6.35 | 7.29 | 7.3 |
| SD - img2img | 6.33 | 6.27 | 7.31 | 7.26 |
| SD - inpaint | 6.47 | 6.4 | 7.44 | 7.39 |
| SD - controlnet | 4.59 | 4.54 | 5.27 | 5.26 |
| IF | 16.81 | 16.62 | β | 21.57 |
### RTX 3090 (batch size: 16)
| **Pipeline** | **torch 2.0 - <br>no compile** | **torch nightly - <br>no compile** | **torch 2.0 - <br>compile** | **torch nightly - <br>compile** |
|:---:|:---:|:---:|:---:|:---:|
| SD - txt2img | 1.7 | 1.69 | 1.93 | 1.91 |
| SD - img2img | 1.68 | 1.67 | 1.93 | 1.9 |
| SD - inpaint | 1.72 | 1.71 | 1.97 | 1.94 |
| SD - controlnet | 1.23 | 1.22 | 1.4 | 1.38 |
| IF | 5.01 | 5.00 | β | 6.33 |
### RTX 4090 (batch size: 1)
| **Pipeline** | **torch 2.0 - <br>no compile** | **torch nightly - <br>no compile** | **torch 2.0 - <br>compile** | **torch nightly - <br>compile** |
|:---:|:---:|:---:|:---:|:---:|
| SD - txt2img | 40.5 | 41.89 | 44.65 | 49.81 |
| SD - img2img | 40.39 | 41.95 | 44.46 | 49.8 |
| SD - inpaint | 40.51 | 41.88 | 44.58 | 49.72 |
| SD - controlnet | 29.27 | 30.29 | 32.26 | 36.03 |
| IF | 69.71 / <br>18.78 / <br>85.49 | 69.13 / <br>18.80 / <br>85.56 | β | 124.60 / <br>26.37 / <br>138.79 |
| SDXL - txt2img | 6.8 | 8.18 | - | - |
### RTX 4090 (batch size: 4)
| **Pipeline** | **torch 2.0 - <br>no compile** | **torch nightly - <br>no compile** | **torch 2.0 - <br>compile** | **torch nightly - <br>compile** |
|:---:|:---:|:---:|:---:|:---:|
| SD - txt2img | 12.62 | 12.84 | 15.32 | 15.59 |
| SD - img2img | 12.61 | 12,.79 | 15.35 | 15.66 |
| SD - inpaint | 12.65 | 12.81 | 15.3 | 15.58 |
| SD - controlnet | 9.1 | 9.25 | 11.03 | 11.22 |
| IF | 31.88 | 31.14 | β | 43.92 |
| SDXL - txt2img | 2.19 | 2.35 | - | - |
### RTX 4090 (batch size: 16)
| **Pipeline** | **torch 2.0 - <br>no compile** | **torch nightly - <br>no compile** | **torch 2.0 - <br>compile** | **torch nightly - <br>compile** |
|:---:|:---:|:---:|:---:|:---:|
| SD - txt2img | 3.17 | 3.2 | 3.84 | 3.85 |
| SD - img2img | 3.16 | 3.2 | 3.84 | 3.85 |
| SD - inpaint | 3.17 | 3.2 | 3.85 | 3.85 |
| SD - controlnet | 2.23 | 2.3 | 2.7 | 2.75 |
| IF | 9.26 | 9.2 | β | 13.31 |
| SDXL - txt2img | 0.52 | 0.53 | - | - |
## Notes
* Follow this [PR](https://github.com/huggingface/diffusers/pull/3313) for more details on the environment used for conducting the benchmarks.
* For the DeepFloyd IF pipeline where batch sizes > 1, we only used a batch size of > 1 in the first IF pipeline for text-to-image generation and NOT for upscaling. That means the two upscaling pipelines received a batch size of 1.
*Thanks to [Horace He](https://github.com/Chillee) from the PyTorch team for their support in improving our support of `torch.compile()` in Diffusers.*
|