Spaces:
Paused
Paused
File size: 83,735 Bytes
ee6e328 |
1 2 3 4 5 6 7 8 9 10 11 12 13 14 15 16 17 18 19 20 21 22 23 24 25 26 27 28 29 30 31 32 33 34 35 36 37 38 39 40 41 42 43 44 45 46 47 48 49 50 51 52 53 54 55 56 57 58 59 60 61 62 63 64 65 66 67 68 69 70 71 72 73 74 75 76 77 78 79 80 81 82 83 84 85 86 87 88 89 90 91 92 93 94 95 96 97 98 99 100 101 102 103 104 105 106 107 108 109 110 111 112 113 114 115 116 117 118 119 120 121 122 123 124 125 126 127 128 129 130 131 132 133 134 135 136 137 138 139 140 141 142 143 144 145 146 147 148 149 150 151 152 153 154 155 156 157 158 159 160 161 162 163 164 165 166 167 168 169 170 171 172 173 174 175 176 177 178 179 180 181 182 183 184 185 186 187 188 189 190 191 192 193 194 195 196 197 198 199 200 201 202 203 204 205 206 207 208 209 210 211 212 213 214 215 216 217 218 219 220 221 222 223 224 225 226 227 228 229 230 231 232 233 234 235 236 237 238 239 240 241 242 243 244 245 246 247 248 249 250 251 252 253 254 255 256 257 258 259 260 261 262 263 264 265 266 267 268 269 270 271 272 273 274 275 276 277 278 279 280 281 282 283 284 285 286 287 288 289 290 291 292 293 294 295 296 297 298 299 300 301 302 303 304 305 306 307 308 309 310 311 312 313 314 315 316 317 318 319 320 321 322 323 324 325 326 327 328 329 330 331 332 333 334 335 336 337 338 339 340 341 342 343 344 345 346 347 348 349 350 351 352 353 354 355 356 357 358 359 360 361 362 363 364 365 366 367 368 369 370 371 372 373 374 375 376 377 378 379 380 381 382 383 384 385 386 387 388 389 390 391 392 393 394 395 396 397 398 399 400 401 402 403 404 405 406 407 408 409 410 411 412 413 414 415 416 417 418 419 420 421 422 423 424 425 426 427 428 429 430 431 432 433 434 435 436 437 438 439 440 441 442 443 444 445 446 447 448 449 450 451 452 453 454 455 456 457 458 459 460 461 462 463 464 465 466 467 468 469 470 471 472 473 474 475 476 477 478 479 480 481 482 483 484 485 486 487 488 489 490 491 492 493 494 495 496 497 498 499 500 501 502 503 504 505 506 507 508 509 510 511 512 513 514 515 516 517 518 519 520 521 522 523 524 525 526 527 528 529 530 531 532 533 534 535 536 537 538 539 540 541 542 543 544 545 546 547 548 549 550 551 552 553 554 555 556 557 558 559 560 561 562 563 564 565 566 567 568 569 570 571 572 573 574 575 576 577 578 579 580 581 582 583 584 585 586 587 588 589 590 591 592 593 594 595 596 597 598 599 600 601 602 603 604 605 606 607 608 609 610 611 612 613 614 615 616 617 618 619 620 621 622 623 624 625 626 627 628 629 630 631 632 633 634 635 636 637 638 639 640 641 642 643 644 645 646 647 648 649 650 651 652 653 654 655 656 657 658 659 660 661 662 663 664 665 666 667 668 669 670 671 672 673 674 675 676 677 678 679 680 681 682 683 684 685 686 687 688 689 690 691 692 693 694 695 696 697 698 699 700 701 702 703 704 705 706 707 708 709 710 711 712 713 714 715 716 717 718 719 720 721 722 723 724 725 726 727 728 729 730 731 732 733 734 735 736 737 738 739 740 741 742 743 744 745 746 747 748 749 750 751 752 753 754 755 756 757 758 759 760 761 762 763 764 765 766 767 768 769 770 771 772 773 774 775 776 777 778 779 780 781 782 783 784 785 786 787 788 789 790 791 792 793 794 795 796 797 798 799 800 801 802 803 804 805 806 807 808 809 810 811 812 813 814 815 816 817 818 819 820 821 822 823 824 825 826 827 828 829 830 831 832 833 834 835 836 837 838 839 840 841 842 843 844 845 846 847 848 849 850 851 852 853 854 855 856 857 858 859 860 861 862 863 864 865 866 867 868 869 870 871 872 873 874 875 876 877 878 879 880 881 882 883 884 885 886 887 888 889 890 891 892 893 894 895 896 897 898 899 900 901 902 903 904 905 906 907 908 909 910 911 912 913 914 915 916 917 918 919 920 921 922 923 924 925 926 927 928 929 930 931 932 933 934 935 936 937 938 939 940 941 942 943 944 945 946 947 948 949 950 951 952 953 954 955 956 957 958 959 960 961 962 963 964 965 966 967 968 969 970 971 972 973 974 975 976 977 978 979 980 981 982 983 984 985 986 987 988 989 990 991 992 993 994 995 996 997 998 999 1000 1001 1002 1003 1004 1005 1006 1007 1008 1009 1010 1011 1012 1013 1014 1015 1016 1017 1018 1019 1020 1021 1022 1023 1024 1025 1026 1027 1028 1029 1030 1031 1032 1033 1034 1035 1036 1037 1038 1039 1040 1041 1042 1043 1044 1045 1046 1047 1048 1049 1050 1051 1052 1053 1054 1055 1056 1057 1058 1059 1060 1061 1062 1063 1064 1065 1066 1067 1068 1069 1070 1071 1072 1073 1074 1075 1076 1077 1078 1079 1080 1081 1082 1083 1084 1085 1086 1087 1088 1089 1090 1091 1092 1093 1094 1095 1096 1097 1098 1099 1100 1101 1102 1103 1104 1105 1106 1107 1108 1109 1110 1111 1112 1113 1114 1115 1116 1117 1118 1119 1120 1121 1122 1123 1124 1125 1126 1127 1128 1129 1130 1131 1132 1133 1134 1135 1136 1137 1138 1139 1140 1141 1142 1143 1144 1145 1146 1147 1148 1149 1150 1151 1152 1153 1154 1155 1156 1157 1158 1159 1160 1161 1162 1163 1164 1165 1166 1167 1168 1169 1170 1171 1172 1173 1174 1175 1176 1177 1178 1179 1180 1181 1182 1183 1184 1185 1186 1187 1188 1189 1190 1191 1192 1193 1194 1195 1196 1197 1198 1199 1200 1201 1202 1203 1204 1205 1206 1207 1208 1209 1210 1211 1212 1213 1214 1215 1216 1217 1218 1219 1220 1221 1222 1223 1224 1225 1226 1227 1228 1229 1230 1231 1232 1233 1234 1235 1236 1237 1238 1239 1240 1241 1242 1243 1244 1245 1246 1247 1248 1249 1250 1251 1252 1253 1254 1255 1256 1257 1258 1259 1260 1261 1262 1263 1264 1265 1266 1267 1268 1269 1270 1271 1272 1273 1274 1275 1276 1277 1278 1279 1280 1281 1282 1283 1284 1285 1286 1287 1288 1289 1290 1291 1292 1293 1294 1295 1296 |
# Flax/JAX community week 🤗
Welcome to the Flax/JAX community week! The goal of this week is to make compute-intensive NLP and CV projects (like pre-training BERT, GPT2, CLIP, ViT)
practicable for a wider audience of engineers and researchers.
To do so, we will try to teach **you** how to effectively use JAX/Flax on TPU and help you to complete a fun NLP and/or CV project in JAX/Flax during the community week.
Free access to a TPUv3-8 will kindly be provided by the Google Cloud team!
In this document, we list all the important information that you will need during the Flax/JAX community week.
Don't forget to sign up [here](https://forms.gle/tVGPhjKXyEsSgUcs8)!
## Table of Contents
- [Organization](#organization)
- [Important dates](#important-dates)
- [Communication](#communication)
- [Projects](#projects)
- [How to propose](#how-to-propose-a-project)
- [How to form a team](#how-to-form-a-team-around-a-project)
- [Tips & Tricks for project](#tips-on-how-to-organize-the-project)
- [How to install flax, jax, optax, transformers, datasets](#how-to-install-relevant-libraries)
- [Quickstart Flax/JAX](#quickstart-flax-and-jax)
- [Quickstart Flax/JAX in 🤗 Transformers](#quickstart-flax-and-jax-in-transformers)
- [Flax design philosophy in 🤗 Transformers](#flax-design-philosophy-in-transformers)
- [How to use flax models & scripts](#how-to-use-flax-models-and-example-scripts)
- [Talks](#talks)
- [How to use the 🤗 Hub for training](#how-to-use-the-hub-for-collaboration)
- [How to setup TPU VM](#how-to-setup-tpu-vm)
- [How to build a demo](#how-to-build-a-demo)
- [Using the Hugging Face Widgets](#using-the-hugging-face-widgets)
- [Using a Streamlit demo](#using-a-streamlit-demo)
- [Using a Gradio demo](#using-a-gradio-demo)
- [Project evaluation](#project-evaluation)
- [General Tips & Tricks](#general-tips-and-tricks)
- [FAQ](#faq)
## Organization
Participants can propose ideas for an interesting NLP and/or CV project. Teams of 3 to 5 will then be formed around the most promising and interesting projects. Make sure to read through the [Projects](#projects) section on how to propose projects, comment on other participants' project ideas, and create a team.
To help each team successfully finish their project, we have organized talks by leading scientists and engineers from Google, Hugging Face, and the open-source NLP & CV community. The talks will take place before the community week from June 30th to July 2nd. Make sure to attend the talks to get the most out of your participation! Check out the [Talks](#talks) section to get an overview of the talks, including the speaker and the time of the talk.
Each team is then given **free access to a TPUv3-8 VM** from July 7th to July 14th. In addition, we will provide training examples in JAX/Flax for a variety of NLP and Vision models to kick-start your project. During the week, we'll make sure to answer any questions you might have about JAX/Flax and Transformers and help each team as much as possible to complete their project!
At the end of the community week, each team should submit a demo of their project. All demonstrations will be evaluated by a jury and the top-3 demos will be awarded a prize. Check out the [How to submit a demo](#how-to-submit-a-demo) section for more information and suggestions on how to submit your project.
## Important dates
- **23.06.** Official announcement of the community week. Make sure to sign-up in [this google form](https://forms.gle/tVGPhjKXyEsSgUcs8).
- **23.06. - 30.06.** Participants will be added to an internal Slack channel. Project ideas can be proposed here and groups of 3-5 are formed. Read this document for more information.
- **30.06.** Release of all relevant training scripts in JAX/Flax as well as other documents on how to set up a TPU, how to use the training scripts, how to submit a demo, tips & tricks for JAX/Flax, tips & tricks for efficient use of the hub.
- **30.06. - 2.07.** Talks about JAX/Flax, TPU, Transformers, Computer Vision & NLP will be held.
- **7.07.** Start of the community week! Access to TPUv3-8 will be given to each team.
- **7.07. - 14.07.** The Hugging Face & JAX/Flax & Cloud team will be available for any questions, problems the teams might run into.
- **15.07.** Access to TPU is deactivated and community week officially ends.
- **16.07.** Deadline for each team to submit a demo.
## Communication
All important communication will take place in an internal Slack channel, called `#flax-jax-community-week`.
Important announcements of the Hugging Face, Flax/JAX, and Google Cloud team will be posted there.
Such announcements include general information about the community week (Dates, Rules, ...), release of relevant training scripts (Flax/JAX example scripts for NLP and Vision), release of other important documents (How to access the TPU), etc.
The Slack channel will also be the central place for participants to post about their results, share their learning experiences, ask questions, etc.
For issues with Flax/JAX, Transformers, Datasets or for questions that are specific to your project we would be **very happy** if you could use the following public repositories and forums:
- Flax: [Issues](https://github.com/google/flax/issues), [Questions](https://github.com/google/flax/discussions)
- JAX: [Issues](https://github.com/google/jax/issues), [Questions](https://github.com/google/jax/discussions)
- 🤗 Transformers: [Issues](https://github.com/huggingface/transformers/issues), [Questions](https://discuss.huggingface.co/c/transformers/9)
- 🤗 Datasets: [Issues](https://github.com/huggingface/datasets/issues), [Questions](https://discuss.huggingface.co/c/datasets/10)
- Project specific questions: [Forum](https://discuss.huggingface.co/c/flax-jax-projects/22)
- TPU related questions: [TODO]()
Please do **not** post the complete issue/project-specific question in the Slack channel, but instead a link to your issue/question that we will try to answer as soon as possible.
This way, we make sure that the everybody in the community can benefit from your questions - even after the community week - and that the same question is not answered twice.
To be invited to the Slack channel, please make sure you have signed up [on the Google form](https://forms.gle/tVGPhjKXyEsSgUcs8).
**Note**: If you have signed up on the google form, but you are not in the Slack channel, please leave a message on [(TODO) the official forum announcement]( ) and ping `@Suzana` and `@patrickvonplaten`.
## Projects
During the first week after the community week announcement, **23.06. - 30.06.**, teams will be formed around the most promising and interesting project ideas. Each team can consist of 2 to 10 participants. Projects can be accessed [here](https://discuss.huggingface.co/c/flax-jax-projects/22).
All officially defined projects can be seen [here](https://docs.google.com/spreadsheets/d/1GpHebL7qrwJOc9olTpIPgjf8vOS0jNb6zR_B8x_Jtik/edit?usp=sharing).
### How to propose a project
Some default project ideas are given by the organizers. **However, we strongly encourage participants to submit their own project ideas!**
Check out the [HOW_TO_PROPOSE_PROJECT.md](https://github.com/huggingface/transformers/tree/main/examples/research_projects/jax-projects/HOW_TO_PROPOSE_PROJECT.md) for more information on how to propose a new project.
### How to form a team around a project
You can check out all existing projects ideas on the forum under [Flax/JAX projects category](https://discuss.huggingface.co/c/flax-jax-projects/22).
Make sure to quickly check out each project idea and leave a ❤️ if you like an idea.
Feel free to leave comments, suggestions for improvement, or questions about more details directly on the discussion thread.
If you have found the project that you ❤️ the most, leave a message "I would like to join this project" on the discussion thread.
We strongly advise you to also shortly state who you are, which time zone you are in and why you would like to work on this project, how you can contribute to the project and what your vision is for the project.
For projects that see a lot of interest and for which enough participants have expressed interest in joining, an official team will be created by the organizers.
One of the organizers (`@Suzana`, `@valhalla`, `@osanseviero`, `@patrickvonplaten`) will leave a message "For this project the team: `<team_name>`, `<team_members>` , is officially created" on the thread and note down the teams on [this google sheet](https://docs.google.com/spreadsheets/d/1GpHebL7qrwJOc9olTpIPgjf8vOS0jNb6zR_B8x_Jtik/edit?usp=sharing).
Once created, the team can start refining their project:
- What is the goal of the project? *E.g.*, Present a language model that writes poetry in Russian.
- What model will we use? *E.g.*, FlaxGPT2
- What data will we use? *E.g.* Russian dataset of OSCAR & publicly available book on poetry
- Should we use a pre-trained model or train a model from scratch? E.g. Train a model from scratch
- What training scripts do we need? *E.g.* `transformers/examples/flax/run_clm_flax.py` can be used
- What kind of demo would we like to present? E.g. Text-generation API of the 🤗 Hub in combination with a Streamlit demo that lets the user generate a poem of a given length
- How will the work be divided? *E.g.* Team member 1 works on data preprocessing, Team member 2 works on adapting the Flax script, ...
We highly recommend that each team discusses all relevant ideas for their project directly on the forum thread.
This way valuable learning experiences are shared and accessible by the whole community in the future.
Additionally, the organizers, other participants, or anybody in the community really can read through your discussions and leave comments/tips for improvement. Obviously, you can also create private chats, ... to discuss more sensitive topics, etc.
**Important**:
- For project ideas that see a lot of interest, we are more than happy to create more than one team.
- Participants are welcome to join multiple teams, even though we encourage them to only work on a single project.
- Under special circumstances, participants can change/create new teams. Please note that we would like to keep this the exception. If however, you would like to change/leave existing teams, please leave a post on the project's thread where you ping the corresponding organizer that created the group.
- It is often easy to propose/join a project that is done in your native language. Feel free to reach out to existing [language-specific groups](https://discuss.huggingface.co/c/languages-at-hugging-face/15) to look for community members that might be interested in joining your project.
## Tips on how to organize the project
This section gives you some tips on how to most efficiently & effectively
work as a team to achieve your goal. It is by no means a strict recipe to follow,
but rather a collection of tips from the 🤗 team.
Once your team is defined, you can start working on the project as soon as possible.
### Communication
At first, it is always useful to get to know each other and to set up a means of communication.
While we recommend that all technical aspects of work can be discussed directly on the [forum](https://discuss.huggingface.co/c/flax-jax-projects/22) under your project thread,
it can be very helpful to have a more direct way of communicating, *e.g.* in a channel.
For this we have created a discord that you can access [here](https://discord.com/channels/858019234139602994/858019234139602997).
This discord will not be managed by anybody and is just there so that you can communicate more effectively with your team members.
Feel free to create a new channel for you and your team where you can discuss everything. If you and your team have already set up other ways of communicating, it is absolutely not required to make use of the discord. However, we do recommend each team to set up some kind
of channel or group for quick discussions.
### Project definition
In the very beginning, you should make sure your project is well-defined and that
everybody in the team understands the goal of the project and the work that needs to be
done in order to achieve the goal. A well-defined project:
- has defined the task on which the model will be trained
- has defined the model that will be trained
- has defined the datasets that will be used for training
- has defined the type of training scripts that need to be written
- has defined the desired outcome of the project
- has defined the workflows
By "has defined" we don't meant that the corresponding code already has to be written and ready
to be used, but that everybody in team is on the same page on what type of model, data and training script should be used.
To give an example, a well-defined project would be the following:
- task: summarization
- model: [t5-small](https://huggingface.co/t5-small)
- dataset: [CNN/Daily mail](https://huggingface.co/datasets/cnn_dailymail)
- training script: [run_summarization_flax.py](https://github.com/huggingface/transformers/blob/main/examples/flax/summarization/run_summarization_flax.py)
- outcome: t5 model that can summarize news
- work flow: adapt `run_summarization_flax.py` to work with `t5-small`.
This example is a very easy and not the most interesting project since a `t5-small`
summarization model exists already for CNN/Daily mail and pretty much no code has to be
written.
A well-defined project does not need to have the dataset be part of
the `datasets` library and the training script already be pre-written, however it should
be clear how the desired dataset can be accessed and how the training script can be
written.
It is also important to have a clear plan regarding the workflow. Usually, the
data processing is done in a first step. Once the data is in a format that the model can
work with, the training script can be written, etc. These steps should be more detailed
once the team has a clearly defined project. It can be helpful to set deadlines for each step.
### Workload division
To effectively work as a team, it is crucial to divide the workload among everybody.
Some team members will be more motivated and experienced than others and
some team members simply want to participate to learn more and cannot contribute that
much to the team. This is totally fine! One cannot expect everybody in the team to have the same level of experience and time/motivation during the community week.
As a conclusion, being honest about one's expected involvement is crucial so that
the workload can be divided accordingly. If someone doesn't think her/his tasks are feasible - let
the team know early on so that someone else can take care of it!
It is recommended that the motivated and experienced team members take the lead in dividing the work and are ready to take over the tasks of another team member if necessary.
The workload can often be divided according to:
- data preprocessing (load the data and preprocess data in the correct format)
- data tokenization / data collator (process data samples into tokens or images)
- model configuration (writing the code that defines the model)
- model forward pass (make sure input / output work correctly)
- loss function (define the loss function)
- putting the pieces together in a training script
Many of the steps above require other steps to be finished, so it often makes sense
to use dummy data in the expected format to start, *e.g.*, with the model forward pass
before the data preprocessing is done.
### Expectations
It is also very important to stay realistic with the scope of your project. Each team
has access to a TPUv3-8 for only *ca.* 10 days, so it's important to keep the scope of
the project reasonable. While we do want each team to work on interesting projects, each
team should make sure that the project goals can be achieved within the provided compute
time on TPU. For instance, pretraining a 11 billion parameters T5 model is not really a realistic
task with just 10 days of TPUv3-8 compute.
Also, it might be difficult to finish a project where the whole modeling, dataset and training code has to be written from scratch.
Having defined your project, feel free to reach out on Slack or the forum for feedback from the organizers. We can surely give you our opinion on whether the project is feasible and what can be done to improve it.
the project is feasible.
### Other tips
Here is a collection of some more tips:
- We strongly recommend to work as publicly and collaboratively as possible during the week so that other teams
and the organizers can best help you. This includes publishing important discussions on
the forum and making use of the [🤗 hub](http://huggingface.co/) to have a version
control for your models and training logs.
- When debugging, it is important that the debugging cycle is kept as short as possible to
be able to effectively debug. *E.g.* if there is a problem with your training script,
you should run it with just a couple of hundreds of examples and not the whole dataset script. This can be done by either making use of [datasets streaming](https://huggingface.co/docs/datasets/master/dataset_streaming.html?highlight=streaming) or by selecting just the first
X number of data samples after loading:
```python
datasets["train"] = datasets["train"].select(range(1000))
```
- Ask for help. If you are stuck, use the public Slack channel or the [forum](https://discuss.huggingface.co/c/flax-jax-projects/22) to ask for help.
## How to install relevant libraries
In the following we will explain how to install all relevant libraries on your local computer and on TPU VM.
It is recommended to install all relevant libraries both on your local machine
and on the TPU virtual machine. This way, quick prototyping and testing can be done on
your local machine and the actual training can be done on the TPU VM.
### Local computer
The following libraries are required to train a JAX/Flax model with 🤗 Transformers and 🤗 Datasets:
- [JAX](https://github.com/google/jax/)
- [Flax](https://github.com/google/flax)
- [Optax](https://github.com/deepmind/optax)
- [Transformers](https://github.com/huggingface/transformers)
- [Datasets](https://github.com/huggingface/datasets)
You should install the above libraries in a [virtual environment](https://docs.python.org/3/library/venv.html).
If you're unfamiliar with Python virtual environments, check out the [user guide](https://packaging.python.org/guides/installing-using-pip-and-virtual-environments/). Create a virtual environment with the version of Python you're going
to use and activate it.
You should be able to run the command:
```bash
python3 -m venv <your-venv-name>
```
You can activate your venv by running
```bash
source ~/<your-venv-name>/bin/activate
```
We strongly recommend to make use of the provided JAX/Flax examples scripts in [transformers/examples/flax](https://github.com/huggingface/transformers/tree/main/examples/flax) even if you want to train a JAX/Flax model of another github repository that is not integrated into 🤗 Transformers.
In all likelihood, you will need to adapt one of the example scripts, so we recommend forking and cloning the 🤗 Transformers repository as follows.
Doing so will allow you to share your fork of the Transformers library with your team members so that the team effectively works on the same code base. It will also automatically install the newest versions of `flax`, `jax` and `optax`.
1. Fork the [repository](https://github.com/huggingface/transformers) by
clicking on the 'Fork' button on the repository's page. This creates a copy of the code
under your GitHub user account.
2. Clone your fork to your local disk, and add the base repository as a remote:
```bash
$ git clone https://github.com/<your Github handle>/transformers.git
$ cd transformers
$ git remote add upstream https://github.com/huggingface/transformers.git
```
3. Create a new branch to hold your development changes. This is especially useful to share code changes with your team:
```bash
$ git checkout -b a-descriptive-name-for-my-project
```
4. Set up a flax environment by running the following command in a virtual environment:
```bash
$ pip install -e ".[flax]"
```
(If transformers was already installed in the virtual environment, remove
it with `pip uninstall transformers` before reinstalling it in editable
mode with the `-e` flag.)
If you have already cloned that repo, you might need to `git pull` to get the most recent changes in the `datasets`
library.
Running this command will automatically install `flax`, `jax` and `optax`.
Next, you should also install the 🤗 Datasets library. We strongly recommend installing the
library from source to profit from the most current additions during the community week.
Simply run the following steps:
```
$ cd ~/
$ git clone https://github.com/huggingface/datasets.git
$ cd datasets
$ pip install -e ".[streaming]"
```
If you plan on contributing a specific dataset during
the community week, please fork the datasets repository and follow the instructions
[here](https://github.com/huggingface/datasets/blob/master/CONTRIBUTING.md#how-to-create-a-pull-request).
To verify that all libraries are correctly installed, you can run the following command.
It assumes that both `transformers` and `datasets` were installed from main - otherwise
datasets streaming will not work correctly.
```python
from transformers import FlaxRobertaModel, RobertaTokenizerFast
from datasets import load_dataset
import jax
dataset = load_dataset('oscar', "unshuffled_deduplicated_en", split='train', streaming=True)
dummy_input = next(iter(dataset))["text"]
tokenizer = RobertaTokenizerFast.from_pretrained("roberta-base")
input_ids = tokenizer(dummy_input, return_tensors="np").input_ids[:, :10]
model = FlaxRobertaModel.from_pretrained("julien-c/dummy-unknown")
# run a forward pass, should return an object `FlaxBaseModelOutputWithPooling`
model(input_ids)
```
### TPU VM
**VERY IMPORTANT** - Only one process can access the TPU cores at a time. This means that if multiple team members
are trying to connect to the TPU cores errors, such as:
```
libtpu.so already in used by another process. Not attempting to load libtpu.so in this process.
```
are thrown. As a conclusion, we recommend every team member to create her/his own virtual environment, but only one
person should run the heavy training processes. Also, please take turns when setting up the TPUv3-8 so that everybody
can verify that JAX is correctly installed.
The following libraries are required to train a JAX/Flax model with 🤗 Transformers and 🤗 Datasets on TPU VM:
- [JAX](https://github.com/google/jax/)
- [Flax](https://github.com/google/flax)
- [Optax](https://github.com/deepmind/optax)
- [Transformers](https://github.com/huggingface/transformers)
- [Datasets](https://github.com/huggingface/datasets)
You should install the above libraries in a [virtual environment](https://docs.python.org/3/library/venv.html).
If you're unfamiliar with Python virtual environments, check out the [user guide](https://packaging.python.org/guides/installing-using-pip-and-virtual-environments/). Create a virtual environment with the version of Python you're going
to use and activate it.
You should be able to run the command:
```bash
python3 -m venv <your-venv-name>
```
If this doesn't work, you first might to have install `python3-venv`. You can do this as follows:
```bash
sudo apt-get install python3-venv
```
You can activate your venv by running
```bash
source ~/<your-venv-name>/bin/activate
```
Next you should install JAX's TPU version on TPU by running the following command:
```
$ pip install requests
```
and then:
```
$ pip install "jax[tpu]>=0.2.16" -f https://storage.googleapis.com/jax-releases/libtpu_releases.html
```
**Note**: Running this command might actually throw an error, such as:
```
Building wheel for jax (setup.py) ... error
ERROR: Command errored out with exit status 1:
command: /home/patrick/patrick/bin/python3 -u -c 'import sys, setuptools, tokenize; sys.argv[0] = '"'"'/tmp/pip-install-lwseckn1/jax/setup.py'"'"'; __file__='"'"'/tmp/pip-install-lwseckn1/jax/setup.py'"'"';f=getattr(tokenize, '"'"'open'"'"', open)(__file__);code=f.read().replace('"'"'\r\n'"'"', '"'"'\n'"'"');f.close();exec(compile(code, __file__, '"'"'exec'"'"'))' bdist_wheel -d /tmp/pip-wheel-pydotzlo
cwd: /tmp/pip-install-lwseckn1/jax/
Complete output (6 lines):
usage: setup.py [global_opts] cmd1 [cmd1_opts] [cmd2 [cmd2_opts] ...]
or: setup.py --help [cmd1 cmd2 ...]
or: setup.py --help-commands
or: setup.py cmd --help
error: invalid command 'bdist_wheel'
----------------------------------------
ERROR: Failed building wheel for jax
```
Jax should have been installed correctly nevertheless.
To verify that JAX was correctly installed, you can run the following command:
```python
import jax
jax.device_count()
```
This should display the number of TPU cores, which should be 8 on a TPUv3-8 VM.
We strongly recommend to make use of the provided JAX/Flax examples scripts in [transformers/examples/flax](https://github.com/huggingface/transformers/tree/main/examples/flax) even if you want to train a JAX/Flax model of another github repository that is not integrated into 🤗 Transformers.
In all likelihood, you will need to adapt one of the example scripts, so we recommend forking and cloning the 🤗 Transformers repository as follows.
Doing so will allow you to share your fork of the Transformers library with your team members so that the team effectively works on the same code base. It will also automatically install the newest versions of `flax`, `jax` and `optax`.
1. Fork the [repository](https://github.com/huggingface/transformers) by
clicking on the 'Fork' button on the repository's page. This creates a copy of the code
under your GitHub user account.
2. Clone your fork to your local disk, and add the base repository as a remote:
```bash
$ git clone https://github.com/<your Github handle>/transformers.git
$ cd transformers
$ git remote add upstream https://github.com/huggingface/transformers.git
```
3. Create a new branch to hold your development changes. This is especially useful to share code changes with your team:
```bash
$ git checkout -b a-descriptive-name-for-my-project
```
4. Set up a flax environment by running the following command in a virtual environment:
```bash
$ pip install -e ".[flax]"
```
(If transformers was already installed in the virtual environment, remove
it with `pip uninstall transformers` before reinstalling it in editable
mode with the `-e` flag.)
If you have already cloned that repo, you might need to `git pull` to get the most recent changes in the `datasets`
library.
Running this command will automatically install `flax`, `jax` and `optax`.
Next, you should also install the 🤗 Datasets library. We strongly recommend installing the
library from source to profit from the most current additions during the community week.
Simply run the following steps:
```
$ cd ~/
$ git clone https://github.com/huggingface/datasets.git
$ cd datasets
$ pip install -e ".[streaming]"
```
If you plan on contributing a specific dataset during
the community week, please fork the datasets repository and follow the instructions
[here](https://github.com/huggingface/datasets/blob/master/CONTRIBUTING.md#how-to-create-a-pull-request).
To verify that all libraries are correctly installed, you can run the following command.
It assumes that both `transformers` and `datasets` were installed from main - otherwise
datasets streaming will not work correctly.
```python
from transformers import FlaxRobertaModel, RobertaTokenizerFast
from datasets import load_dataset
import jax
dataset = load_dataset('oscar', "unshuffled_deduplicated_en", split='train', streaming=True)
dummy_input = next(iter(dataset))["text"]
tokenizer = RobertaTokenizerFast.from_pretrained("roberta-base")
input_ids = tokenizer(dummy_input, return_tensors="np").input_ids[:, :10]
model = FlaxRobertaModel.from_pretrained("julien-c/dummy-unknown")
# run a forward pass, should return an object `FlaxBaseModelOutputWithPooling`
model(input_ids)
```
## Quickstart flax and jax
[JAX](https://jax.readthedocs.io/en/latest/index.html) is Autograd and XLA, brought together for high-performance numerical computing and machine learning research. It provides composable transformations of Python+NumPy programs: differentiate, vectorize, parallelize, Just-In-Time compile to GPU/TPU, and more. A great place for getting started with JAX is the [JAX 101 Tutorial](https://jax.readthedocs.io/en/latest/jax-101/index.html).
[Flax](https://flax.readthedocs.io/en/latest/index.html) is a high-performance neural network library designed for flexibility built on top of JAX. It aims to provide users with full control of their training code and is carefully designed to work well with JAX transformations such as `grad` and `pmap` (see the [Flax philosophy](https://flax.readthedocs.io/en/latest/philosophy.html)). For an introduction to Flax see the [Flax Basics Colab](https://flax.readthedocs.io/en/latest/notebooks/flax_basics.html) or the list of curated [Flax examples](https://flax.readthedocs.io/en/latest/examples.html).
## Quickstart flax and jax in transformers
Currently, we support the following models in Flax.
Note that some models are about to be merged to `main` and will
be available in a couple of days.
- [BART](https://github.com/huggingface/transformers/blob/main/src/transformers/models/bart/modeling_flax_bart.py)
- [BERT](https://github.com/huggingface/transformers/blob/main/src/transformers/models/bert/modeling_flax_bert.py)
- [BigBird](https://github.com/huggingface/transformers/blob/main/src/transformers/models/big_bird/modeling_flax_big_bird.py)
- [CLIP](https://github.com/huggingface/transformers/blob/main/src/transformers/models/clip/modeling_flax_clip.py)
- [ELECTRA](https://github.com/huggingface/transformers/blob/main/src/transformers/models/electra/modeling_flax_electra.py)
- [GPT2](https://github.com/huggingface/transformers/blob/main/src/transformers/models/gpt2/modeling_flax_gpt2.py)
- [(TODO) MBART](https://github.com/huggingface/transformers/blob/main/src/transformers/models/mbart/modeling_flax_mbart.py)
- [RoBERTa](https://github.com/huggingface/transformers/blob/main/src/transformers/models/roberta/modeling_flax_roberta.py)
- [T5](https://github.com/huggingface/transformers/blob/main/src/transformers/models/t5/modeling_flax_t5.py)
- [ViT](https://github.com/huggingface/transformers/blob/main/src/transformers/models/vit/modeling_flax_vit.py)
- [Wav2Vec2](https://github.com/huggingface/transformers/blob/main/src/transformers/models/wav2vec2/modeling_flax_wav2vec2.py)
You can find all available training scripts for JAX/Flax under the
official [flax example folder](https://github.com/huggingface/transformers/tree/main/examples/flax). Note that a couple of training scripts will be released in the following week.
- [Causal language modeling (GPT2)](https://github.com/huggingface/transformers/blob/main/examples/flax/language-modeling/run_clm_flax.py)
- [Masked language modeling (BERT, RoBERTa, ELECTRA, BigBird)](https://github.com/huggingface/transformers/blob/main/examples/flax/language-modeling/run_mlm_flax.py)
- [Text classification (BERT, RoBERTa, ELECTRA, BigBird)](https://github.com/huggingface/transformers/blob/main/examples/flax/text-classification/run_flax_glue.py)
- [Summarization / Seq2Seq (BART, MBART, T5)](https://github.com/huggingface/transformers/blob/main/examples/flax/summarization/run_summarization_flax.py)
- [Masked Seq2Seq pret-training (T5)](https://github.com/huggingface/transformers/blob/main/examples/flax/language-modeling/run_t5_mlm_flax.py)
- [Contrastive Loss pretraining for Wav2Vec2](https://github.com/huggingface/transformers/blob/main/examples/research_projects/jax-projects/wav2vec2)
- [Fine-tuning long-range QA for BigBird](https://github.com/huggingface/transformers/blob/main/examples/research_projects/jax-projects/big_bird)
- [(TODO) Image classification (ViT)]( )
- [(TODO) CLIP pretraining, fine-tuning (CLIP)]( )
### **Flax design philosophy in Transformers**
This section will explain how Flax models are implemented in Transformers and how the design differs from PyTorch.
Let's first go over the difference between Flax and PyTorch.
In JAX, most transformations (notably `jax.jit`) require functions that are transformed to be stateless so that they have no side effects. This is because any such side-effects will only be executed once when the transformed function is run during compilation and all subsequent calls of the compiled function would re-use the same side-effects of the compiled run instead of the "actual" side-effects (see [Stateful Computations in JAX](https://jax.readthedocs.io/en/latest/jax-101/07-state.html)). As a consequence, Flax models, which are designed to work well with JAX transformations, are stateless. This means that when running a model in inference, both the inputs and the model weights are passed to the forward pass. In contrast, PyTorch model are very much stateful with the weights being stored within the model instance and the user just passing the inputs to the forward pass.
Let's illustrate the difference between stateful models in PyTorch and stateless models in Flax.
For simplicity, let's assume the language model consists simply of a single attention layer [`key_proj`, `value_proj`, `query_proj`] and a linear layer `logits_proj` to project the transformed word embeddings to the output logit vectors.
#### **Stateful models in PyTorch**
In PyTorch, the weights matrices would be stored as `torch.nn.Linear` objects alongside the model's config inside the model class `ModelPyTorch`:
```python
class ModelPyTorch:
def __init__(self, config):
self.config = config
self.key_proj = torch.nn.Linear(config)
self.value_proj = torch.nn.Linear(config)
self.query_proj = torch.nn.Linear(config)
self.logits_proj = torch.nn.Linear(config)
```
Instantiating an object `model_pytorch` of the class `ModelPyTorch` would actually allocate memory for the model weights and attach them to the attributes `self.key_proj`, `self.value_proj`, `self.query_proj`, and `self.logits.proj`. We could access the weights via:
```
key_projection_matrix = model_pytorch.key_proj.weight.data
```
Visually, we would represent an object of `model_pytorch` therefore as follows:
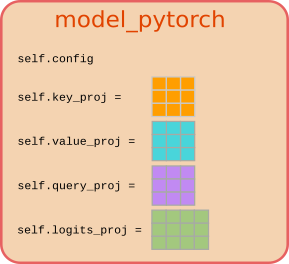
Executing a forward pass then simply corresponds to passing the `input_ids` to the object `model_pytorch`:
```python
sequences = model_pytorch(input_ids)
```
In a more abstract way, this can be represented as passing the word embeddings to the model function to get the output logits:

This design is called **stateful** because the output logits, the `sequences`, can change even if the word embeddings, the `input_ids`, stay the same. Hence, the function's output does not only depend on its inputs, but also on its **state**, `[self.key_proj, self.value_proj, self.query_proj, self.logits_proj]`, which makes `model_pytorch` stateful.
#### **Stateless models in Flax/JAX**
Now, let's see how the mathematically equivalent model would be written in JAX/Flax. The model class `ModelFlax` would define the self-attention and logits projection weights as [**`flax.linen.Dense`**](https://flax.readthedocs.io/en/latest/_autosummary/flax.linen.Dense.html#flax.linen.Dense) objects:
```python
class ModelFlax:
def __init__(self, config):
self.config = config
self.key_proj = flax.linen.Dense(config)
self.value_proj = flax.linen.Dense(config)
self.query_proj = flax.linen.Dense(config)
self.logits_proj = flax.linen.Dense(config)
```
At first glance the linear layer class `flax.linen.Dense` looks very similar to PyTorch's `torch.nn.Linear` class. However, instantiating an object `model_flax` only defines the linear transformation functions and does **not** allocate memory to store the linear transformation weights. In a way, the attribute `self.key_proj` tell the instantiated object `model_flax` to perform a linear transformation on some input and force it to expect a weight, called `key_proj`, as an input.
This time we would illustrate the object `model_flax` without the weight matrices:
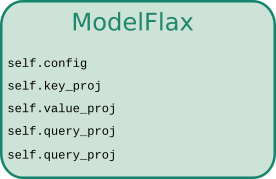
Accordingly, the forward pass requires both `input_ids` as well as a dictionary consisting of the model's weights (called `state` here) to compute the `sequences`:
To get the initial `state` we need to explicitly do a forward pass by passing a dummy input:
```python
state = model_flax.init(rng, dummy_input_ids)
```
and then we can do the forward pass.
```python
sequences = model_flax.apply(state, input_ids)
```
Visually, the forward pass would now be represented as passing all tensors required for the computation to the model's object:
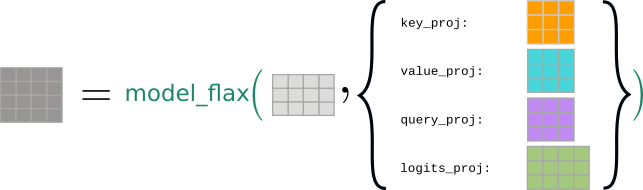
This design is called **stateless** because the output logits, the `sequences`, **cannot** change if the word embeddings, the `input_ids`, stay the same. Hence, the function's output only depends on its inputs, being the `input_ids` and the `state` dictionary consisting of the weights **state**, `[key_proj, value_proj, query_proj, logits_proj]`.
Another term which is often used to describe the design difference between Flax/JAX and PyTorch is **immutable** vs **mutable**. A instantiated Flax model, `model_flax`, is **immutable** as a logical consequence of `model_flax`'s output being fully defined by its input: If calling `model_flax` could mutate `model_flax`, then calling `model_flax` twice with the same inputs could lead to different results which would violate the "*statelessness*" of Flax models.
#### **Flax models in Transformers**
Now let us see how this is handled in `Transformers.` If you have used a Flax model in Transformers already, you might wonder how come you don't always have to pass the parameters to the function of the forward pass. This is because the `FlaxPreTrainedModel` class abstracts it away.
It is designed this way so that the Flax models in Transformers will have a similar API to PyTorch and Tensorflow models.
The `FlaxPreTrainedModel` is an abstract class that holds a Flax module, handles weights initialization, and provides a simple interface for downloading and loading pre-trained weights i.e. the `save_pretrained` and `from_pretrained` methods. Each Flax model then defines its own subclass of `FlaxPreTrainedModel`; *e.g.* the BERT model has `FlaxBertPreTrainedModel`. Each such class provides two important methods, `init_weights` and `__call__`. Let's see what each of those methods do:
- The `init_weights` method takes the expected input shape and a [`PRNGKey`](https://jax.readthedocs.io/en/latest/_autosummary/jax.random.PRNGKey.html) (and any other arguments that are required to get initial weights) and calls `module.init` by passing it a random example to get the initial weights with the given `dtype` (for ex. `fp32` or `bf16` etc). This method is called when we create an instance of the model class, so the weights are already initialized when you create a model i.e., when you do
model = FlaxBertModel(config)
- The `__call__` method defines forward pass. It takes all necessary model inputs and parameters (and any other arguments required for the forward pass). The parameters are optional; when no parameters are passed, it uses the previously initialized or loaded parameters which can be accessed using `model.params`. It then calls the `module.apply` method, passing it the parameters and inputs to do the actual forward pass. So we can do a forward pass using
output = model(inputs, params=params)
Let's look at an example to see how this works. We will write a simple two-layer MLP model.
First, write a Flax module that will declare the layers and computation.
```python
import flax.linen as nn
import jax.numpy as jnp
class MLPModule(nn.Module):
config: MLPConfig
dtype: jnp.dtype = jnp.float32
def setup(self):
self.dense1 = nn.Dense(self.config.hidden_dim, dtype=self.dtype)
self.dense2 = nn.Desne(self.config.hidden_dim, dtype=self.dtype)
def __call__(self, inputs):
hidden_states = self.dense1(inputs)
hidden_states = nn.relu(hidden_states)
hidden_states = self.dense2(hidden_states)
return hidden_states
```
Now let's define the `FlaxPreTrainedModel` model class.
```python
from transformers.modeling_flax_utils import FlaxPreTrainedModel
class FlaxMLPPreTrainedModel(FlaxPreTrainedModel):
config_class = MLPConfig
base_model_prefix = "model"
module_class: nn.Module = None
def __init__(self, config: BertConfig, input_shape: Tuple = (1, 8), seed: int = 0, dtype: jnp.dtype = jnp.float32, **kwargs):
# initialize the flax module
module = self.module_class(config=config, dtype=dtype, **kwargs)
super().__init__(config, module, input_shape=input_shape, seed=seed, dtype=dtype)
def init_weights(self, rng, input_shape):
# init input tensors
inputs = jnp.zeros(input_shape, dtype="i4")
params_rng, dropout_rng = jax.random.split(rng)
rngs = {"params": params_rng, "dropout": dropout_rng}
params = self.module.init(rngs, inputs)["params"]
return params
def __call__(self, inputs, params: dict = None):
params = {"params": params or self.params}
outputs = self.module.apply(params, jnp.array(inputs))
return outputs
```
Now we can define our model class as follows.
```python
class FlaxMLPModel(FlaxMLPPreTrainedModel):
module_class = FlaxMLPModule
```
Now the `FlaxMLPModel` will have a similar interface as PyTorch or Tensorflow models and allows us to attach loaded or randomly initialized weights to the model instance.
So the important point to remember is that the `model` is not an instance of `nn.Module`; it's an abstract class, like a container that holds a Flax module, its parameters and provides convenient methods for initialization and forward pass. The key take-away here is that an instance of `FlaxMLPModel` is very much stateful now since it holds all the model parameters, whereas the underlying Flax module `FlaxMLPModule` is still stateless. Now to make `FlaxMLPModel` fully compliant with JAX transformations, it is always possible to pass the parameters to `FlaxMLPModel` as well to make it stateless and easier to work with during training. Feel free to take a look at the code to see how exactly this is implemented for ex. [`modeling_flax_bert.py`](https://github.com/huggingface/transformers/blob/main/src/transformers/models/bert/modeling_flax_bert.py#L536)
Another significant difference between Flax and PyTorch models is that, we can pass the `labels` directly to PyTorch's forward pass to compute the loss, whereas Flax models never accept `labels` as an input argument. In PyTorch, gradient backpropagation is performed by simply calling `.backward()` on the computed loss which makes it very handy for the user to be able to pass the `labels`. In Flax however, gradient backpropagation cannot be done by simply calling `.backward()` on the loss output, but the loss function itself has to be transformed by `jax.grad` or `jax.value_and_grad` to return the gradients of all parameters. This transformation cannot happen under-the-hood when one passes the `labels` to Flax's forward function, so that in Flax, we simply don't allow `labels` to be passed by design and force the user to implement the loss function oneself. As a conclusion, you will see that all training-related code is decoupled from the modeling code and always defined in the training scripts themselves.
### **How to use flax models and example scripts**
#### **How to do a forward pass**
Let's first see how to load, save and do inference with Flax models. As explained in the above section, all Flax models in Transformers have similar API to PyTorch models, so we can use the familiar `from_pretrained` and `save_pretrained` methods to load and save Flax models.
Let's use the base `FlaxRobertaModel` without any heads as an example.
```python
from transformers import FlaxRobertaModel, RobertaTokenizerFast
import jax
tokenizer = RobertaTokenizerFast.from_pretrained("roberta-base")
inputs = tokenizer("JAX/Flax is amazing ", padding="max_length", max_length=128, return_tensors="np")
model = FlaxRobertaModel.from_pretrained("julien-c/dummy-unknown")
@jax.jit
def run_model(input_ids, attention_mask):
# run a forward pass, should return an object `FlaxBaseModelOutputWithPooling`
return model(input_ids, attention_mask)
outputs = run_model(**inputs)
```
We use `jax.jit` to compile the function to get maximum performance. Note that in the above example, we set `padding=max_length` to pad all examples to the same length. We do this because JAX's compiler has to recompile a function everytime its input shape changes - in a sense a compiled function is not only defined by its code but also by its input and output shape. It is usually much more effective to pad the input to be of a fixed static shape than having to recompile every the function multiple times.
#### **How to write a training loop**
Now let's see how we can write a simple training loop to train Flax models, we will use `FlaxGPT2ForCausalLM` as an example.
A training loop for Flax models typically consists of
- A loss function that takes the parameters and inputs, runs the forward pass and returns the loss.
- We then transform the loss function using `jax.grad` or `jax.value_and_grad` so that we get the gradients of all parameters.
- An optimizer to update the paramteres using the gradients returned by the transformed loss function.
- A train step function which combines the loss function and optimizer update, does the forward and backward pass and returns the updated parameters.
Lets see how that looks like in code:
First initialize our model
```python
import jax
import jax.numpy as jnp
from transformers import FlaxGPT2ForCausalLM
model = FlaxGPT2ForCausalLM(config)
```
As explained above we don't compute the loss inside the model, but rather in the task-specific training script.
For demonstration purposes, we write a pseudo training script for causal language modeling in the following.
```python
from flax.training.common_utils import onehot
def cross_entropy(logits, labels):
return -jnp.sum(labels * jax.nn.log_softmax(logits, axis=-1), axis=-1)
# define a function which will run the forward pass return loss
def compute_loss(params, input_ids, labels):
logits = model(input_ids, params=params, train=True)
num_classes = logits.shape[-1]
loss = cross_entropy(logits, onehot(labels, num_classes)).mean()
return loss
```
Now we transform the loss function with `jax.value_and_grad`.
```python
# transform the loss function to get the gradients
grad_fn = jax.value_and_grad(compute_loss)
```
We use the [optax](https://github.com/deepmind/optax) library to Initialize the optimizer.
```python
import optax
params = model.params
tx = optax.sgd(learning_rate=3e-3)
opt_state = tx.init(params)
```
Now we define a single training step which will do a forward and a backward pass.
```python
def _train_step(params, opt_state, input_ids, labels)
# do the forward pass and get the loss and gradients
loss, grads = grad_fn(params, input_ids, labels)
# use the gradients to update parameters
updates, opt_state = tx.update(grads, opt_state)
updated_params = optax.apply_updates(params, updates)
return updates_params, opt_state, loss
train_step = jax.jit(_train_step)
```
Finally, let's run our training loop.
```python
# train loop
for i in range(10):
params, opt_state, loss = train_step(params, opt_state, input_ids, labels)
```
Note how we always pass the `params` and `opt_state` to the `train_step` which then returns the updated `params` and `opt_state`. This is because of the staless nature of JAX/Flax models, all the state
like parameters, optimizer state is kept external.
We can now save the model with the trained parameters using
```python
model.save_pretrained("awesome-flax-model", params=params)
```
Note that, as JAX is backed by the [XLA](https://www.tensorflow.org/xla) compiler any JAX/Flax code can run on all `XLA` compliant device without code change!
That menas you could use the same training script on CPUs, GPUs, TPUs.
To know more about how to train the Flax models on different devices (GPU, multi-GPUs, TPUs) and use the example scripts, please look at the [examples README](https://github.com/huggingface/transformers/tree/main/examples/flax).
## Talks
3 days of talks around JAX / Flax, Transformers, large-scale language modeling and other great topics during our community event!
### Wednesday, June 30th
- [Watch the talks on YouTube](https://www.youtube.com/watch?v=fuAyUQcVzTY)
- [Chat history](https://docs.google.com/spreadsheets/d/1PZ5xYV2hVwlAVQSqDag65ympv5YNCSDmXyG-eWTaZ_o/edit?usp=sharing)
Speaker | Topic | Time | Video |
|-------------|---------------------------------|------------------------|------------------------|
| Skye Wanderman-Milne, Google Brain | Intro to JAX on Cloud TPUs | 6.00pm-6.45pm CEST / 9.00am-9.45am PST | [](https://www.youtube.com/watch?v=fuAyUQcVzTY) |
| Marc van Zee, Google Brain | Introduction to Flax | 6.45pm-7.30pm CEST / 9.45am-10.30am PST | [](https://youtu.be/fuAyUQcVzTY?t=2569) |
| Pablo Castro, Google Brain | Using Jax & Flax for RL with the Dopamine library | 7.30pm-8.00pm CEST / 10.30am-11.00am PST | [](https://youtu.be/fuAyUQcVzTY?t=5306) |
### Thursday, July 1st
- [Watch the talks on YouTube](https://www.youtube.com/watch?v=__eG63ZP_5g)
- [Chat history](https://docs.google.com/spreadsheets/d/1PZ5xYV2hVwlAVQSqDag65ympv5YNCSDmXyG-eWTaZ_o/edit#gid=1515796400)
Speaker | Topic | Time | Video |
|-------------|---------------------------------|------------------------|------------------------|
| Suraj Patil & Patrick von Platen, Hugging Face | How to use JAX/Flax with Transformers | 5.30pm-6.00pm CEST / 8.30am-9.00am PST | [](https://www.youtube.com/watch?v=__eG63ZP_5g) |
| Sabrina J. Mielke, Johns Hopkins University & HuggingFace | From stateful code to purified JAX: how to build your neural net framework | 6.00pm-6.30pm CEST / 9.00am-9.30am PST | [](https://youtu.be/__eG63ZP_5g?t=1576) |
| Mostafa Dehghani, Google Brain | Long Range Arena: Benchmarking Efficient Transformers | 6.30pm-7.00pm CEST / 9.30am-10.00am PST | [](https://youtu.be/__eG63ZP_5g?t=3695) |
| Rohan Anil, Google Brain | Scalable Second Order Optimization for Deep Learning | 7.00pm-7.30pm CEST / 10.00am-10.30am PST | [](https://youtu.be/__eG63ZP_5g?t=5285) |
### Friday, July 2nd
- [Watch the talks on YouTube](https://www.youtube.com/watch?v=ZCMOPkcTu3s)
- [Chat history](https://docs.google.com/spreadsheets/d/1PZ5xYV2hVwlAVQSqDag65ympv5YNCSDmXyG-eWTaZ_o/edit#gid=1166061401)
Speaker | Topic | Time | Video |
|-------------|---------------------------------|------------------------|------------------------|
| Lucas Beyer, Google Brain | Vision Transformer | 5.00pm-5.30 CEST / 8.00am-8.30 PST | [](https://www.youtube.com/watch?v=ZCMOPkcTu3s) |
| Ben Wang, EleutherAI | Multihost Training in Mesh Transformer JAX | 5.30pm-6.00 CEST / 8.30am-9.00 PST | [](https://youtu.be/ZCMOPkcTu3s?t=1803) |
| Iurii Kemaev, Soňa Mokrá, Junhyuk Oh, DeepMind | DeepMind JAX Ecosystem | 6.00pm-6.30 CEST / 9.00am-9.30am PST | [](https://youtu.be/ZCMOPkcTu3s?t=3388) |
| Siddhartha Kamalakara, Joanna Yoo & João G M Araújo, Cohere | Training large scale language models | 6:30pm-7.00pm CEST / 9:30am-10.00am PST | [](https://youtu.be/ZCMOPkcTu3s?t=5095) |
### Talks & Speakers
#### Skye Wanderman-Milne, JAX developer, Google Brain
- Talk: Intro to JAX on Cloud TPUs
- Abstract: JAX is a system for high-performance machine-learning research that combines the familiarity of Python + NumPy together with the power of hardware acceleration on CPUs, GPUs, and TPUs. It offers composable function transformations for automatic differentiation, automatic batching, end-to-end compilation, and both data and model parallelism. This talk will show you how to get up and running with JAX on a Cloud TPU VM.
- Speaker info: Skye Wanderman-Milne is a software engineer working on JAX. She has previously worked on TensorFlow and Apache Impala, a high-performance distributed database.
#### Marc van Zee, Research SWE, Google Brain (Flax team)
- Talk: Introduction to Flax
- Abstract: In this talk I will provide a high-level introduction to the neural network library Flax. I will discuss the Flax philosophy, talk about the ecosystem around Flax and provide a high-level introduction to the code. I explain the Module abstraction and how to use it to train your models.
- Speaker info: Marc is at Google Research for over 4 years. First he worked on conceptual AI, developing a next generation language understanding and reasoning prototype and he authored the CFQ dataset for compositional generalization. Currently, Marc works as a research software engineer in the Flax team.
#### Pablo Castro, Staff Research Software Developer; Google Research, Brain Team
- Talk: Using Jax & Flax for RL with the Dopamine library
- Abstract: The Dopamine library was launched with TensorFlow in 2018 and we added a Jax/Flax variant of it last year. Internally, Jax's flexibility has facilitated our RL research tremendously, and we are excited to demonstrate its potential.
- Speaker info: Pablo Samuel has been at Google for over 9 years, and is currently a researcher with the Brain team, focusing on fundamental reinforcement learning, as well as machine learning and creativity. Aside from his research, Pablo Samuel is an active musician (with a channel exploring the intersection of music and computer science), and is helping increase the representation of the LatinX community in the research world.
- Dopamine repo: https://github.com/google/dopamine
- Homepage: https://psc-g.github.io/
- Twitter: https://twitter.com/pcastr
#### Suraj Patil & Patrick von Platen, Machine Learning Engineers at Hugging Face
- Talk: How to use JAX/Flax with Transformers
- Abstract: Transformers is one of the most popular open-source ML libraries and supports PyTorch, Tensorflow, and JAX/Flax. In this talk, we will explain how JAX/Flax models should be used in Transformers and compare their design in Transformers with the design of PyTorch models in Transformers. In the second part, we will give you a hands-on presentation of how a model can be trained end-to-end with the official JAX/Flax example scripts using Transformers & Datasets. Along the way, we want to give you some tips and tricks on how to best realize your project.
- Speaker info: Suraj and Patrick are part of Hugging Face’s open source team and lead the integration of JAX/Flax into Transformers.
- GitHub: https://github.com/patil-suraj & https://github.com/patrickvonplaten
#### Sabrina J. Mielke, PhD student at The Johns Hopkins University & Part-time research intern at HuggingFace
- Talk: From stateful code to purified JAX: how to build your neural net framework
- Abstract: Moving from object-oriented (and stateful) PyTorch- or TF2-code with tape-based backprop to JAX isn't easy---and while running grad() on numpy-oneliners is cool and all, you do wonder... how do I build actual big neural nets? Libraries like flax, trax, or haiku make it easy---but how could you build machinery like that yourself?
- Speaker info: Sabrina is a PhD student at the Johns Hopkins University and a part-time research intern at HuggingFace, researching open-vocabulary language models for segmentation and tokenization. She has published and co-organized workshops and shared tasks on these topics as well as on morphology and typological analysis in ACL, NAACL, EMNLP, LREC, and AAAI. You can find her reminisce for a time when formal language theory played a bigger role in NLP on Twitter at @sjmielke.
- Links: The 2020 blogpost this talk will be based on: https://sjmielke.com/jax-purify.htm, leading to our experiment Parallax and eventually Haiku
#### Mostafa Dehghani, Research Scientist, Google Brain
- Talk: Long Range Arena: Benchmarking Efficient Transformers
- Abstract: Transformers do not scale very well to long sequence lengths largely because of quadratic self-attention complexity. In the recent months, a wide spectrum of efficient, fast Transformers have been proposed to tackle this problem, more often than not claiming superior or comparable model quality to vanilla Transformer models. So, we now need a well-established consensus on how to evaluate this class of models. Moreover, inconsistent benchmarking on a wide spectrum of tasks and datasets makes it difficult to assess relative model quality amongst many models. I'll talk about a systematic and unified benchmark, LRA, specifically focused on evaluating model quality under long-context scenarios. LRA is a suite of tasks consisting of sequences ranging from 1K to 16K tokens, encompassing a wide range of data types and modalities such as text, natural, synthetic images, and mathematical expressions requiring similarity, structural, and visual-spatial reasoning. We systematically evaluate ten well-established long-range Transformer models (Reformers, Linformers, Linear Transformers, Sinkhorn Transformers, Performers, Synthesizers, Sparse Transformers, and Longformers) on LRA. LRA paves the way towards better understanding this class of efficient Transformer models, facilitates more research in this direction, and presents new challenging tasks to tackle.
- Speaker info: https://mostafadehghani.com/
#### Rohan Anil, Senior Staff Software Engineer, Google Research, Brain Team
- Talk: Scalable Second Order Optimization for Deep Learning
- Abstract: Optimization in machine learning, both theoretical and applied, is presently dominated by first-order gradient methods such as stochastic gradient descent. Second-order optimization methods, that involve second derivatives and/or second order statistics of the data, are far less prevalent despite strong theoretical properties, due to their prohibitive computation, memory and communication costs. In an attempt to bridge this gap between theoretical and practical optimization, we present a scalable implementation of a second-order preconditioned method (concretely, a variant of full-matrix Adagrad), that along with several critical algorithmic and numerical improvements, provides significant convergence and wall-clock time improvements compared to conventional first-order methods on state-of-the-art deep models. Our novel design effectively utilizes the prevalent heterogeneous hardware architecture for training deep models, consisting of a multicore CPU coupled with multiple accelerator units. We demonstrate superior performance compared to state-of-the-art on very large learning tasks such as machine translation with Transformers, language modeling with BERT, click-through rate prediction on Criteo, and image classification on ImageNet with ResNet-50.
- Speaker info: Rohan Anil is a software engineer at Google Research, Mountain View. Lately, he has been working on scalable and practical optimization techniques for efficient training of neural networks in various regimes.
- Resources:
- https://arxiv.org/abs/2002.09018
- https://arxiv.org/abs/1901.11150
- https://arxiv.org/abs/2106.06199
#### Lucas Beyer, Senior Research Engineer, Google Brain
- Talk: Vision Transformer
- Abstract: This talk will discuss the learning of general visual representations via large-scale pre-training and few-shot transfer, with a special focus on the Vision Transformer (ViT) architecture, which popularized transformers for the visual domain.
- Speaker info: Lucas Beyer is a self-taught hacker and studied engineer. He went on to do his PhD in robotic perception at RWTH Aachen and is currently on a quest to find the ultimate visual representation at Google Brain in Zürich
#### Ben Wang, Independent AI Researcher, EleutherAI
- Talk: Multihost Training in Mesh Transformer JAX
- Abstract: As models become larger, training must be scaled across multiple nodes. This talk discusses some design decisions and tradeoffs made for scaling to multiple nodes in Mesh Transformer JAX, a library for running model parallel transformers on TPU pods.
- Speaker info: Ben is an independent AI researcher who contributes to EleutherAI, an open source research collective centered around democratizing access to powerful AI models. Recently he has released GPT-J-6B, a 6 billion parameter transformer which is the most powerful autoregressive language model in terms of zero-shot performance with public weights.
- Website: https://www.eleuther.ai/
#### Iurii Kemaev, Research Engineer, Soňa Mokrá, Research Engineer, and Junhyuk Oh, Research Scientist, DeepMind
- Talk: DeepMind JAX Ecosystem
- Abstract: The DeepMind JAX Ecosystem is an effort to build a shared substrate of components to enable all aspects of AGI Research. In this talk, our researchers and engineers will give a high-level overview of our Ecosystem goals and design philosophies, using our Haiku (neural network), Optax (optimization) and RLax (reinforcement learning) libraries as examples. We will then deep dive on two examples of recent DeepMind research that have been enabled by JAX and these libraries: generative models and meta-gradient reinforcement learning.
- Speaker info:
- Iurii Kemaev is a Research Engineer at DeepMind. He has been using JAX for 2 years advancing RL research. Iurii is one of the DM JAX ecosystem leads.
- Soňa Mokrá is a Research Engineer at DeepMind. She has a background in machine translation and has been using JAX as the main ML framework for the past 6 months.
- Junhyuk Oh is a Research Scientist at DeepMind, working on reinforcement learning and meta-learning. More information is available at https://junhyuk.com/
#### Siddhartha Kamalakara, Joanna Yoo, João G M Araújo, MLE at Cohere
- Talk: Training large scale language models
- Abstract: A journey through Cohere’s experiences with training large scale language models. Join us in our exploration of pipeline and model parallelism as strategies for efficient training of large language models. We will present and motivate our recent transition to JAX+Flax as our choice of internal tech stack.
- Speaker info:
- João G M Araújo is a Brazilian college student with a passion for mathematics and a fascination for Deep Learning. João conducted research on representation learning and spent 3 months in Japan working on NeuroEvolution. João likes reading fantasy books and spending quality time with family and friends, and also runs a YouTube series on theoretical understanding of Deep Learning where researchers talk about their findings
- Joanna Yoo is one of the founding engineers at Cohere, working on scaling language models for the last year and half. Joanna loves live concerts and rock climbing!
- Siddhartha Rao Kamalakara is an MLE at Cohere and a researcher at FOR.ai with research interests at the intersection of efficient training and empirical understanding of DL.
- Website: https://cohere.ai/
## How to use the hub for collaboration
In this section, we will explain how a team can use the 🤗 hub to collaborate on a project.
The 🤗 hub allows each team to create a repository with integrated git version control that
should be used for their project.
The advantages of using a repository on the 🤗 hub are:
- easy collaboration - each team member has write access to the model repository
- integrated git version control - code scripts as well as large model files are tracked using git version control
- easy sharing - the hub allows each team to easily share their work during and after the event
- integrated tensorboard functionality - uploaded tensorboard traces are automatically displayed on an integrated tensorboard tab
We highly recommend each team to make use of the 🤗 hub during the event.
To better understand how the repository and the hub in general functions, please take a look at the documentation and the videos [here](https://huggingface.co/docs/hub).
Now let's explain in more detail how a project can be created on the hub. Having an officially defined project on [this](https://docs.google.com/spreadsheets/d/1GpHebL7qrwJOc9olTpIPgjf8vOS0jNb6zR_B8x_Jtik/edit?usp=sharing) Google Sheet you should be part of [the Flax Community organization on the hub](https://huggingface.co/flax-community). All repositories should be created under this organization so that write access can be shared and everybody can easily access other participants'
work 🤗. Note that we are giving each team member access to all repositories created under [flax-community](https://huggingface.co/flax-community), but we encourage participants to only clone and edit repositories corresponding to one's teams. If you want to help other teams, please ask them before changing files in their repository! The integrated git version control keeps track of
all changes, so in case a file was deleted by mistake, it is trivial to re-create it.
Awesome! Now, let's first go over a simple example where most of the required we'll pre-train a RoBERTa model on a low-resource language. To begin with, we create a repository
under [the Flax Community organization on the hub](https://huggingface.co/flax-community) by logging in to the hub and going to [*"Add model"*](https://huggingface.co/new). By default
the username should be displayed under "*Owner*", which we want to change to *flax-community*. Next, we give our repository a fitting name for the project - here we'll just call it
*roberta-base-als* because we'll be pretraining a RoBERTa model on the super low-resource language *Alemannic* (`als`). We make sure that the model is a public repository and create it!
It should then be displayed on [the Flax Community organization on the hub](https://huggingface.co/flax-community).
Great, now we have a project directory with integrated git version control and a public model page, which we can access under [flax-community/roberta-base-als](https://huggingface.co/flax-community/roberta-base-als). Let's create a short README so that other participants know what this model is about. You can create the README.md directly on the model page as a markdown file.
Let's now make use of the repository for training.
We assume that the 🤗 Transformers library and [git-lfs](https://git-lfs.github.com/) are correctly installed on our machine or the TPU attributed to us.
If this is not the case, please refer to the [Installation guide](#how-to-install-relevant-libraries) and the official [git-lfs](https://git-lfs.github.com/) website.
At first we should log in:
```bash
$ huggingface-cli login
```
Next we can clone the repo:
```bash
$ git clone https://huggingface.co/flax-community/roberta-base-als
```
We have now cloned the model's repository and it should be under `roberta-base-als`. As you can see,
we have all the usual git functionalities in this repo - when adding a file, we can do `git add .`, `git commit -m "add file"` and `git push`
as usual. Let's try it out by adding the model's config.
We go into the folder:
```bash
$ cd ./roberta-base-als
```
and run the following commands in a Python shell to save a config.
```python
from transformers import RobertaConfig
config = RobertaConfig.from_pretrained("roberta-base")
config.save_pretrained("./")
```
Now we've added a `config.json` file and can upload it by running
```bash
$ git add . && git commit -m "add config" && git push
```
Cool! The file is now displayed on the model page under the [files tab](https://huggingface.co/flax-community/roberta-base-als/tree/main).
We encourage you to upload all files except maybe the actual data files to the repository. This includes training scripts, model weights,
model configurations, training logs, etc...
Next, let's create a tokenizer and save it to the model dir by following the instructions of the [official Flax MLM README](https://github.com/huggingface/transformers/tree/main/examples/flax/language-modeling#train-tokenizer). We can again use a simple Python shell.
```python
from datasets import load_dataset
from tokenizers import ByteLevelBPETokenizer
# load dataset
dataset = load_dataset("oscar", "unshuffled_deduplicated_als", split="train")
# Instantiate tokenizer
tokenizer = ByteLevelBPETokenizer()
def batch_iterator(batch_size=1000):
for i in range(0, len(dataset), batch_size):
yield dataset[i: i + batch_size]["text"]
# Customized training
tokenizer.train_from_iterator(batch_iterator(), vocab_size=50265, min_frequency=2, special_tokens=[
"<s>",
"<pad>",
"</s>",
"<unk>",
"<mask>",
])
# Save files to disk
tokenizer.save("./tokenizer.json")
```
This creates and saves our tokenizer directly in the cloned repository.
Finally, we can start training. For now, we'll simply use the official [`run_mlm_flax`](https://github.com/huggingface/transformers/blob/main/examples/flax/language-modeling/run_mlm_flax.py)
script, but we might make some changes later. So let's copy the script into our model repository.
```bash
$ cp ~/transformers/examples/flax/language-modeling/run_mlm_flax.py ./
```
This way we are certain to have all the code used to train the model tracked in our repository.
Let's start training by running:
```bash
./run_mlm_flax.py \
--output_dir="./" \
--model_type="roberta" \
--config_name="./" \
--tokenizer_name="./" \
--dataset_name="oscar" \
--dataset_config_name="unshuffled_deduplicated_als" \
--max_seq_length="128" \
--per_device_train_batch_size="4" \
--per_device_eval_batch_size="4" \
--learning_rate="3e-4" \
--warmup_steps="1000" \
--overwrite_output_dir \
--num_train_epochs="8" \
--push_to_hub
```
Since the dataset is tiny this command should actually run in less than 5 minutes. Note that we attach
the flag ``--push_to_hub`` so that both model weights and tensorboard traces are automatically uploaded to the hub.
You can see the tensorboard directly on the model page, under the [Training metrics tab](https://huggingface.co/flax-community/roberta-base-als/tensorboard).
As you can see, it is pretty simple to upload model weights and training logs to the model hub. Since the repository
has git version control, you & your team probably already have the necessary skills to collaborate. Thanks
to `git-lfs` being integrated into the hub, model weights and other larger file can just as easily be uploaded
and changed. Finally, at Hugging Face, we believe that the model hub is a great platform to share your project
while you are still working on it:
- Bugs in training scripts can be found and corrected by anybody participating in the event
- Loss curves can be analyzed directly on the model page
- Model weights can be accessed and analyzed by everybody from the model repository
If you are not using a transformers model, don't worry - you should still be able to make use of the hub's functionalities!
The [huggingface_hub](https://github.com/huggingface/huggingface_hub) allows you to upload essentially any JAX/Flax model to the hub with
just a couple of lines of code. *E.g.* assuming you want to call your model simply `flax-model-dummy`, you can upload it to the hub with
just three lines of code:
```python
from flax import serialization
from jax import random
from flax import linen as nn
from huggingface_hub import Repository
model = nn.Dense(features=5)
key1, key2 = random.split(random.PRNGKey(0))
x = random.normal(key1, (10,))
params = model.init(key2, x)
bytes_output = serialization.to_bytes(params)
repo = Repository("flax-model", clone_from="flax-community/flax-model-dummy", use_auth_token=True)
with repo.commit("My cool Flax model :)"):
with open("flax_model.msgpack", "wb") as f:
f.write(bytes_output)
# Repo is created and available here: https://huggingface.co/flax-community/flax-model-dummy
```
**Note**: Make sure to have `huggingface_hub >= 0.0.13` to make this command work.
For more information, check out [this PR](https://github.com/huggingface/huggingface_hub/pull/143) on how to upload any framework to the hub.
## How to setup TPU VM
In this section we will explain how you can ssh into a TPU VM that has been given to your team.
If your username is in one of the officially defined projects [here](https://docs.google.com/spreadsheets/d/1GpHebL7qrwJOc9olTpIPgjf8vOS0jNb6zR_B8x_Jtik/edit?usp=sharing), you should have received two emails:
- one that states that you have been granted the role "Community Week Participants" for the project hf-flax, and
- one (or more if you are in multiple projects) that gives you the TPU name and the TPU zone for the TPU of your team
You should click on "Open Cloud Console" on the first mail and agree to the pop up windows that follows. It will allow you to use a TPU VM. Don't worry if you cannot access the actual project `hf-flax` visually on the google cloud console and receive an error:
```
You don't have sufficient permission to view this page
```
- this is expected!
Great, now you and your team can access your TPU VM!
In the following, we will describe how to do so using a standard console, but you should also be able to connect to the TPU VM via IDEs, like Visual Studio Code, etc.
1. You need to install the Google Cloud SDK. Please follow the instructions on [cloud.google.com/sdk](https://cloud.google.com/sdk/docs/install#linux).
2. Once you've installed the google cloud sdk, you should set your account by running the following command. Make sure that `<your-email-address>` corresponds to the gmail address you used to sign up for this event.
```bash
$ gcloud config set account <your-email-adress>
```
3. Let's also make sure the correct project is set in case your email is used for multiple gcloud projects:
```bash
$ gcloud config set project hf-flax
```
4. Next, you will need to authenticate yourself. You can do so by running:
```bash
$ gcloud auth login
```
This should give you a link to a website, where you can authenticate your gmail account.
5. Finally, you can ssh into the TPU VM! Please run the following command by setting <zone> to either `europe-west4-a` or `us-central1-a` (depending on what is stated in the second email you received) and <tpu-name> to the TPU name also sent to you in the second email.
```bash
$ gcloud alpha compute tpus tpu-vm ssh <tpu-name> --zone <zone> --project hf-flax
```
This should ssh you into the TPU VM!
Now you can follow the steps of the section [How to install relevant libraries](#how-to-install-relevant-libraries) to install all necessary
libraries. Make sure to carefully follow the explanations of the "**IMPORTANT**" statement to correctly install JAX on TPU.
Also feel free to install other `python` or `apt` packages on your machine if it helps you to work more efficiently!
## How to build a demo
### Using the Hugging Face Widgets
Hugging Face has over [15 widgets](https://huggingface-widgets.netlify.app/) for different use cases using 🤗 Transformers library. Some of them also support [3rd party libraries](https://huggingface.co/docs/hub/libraries) such as [Sentence Similarity](https://huggingface.co/sentence-transformers/paraphrase-xlm-r-multilingual-v1) with Sentence Transformers and [Text to Speech](https://huggingface.co/julien-c/ljspeech_tts_train_tacotron2_raw_phn_tacotron_g2p_en_no_space_train) with [ESPnet](https://github.com/espnet/espnet).
All the widgets are open sourced in the `huggingface_hub` [repo](https://github.com/huggingface/huggingface_hub/tree/main/widgets). Here is a summary of existing widgets:
**NLP**
* **Conversational:** To have the best conversations!. [Example](https://huggingface.co/microsoft/DialoGPT-large?).
* **Feature Extraction:** Retrieve the input embeddings. [Example](https://huggingface.co/sentence-transformers/distilbert-base-nli-mean-tokens?text=test).
* **Fill Mask:** Predict potential words for a mask token. [Example](https://huggingface.co/bert-base-uncased?).
* **Question Answering:** Given a context and a question, predict the answer. [Example](https://huggingface.co/bert-large-uncased-whole-word-masking-finetuned-squad).
* **Sentence Simmilarity:** Predict how similar a set of sentences are. Useful for Sentence Transformers.
* **Summarization:** Given a text, output a summary of it. [Example](https://huggingface.co/sshleifer/distilbart-cnn-12-6).
* **Table Question Answering:** Given a table and a question, predict the answer. [Example](https://huggingface.co/google/tapas-base-finetuned-wtq).
* **Text Generation:** Generate text based on a prompt. [Example](https://huggingface.co/gpt2)
* **Token Classification:** Useful for tasks such as Named Entity Recognition and Part of Speech. [Example](https://huggingface.co/dslim/bert-base-NER).
* **Zero-Shot Classification:** Too cool to explain with words. Here is an [example](https://huggingface.co/typeform/distilbert-base-uncased-mnli)
* ([WIP](https://github.com/huggingface/huggingface_hub/issues/99)) **Table to Text Generation**.
**Speech**
* **Audio to Audio:** For tasks such as audio source separation or speech enhancement.
* **Automatic Speech Recognition:** Convert audio to text. [Example](https://huggingface.co/facebook/wav2vec2-base-960h)
* **Text to Speech**: Convert text to audio.
**Image**
* **Image Classification:** Given an image, predict its class. [Example](https://huggingface.co/osanseviero/llamastic).
* ([WIP](https://github.com/huggingface/huggingface_hub/issues/100)) **Zero Shot Image Classification**
* ([WIP](https://github.com/huggingface/huggingface_hub/issues/112)) **Image Captioning**
* ([WIP](https://github.com/huggingface/huggingface_hub/issues/113)) **Text to Image Generation**
* ([Proposed](https://github.com/huggingface/huggingface_hub/issues/127)) **Visual Question Answering**
You can propose and implement new widgets by [opening an issue](https://github.com/huggingface/huggingface_hub/issues). Contributions are welcomed!
### Using a Streamlit demo
Sometimes you might be using different libraries or a very specific application that is not well supported by the current widgets. In this case, [Streamlit](https://streamlit.io/) can be an excellent option to build a cool visual demo. Setting up a Streamlit application is straightforward and in Python!
A common use case is how to load files you have in your model repository in the Hub from the Streamlit demo. The `huggingface_hub` library is here to help you!
```
pip install huggingface_hub
```
Here is an example downloading (and caching!) a specific file directly from the Hub
```
from huggingface_hub import hf_hub_download
filepath = hf_hub_download("flax-community/roberta-base-als", "flax_model.msgpack");
```
In many cases you will want to download the full repository. Here is an example downloading all the files from a repo. You can even specify specific revisions!
```
from huggingface_hub import snapshot_download
local_path = snapshot_download("flax-community/roberta-base-als");
```
Note that if you're using 🤗 Transformers library, you can quickly load the model and tokenizer as follows
```
from transformers import AutoTokenizer, AutoModelForMaskedLM
tokenizer = AutoTokenizer.from_pretrained("REPO_ID")
model = AutoModelForMaskedLM.from_pretrained("REPO_ID")
```
We'll provide more examples on Streamlit demos next week. Stay tuned!
### Using a Gradio demo
You can also use [Gradio](https://gradio.app/) to share your demos! [Here](https://huggingface.co/blog/gradio) is an example using the Gradio library to create a GUI for a Hugging Face model.
More to come!
## Project evaluation
For your project to be evaluated, please fill out [this google form](https://forms.gle/jQaMkj3JJdD4Xcwn9).
Please make sure that your submitted project includes a demo as well as information about the model, data, training methods, etc.
### Criteria
* **Demo.** All projects are required to have a demo. It’s open ended, but we provide some ideas on how to build demos in the [How to build a demo](#how-to-build-a-demo) section.
* **Technical difficulty.** Difficulty has different aspects, such as working with complex architectures, obtaining better evaluation metrics than existing models, or implementing models for low-resource languages.
* **Social impact.** The project is expected to have a positive social impact, e.g. by tackling under-explored area of practical interest for minorities or under-represented group (low-ressources languages, specific focus on bias, fairness or ethical issues in ML) or by tackling general societal challenges, e.g. health or climate related challenges.
* **Innovativeness.** Projects that propose novel applications or bring new ideas will be rewarded more.
### Jury
* [Niki Parmar](https://research.google/people/NikiParmar/): Staff Research Scientist at Google.
* [Ross Wightman](https://www.linkedin.com/in/wightmanr/): Angel Investor.
* [Thomas Wolf](https://www.linkedin.com/in/thomas-wolf-a056857/): Co-founder and CSO at Hugging Face.
* [Ashish Vaswani](https://research.google/people/AshishVaswani/): Staff Research Scientist at Google Brain.
### Process
* **July 17, 12h00 CEST**: TPU VM access closes.
* **July 19, 12h00 CEST**: Project completition ends (including demo).
* **July 19-21** A group of event organizers (Suraj, Patrick, Suzana, and Omar) will do an initial filter to find the top 15 projects.
* **July 22-26** The jury will go over the 15 projects and pick the top three projects out of them.
* **July 27.** Winner projects are announced
## General tips and tricks
TODO (will be filled continuously)...
## FAQ
TODO (will be filled continuously)...
|