Spaces:
Paused
Paused
File size: 19,745 Bytes
ee6e328 |
1 2 3 4 5 6 7 8 9 10 11 12 13 14 15 16 17 18 19 20 21 22 23 24 25 26 27 28 29 30 31 32 33 34 35 36 37 38 39 40 41 42 43 44 45 46 47 48 49 50 51 52 53 54 55 56 57 58 59 60 61 62 63 64 65 66 67 68 69 70 71 72 73 74 75 76 77 78 79 80 81 82 83 84 85 86 87 88 89 90 91 92 93 94 95 96 97 98 99 100 101 102 103 104 105 106 107 108 109 110 111 112 113 114 115 116 117 118 119 120 121 122 123 124 125 126 127 128 129 130 131 132 133 134 135 136 137 138 139 140 141 142 143 144 145 146 147 148 149 150 151 152 153 154 155 156 157 158 159 160 161 162 163 164 165 166 167 168 169 170 171 172 173 174 175 176 177 178 179 180 181 182 183 184 185 186 187 188 189 190 191 192 193 194 195 196 197 198 199 200 201 202 203 204 205 206 207 208 209 210 211 212 213 214 215 216 217 218 219 220 221 222 223 224 225 226 227 228 229 230 231 232 233 234 235 236 237 238 239 240 241 242 243 244 245 246 247 248 249 250 251 252 253 254 255 256 257 258 259 260 261 262 263 264 265 266 267 268 269 270 271 272 273 274 275 276 277 278 279 280 281 282 283 284 285 286 287 288 289 290 291 292 293 294 295 296 297 298 299 300 301 302 303 304 305 306 307 308 309 310 311 312 313 314 315 316 317 318 319 320 321 322 323 324 325 326 327 328 329 330 331 332 333 334 335 336 337 338 339 340 341 342 343 344 345 346 347 348 349 350 351 352 353 354 355 356 357 358 359 360 361 362 363 364 365 366 367 368 369 370 371 372 373 374 375 376 377 378 379 380 381 382 383 384 385 386 387 388 389 390 391 392 393 394 395 396 397 398 399 400 401 402 403 404 405 406 407 408 409 410 411 412 413 414 415 416 417 418 419 420 421 422 423 424 425 426 427 428 429 430 431 432 433 434 435 |
## Sequence to Sequence Training and Evaluation
This directory contains examples for finetuning and evaluating transformers on summarization and translation tasks.
Author: Sam Shleifer (https://github.com/sshleifer)
### Supported Architectures
- `BartForConditionalGeneration` (and anything that inherits from it)
- `MarianMTModel`
- `PegasusForConditionalGeneration`
- `MBartForConditionalGeneration`
- `FSMTForConditionalGeneration`
- `T5ForConditionalGeneration`
# Note
β οΈ This project should be run with pytorch-lightning==1.0.4 which has a potential security vulnerability
## Datasets
#### XSUM
```bash
cd examples/contrib/pytorch-lightning/seq2seq
wget https://cdn-datasets.huggingface.co/summarization/xsum.tar.gz
tar -xzvf xsum.tar.gz
export XSUM_DIR=${PWD}/xsum
```
this should make a directory called `xsum/` with files like `test.source`.
To use your own data, copy that files format. Each article to be summarized is on its own line.
#### CNN/DailyMail
```bash
cd examples/contrib/pytorch-lightning/seq2seq
wget https://cdn-datasets.huggingface.co/summarization/cnn_dm_v2.tgz
tar -xzvf cnn_dm_v2.tgz # empty lines removed
mv cnn_cln cnn_dm
export CNN_DIR=${PWD}/cnn_dm
```
this should make a directory called `cnn_dm/` with 6 files.
#### WMT16 English-Romanian Translation Data
download with this command:
```bash
wget https://cdn-datasets.huggingface.co/translation/wmt_en_ro.tar.gz
tar -xzvf wmt_en_ro.tar.gz
export ENRO_DIR=${PWD}/wmt_en_ro
```
this should make a directory called `wmt_en_ro/` with 6 files.
#### WMT English-German
```bash
wget https://cdn-datasets.huggingface.co/translation/wmt_en_de.tgz
tar -xzvf wmt_en_de.tgz
export DATA_DIR=${PWD}/wmt_en_de
```
#### FSMT datasets (wmt)
Refer to the scripts starting with `eval_` under:
https://github.com/huggingface/transformers/tree/main/scripts/fsmt
#### Pegasus (multiple datasets)
Multiple eval datasets are available for download from:
https://github.com/stas00/porting/tree/master/datasets/pegasus
#### Your Data
If you are using your own data, it must be formatted as one directory with 6 files:
```
train.source
train.target
val.source
val.target
test.source
test.target
```
The `.source` files are the input, the `.target` files are the desired output.
### Potential issues
- native AMP (`--fp16` and no apex) may lead to a huge memory leak and require 10x gpu memory. This has been fixed in pytorch-nightly and the minimal official version to have this fix will be pytorch-1.8. Until then if you have to use mixed precision please use AMP only with pytorch-nightly or NVIDIA's apex. Reference: https://github.com/huggingface/transformers/issues/8403
### Tips and Tricks
General Tips:
- since you need to run from this folder, and likely need to modify code, the easiest workflow is fork transformers, clone your fork, and run `pip install -e .` before you get started.
- try `--freeze_encoder` or `--freeze_embeds` for faster training/larger batch size. (3hr per epoch with bs=8, see the "xsum_shared_task" command below)
- `fp16_opt_level=O1` (the default works best).
- In addition to the pytorch-lightning .ckpt checkpoint, a transformers checkpoint will be saved.
Load it with `BartForConditionalGeneration.from_pretrained(f'{output_dir}/best_tfmr)`.
- At the moment, `--do_predict` does not work in a multi-gpu setting. You need to use `evaluate_checkpoint` or the `run_eval.py` code.
- This warning can be safely ignored:
> "Some weights of BartForConditionalGeneration were not initialized from the model checkpoint at facebook/bart-large-xsum and are newly initialized: ['final_logits_bias']"
- Both finetuning and eval are 30% faster with `--fp16`. For that you need to [install apex](https://github.com/NVIDIA/apex#quick-start).
- Read scripts before you run them!
Summarization Tips:
- (summ) 1 epoch at batch size 1 for bart-large takes 24 hours and requires 13GB GPU RAM with fp16 on an NVIDIA-V100.
- If you want to run experiments on improving the summarization finetuning process, try the XSUM Shared Task (below). It's faster to train than CNNDM because the summaries are shorter.
- For CNN/DailyMail, the default `val_max_target_length` and `test_max_target_length` will truncate the ground truth labels, resulting in slightly higher rouge scores. To get accurate rouge scores, you should rerun calculate_rouge on the `{output_dir}/test_generations.txt` file saved by `trainer.test()`
- `--max_target_length=60 --val_max_target_length=60 --test_max_target_length=100 ` is a reasonable setting for XSUM.
- `wandb` can be used by specifying `--logger_name wandb`. It is useful for reproducibility. Specify the environment variable `WANDB_PROJECT='hf_xsum'` to do the XSUM shared task.
- If you are finetuning on your own dataset, start from `distilbart-cnn-12-6` if you want long summaries and `distilbart-xsum-12-6` if you want short summaries.
(It rarely makes sense to start from `bart-large` unless you are a researching finetuning methods).
**Update 2018-07-18**
Datasets: `LegacySeq2SeqDataset` will be used for all tokenizers without a `prepare_seq2seq_batch` method. Otherwise, `Seq2SeqDataset` will be used.
Future work/help wanted: A new dataset to support multilingual tasks.
### Finetuning Scripts
All finetuning bash scripts call finetune.py (or distillation.py) with reasonable command line arguments. They usually require extra command line arguments to work.
To see all the possible command line options, run:
```bash
./finetune.py --help
```
### Finetuning Training Params
To override the pretrained model's training params, you can pass them to `./finetune.sh`:
```bash
./finetune.sh \
[...]
--encoder_layerdrop 0.1 \
--decoder_layerdrop 0.1 \
--dropout 0.1 \
--attention_dropout 0.1 \
```
### Summarization Finetuning
Run/modify `finetune.sh`
The following command should work on a 16GB GPU:
```bash
./finetune.sh \
--data_dir $XSUM_DIR \
--train_batch_size=1 \
--eval_batch_size=1 \
--output_dir=xsum_results \
--num_train_epochs 6 \
--model_name_or_path facebook/bart-large
```
There is a starter finetuning script for pegasus at `finetune_pegasus_xsum.sh`.
### Translation Finetuning
First, follow the wmt_en_ro download instructions.
Then you can finetune mbart_cc25 on english-romanian with the following command.
**Recommendation:** Read and potentially modify the fairly opinionated defaults in `train_mbart_cc25_enro.sh` script before running it.
Best performing command:
```bash
# optionally
export ENRO_DIR='wmt_en_ro' # Download instructions above
# export WANDB_PROJECT="MT" # optional
export MAX_LEN=128
export BS=4
./train_mbart_cc25_enro.sh --output_dir enro_finetune_baseline --label_smoothing 0.1 --fp16_opt_level=O1 --logger_name wandb --sortish_sampler
```
This should take < 6h/epoch on a 16GB v100 and achieve test BLEU above 26
To get results in line with fairseq, you need to do some postprocessing. (see `romanian_postprocessing.md`)
MultiGPU command
(using 8 GPUS as an example)
```bash
export ENRO_DIR='wmt_en_ro' # Download instructions above
# export WANDB_PROJECT="MT" # optional
export MAX_LEN=128
export BS=4
./train_mbart_cc25_enro.sh --output_dir enro_finetune_baseline --gpus 8 --logger_name wandb
```
### Finetuning Outputs
As you train, `output_dir` will be filled with files, that look kind of like this (comments are mine).
Some of them are metrics, some of them are checkpoints, some of them are metadata. Here is a quick tour:
```bash
output_dir
βββ best_tfmr # this is a huggingface checkpoint generated by save_pretrained. It is the same model as the PL .ckpt file below
β βββ config.json
β βββ merges.txt
β βββ pytorch_model.bin
β βββ special_tokens_map.json
β βββ tokenizer_config.json
β βββ vocab.json
βββ git_log.json # repo, branch, and commit hash
βββ val_avg_rouge2=0.1984-step_count=11.ckpt # this is a pytorch lightning checkpoint associated with the best val score. (it will be called BLEU for MT)
βββ metrics.json # new validation metrics will continually be appended to this
βββ student # this is a huggingface checkpoint generated by SummarizationDistiller. It is the student before it gets finetuned.
β βββ config.json
β βββ pytorch_model.bin
βββ test_generations.txt
# ^^ are the summaries or translations produced by your best checkpoint on the test data. Populated when training is done
βββ test_results.txt # a convenience file with the test set metrics. This data is also in metrics.json['test']
βββ hparams.pkl # the command line args passed after some light preprocessing. Should be saved fairly quickly.
```
After training, you can recover the best checkpoint by running
```python
from transformers import AutoModelForSeq2SeqLM
model = AutoModelForSeq2SeqLM.from_pretrained(f'{output_dir}/best_tfmr')
```
### Converting pytorch-lightning checkpoints
pytorch lightning ``-do_predict`` often fails, after you are done training, the best way to evaluate your model is to convert it.
This should be done for you, with a file called `{save_dir}/best_tfmr`.
If that file doesn't exist but you have a lightning `.ckpt` file, you can run
```bash
python convert_pl_checkpoint_to_hf.py PATH_TO_CKPT randomly_initialized_hf_model_path save_dir/best_tfmr
```
Then either `run_eval` or `run_distributed_eval` with `save_dir/best_tfmr` (see previous sections)
# Experimental Features
These features are harder to use and not always useful.
### Dynamic Batch Size for MT
`finetune.py` has a command line arg `--max_tokens_per_batch` that allows batches to be dynamically sized.
This feature can only be used:
- with fairseq installed
- on 1 GPU
- without sortish sampler
- after calling `./save_len_file.py $tok $data_dir`
For example,
```bash
./save_len_file.py Helsinki-NLP/opus-mt-en-ro wmt_en_ro
./dynamic_bs_example.sh --max_tokens_per_batch=2000 --output_dir benchmark_dynamic_bs
```
splits `wmt_en_ro/train` into 11,197 uneven lengthed batches and can finish 1 epoch in 8 minutes on a v100.
For comparison,
```bash
./dynamic_bs_example.sh --sortish_sampler --train_batch_size 48
```
uses 12,723 batches of length 48 and takes slightly more time 9.5 minutes.
The feature is still experimental, because:
+ we can make it much more robust if we have memory mapped/preprocessed datasets.
+ The speedup over sortish sampler is not that large at the moment.
# DistilBART
<!---It should be called distilling bart and pegasus, but I don't want to break the link in the paper.-->
This section describes all code and artifacts from our [Paper](http://arxiv.org/abs/2010.13002)
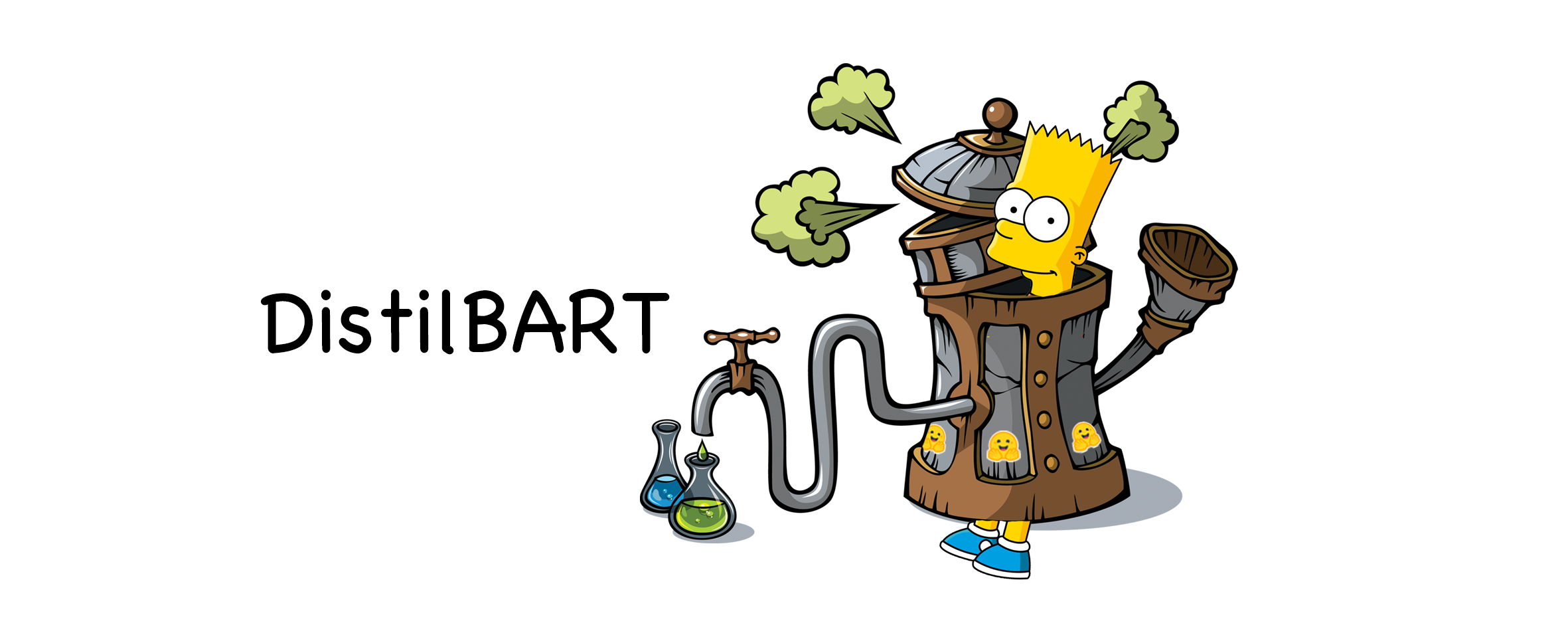
+ For the CNN/DailyMail dataset, (relatively longer, more extractive summaries), we found a simple technique that works, which we call "Shrink and Fine-tune", or SFT.
you just copy alternating layers from `facebook/bart-large-cnn` and fine-tune more on the cnn/dm data. `sshleifer/distill-pegasus-cnn-16-4`, `sshleifer/distilbart-cnn-12-6` and all other checkpoints under `sshleifer` that start with `distilbart-cnn` were trained this way.
+ For the XSUM dataset, training on pseudo-labels worked best for Pegasus (`sshleifer/distill-pegasus-16-4`), while training with KD worked best for `distilbart-xsum-12-6`
+ For `sshleifer/dbart-xsum-12-3`
+ We ran 100s experiments, and didn't want to document 100s of commands. If you want a command to replicate a figure from the paper that is not documented below, feel free to ask on the [forums](https://discuss.huggingface.co/t/seq2seq-distillation-methodology-questions/1270) and tag `@sshleifer`.
+ You can see the performance tradeoffs of model sizes [here](https://docs.google.com/spreadsheets/d/1EkhDMwVO02m8jCD1cG3RoFPLicpcL1GQHTQjfvDYgIM/edit#gid=0).
and more granular timing results [here](https://docs.google.com/spreadsheets/d/1EkhDMwVO02m8jCD1cG3RoFPLicpcL1GQHTQjfvDYgIM/edit#gid=1753259047&range=B2:I23).
### Evaluation
use [run_distributed_eval](./run_distributed_eval.py), with the following convenient alias
```bash
deval () {
proc=$1
m=$2
dd=$3
sd=$4
shift
shift
shift
shift
python -m torch.distributed.launch --nproc_per_node=$proc run_distributed_eval.py \
--model_name $m --save_dir $sd --data_dir $dd $@
}
```
On a 1 GPU system, here are four commands (that assume `xsum`, `cnn_dm` are downloaded, cmd-F for those links in this file).
`distilBART`:
```bash
deval 1 sshleifer/distilbart-xsum-12-3 xsum dbart_12_3_xsum_eval --fp16 # --help for more choices.
deval 1 sshleifer/distilbart-cnn_dm-12-6 cnn_dm dbart_12_6_cnn_eval --fp16
```
`distill-pegasus`:
```bash
deval 1 sshleifer/distill-pegasus-cnn-16-4 cnn_dm dpx_cnn_eval
deval 1 sshleifer/distill-pegasus-xsum-16-4 xsum dpx_xsum_eval
```
### Distillation
+ For all of the following commands, you can get roughly equivalent result and faster run times by passing `--num_beams=4`. That's not what we did for the paper.
+ Besides the KD section, you can also run commands with the built-in transformers trainer. See, for example, [builtin_trainer/train_distilbart_cnn.sh](./builtin_trainer/train_distilbart_cnn.sh).
+ Large performance deviations (> 5X slower or more than 0.5 Rouge-2 worse), should be reported.
+ Multi-gpu (controlled with `--gpus` should work, but might require more epochs).
#### Recommended Workflow
+ Get your dataset in the right format. (see 6 files above).
+ Find a teacher model [Pegasus](https://huggingface.co/models?search=pegasus) (slower, better ROUGE) or `facebook/bart-large-xsum`/`facebook/bart-large-cnn` (faster, slightly lower.).
Choose the checkpoint where the corresponding dataset is most similar (or identical to) your dataset.
+ Follow the sections in order below. You can stop after SFT if you are satisfied, or move on to pseudo-labeling if you want more performance.
+ student size: If you want a close to free 50% speedup, cut the decoder in half. If you want a larger speedup, cut it in 4.
+ If your SFT run starts at a validation ROUGE-2 that is more than 10 pts below the teacher's validation ROUGE-2, you have a bug. Switching to a more expensive technique will not help. Try setting a breakpoint and looking at generation and truncation defaults/hyper-parameters, and share your experience on the forums!
#### Initialization
We use [make_student.py](./make_student.py) to copy alternating layers from the teacher, and save the resulting model to disk
```bash
python make_student.py facebook/bart-large-xsum --save_path dbart_xsum_12_3 -e 12 -d 3
```
or for `pegasus-xsum`
```bash
python make_student.py google/pegasus-xsum --save_path dpx_xsum_16_4 --e 16 --d 4
```
we now have an initialized student saved to `dbart_xsum_12_3`, which we will use for the following commands.
+ Extension: To replicate more complicated initialize experiments in section 6.1, or try your own. Use the `create_student_by_copying_alternating_layers` function.
#### Pegasus
+ The following commands are written for BART and will require, at minimum, the following modifications
+ reduce batch size, and increase gradient accumulation steps so that the product `gpus * batch size * gradient_accumulation_steps = 256`. We used `--learning-rate` = 1e-4 * gradient accumulation steps.
+ don't use fp16
+ `--tokenizer_name google/pegasus-large`
### SFT (No Teacher Distillation)
You don't need `distillation.py`, you can just run:
```bash
python finetune.py \
--data_dir xsum \
--freeze_encoder --freeze_embeds \
--learning_rate=3e-4 \
--do_train \
--do_predict \
--fp16 --fp16_opt_level=O1 \
--val_check_interval 0.1 --n_val 1000 --eval_beams 2 --length_penalty=0.5 \
--max_target_length=60 --val_max_target_length=60 --test_max_target_length=100 \
--model_name_or_path dbart_xsum_12_3 \
--train_batch_size=64 --eval_batch_size=64 \
--sortish_sampler \
--num_train_epochs=6 \
--warmup_steps 500 \
--output_dir distilbart_xsum_sft_12_3 --gpus 1
```
+ Note: The command that produced `sshleifer/distilbart-cnn-12-6` is at [train_distilbart_cnn.sh](./[train_distilbart_cnn.sh)
```bash
./train_distilbart_cnn.sh
```
<!--- runtime: 6H on NVIDIA RTX 24GB GPU -->
+ Tip: You can get the same simple distillation logic by using `distillation.py --no_teacher ` followed by identical arguments as the ones in `train_distilbart_cnn.sh`.
If you are using `wandb` and comparing the two distillation methods, using this entry point will make your logs consistent,
because you will have the same hyper-parameters logged in every run.
### Pseudo-Labeling
+ You don't need `distillation.py`.
+ Instructions to generate pseudo-labels and use pre-computed pseudo-labels can be found [here](./precomputed_pseudo_labels.md).
Simply run `finetune.py` with one of those pseudo-label datasets as `--data_dir` (`DATA`, below).
```bash
python finetune.py \
--teacher facebook/bart-large-xsum --data_dir DATA \
--freeze_encoder --freeze_embeds \
--learning_rate=3e-4 \
--do_train \
--do_predict \
--fp16 --fp16_opt_level=O1 \
--val_check_interval 0.1 --n_val 1000 --eval_beams 2 --length_penalty=0.5 \
--max_target_length=60 --val_max_target_length=60 --test_max_target_length=100 \
--model_name_or_path dbart_xsum_12_3 \
--train_batch_size=32 --eval_batch_size=32 \
--sortish_sampler \
--num_train_epochs=5 \
--warmup_steps 500 \
--output_dir dbart_xsum_12_3_PL --gpus 1 --logger_name wandb
```
To combine datasets, as in Section 6.2, try something like:
```bash
curl -S https://cdn-datasets.huggingface.co/pseudo/xsum/bart_xsum_pl.tgz | tar -xvz -C .
curl -S https://cdn-datasets.huggingface.co/pseudo/xsum/pegasus_xsum.tgz | tar -xvz -C .
curl -S https://cdn-datasets.huggingface.co/summarization/xsum.tar.gz | tar -xvz -C .
mkdir all_pl
cat bart_xsum_pl/train.source pegasus_xsum/train.source xsum/train.source > all_pl/train.source
cat bart_xsum_pl/train.target pegasus_xsum/train.target xsum/train.target > all_pl/train.target
cp xsum/val* all_pl
cp xsum/test* all_pl
```
then use `all_pl` as DATA in the command above.
#### Direct Knowledge Distillation (KD)
+ In this method, we use try to enforce that the student and teacher produce similar encoder_outputs, logits, and hidden_states using `SummarizationDistiller`.
+ This method was used for `sshleifer/distilbart-xsum-12-6`, `6-6`, and `9-6` checkpoints were produced.
+ You must use [`distillation.py`](./distillation.py). Note that this command initializes the student for you.
The command that produced `sshleifer/distilbart-xsum-12-6` is at [./train_distilbart_xsum.sh](train_distilbart_xsum.sh)
```bash
./train_distilbart_xsum.sh --logger_name wandb --gpus 1
```
+ Expected ROUGE-2 between 21.3 and 21.6, run time ~13H.
+ direct KD + Pegasus is VERY slow and works best with `--supervise_forward --normalize_hidden`.
<!--- runtime: 13H on V-100 16GB GPU. -->
### Citation
```bibtex
@misc{shleifer2020pretrained,
title={Pre-trained Summarization Distillation},
author={Sam Shleifer and Alexander M. Rush},
year={2020},
eprint={2010.13002},
archivePrefix={arXiv},
primaryClass={cs.CL}
}
@article{Wolf2019HuggingFacesTS,
title={HuggingFace's Transformers: State-of-the-art Natural Language Processing},
author={Thomas Wolf and Lysandre Debut and Victor Sanh and Julien Chaumond and Clement Delangue and Anthony Moi and Pierric Cistac and Tim Rault and RΓ©mi Louf and Morgan Funtowicz and Joe Davison and Sam Shleifer and Patrick von Platen and Clara Ma and Yacine Jernite and Julien Plu and Canwen Xu and Teven Le Scao and Sylvain Gugger and Mariama Drame and Quentin Lhoest and Alexander M. Rush},
journal={ArXiv},
year={2019},
volume={abs/1910.03771}
}
```
|