Spaces:
Build error
Build error
File size: 18,698 Bytes
c145e8a |
1 2 3 4 5 6 7 8 9 10 11 12 13 14 15 16 17 18 19 20 21 22 23 24 25 26 27 28 29 30 31 32 33 34 35 36 37 38 39 40 41 42 43 44 45 46 47 48 49 50 51 52 53 54 55 56 57 58 59 60 61 62 63 64 65 66 67 68 69 70 71 72 73 74 75 76 77 78 79 80 81 82 83 84 85 86 87 88 89 90 91 92 93 94 95 96 97 98 99 100 101 102 103 104 105 106 107 108 109 110 111 112 113 114 115 116 117 118 119 120 121 122 123 124 125 126 127 128 129 130 131 132 133 134 135 136 137 138 139 140 141 142 143 144 145 146 147 148 149 150 151 152 153 154 155 156 157 158 159 160 161 162 163 164 165 166 167 168 169 170 171 172 173 174 175 176 177 178 179 180 181 182 183 184 185 186 187 188 189 190 191 192 193 194 195 196 197 198 199 200 201 202 203 204 205 206 207 208 209 210 211 212 213 214 215 216 217 218 219 220 221 222 223 224 225 226 227 228 229 230 231 232 233 234 235 236 237 238 239 240 241 242 243 244 245 246 247 248 249 250 251 252 253 254 255 256 257 258 259 260 261 262 263 264 265 266 267 268 269 270 271 272 273 274 275 276 277 278 279 280 281 282 283 284 285 286 287 288 289 290 291 292 293 294 295 296 297 298 299 300 301 302 303 304 305 306 307 308 309 310 311 312 313 314 315 316 317 318 319 320 321 322 323 324 325 326 327 328 329 330 331 332 333 334 335 336 337 338 339 340 341 342 343 344 345 346 347 348 349 350 351 352 353 354 355 356 357 358 359 360 361 362 363 364 365 366 367 368 369 370 371 372 373 374 375 376 377 378 379 380 381 382 383 384 385 386 387 388 389 390 391 392 393 394 395 396 397 398 399 400 401 402 403 404 405 |
import os,sys
# install environment goods
#os.system("pip -q install dgl -f https://data.dgl.ai/wheels/cu113/repo.html")
os.system('pip install dgl==1.0.2+cu116 -f https://data.dgl.ai/wheels/cu116/repo.html')
#os.system('pip install gradio')
os.environ["DGLBACKEND"] = "pytorch"
#os.system(f'pip install -r ./PROTEIN_GENERATOR/requirements.txt')
print('Modules installed')
os.system('pip install --force gradio==3.28.3')
os.environ["DGLBACKEND"] = "pytorch"
if not os.path.exists('./SEQDIFF_230205_dssp_hotspots_25mask_EQtasks_mod30.pt'):
print('Downloading model weights 1')
os.system('wget http://files.ipd.uw.edu/pub/sequence_diffusion/checkpoints/SEQDIFF_230205_dssp_hotspots_25mask_EQtasks_mod30.pt')
print('Successfully Downloaded')
if not os.path.exists('./SEQDIFF_221219_equalTASKS_nostrSELFCOND_mod30.pt'):
print('Downloading model weights 2')
os.system('wget http://files.ipd.uw.edu/pub/sequence_diffusion/checkpoints/SEQDIFF_221219_equalTASKS_nostrSELFCOND_mod30.pt')
print('Successfully Downloaded')
import numpy as np
import gradio as gr
import py3Dmol
from io import StringIO
import json
import secrets
import copy
import matplotlib.pyplot as plt
from utils.sampler import HuggingFace_sampler
plt.rcParams.update({'font.size': 13})
with open('./tmp/args.json','r') as f:
args = json.load(f)
# manually set checkpoint to load
args['checkpoint'] = None
args['dump_trb'] = False
args['dump_args'] = True
args['save_best_plddt'] = True
args['T'] = 25
args['strand_bias'] = 0.0
args['loop_bias'] = 0.0
args['helix_bias'] = 0.0
def protein_diffusion_model(sequence, seq_len, helix_bias, strand_bias, loop_bias,
secondary_structure, aa_bias, aa_bias_potential,
#target_charge, target_ph, charge_potential,
num_steps, noise, hydrophobic_target_score, hydrophobic_potential):
dssp_checkpoint = './SEQDIFF_230205_dssp_hotspots_25mask_EQtasks_mod30.pt'
og_checkpoint = './SEQDIFF_221219_equalTASKS_nostrSELFCOND_mod30.pt'
model_args = copy.deepcopy(args)
# make sampler
S = HuggingFace_sampler(args=model_args)
# get random prefix
S.out_prefix = './tmp/'+secrets.token_hex(nbytes=10).upper()
# set args
S.args['checkpoint'] = None
S.args['dump_trb'] = False
S.args['dump_args'] = True
S.args['save_best_plddt'] = True
S.args['T'] = 20
S.args['strand_bias'] = 0.0
S.args['loop_bias'] = 0.0
S.args['helix_bias'] = 0.0
S.args['potentials'] = None
S.args['potential_scale'] = None
S.args['aa_composition'] = None
# get sequence if entered and make sure all chars are valid
alt_aa_dict = {'B':['D','N'],'J':['I','L'],'U':['C'],'Z':['E','Q'],'O':['K']}
if sequence not in ['',None]:
L = len(sequence)
aa_seq = []
for aa in sequence.upper():
if aa in alt_aa_dict.keys():
aa_seq.append(np.random.choice(alt_aa_dict[aa]))
else:
aa_seq.append(aa)
S.args['sequence'] = aa_seq
else:
S.args['contigs'] = [f'{seq_len}']
L = int(seq_len)
if secondary_structure in ['',None]:
secondary_structure = None
else:
secondary_structure = ''.join(['E' if x == 'S' else x for x in secondary_structure])
if L < len(secondary_structure):
secondary_structure = secondary_structure[:len(sequence)]
elif L == len(secondary_structure):
pass
else:
dseq = L - len(secondary_structure)
secondary_structure += secondary_structure[-1]*dseq
# potentials
potential_list = []
potential_bias_list = []
if aa_bias not in ['',None]:
potential_list.append('aa_bias')
S.args['aa_composition'] = aa_bias
if aa_bias_potential in ['',None]:
aa_bias_potential = 3
potential_bias_list.append(str(aa_bias_potential))
'''
if target_charge not in ['',None]:
potential_list.append('charge')
if charge_potential in ['',None]:
charge_potential = 1
potential_bias_list.append(str(charge_potential))
S.args['target_charge'] = float(target_charge)
if target_ph in ['',None]:
target_ph = 7.4
S.args['target_pH'] = float(target_ph)
'''
if hydrophobic_target_score not in ['',None]:
potential_list.append('hydrophobic')
S.args['hydrophobic_score'] = float(hydrophobic_target_score)
if hydrophobic_potential in ['',None]:
hydrophobic_potential = 3
potential_bias_list.append(str(hydrophobic_potential))
if len(potential_list) > 0:
S.args['potentials'] = ','.join(potential_list)
S.args['potential_scale'] = ','.join(potential_bias_list)
# normalise secondary_structure bias from range 0-0.3
S.args['secondary_structure'] = secondary_structure
S.args['helix_bias'] = helix_bias
S.args['strand_bias'] = strand_bias
S.args['loop_bias'] = loop_bias
# set T
if num_steps in ['',None]:
S.args['T'] = 20
else:
S.args['T'] = int(num_steps)
# noise
if 'normal' in noise:
S.args['sample_distribution'] = noise
S.args['sample_distribution_gmm_means'] = [0]
S.args['sample_distribution_gmm_variances'] = [1]
elif 'gmm2' in noise:
S.args['sample_distribution'] = noise
S.args['sample_distribution_gmm_means'] = [-1,1]
S.args['sample_distribution_gmm_variances'] = [1,1]
elif 'gmm3' in noise:
S.args['sample_distribution'] = noise
S.args['sample_distribution_gmm_means'] = [-1,0,1]
S.args['sample_distribution_gmm_variances'] = [1,1,1]
if secondary_structure not in ['',None] or helix_bias+strand_bias+loop_bias > 0:
S.args['checkpoint'] = dssp_checkpoint
S.args['d_t1d'] = 29
print('using dssp checkpoint')
else:
S.args['checkpoint'] = og_checkpoint
S.args['d_t1d'] = 24
print('using og checkpoint')
for k,v in S.args.items():
print(f"{k} --> {v}")
# init S
S.model_init()
S.diffuser_init()
S.setup()
# sampling loop
plddt_data = []
for j in range(S.max_t):
output_seq, output_pdb, plddt = S.take_step_get_outputs(j)
plddt_data.append(plddt)
yield output_seq, output_pdb, display_pdb(output_pdb), get_plddt_plot(plddt_data, S.max_t)
output_seq, output_pdb, plddt = S.get_outputs()
yield output_seq, output_pdb, display_pdb(output_pdb), get_plddt_plot(plddt_data, S.max_t)
def get_plddt_plot(plddt_data, max_t):
x = [i+1 for i in range(len(plddt_data))]
fig, ax = plt.subplots(figsize=(15,6))
ax.plot(x,plddt_data,color='#661dbf', linewidth=3,marker='o')
ax.set_xticks([i+1 for i in range(max_t)])
ax.set_yticks([(i+1)/10 for i in range(10)])
ax.set_ylim([0,1])
ax.set_ylabel('model confidence (plddt)')
ax.set_xlabel('diffusion steps (t)')
return fig
def display_pdb(path_to_pdb):
'''
#function to display pdb in py3dmol
'''
pdb = open(path_to_pdb, "r").read()
view = py3Dmol.view(width=500, height=500)
view.addModel(pdb, "pdb")
view.setStyle({'model': -1}, {"cartoon": {'colorscheme':{'prop':'b','gradient':'roygb','min':0,'max':1}}})#'linear', 'min': 0, 'max': 1, 'colors': ["#ff9ef0","#a903fc",]}}})
view.zoomTo()
output = view._make_html().replace("'", '"')
print(view._make_html())
x = f"""<!DOCTYPE html><html></center> {output} </center></html>""" # do not use ' in this input
return f"""<iframe height="500px" width="100%" name="result" allow="midi; geolocation; microphone; camera;
display-capture; encrypted-media;" sandbox="allow-modals allow-forms
allow-scripts allow-same-origin allow-popups
allow-top-navigation-by-user-activation allow-downloads" allowfullscreen=""
allowpaymentrequest="" frameborder="0" srcdoc='{x}'></iframe>"""
'''
return f"""<iframe style="width: 100%; height:700px" name="result" allow="midi; geolocation; microphone; camera;
display-capture; encrypted-media;" sandbox="allow-modals allow-forms
allow-scripts allow-same-origin allow-popups
allow-top-navigation-by-user-activation allow-downloads" allowfullscreen=""
allowpaymentrequest="" frameborder="0" srcdoc='{x}'></iframe>"""
'''
def toggle_seq_input(choice):
if choice == "protein length":
return gr.update(visible=True, value=None), gr.update(visible=False, value=None)
elif choice == "custom sequence":
return gr.update(visible=False, value=None), gr.update(visible=True, value=None)
def toggle_secondary_structure(choice):
if choice == "sliders":
return gr.update(visible=True, value=None),gr.update(visible=True, value=None),gr.update(visible=True, value=None),gr.update(visible=False, value=None)
elif choice == "explicit":
return gr.update(visible=False, value=None),gr.update(visible=False, value=None),gr.update(visible=False, value=None),gr.update(visible=True, value=None)
# Define the Gradio interface
with gr.Blocks(theme='ParityError/Interstellar') as demo:
gr.Markdown(f"""# Protein Generation via Diffusion in Sequence Space""")
with gr.Row():
with gr.Column(min_width=500):
gr.Markdown(f"""
## How does it work?\n
--- [PREPRINT](https://biorxiv.org/content/10.1101/2023.05.08.539766v1) ---
Protein sequence and structure co-generation is a long outstanding problem in the field of protein design. By implementing [ddpm](https://arxiv.org/abs/2006.11239) style diffusion over protein seqeuence space we generate protein sequence and structure pairs. Starting with [RoseTTAFold](https://www.science.org/doi/10.1126/science.abj8754), a protein structure prediction network, we finetuned it to predict sequence and structure given a partially noised sequence. By applying losses to both the predicted sequence and structure the model is forced to generate meaningful pairs. Diffusing in sequence space makes it easy to implement potentials to guide the diffusive process toward particular amino acid composition, net charge, and more! Furthermore, you can sample proteins from a family of sequences or even train a small sequence to function classifier to guide generation toward desired sequences.
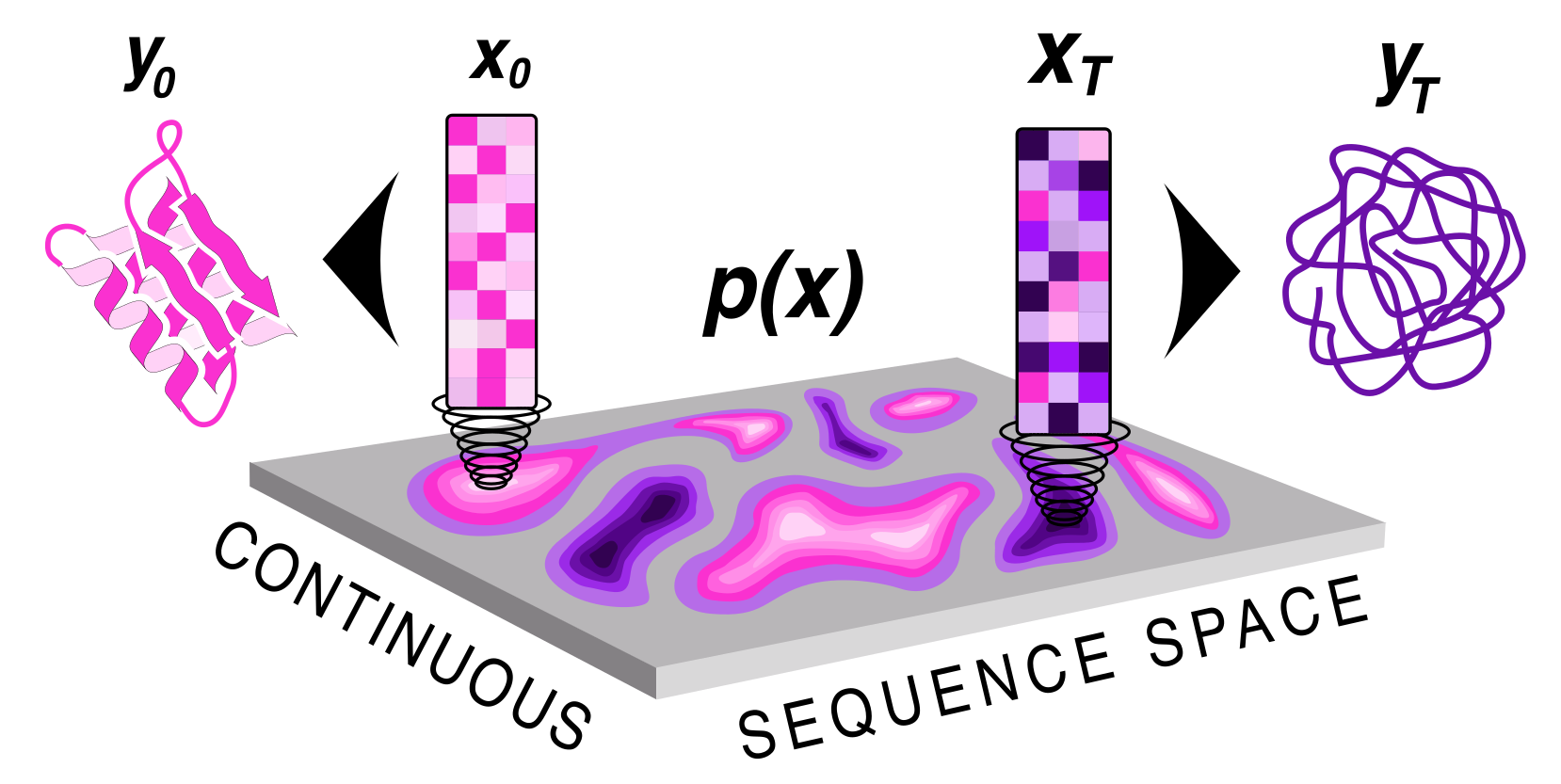
## How to use it?\n
A user can either design a custom input sequence to diffuse from or specify a length below. To scaffold a sequence use the following format where X represent residues to diffuse: XXXXXXXXSCIENCESCIENCEXXXXXXXXXXXXXXXXXXX. You can even design a protein with your name XXXXXXXXXXXXNAMEHEREXXXXXXXXXXXXX!
### Acknowledgements\n
Thank you to Simon Dürr and the Hugging Face team for setting us up with a community GPU grant!
""")
gr.Markdown("""
## Model in Action
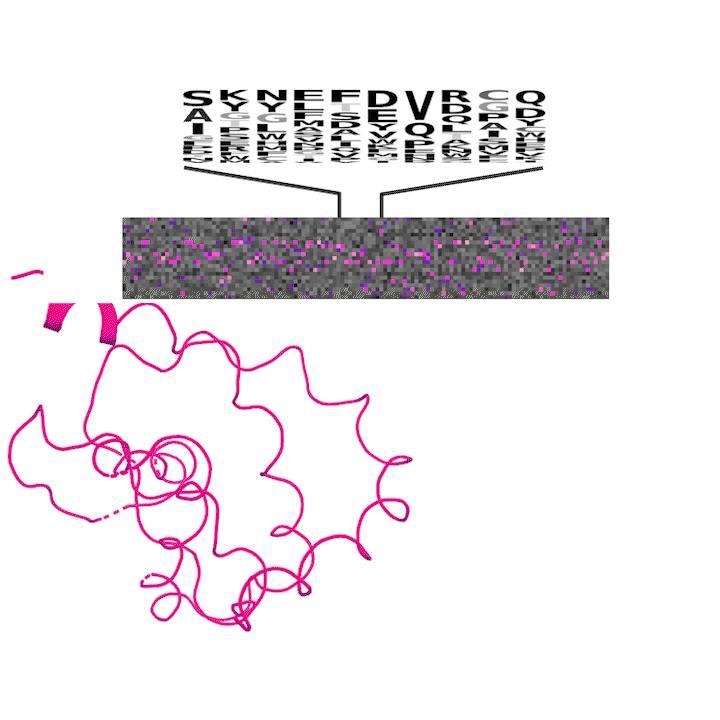
""")
with gr.Row().style(equal_height=False):
with gr.Column():
gr.Markdown("""## INPUTS""")
gr.Markdown("""#### Start Sequence
Specify the protein length for complete unconditional generation, or scaffold a motif (or your name) using the custom sequence input""")
seq_opt = gr.Radio(["protein length","custom sequence"], label="How would you like to specify the starting sequence?", value='protein length')
sequence = gr.Textbox(label="custom sequence", lines=1, placeholder='AMINO ACIDS: A,C,D,E,F,G,H,I,K,L,M,N,P,Q,R,S,T,V,W,Y\n MASK TOKEN: X', visible=False)
seq_len = gr.Slider(minimum=5.0, maximum=250.0, label="protein length", value=100, visible=True)
seq_opt.change(fn=toggle_seq_input,
inputs=[seq_opt],
outputs=[seq_len, sequence],
queue=False)
gr.Markdown("""### Optional Parameters""")
with gr.Accordion(label='Secondary Structure',open=True):
gr.Markdown("""Try changing the sliders or inputing explicit secondary structure conditioning for each residue""")
sec_str_opt = gr.Radio(["sliders","explicit"], label="How would you like to specify secondary structure?", value='sliders')
secondary_structure = gr.Textbox(label="secondary structure", lines=1, placeholder='HELIX = H STRAND = S LOOP = L MASK = X(must be the same length as input sequence)', visible=False)
with gr.Column():
helix_bias = gr.Slider(minimum=0.0, maximum=0.05, label="helix bias", visible=True)
strand_bias = gr.Slider(minimum=0.0, maximum=0.05, label="strand bias", visible=True)
loop_bias = gr.Slider(minimum=0.0, maximum=0.20, label="loop bias", visible=True)
sec_str_opt.change(fn=toggle_secondary_structure,
inputs=[sec_str_opt],
outputs=[helix_bias,strand_bias,loop_bias,secondary_structure],
queue=False)
with gr.Accordion(label='Amino Acid Compositional Bias',open=False):
gr.Markdown("""Bias sequence composition for particular amino acids by specifying the one letter code followed by the fraction to bias. This can be input as a list for example: W0.2,E0.1""")
with gr.Row():
aa_bias = gr.Textbox(label="aa bias", lines=1, placeholder='specify one letter AA and fraction to bias, for example W0.1 or M0.1,K0.1' )
aa_bias_potential = gr.Textbox(label="aa bias scale", lines=1, placeholder='AA Bias potential scale (recomended range 1.0-5.0)')
'''
with gr.Accordion(label='Charge Bias',open=False):
gr.Markdown("""Bias for a specified net charge at a particular pH using the boxes below""")
with gr.Row():
target_charge = gr.Textbox(label="net charge", lines=1, placeholder='net charge to target')
target_ph = gr.Textbox(label="pH", lines=1, placeholder='pH at which net charge is desired')
charge_potential = gr.Textbox(label="charge potential scale", lines=1, placeholder='charge potential scale (recomended range 1.0-5.0)')
'''
with gr.Accordion(label='Hydrophobic Bias',open=False):
gr.Markdown("""Bias for or against hydrophobic composition, to get more soluble proteins, bias away with a negative target score (ex. -5)""")
with gr.Row():
hydrophobic_target_score = gr.Textbox(label="hydrophobic score", lines=1, placeholder='hydrophobic score to target (negative score is good for solublility)')
hydrophobic_potential = gr.Textbox(label="hydrophobic potential scale", lines=1, placeholder='hydrophobic potential scale (recomended range 1.0-2.0)')
with gr.Accordion(label='Diffusion Params',open=False):
gr.Markdown("""Increasing T to more steps can be helpful for harder design challenges, sampling from different distributions can change the sequence and structural composition""")
with gr.Row():
num_steps = gr.Textbox(label="T", lines=1, placeholder='number of diffusion steps (25 or less will speed things up)')
noise = gr.Dropdown(['normal','gmm2 [-1,1]','gmm3 [-1,0,1]'], label='noise type', value='normal')
btn = gr.Button("GENERATE")
#with gr.Row():
with gr.Column():
gr.Markdown("""## OUTPUTS""")
gr.Markdown("""#### Confidence score for generated structure at each timestep""")
plddt_plot = gr.Plot(label='plddt at step t')
gr.Markdown("""#### Output protein sequnece""")
output_seq = gr.Textbox(label="sequence")
gr.Markdown("""#### Download PDB file""")
output_pdb = gr.File(label="PDB file")
gr.Markdown("""#### Structure viewer""")
output_viewer = gr.HTML()
gr.Markdown("""### Don't know where to get started? Click on an example below to try it out!""")
gr.Examples(
[["","125",0.0,0.0,0.2,"","","","20","normal",'',''],
["","100",0.0,0.0,0.0,"","W0.2","2","20","normal",'',''],
["","100",0.0,0.0,0.0,"XXHHHHHHHHHXXXXXXXHHHHHHHHHXXXXXXXHHHHHHHHXXXXSSSSSSSSSSSXXXXXXXXSSSSSSSSSSSSXXXXXXXSSSSSSSSSXXXXXXX","","","25","normal",'',''],
["XXXXXXXXXXXXXXXXXXXXXXXXXIPDXXXXXXXXXXXXXXXXXXXXXXPEPSEQXXXXXXXXXXXXXXXXXXXXXXXXXXIPDXXXXXXXXXXXXXXXXXXX","",0.0,0.0,0.0,"","","","25","normal",'','']],
inputs=[sequence,
seq_len,
helix_bias,
strand_bias,
loop_bias,
secondary_structure,
aa_bias,
aa_bias_potential,
#target_charge,
#target_ph,
#charge_potential,
num_steps,
noise,
hydrophobic_target_score,
hydrophobic_potential],
outputs=[output_seq,
output_pdb,
output_viewer,
plddt_plot],
fn=protein_diffusion_model,
)
btn.click(protein_diffusion_model,
[sequence,
seq_len,
helix_bias,
strand_bias,
loop_bias,
secondary_structure,
aa_bias,
aa_bias_potential,
#target_charge,
#target_ph,
#charge_potential,
num_steps,
noise,
hydrophobic_target_score,
hydrophobic_potential],
[output_seq,
output_pdb,
output_viewer,
plddt_plot])
demo.queue()
demo.launch(debug=True)
|