A newer version of the Gradio SDK is available:
5.3.0
Image Segmentation Using Text and Image Prompts
This repository contains the code used in the paper "Image Segmentation Using Text and Image Prompts".
The Paper has been accepted to CVPR 2022!
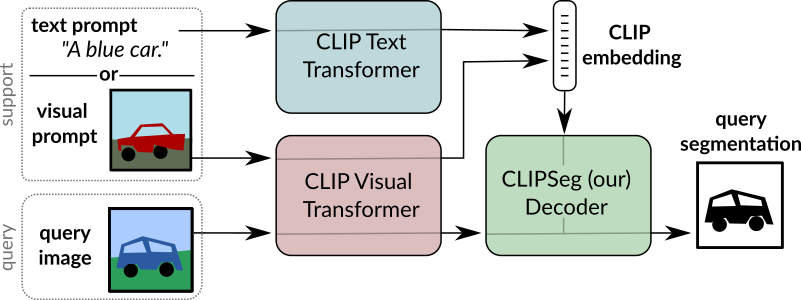
The systems allows to create segmentation models without training based on:
- An arbitrary text query
- Or an image with a mask highlighting stuff or an object.
Quick Start
In the Quickstart.ipynb
notebook we provide the code for using a pre-trained CLIPSeg model. If you run the notebook locally, make sure you downloaded the rd64-uni.pth
weights, either manually or via git lfs extension.
It can also be used interactively using MyBinder
(please note that the VM does not use a GPU, thus inference takes a few seconds).
Dependencies
This code base depends on pytorch, torchvision and clip (pip install git+https://github.com/openai/CLIP.git
).
Additional dependencies are hidden for double blind review.
Datasets
PhraseCut
andPhraseCutPlus
: Referring expression datasetPFEPascalWrapper
: Wrapper class for PFENet's Pascal-5i implementationPascalZeroShot
: Wrapper class for PascalZeroShotCOCOWrapper
: Wrapper class for COCO.
Models
CLIPDensePredT
: CLIPSeg model with transformer-based decoder.ViTDensePredT
: CLIPSeg model with transformer-based decoder.
Third Party Dependencies
For some of the datasets third party dependencies are required. Run the following commands in the third_party
folder.
git clone https://github.com/cvlab-yonsei/JoEm
git clone https://github.com/Jia-Research-Lab/PFENet.git
git clone https://github.com/ChenyunWu/PhraseCutDataset.git
git clone https://github.com/juhongm999/hsnet.git
Weights
The MIT license does not apply to these weights.
We provide two model weights, for D=64 (4.1MB) and D=16 (1.1MB).
wget https://owncloud.gwdg.de/index.php/s/ioHbRzFx6th32hn/download -O weights.zip
unzip -d weights -j weights.zip
Training and Evaluation
To train use the training.py
script with experiment file and experiment id parameters. E.g. python training.py phrasecut.yaml 0
will train the first phrasecut experiment which is defined by the configuration
and first individual_configurations
parameters. Model weights will be written in logs/
.
For evaluation use score.py
. E.g. python score.py phrasecut.yaml 0 0
will train the first phrasecut experiment of test_configuration
and the first configuration in individual_configurations
.
Usage of PFENet Wrappers
In order to use the dataset and model wrappers for PFENet, the PFENet repository needs to be cloned to the root folder.
git clone https://github.com/Jia-Research-Lab/PFENet.git
License
The source code files in this repository (excluding model weights) are released under MIT license.
Citation
@InProceedings{lueddecke22_cvpr,
author = {L\"uddecke, Timo and Ecker, Alexander},
title = {Image Segmentation Using Text and Image Prompts},
booktitle = {Proceedings of the IEEE/CVF Conference on Computer Vision and Pattern Recognition (CVPR)},
month = {June},
year = {2022},
pages = {7086-7096}
}