Spaces:
Runtime error
Runtime error
File size: 6,601 Bytes
0ef6060 a73da3e 0ef6060 d1db404 4f9e71f ff6fe12 8b48698 8ca1b0d 0bcb3a1 0ef6060 |
1 2 3 4 5 6 7 8 9 10 11 12 13 14 15 16 17 18 19 20 21 22 23 24 25 26 27 28 29 30 31 32 33 34 35 36 37 38 39 40 41 42 43 44 45 46 47 48 49 50 51 52 53 54 55 56 57 58 59 60 61 62 63 64 65 66 67 68 69 70 71 72 73 74 75 76 77 78 79 80 81 82 83 84 85 86 87 88 89 90 91 92 93 94 95 96 97 98 99 100 101 102 103 104 105 106 107 108 109 110 111 112 113 114 115 116 117 118 119 120 121 122 123 124 125 126 127 128 129 130 131 132 133 134 135 136 137 138 139 140 141 142 143 144 145 146 147 148 149 150 151 152 153 154 155 156 157 158 159 160 161 162 163 164 165 166 167 168 169 170 171 172 173 174 175 176 177 178 179 180 181 182 183 184 185 186 |
#!/usr/bin/env python
from __future__ import annotations
import argparse
import os
import pathlib
import subprocess
if os.getenv("SYSTEM") == "spaces":
import mim
mim.uninstall("mmcv-full", confirm_yes=True)
mim.install("mmcv-full==1.6.1", is_yes=True)
subprocess.call("pip uninstall -y opencv-python".split())
subprocess.call("pip uninstall -y opencv-python-headless".split())
subprocess.call("pip install opencv-python-headless==4.5.5.64".split())
subprocess.call("pip install package/mmdet_huggingface-2.25.1.tar.gz".split())
import cv2
import gradio as gr
import numpy as np
from model import AppModel
## Edit and
DESCRIPTION = """# MMDetection
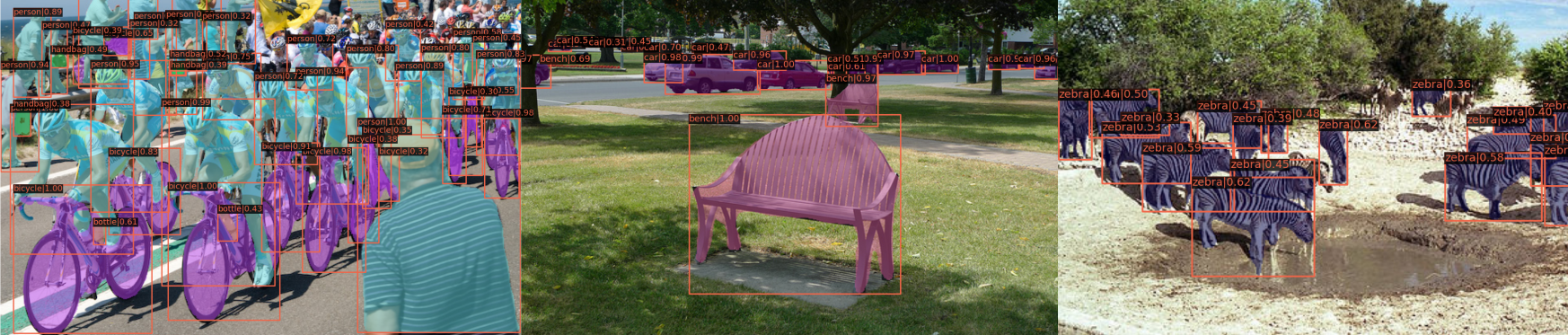
This is an unofficial demo for [https://github.com/open-mmlab/mmdetection](https://github.com/open-mmlab/mmdetection).
This space demonstrates the use of an integration of Vision pretained models from Open MMlab and hugging face hub. Model configurations and checkpoints are uploaded to hugging face space and can be used for inference directly from [MMdetection](https://github.com/open-mmlab/mmdetection) library.
With this demo, object detection using the Mask R-CNN & Faster R-CNN model can be performed.
You can upload image files, or use the example files below.
For more information on the Models, find some helpful resources below.
- Pretrained model from [OpenMMlab](https://github.com/open-mmlab/mmdetection)
- [Faster R-CNN Paper: Towards Real-Time Object
Detection with Region Proposal Networks](https://arxiv.org/pdf/1506.01497.pdf)
- [Mask R-CNN Paper](https://arxiv.org/pdf/1703.06870.pdf)
"""
FOOTER = '<img id="visitor-badge" src="https://visitor-badge.glitch.me/badge?page_id=hf-technical-mmdetection" alt="visitor badge" />'
DEFAULT_MODEL_TYPE = "detection"
DEFAULT_MODEL_NAMES = {
"detection": "faster_rcnn"
}
DEFAULT_MODEL_NAME = DEFAULT_MODEL_NAMES[DEFAULT_MODEL_TYPE]
def parse_args() -> argparse.Namespace:
parser = argparse.ArgumentParser()
parser.add_argument("--device", type=str, default="cpu")
parser.add_argument("--theme", type=str)
parser.add_argument("--share", action="store_true")
parser.add_argument("--port", type=int)
parser.add_argument("--disable-queue", dest="enable_queue", action="store_false")
return parser.parse_args()
def update_input_image(image: np.ndarray) -> dict:
if image is None:
return gr.Image.update(value=None)
scale = 1500 / max(image.shape[:2])
if scale < 1:
image = cv2.resize(image, None, fx=scale, fy=scale)
return gr.Image.update(value=image)
def update_model_name(model_type: str) -> dict:
model_dict = getattr(AppModel, f"{model_type.upper()}_MODEL_DICT")
model_names = list(model_dict.keys())
model_name = DEFAULT_MODEL_NAMES[model_type]
return gr.Dropdown.update(choices=model_names, value=model_name)
def update_visualization_score_threshold(model_type: str) -> dict:
return gr.Slider.update(visible=model_type != "panoptic_segmentation")
def update_redraw_button(model_type: str) -> dict:
return gr.Button.update(visible=model_type != "panoptic_segmentation")
def set_example_image(example: list) -> dict:
return gr.Image.update(value=example[0])
def main():
args = parse_args()
model = AppModel(DEFAULT_MODEL_NAME, args.device)
with gr.Blocks(theme=args.theme, css="style.css") as demo:
gr.Markdown(DESCRIPTION)
with gr.Row():
with gr.Column():
with gr.Row():
input_image = gr.Image(label="Input Image", type="numpy")
with gr.Group():
with gr.Row():
model_type = gr.Radio(
list(DEFAULT_MODEL_NAMES.keys()),
value=DEFAULT_MODEL_TYPE,
label="Model Type",
)
with gr.Row():
model_name = gr.Dropdown(
model.model_list(),
value=DEFAULT_MODEL_NAME,
label="Model",
)
with gr.Row():
run_button = gr.Button(value="Run")
prediction_results = gr.Variable()
with gr.Column():
with gr.Row():
visualization = gr.Image(label="Result", type="numpy")
with gr.Row():
visualization_score_threshold = gr.Slider(
0,
1,
step=0.05,
value=0.3,
label="Visualization Score Threshold",
)
with gr.Row():
redraw_button = gr.Button(value="Redraw")
with gr.Row():
paths = sorted(pathlib.Path("images").rglob("*.jpeg"))
example_images = gr.Dataset(
components=[input_image], samples=[[path.as_posix()] for path in paths]
)
gr.Markdown(FOOTER)
input_image.change(
fn=update_input_image, inputs=input_image, outputs=input_image
)
model_type.change(fn=update_model_name, inputs=model_type, outputs=model_name)
model_type.change(
fn=update_visualization_score_threshold,
inputs=model_type,
outputs=visualization_score_threshold,
)
model_type.change(
fn=update_redraw_button, inputs=model_type, outputs=redraw_button
)
model_name.change(fn=model.set_model, inputs=model_name, outputs=None)
run_button.click(
fn=model.run,
inputs=[
model_name,
input_image,
visualization_score_threshold,
],
outputs=[
prediction_results,
visualization,
],
)
redraw_button.click(
fn=model.visualize_detection_results,
inputs=[
input_image,
prediction_results,
visualization_score_threshold,
],
outputs=visualization,
)
example_images.click(
fn=set_example_image, inputs=example_images, outputs=input_image
)
demo.launch(
enable_queue=args.enable_queue,
server_port=args.port,
share=args.share,
)
if __name__ == "__main__":
main()
|