Spaces:
Running
on
CPU Upgrade
Running
on
CPU Upgrade
File size: 9,316 Bytes
afebd23 d5321e7 afebd23 91a155f 235f6a0 a776cb5 d5321e7 afebd23 a776cb5 de33a84 afebd23 a776cb5 afebd23 f0a18e6 de33a84 a776cb5 91a155f a776cb5 afebd23 2d1776b afebd23 3a5e47f 0ac0d39 0976e6f 7f96439 3a5e47f 235f6a0 2d1776b 3a5e47f 235f6a0 2d1776b afebd23 235f6a0 2d1776b 3a5e47f 2d1776b f736a5a 2d1776b afebd23 235f6a0 79cb63c 235f6a0 2d1776b 3a5e47f 235f6a0 a47ca85 235f6a0 2d1776b afebd23 b49ef4d 3a5e47f afebd23 2d1776b afebd23 235f6a0 3a5e47f 235f6a0 d065c26 30396d6 3a5e47f d065c26 235f6a0 3a5e47f 235f6a0 af17c2f 5149c66 |
1 2 3 4 5 6 7 8 9 10 11 12 13 14 15 16 17 18 19 20 21 22 23 24 25 26 27 28 29 30 31 32 33 34 35 36 37 38 39 40 41 42 43 44 45 46 47 48 49 50 51 52 53 54 55 56 57 58 59 60 61 62 63 64 65 66 67 68 69 70 71 72 73 74 75 76 77 78 79 80 81 82 83 84 85 86 87 88 89 90 91 92 93 94 95 96 97 98 99 100 101 102 103 104 105 106 107 108 109 110 111 112 113 114 115 116 117 118 119 120 121 122 123 124 125 126 127 128 129 130 131 132 133 134 135 136 137 138 139 140 141 142 143 144 145 146 147 148 149 150 151 152 153 154 155 156 157 158 159 160 161 162 163 164 165 166 167 168 169 170 171 172 173 174 175 176 177 178 179 180 181 182 183 184 185 186 187 188 189 190 191 192 193 194 195 196 197 198 199 200 201 202 203 204 |
import multiprocessing
import random
from datasets import load_dataset
from sentence_transformers import SentenceTransformer
from PIL.Image import Image, ANTIALIAS
import gradio as gr
from faiss import METRIC_INNER_PRODUCT
import requests
import pandas as pd
import os
import backoff
from functools import lru_cache
from huggingface_hub import list_models, ModelFilter, login
import copy
os.environ["HF_HUB_ENABLE_HF_TRANSFER"] = "1"
cpu_count = multiprocessing.cpu_count()
model = SentenceTransformer("clip-ViT-B-16")
def resize_image(image: Image, size: int = 224) -> Image:
"""Resizes an image retaining the aspect ratio."""
w, h = image.size
if w == h:
image = image.resize((size, size), ANTIALIAS)
return image
if w > h:
height_percent = size / float(h)
width_size = int(float(w) * float(height_percent))
image = image.resize((width_size, size), ANTIALIAS)
return image
if w < h:
width_percent = size / float(w)
height_size = int(float(w) * float(width_percent))
image = image.resize((size, height_size), ANTIALIAS)
return image
dataset = load_dataset("davanstrien/ia-loaded-embedded-gpu", split="train")
dataset = dataset.filter(lambda x: x["embedding"] is not None)
dataset.add_faiss_index("embedding", metric_type=METRIC_INNER_PRODUCT)
def get_nearest_k_examples(input, k):
query = model.encode(input)
# faiss_index = dataset.get_index("embedding").faiss_index # TODO maybe add range?
# threshold = 0.95
# limits, distances, indices = faiss_index.range_search(x=query, thresh=threshold)
# images = dataset[indices]
_, retrieved_examples = dataset.get_nearest_examples("embedding", query=query, k=k)
images = retrieved_examples["image"][:k]
last_modified = retrieved_examples["last_modified_date"] # [:k]
crawl_date = retrieved_examples["crawl_date"] # [:k]
metadata = [
f"last_modified {modified}, crawl date:{crawl}"
for modified, crawl in zip(last_modified, crawl_date)
]
return list(zip(images, metadata))
def return_random_sample(k=27):
sample = random.sample(range(len(dataset)), k)
images = dataset[sample]["image"]
return [resize_image(image).convert("RGB") for image in images]
@lru_cache()
def get_valid_hub_image_classification_model_ids():
models = list_models(limit=None, filter=ModelFilter(task="image-classification"))
return {model.id for model in models}
def predict_subset(model_id, token):
# if token.value is None:
# raise gr.Error("Please enter a valid token")
valid_model_ids = get_valid_hub_image_classification_model_ids()
if model_id not in valid_model_ids:
raise gr.Error(
f"model_id {model_id} is not a valid image classification model id"
)
try:
login(token)
except ValueError:
raise gr.Error("Invalid Hub token")
API_URL = f"https://api-inference.huggingface.co/models/{model_id}"
headers = {"Authorization": f"Bearer {token}"}
@backoff.on_predicate(backoff.expo, lambda x: x.status_code == 503, max_time=30)
def _query(url):
r = requests.post(API_URL, headers=headers, data=url)
return r
@lru_cache(maxsize=1000)
def query(url):
response = _query(url)
try:
data = response.json()
argmax = data[0]
return {"score": argmax["score"], "label": argmax["label"]}
except Exception:
return {"score": None, "label": None}
# dataset2 = copy.deepcopy(dataset)
# dataset2.drop_index("embedding")
dataset = load_dataset("davanstrien/ia-loaded-embedded-gpu", split="train")
sample = random.sample(range(len(dataset)), 10)
sample = dataset.select(sample)
print("predicting...")
predictions = []
for row in sample:
url = row["url"]
predictions.append(query(url))
gallery = []
for url, prediction in zip(sample["url"], predictions):
gallery.append((url, f"{prediction['label'], prediction['score']}"))
# sample = sample.map(lambda x: query(x['url']))
labels = [d["label"] for d in predictions]
from toolz import frequencies
df = pd.DataFrame(
{
"labels": frequencies(labels).keys(),
"freqs": frequencies(labels).values(),
}
)
return gallery, df
with gr.Blocks() as demo:
gr.Markdown(
"""# ARCH Image Dataset Explorer
This [Gradio](https://gradio.app/) [Space](https://huggingface.co/spaces/launch) allows you to explore an image dataset exported from ARCH: Archive Research Compute Hub from the [Internet Archive](https://webservices.archive.org/pages/arch)
Each tab allows you to explore the dataset in a slightly different way by making use of Machine Learning models and tools from the Hugging Face ecosystem.
**NOTE**: Images in the dataset are sourced from a collection generated from the web and may contain images that are Not Suitable for All.
"""
)
with gr.Tab("Random Image Gallery"):
gr.Markdown(
"""## Random image gallery
This tab allows you to explore images in your ARCH collection. You can refresh the images by clicking the refresh button.
**Please note** not all images will be displayed as some images may not available via the original URLS anymore."""
)
button = gr.Button("Refresh")
gallery = gr.Gallery().style(grid=9, height="1400")
button.click(return_random_sample, [], [gallery])
with gr.Tab("Image Search"):
gr.Markdown(
"""## Image search
You can search for images by entering a search term and clicking the search button.
You can also change the number of images to be returned.
This model uses the [clip-ViT-B-16](https://huggingface.co/sentence-transformers/clip-ViT-B-16) model to embed your images and search term"""
)
text = gr.Textbox(label="Search for images")
k = gr.Slider(minimum=3, maximum=18, step=1)
button = gr.Button("search")
gallery = gr.Gallery().style(grid=3)
button.click(get_nearest_k_examples, [text, k], [gallery])
# gr.Markdown(
# """### More info
# 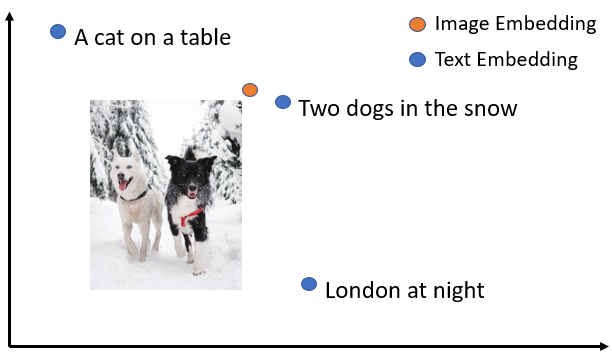"""
# )
with gr.Tab("Image Classification Model Tester"):
gr.Markdown(
"""## Image classification model tester
You can use this to test out [image classification models](https://huggingface.co/models?pipeline_tag=image-classification) on the Hugging Face Hub:
- To use this tab you will need to have a Hugging Face account and a valid token.
- You can get a token from your [Hugging Face account page](https://huggingface.co/settings/token).
- Input this token into the token box and then input a valid image classification model id from the Hub. For example `microsoft/resnet-50`. You can use the [Hub](https://huggingface.co/models?pipeline_tag=image-classification) to find suitable models.
This tab uses Hugging Face's [Inference API](https://huggingface.co/docs/api-inference/index) to make predictions. It will randomly select 10 images from your dataset and make predictions on them using your chosen model.
**Please note** the predictions will take some time since the model needs to be loaded for inference first. If you make a second batch of prediction using the same model the predictions should be quicker."""
)
token = gr.Textbox(label="token", type="password")
model_id = gr.Textbox(
label="model_id", value="davanstrien/autotrain-wikiart-sample2-42615108993"
)
button = gr.Button("predict")
gr.Markdown("## Results")
plot = gr.BarPlot(x="labels", y="freqs", width=600, height=400, vertical=False)
gallery = gr.Gallery()
button.click(predict_subset, [model_id, token], [gallery, plot])
with gr.Tab("Export to Label Studio format"):
gr.Markdown(
"""
## Export to Label Studio format
<img align=left src="https://warehouse-camo.ingress.cmh1.psfhosted.org/ba8de1e22c982bbfc28201dcc953ca15e92a399c/68747470733a2f2f7261772e67697468756275736572636f6e74656e742e636f6d2f686561727465786c6162732f6c6162656c2d73747564696f2f6d61737465722f696d616765732f6c735f6769746875625f6865616465722e706e67">
This will export the current dataset to a csv file which can be imported into [Label Studio](https://labelstud.io/). You can then import this into Label Studio to label your images by hand.
You can run Label Studio using Hugging Face Spaces using this [Spaces template](https://huggingface.co/new-space?template=LabelStudio/LabelStudio)"""
)
dataset2 = copy.deepcopy(dataset)
dataset2 = dataset2.remove_columns("image")
dataset2 = dataset2.rename_column("url", "image")
csv = dataset2.to_csv("label_studio.csv")
csv_file = gr.File("label_studio.csv")
button.click(dataset.save_to_disk, [], [csv_file])
demo.queue(concurrency_count=8, max_size=5)
demo.launch()
|