Spaces:
Running
Running
File size: 62,650 Bytes
4bdb245 |
1 2 3 4 5 6 7 8 9 10 11 12 13 14 15 16 17 18 19 20 21 22 23 24 25 26 27 28 29 30 31 32 33 34 35 36 37 38 39 40 41 42 43 44 45 46 47 48 49 50 51 52 53 54 55 56 57 58 59 60 61 62 63 64 65 66 67 68 69 70 71 72 73 74 75 76 77 78 79 80 81 82 83 84 85 86 87 88 89 90 91 92 93 94 95 96 97 98 99 100 101 102 103 104 105 106 107 108 109 110 111 112 113 114 115 116 117 118 119 120 121 122 123 124 125 126 127 128 129 130 131 132 133 134 135 136 137 138 139 140 141 142 143 144 145 146 147 148 149 150 151 152 153 154 155 156 157 158 159 160 161 162 163 164 165 166 167 168 169 170 171 172 173 174 175 176 177 178 179 180 181 182 183 184 185 186 187 188 189 190 191 192 193 194 195 196 197 198 199 200 201 202 203 204 205 206 207 208 209 210 211 212 213 214 215 216 217 218 219 220 221 222 223 224 225 226 227 228 229 230 231 232 233 234 235 236 237 238 239 240 241 242 243 244 245 246 247 248 249 250 251 252 253 254 255 256 257 258 259 260 261 262 263 264 265 266 267 268 269 270 271 272 273 274 275 276 277 278 279 280 281 282 283 284 285 286 287 288 289 290 291 292 293 294 295 296 297 298 299 300 301 302 303 304 305 306 307 308 309 310 311 312 313 314 315 316 317 318 319 320 321 322 323 324 325 326 327 328 329 330 331 332 333 334 335 336 337 338 339 340 341 342 343 344 345 346 347 348 349 350 351 352 353 354 355 356 357 358 359 360 361 362 363 364 365 366 367 368 369 370 371 372 373 374 375 376 377 378 379 380 381 382 383 384 385 386 387 388 389 390 391 392 393 394 395 396 397 398 399 400 401 402 403 404 405 406 407 408 409 410 411 412 413 414 415 416 417 418 419 420 421 422 423 424 425 426 427 428 429 430 431 432 433 434 435 436 437 438 439 440 441 442 443 444 445 446 447 448 449 450 451 452 453 454 455 456 457 458 459 460 461 462 463 464 465 466 467 468 469 470 471 472 473 474 475 476 477 478 479 480 481 482 483 484 485 486 487 488 489 490 491 492 493 494 495 496 497 498 499 500 501 502 503 504 505 506 507 508 509 510 511 512 513 514 515 516 517 518 519 520 521 522 523 524 525 526 527 528 529 530 531 532 533 534 535 536 537 538 539 540 541 542 543 544 545 546 547 548 549 550 551 552 553 554 555 556 557 558 559 560 561 562 563 564 565 566 567 568 569 570 571 572 573 574 575 576 577 578 579 580 581 582 583 584 585 586 587 588 589 590 591 592 593 594 595 596 597 598 599 600 601 602 603 604 605 606 607 608 609 610 611 612 613 614 615 616 617 618 619 620 621 622 623 624 625 626 627 628 629 630 631 632 633 634 635 636 637 638 639 640 641 642 643 644 645 646 647 648 649 650 651 652 653 654 655 656 657 658 659 660 661 662 663 664 665 666 667 668 669 670 671 672 673 674 675 676 677 678 679 680 681 682 683 684 685 686 687 688 689 690 691 692 693 694 695 696 697 698 699 700 701 702 703 704 705 706 707 708 709 710 711 712 713 714 715 716 717 718 719 720 721 722 723 724 725 726 727 728 729 730 731 732 733 734 735 736 737 738 739 740 741 742 743 744 745 746 747 748 749 750 751 752 753 754 755 756 757 758 759 760 761 762 763 764 765 766 767 768 769 770 771 772 773 774 775 776 777 778 779 780 781 782 783 784 785 786 787 788 789 790 791 792 793 794 795 796 797 798 799 800 801 802 803 804 805 806 807 808 809 810 811 812 813 814 815 816 817 818 819 820 821 822 823 824 825 826 827 828 829 830 831 832 833 834 835 836 837 838 839 840 841 842 843 844 845 846 847 848 849 850 851 852 853 854 855 856 857 858 859 860 861 862 863 864 865 866 867 868 869 870 871 872 873 874 875 876 877 878 879 880 881 882 883 884 885 886 887 888 889 890 891 892 893 894 895 896 897 898 899 900 901 902 903 904 905 906 907 908 909 910 911 912 913 914 915 916 917 918 919 920 921 922 923 924 925 926 927 928 929 930 931 932 933 934 935 936 937 938 939 940 941 942 943 944 945 946 947 948 949 950 951 952 953 954 955 956 957 958 959 960 961 962 963 964 965 966 967 968 969 970 971 972 973 974 975 976 977 978 979 980 981 982 983 984 985 986 987 988 989 990 991 992 993 994 995 996 997 998 999 1000 1001 1002 1003 1004 1005 1006 1007 1008 1009 1010 1011 1012 1013 1014 1015 1016 1017 1018 1019 1020 1021 1022 1023 1024 1025 1026 1027 1028 1029 1030 1031 1032 1033 1034 1035 1036 1037 1038 1039 1040 1041 1042 1043 1044 1045 1046 1047 1048 1049 1050 1051 1052 1053 1054 1055 1056 1057 1058 1059 1060 1061 1062 1063 1064 1065 1066 1067 1068 1069 1070 1071 1072 1073 1074 1075 1076 1077 1078 1079 1080 1081 1082 1083 1084 1085 1086 1087 1088 1089 1090 1091 1092 1093 1094 1095 1096 1097 1098 1099 1100 1101 1102 1103 1104 1105 1106 1107 1108 1109 1110 1111 1112 1113 1114 1115 1116 1117 1118 1119 1120 1121 1122 1123 1124 1125 1126 1127 1128 1129 1130 1131 1132 1133 1134 1135 1136 1137 1138 1139 1140 1141 |
# llama.cpp
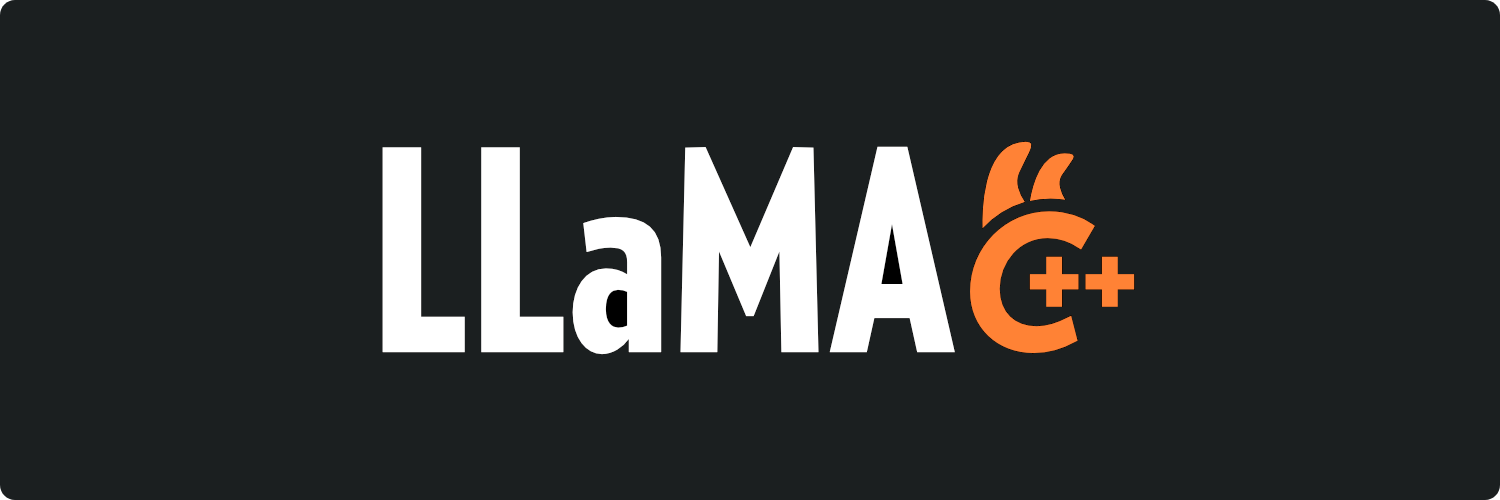
[](https://opensource.org/licenses/MIT)
[Roadmap](https://github.com/users/ggerganov/projects/7) / [Project status](https://github.com/ggerganov/llama.cpp/discussions/3471) / [Manifesto](https://github.com/ggerganov/llama.cpp/discussions/205) / [ggml](https://github.com/ggerganov/ggml)
Inference of Meta's [LLaMA](https://arxiv.org/abs/2302.13971) model (and others) in pure C/C++
### Recent API changes
- [2024 Apr 21] `llama_token_to_piece` can now optionally render special tokens https://github.com/ggerganov/llama.cpp/pull/6807
- [2024 Apr 4] State and session file functions reorganized under `llama_state_*` https://github.com/ggerganov/llama.cpp/pull/6341
- [2024 Mar 26] Logits and embeddings API updated for compactness https://github.com/ggerganov/llama.cpp/pull/6122
- [2024 Mar 13] Add `llama_synchronize()` + `llama_context_params.n_ubatch` https://github.com/ggerganov/llama.cpp/pull/6017
- [2024 Mar 8] `llama_kv_cache_seq_rm()` returns a `bool` instead of `void`, and new `llama_n_seq_max()` returns the upper limit of acceptable `seq_id` in batches (relevant when dealing with multiple sequences) https://github.com/ggerganov/llama.cpp/pull/5328
- [2024 Mar 4] Embeddings API updated https://github.com/ggerganov/llama.cpp/pull/5796
- [2024 Mar 3] `struct llama_context_params` https://github.com/ggerganov/llama.cpp/pull/5849
### Hot topics
- **BPE pre-tokenization support has been added: https://github.com/ggerganov/llama.cpp/pull/6920**
- MoE memory layout has been updated - reconvert models for `mmap` support and regenerate `imatrix` https://github.com/ggerganov/llama.cpp/pull/6387
- Model sharding instructions using `gguf-split` https://github.com/ggerganov/llama.cpp/discussions/6404
- Fix major bug in Metal batched inference https://github.com/ggerganov/llama.cpp/pull/6225
- Multi-GPU pipeline parallelism support https://github.com/ggerganov/llama.cpp/pull/6017
- Looking for contributions to add Deepseek support: https://github.com/ggerganov/llama.cpp/issues/5981
- Quantization blind testing: https://github.com/ggerganov/llama.cpp/discussions/5962
- Initial Mamba support has been added: https://github.com/ggerganov/llama.cpp/pull/5328
----
<details>
<summary>Table of Contents</summary>
<ol>
<li>
<a href="#description">Description</a>
</li>
<li>
<a href="#usage">Usage</a>
<ul>
<li><a href="#get-the-code">Get the Code</a></li>
<li><a href="#build">Build</a></li>
<li><a href="#blas-build">BLAS Build</a></li>
<li><a href="#prepare-and-quantize">Prepare and Quantize</a></li>
<li><a href="#run-the-quantized-model">Run the quantized model</a></li>
<li><a href="#memorydisk-requirements">Memory/Disk Requirements</a></li>
<li><a href="#quantization">Quantization</a></li>
<li><a href="#interactive-mode">Interactive mode</a></li>
<li><a href="#constrained-output-with-grammars">Constrained output with grammars</a></li>
<li><a href="#instruct-mode">Instruct mode</a></li>
<li><a href="#obtaining-and-using-the-facebook-llama-2-model">Obtaining and using the Facebook LLaMA 2 model</a></li>
<li><a href="#seminal-papers-and-background-on-the-models">Seminal papers and background on the models</a></li>
<li><a href="#perplexity-measuring-model-quality">Perplexity (measuring model quality)</a></li>
<li><a href="#android">Android</a></li>
<li><a href="#docker">Docker</a></li>
</ul>
</li>
<li><a href="#contributing">Contributing</a></li>
<li><a href="#coding-guidelines">Coding guidelines</a></li>
<li><a href="#docs">Docs</a></li>
</ol>
</details>
## Description
The main goal of `llama.cpp` is to enable LLM inference with minimal setup and state-of-the-art performance on a wide
variety of hardware - locally and in the cloud.
- Plain C/C++ implementation without any dependencies
- Apple silicon is a first-class citizen - optimized via ARM NEON, Accelerate and Metal frameworks
- AVX, AVX2 and AVX512 support for x86 architectures
- 1.5-bit, 2-bit, 3-bit, 4-bit, 5-bit, 6-bit, and 8-bit integer quantization for faster inference and reduced memory use
- Custom CUDA kernels for running LLMs on NVIDIA GPUs (support for AMD GPUs via HIP)
- Vulkan, SYCL, and (partial) OpenCL backend support
- CPU+GPU hybrid inference to partially accelerate models larger than the total VRAM capacity
Since its [inception](https://github.com/ggerganov/llama.cpp/issues/33#issuecomment-1465108022), the project has
improved significantly thanks to many contributions. It is the main playground for developing new features for the
[ggml](https://github.com/ggerganov/ggml) library.
**Supported platforms:**
- [X] Mac OS
- [X] Linux
- [X] Windows (via CMake)
- [X] Docker
- [X] FreeBSD
**Supported models:**
Typically finetunes of the base models below are supported as well.
- [X] LLaMA 🦙
- [x] LLaMA 2 🦙🦙
- [x] LLaMA 3 🦙🦙🦙
- [X] [Mistral 7B](https://huggingface.co/mistralai/Mistral-7B-v0.1)
- [x] [Mixtral MoE](https://huggingface.co/models?search=mistral-ai/Mixtral)
- [x] [DBRX](https://huggingface.co/databricks/dbrx-instruct)
- [X] [Falcon](https://huggingface.co/models?search=tiiuae/falcon)
- [X] [Chinese LLaMA / Alpaca](https://github.com/ymcui/Chinese-LLaMA-Alpaca) and [Chinese LLaMA-2 / Alpaca-2](https://github.com/ymcui/Chinese-LLaMA-Alpaca-2)
- [X] [Vigogne (French)](https://github.com/bofenghuang/vigogne)
- [X] [Koala](https://bair.berkeley.edu/blog/2023/04/03/koala/)
- [X] [Baichuan 1 & 2](https://huggingface.co/models?search=baichuan-inc/Baichuan) + [derivations](https://huggingface.co/hiyouga/baichuan-7b-sft)
- [X] [Aquila 1 & 2](https://huggingface.co/models?search=BAAI/Aquila)
- [X] [Starcoder models](https://github.com/ggerganov/llama.cpp/pull/3187)
- [X] [Refact](https://huggingface.co/smallcloudai/Refact-1_6B-fim)
- [X] [Persimmon 8B](https://github.com/ggerganov/llama.cpp/pull/3410)
- [X] [MPT](https://github.com/ggerganov/llama.cpp/pull/3417)
- [X] [Bloom](https://github.com/ggerganov/llama.cpp/pull/3553)
- [x] [Yi models](https://huggingface.co/models?search=01-ai/Yi)
- [X] [StableLM models](https://huggingface.co/stabilityai)
- [x] [Deepseek models](https://huggingface.co/models?search=deepseek-ai/deepseek)
- [x] [Qwen models](https://huggingface.co/models?search=Qwen/Qwen)
- [x] [PLaMo-13B](https://github.com/ggerganov/llama.cpp/pull/3557)
- [x] [Phi models](https://huggingface.co/models?search=microsoft/phi)
- [x] [GPT-2](https://huggingface.co/gpt2)
- [x] [Orion 14B](https://github.com/ggerganov/llama.cpp/pull/5118)
- [x] [InternLM2](https://huggingface.co/models?search=internlm2)
- [x] [CodeShell](https://github.com/WisdomShell/codeshell)
- [x] [Gemma](https://ai.google.dev/gemma)
- [x] [Mamba](https://github.com/state-spaces/mamba)
- [x] [Grok-1](https://huggingface.co/keyfan/grok-1-hf)
- [x] [Xverse](https://huggingface.co/models?search=xverse)
- [x] [Command-R models](https://huggingface.co/models?search=CohereForAI/c4ai-command-r)
- [x] [SEA-LION](https://huggingface.co/models?search=sea-lion)
- [x] [GritLM-7B](https://huggingface.co/GritLM/GritLM-7B) + [GritLM-8x7B](https://huggingface.co/GritLM/GritLM-8x7B)
- [x] [OLMo](https://allenai.org/olmo)
(instructions for supporting more models: [HOWTO-add-model.md](./docs/HOWTO-add-model.md))
**Multimodal models:**
- [x] [LLaVA 1.5 models](https://huggingface.co/collections/liuhaotian/llava-15-653aac15d994e992e2677a7e), [LLaVA 1.6 models](https://huggingface.co/collections/liuhaotian/llava-16-65b9e40155f60fd046a5ccf2)
- [x] [BakLLaVA](https://huggingface.co/models?search=SkunkworksAI/Bakllava)
- [x] [Obsidian](https://huggingface.co/NousResearch/Obsidian-3B-V0.5)
- [x] [ShareGPT4V](https://huggingface.co/models?search=Lin-Chen/ShareGPT4V)
- [x] [MobileVLM 1.7B/3B models](https://huggingface.co/models?search=mobileVLM)
- [x] [Yi-VL](https://huggingface.co/models?search=Yi-VL)
- [x] [Mini CPM](https://huggingface.co/models?search=MiniCPM)
- [x] [Moondream](https://huggingface.co/vikhyatk/moondream2)
**HTTP server**
[llama.cpp web server](./examples/server) is a lightweight [OpenAI API](https://github.com/openai/openai-openapi) compatible HTTP server that can be used to serve local models and easily connect them to existing clients.
**Bindings:**
- Python: [abetlen/llama-cpp-python](https://github.com/abetlen/llama-cpp-python)
- Go: [go-skynet/go-llama.cpp](https://github.com/go-skynet/go-llama.cpp)
- Node.js: [withcatai/node-llama-cpp](https://github.com/withcatai/node-llama-cpp)
- JS/TS (llama.cpp server client): [lgrammel/modelfusion](https://modelfusion.dev/integration/model-provider/llamacpp)
- JavaScript/Wasm (works in browser): [tangledgroup/llama-cpp-wasm](https://github.com/tangledgroup/llama-cpp-wasm)
- Typescript/Wasm (nicer API, available on npm): [ngxson/wllama](https://github.com/ngxson/wllama)
- Ruby: [yoshoku/llama_cpp.rb](https://github.com/yoshoku/llama_cpp.rb)
- Rust (more features): [edgenai/llama_cpp-rs](https://github.com/edgenai/llama_cpp-rs)
- Rust (nicer API): [mdrokz/rust-llama.cpp](https://github.com/mdrokz/rust-llama.cpp)
- Rust (more direct bindings): [utilityai/llama-cpp-rs](https://github.com/utilityai/llama-cpp-rs)
- C#/.NET: [SciSharp/LLamaSharp](https://github.com/SciSharp/LLamaSharp)
- Scala 3: [donderom/llm4s](https://github.com/donderom/llm4s)
- Clojure: [phronmophobic/llama.clj](https://github.com/phronmophobic/llama.clj)
- React Native: [mybigday/llama.rn](https://github.com/mybigday/llama.rn)
- Java: [kherud/java-llama.cpp](https://github.com/kherud/java-llama.cpp)
- Zig: [deins/llama.cpp.zig](https://github.com/Deins/llama.cpp.zig)
- Flutter/Dart: [netdur/llama_cpp_dart](https://github.com/netdur/llama_cpp_dart)
- PHP (API bindings and features built on top of llama.cpp): [distantmagic/resonance](https://github.com/distantmagic/resonance) [(more info)](https://github.com/ggerganov/llama.cpp/pull/6326)
**UI:**
Unless otherwise noted these projects are open-source with permissive licensing:
- [iohub/collama](https://github.com/iohub/coLLaMA)
- [janhq/jan](https://github.com/janhq/jan) (AGPL)
- [nat/openplayground](https://github.com/nat/openplayground)
- [Faraday](https://faraday.dev/) (proprietary)
- [LMStudio](https://lmstudio.ai/) (proprietary)
- [LocalAI](https://github.com/mudler/LocalAI) (MIT)
- [LostRuins/koboldcpp](https://github.com/LostRuins/koboldcpp) (AGPL)
- [Mozilla-Ocho/llamafile](https://github.com/Mozilla-Ocho/llamafile)
- [nomic-ai/gpt4all](https://github.com/nomic-ai/gpt4all)
- [ollama/ollama](https://github.com/ollama/ollama)
- [oobabooga/text-generation-webui](https://github.com/oobabooga/text-generation-webui) (AGPL)
- [psugihara/FreeChat](https://github.com/psugihara/FreeChat)
- [cztomsik/ava](https://github.com/cztomsik/ava) (MIT)
- [ptsochantaris/emeltal](https://github.com/ptsochantaris/emeltal)
- [pythops/tenere](https://github.com/pythops/tenere) (AGPL)
- [RecurseChat](https://recurse.chat/) (proprietary)
- [semperai/amica](https://github.com/semperai/amica)
- [withcatai/catai](https://github.com/withcatai/catai)
- [Mobile-Artificial-Intelligence/maid](https://github.com/Mobile-Artificial-Intelligence/maid) (MIT)
- [Msty](https://msty.app) (proprietary)
- [LLMFarm](https://github.com/guinmoon/LLMFarm?tab=readme-ov-file) (MIT)
- [KanTV](https://github.com/zhouwg/kantv?tab=readme-ov-file)(Apachev2.0 or later)
- [Dot](https://github.com/alexpinel/Dot) (GPL)
- [MindMac](https://mindmac.app) (proprietary)
- [KodiBot](https://github.com/firatkiral/kodibot) (GPL)
- [eva](https://github.com/ylsdamxssjxxdd/eva) (MIT)
- [AI Sublime Text plugin](https://github.com/yaroslavyaroslav/OpenAI-sublime-text) (MIT)
*(to have a project listed here, it should clearly state that it depends on `llama.cpp`)*
---
Here is a typical run using LLaMA v2 13B on M2 Ultra:
```
$ make -j && ./main -m models/llama-13b-v2/ggml-model-q4_0.gguf -p "Building a website can be done in 10 simple steps:\nStep 1:" -n 400 -e
I llama.cpp build info:
I UNAME_S: Darwin
I UNAME_P: arm
I UNAME_M: arm64
I CFLAGS: -I. -O3 -std=c11 -fPIC -DNDEBUG -Wall -Wextra -Wpedantic -Wcast-qual -Wdouble-promotion -Wshadow -Wstrict-prototypes -Wpointer-arith -Wmissing-prototypes -pthread -DGGML_USE_K_QUANTS -DGGML_USE_ACCELERATE
I CXXFLAGS: -I. -I./common -O3 -std=c++11 -fPIC -DNDEBUG -Wall -Wextra -Wpedantic -Wcast-qual -Wno-unused-function -Wno-multichar -pthread -DGGML_USE_K_QUANTS
I LDFLAGS: -framework Accelerate
I CC: Apple clang version 14.0.3 (clang-1403.0.22.14.1)
I CXX: Apple clang version 14.0.3 (clang-1403.0.22.14.1)
make: Nothing to be done for `default'.
main: build = 1041 (cf658ad)
main: seed = 1692823051
llama_model_loader: loaded meta data with 16 key-value pairs and 363 tensors from models/llama-13b-v2/ggml-model-q4_0.gguf (version GGUF V1 (latest))
llama_model_loader: - type f32: 81 tensors
llama_model_loader: - type q4_0: 281 tensors
llama_model_loader: - type q6_K: 1 tensors
llm_load_print_meta: format = GGUF V1 (latest)
llm_load_print_meta: arch = llama
llm_load_print_meta: vocab type = SPM
llm_load_print_meta: n_vocab = 32000
llm_load_print_meta: n_merges = 0
llm_load_print_meta: n_ctx_train = 4096
llm_load_print_meta: n_ctx = 512
llm_load_print_meta: n_embd = 5120
llm_load_print_meta: n_head = 40
llm_load_print_meta: n_head_kv = 40
llm_load_print_meta: n_layer = 40
llm_load_print_meta: n_rot = 128
llm_load_print_meta: n_gqa = 1
llm_load_print_meta: f_norm_eps = 1.0e-05
llm_load_print_meta: f_norm_rms_eps = 1.0e-05
llm_load_print_meta: n_ff = 13824
llm_load_print_meta: freq_base = 10000.0
llm_load_print_meta: freq_scale = 1
llm_load_print_meta: model type = 13B
llm_load_print_meta: model ftype = mostly Q4_0
llm_load_print_meta: model size = 13.02 B
llm_load_print_meta: general.name = LLaMA v2
llm_load_print_meta: BOS token = 1 '<s>'
llm_load_print_meta: EOS token = 2 '</s>'
llm_load_print_meta: UNK token = 0 '<unk>'
llm_load_print_meta: LF token = 13 '<0x0A>'
llm_load_tensors: ggml ctx size = 0.11 MB
llm_load_tensors: mem required = 7024.01 MB (+ 400.00 MB per state)
...................................................................................................
llama_new_context_with_model: kv self size = 400.00 MB
llama_new_context_with_model: compute buffer total size = 75.41 MB
system_info: n_threads = 16 / 24 | AVX = 0 | AVX2 = 0 | AVX512 = 0 | AVX512_VBMI = 0 | AVX512_VNNI = 0 | FMA = 0 | NEON = 1 | ARM_FMA = 1 | F16C = 0 | FP16_VA = 1 | WASM_SIMD = 0 | BLAS = 1 | SSE3 = 0 | VSX = 0 |
sampling: repeat_last_n = 64, repeat_penalty = 1.100000, presence_penalty = 0.000000, frequency_penalty = 0.000000, top_k = 40, tfs_z = 1.000000, top_p = 0.950000, typical_p = 1.000000, temp = 0.800000, mirostat = 0, mirostat_lr = 0.100000, mirostat_ent = 5.000000
generate: n_ctx = 512, n_batch = 512, n_predict = 400, n_keep = 0
Building a website can be done in 10 simple steps:
Step 1: Find the right website platform.
Step 2: Choose your domain name and hosting plan.
Step 3: Design your website layout.
Step 4: Write your website content and add images.
Step 5: Install security features to protect your site from hackers or spammers
Step 6: Test your website on multiple browsers, mobile devices, operating systems etc…
Step 7: Test it again with people who are not related to you personally – friends or family members will work just fine!
Step 8: Start marketing and promoting the website via social media channels or paid ads
Step 9: Analyze how many visitors have come to your site so far, what type of people visit more often than others (e.g., men vs women) etc…
Step 10: Continue to improve upon all aspects mentioned above by following trends in web design and staying up-to-date on new technologies that can enhance user experience even further!
How does a Website Work?
A website works by having pages, which are made of HTML code. This code tells your computer how to display the content on each page you visit – whether it’s an image or text file (like PDFs). In order for someone else’s browser not only be able but also want those same results when accessing any given URL; some additional steps need taken by way of programming scripts that will add functionality such as making links clickable!
The most common type is called static HTML pages because they remain unchanged over time unless modified manually (either through editing files directly or using an interface such as WordPress). They are usually served up via HTTP protocols – this means anyone can access them without having any special privileges like being part of a group who is allowed into restricted areas online; however, there may still exist some limitations depending upon where one lives geographically speaking.
How to
llama_print_timings: load time = 576.45 ms
llama_print_timings: sample time = 283.10 ms / 400 runs ( 0.71 ms per token, 1412.91 tokens per second)
llama_print_timings: prompt eval time = 599.83 ms / 19 tokens ( 31.57 ms per token, 31.68 tokens per second)
llama_print_timings: eval time = 24513.59 ms / 399 runs ( 61.44 ms per token, 16.28 tokens per second)
llama_print_timings: total time = 25431.49 ms
```
And here is another demo of running both LLaMA-7B and [whisper.cpp](https://github.com/ggerganov/whisper.cpp) on a single M1 Pro MacBook:
https://user-images.githubusercontent.com/1991296/224442907-7693d4be-acaa-4e01-8b4f-add84093ffff.mp4
## Usage
Here are the end-to-end binary build and model conversion steps for most supported models.
### Get the Code
```bash
git clone https://github.com/ggerganov/llama.cpp
cd llama.cpp
```
### Build
In order to build llama.cpp you have three different options.
- Using `make`:
- On Linux or MacOS:
```bash
make
```
**Note**: for `Debug` builds, run `make LLAMA_DEBUG=1`
- On Windows:
1. Download the latest fortran version of [w64devkit](https://github.com/skeeto/w64devkit/releases).
2. Extract `w64devkit` on your pc.
3. Run `w64devkit.exe`.
4. Use the `cd` command to reach the `llama.cpp` folder.
5. From here you can run:
```bash
make
```
- Using `CMake`:
```bash
cmake -B build
cmake --build build --config Release
```
**Note**: for `Debug` builds, there are two cases:
- Single-config generators (e.g. default = `Unix Makefiles`; note that they just ignore the `--config` flag):
```bash
cmake -B build -DCMAKE_BUILD_TYPE=Debug
cmake --build build
```
- Multi-config generators (`-G` param set to Visual Studio, XCode...):
```bash
cmake -B build -G "Xcode"
cmake --build build --config Debug
```
- Using `Zig` (version 0.11 or later):
Building for optimization levels and CPU features can be accomplished using standard build arguments, for example AVX2, FMA, F16C,
it's also possible to cross compile for other operating systems and architectures:
```bash
zig build -Doptimize=ReleaseFast -Dtarget=x86_64-windows-gnu -Dcpu=x86_64+avx2+fma+f16c
```
The `zig targets` command will give you valid options to use.
- Using `gmake` (FreeBSD):
1. Install and activate [DRM in FreeBSD](https://wiki.freebsd.org/Graphics)
2. Add your user to **video** group
3. Install compilation dependencies.
```bash
sudo pkg install gmake automake autoconf pkgconf llvm15 clinfo clover \
opencl clblast openblas
gmake CC=/usr/local/bin/clang15 CXX=/usr/local/bin/clang++15 -j4
```
**Notes:** With this packages you can build llama.cpp with OPENBLAS and
CLBLAST support for use OpenCL GPU acceleration in FreeBSD. Please read
the instructions for use and activate this options in this document below.
### Metal Build
On MacOS, Metal is enabled by default. Using Metal makes the computation run on the GPU.
To disable the Metal build at compile time use the `LLAMA_NO_METAL=1` flag or the `LLAMA_METAL=OFF` cmake option.
When built with Metal support, you can explicitly disable GPU inference with the `--n-gpu-layers|-ngl 0` command-line
argument.
### MPI Build
MPI lets you distribute the computation over a cluster of machines. Because of the serial nature of LLM prediction, this won't yield any end-to-end speed-ups, but it will let you run larger models than would otherwise fit into RAM on a single machine.
First you will need MPI libraries installed on your system. The two most popular (only?) options are [MPICH](https://www.mpich.org) and [OpenMPI](https://www.open-mpi.org). Either can be installed with a package manager (`apt`, Homebrew, MacPorts, etc).
Next you will need to build the project with `LLAMA_MPI` set to true on all machines; if you're building with `make`, you will also need to specify an MPI-capable compiler (when building with CMake, this is configured automatically):
- Using `make`:
```bash
make CC=mpicc CXX=mpicxx LLAMA_MPI=1
```
- Using `CMake`:
```bash
cmake -S . -B build -DLLAMA_MPI=ON
```
Once the programs are built, download/convert the weights on all of the machines in your cluster. The paths to the weights and programs should be identical on all machines.
Next, ensure password-less SSH access to each machine from the primary host, and create a `hostfile` with a list of the hostnames and their relative "weights" (slots). If you want to use localhost for computation, use its local subnet IP address rather than the loopback address or "localhost".
Here is an example hostfile:
```
192.168.0.1:2
malvolio.local:1
```
The above will distribute the computation across 2 processes on the first host and 1 process on the second host. Each process will use roughly an equal amount of RAM. Try to keep these numbers small, as inter-process (intra-host) communication is expensive.
Finally, you're ready to run a computation using `mpirun`:
```bash
mpirun -hostfile hostfile -n 3 ./main -m ./models/7B/ggml-model-q4_0.gguf -n 128
```
### BLAS Build
Building the program with BLAS support may lead to some performance improvements in prompt processing using batch sizes higher than 32 (the default is 512). Support with CPU-only BLAS implementations doesn't affect the normal generation performance. We may see generation performance improvements with GPU-involved BLAS implementations, e.g. cuBLAS, hipBLAS and CLBlast. There are currently several different BLAS implementations available for build and use:
- #### Accelerate Framework:
This is only available on Mac PCs and it's enabled by default. You can just build using the normal instructions.
- #### OpenBLAS:
This provides BLAS acceleration using only the CPU. Make sure to have OpenBLAS installed on your machine.
- Using `make`:
- On Linux:
```bash
make LLAMA_OPENBLAS=1
```
- On Windows:
1. Download the latest fortran version of [w64devkit](https://github.com/skeeto/w64devkit/releases).
2. Download the latest version of [OpenBLAS for Windows](https://github.com/xianyi/OpenBLAS/releases).
3. Extract `w64devkit` on your pc.
4. From the OpenBLAS zip that you just downloaded copy `libopenblas.a`, located inside the `lib` folder, inside `w64devkit\x86_64-w64-mingw32\lib`.
5. From the same OpenBLAS zip copy the content of the `include` folder inside `w64devkit\x86_64-w64-mingw32\include`.
6. Run `w64devkit.exe`.
7. Use the `cd` command to reach the `llama.cpp` folder.
8. From here you can run:
```bash
make LLAMA_OPENBLAS=1
```
- Using `CMake` on Linux:
```bash
cmake -B build -DLLAMA_BLAS=ON -DLLAMA_BLAS_VENDOR=OpenBLAS
cmake --build build --config Release
```
- #### BLIS
Check [BLIS.md](docs/BLIS.md) for more information.
- #### SYCL
SYCL is a higher-level programming model to improve programming productivity on various hardware accelerators.
llama.cpp based on SYCL is used to **support Intel GPU** (Data Center Max series, Flex series, Arc series, Built-in GPU and iGPU).
For detailed info, please refer to [llama.cpp for SYCL](README-sycl.md).
- #### Intel oneMKL
Building through oneAPI compilers will make avx_vnni instruction set available for intel processors that do not support avx512 and avx512_vnni. Please note that this build config **does not support Intel GPU**. For Intel GPU support, please refer to [llama.cpp for SYCL](./README-sycl.md).
- Using manual oneAPI installation:
By default, `LLAMA_BLAS_VENDOR` is set to `Generic`, so if you already sourced intel environment script and assign `-DLLAMA_BLAS=ON` in cmake, the mkl version of Blas will automatically been selected. Otherwise please install oneAPI and follow the below steps:
```bash
source /opt/intel/oneapi/setvars.sh # You can skip this step if in oneapi-basekit docker image, only required for manual installation
cmake -B build -DLLAMA_BLAS=ON -DLLAMA_BLAS_VENDOR=Intel10_64lp -DCMAKE_C_COMPILER=icx -DCMAKE_CXX_COMPILER=icpx -DLLAMA_NATIVE=ON
cmake --build build --config Release
```
- Using oneAPI docker image:
If you do not want to source the environment vars and install oneAPI manually, you can also build the code using intel docker container: [oneAPI-basekit](https://hub.docker.com/r/intel/oneapi-basekit). Then, you can use the commands given above.
Check [Optimizing and Running LLaMA2 on Intel® CPU](https://www.intel.com/content/www/us/en/content-details/791610/optimizing-and-running-llama2-on-intel-cpu.html) for more information.
- #### CUDA
This provides GPU acceleration using the CUDA cores of your Nvidia GPU. Make sure to have the CUDA toolkit installed. You can download it from your Linux distro's package manager (e.g. `apt install nvidia-cuda-toolkit`) or from here: [CUDA Toolkit](https://developer.nvidia.com/cuda-downloads).
For Jetson user, if you have Jetson Orin, you can try this: [Offical Support](https://www.jetson-ai-lab.com/tutorial_text-generation.html). If you are using an old model(nano/TX2), need some additional operations before compiling.
- Using `make`:
```bash
make LLAMA_CUDA=1
```
- Using `CMake`:
```bash
cmake -B build -DLLAMA_CUDA=ON
cmake --build build --config Release
```
The environment variable [`CUDA_VISIBLE_DEVICES`](https://docs.nvidia.com/cuda/cuda-c-programming-guide/index.html#env-vars) can be used to specify which GPU(s) will be used. The following compilation options are also available to tweak performance:
| Option | Legal values | Default | Description |
|--------------------------------|------------------------|---------|-----------------------------------------------------------------------------------------------------------------------------------------------------------------------------------------------------------------------------------------------------------------------------------------|
| LLAMA_CUDA_FORCE_DMMV | Boolean | false | Force the use of dequantization + matrix vector multiplication kernels instead of using kernels that do matrix vector multiplication on quantized data. By default the decision is made based on compute capability (MMVQ for 6.1/Pascal/GTX 1000 or higher). Does not affect k-quants. |
| LLAMA_CUDA_DMMV_X | Positive integer >= 32 | 32 | Number of values in x direction processed by the CUDA dequantization + matrix vector multiplication kernel per iteration. Increasing this value can improve performance on fast GPUs. Power of 2 heavily recommended. Does not affect k-quants. |
| LLAMA_CUDA_MMV_Y | Positive integer | 1 | Block size in y direction for the CUDA mul mat vec kernels. Increasing this value can improve performance on fast GPUs. Power of 2 recommended. |
| LLAMA_CUDA_F16 | Boolean | false | If enabled, use half-precision floating point arithmetic for the CUDA dequantization + mul mat vec kernels and for the q4_1 and q5_1 matrix matrix multiplication kernels. Can improve performance on relatively recent GPUs. |
| LLAMA_CUDA_KQUANTS_ITER | 1 or 2 | 2 | Number of values processed per iteration and per CUDA thread for Q2_K and Q6_K quantization formats. Setting this value to 1 can improve performance for slow GPUs. |
| LLAMA_CUDA_PEER_MAX_BATCH_SIZE | Positive integer | 128 | Maximum batch size for which to enable peer access between multiple GPUs. Peer access requires either Linux or NVLink. When using NVLink enabling peer access for larger batch sizes is potentially beneficial. |
- #### hipBLAS
This provides BLAS acceleration on HIP-supported AMD GPUs.
Make sure to have ROCm installed.
You can download it from your Linux distro's package manager or from here: [ROCm Quick Start (Linux)](https://rocm.docs.amd.com/projects/install-on-linux/en/latest/tutorial/quick-start.html#rocm-install-quick).
- Using `make`:
```bash
make LLAMA_HIPBLAS=1
```
- Using `CMake` for Linux (assuming a gfx1030-compatible AMD GPU):
```bash
CC=/opt/rocm/llvm/bin/clang CXX=/opt/rocm/llvm/bin/clang++ \
cmake -B build -DLLAMA_HIPBLAS=ON -DAMDGPU_TARGETS=gfx1030 -DCMAKE_BUILD_TYPE=Release \
&& cmake --build build --config Release -- -j 16
```
On Linux it is also possible to use unified memory architecture (UMA) to share main memory between the CPU and integrated GPU by setting `-DLLAMA_HIP_UMA=ON"`.
However, this hurts performance for non-integrated GPUs (but enables working with integrated GPUs).
- Using `make` (example for target gfx1030, build with 16 CPU threads):
```bash
make -j16 LLAMA_HIPBLAS=1 LLAMA_HIP_UMA=1 AMDGPU_TARGETS=gfx1030
```
- Using `CMake` for Windows (using x64 Native Tools Command Prompt for VS, and assuming a gfx1100-compatible AMD GPU):
```bash
set PATH=%HIP_PATH%\bin;%PATH%
mkdir build
cd build
cmake -G Ninja -DAMDGPU_TARGETS=gfx1100 -DLLAMA_HIPBLAS=ON -DCMAKE_C_COMPILER=clang -DCMAKE_CXX_COMPILER=clang++ -DCMAKE_BUILD_TYPE=Release ..
cmake --build .
```
Make sure that `AMDGPU_TARGETS` is set to the GPU arch you want to compile for. The above example uses `gfx1100` that corresponds to Radeon RX 7900XTX/XT/GRE. You can find a list of targets [here](https://llvm.org/docs/AMDGPUUsage.html#processors)
Find your gpu version string by matching the most significant version information from `rocminfo | grep gfx | head -1 | awk '{print $2}'` with the list of processors, e.g. `gfx1035` maps to `gfx1030`.
The environment variable [`HIP_VISIBLE_DEVICES`](https://rocm.docs.amd.com/en/latest/understand/gpu_isolation.html#hip-visible-devices) can be used to specify which GPU(s) will be used.
If your GPU is not officially supported you can use the environment variable [`HSA_OVERRIDE_GFX_VERSION`] set to a similar GPU, for example 10.3.0 on RDNA2 (e.g. gfx1030, gfx1031, or gfx1035) or 11.0.0 on RDNA3.
The following compilation options are also available to tweak performance (yes, they refer to CUDA, not HIP, because it uses the same code as the cuBLAS version above):
| Option | Legal values | Default | Description |
|-------------------------|------------------------|---------|------------------------------------------------------------------------------------------------------------------------------------------------------------------------------------------------------------------------------------------------|
| LLAMA_CUDA_DMMV_X | Positive integer >= 32 | 32 | Number of values in x direction processed by the HIP dequantization + matrix vector multiplication kernel per iteration. Increasing this value can improve performance on fast GPUs. Power of 2 heavily recommended. Does not affect k-quants. |
| LLAMA_CUDA_MMV_Y | Positive integer | 1 | Block size in y direction for the HIP mul mat vec kernels. Increasing this value can improve performance on fast GPUs. Power of 2 recommended. Does not affect k-quants. |
| LLAMA_CUDA_KQUANTS_ITER | 1 or 2 | 2 | Number of values processed per iteration and per HIP thread for Q2_K and Q6_K quantization formats. Setting this value to 1 can improve performance for slow GPUs. |
- #### CLBlast
OpenCL acceleration is provided by the matrix multiplication kernels from the [CLBlast](https://github.com/CNugteren/CLBlast) project and custom kernels for ggml that can generate tokens on the GPU.
You will need the [OpenCL SDK](https://github.com/KhronosGroup/OpenCL-SDK).
- For Ubuntu, Debian, and Fedora the packages `opencl-headers`, `ocl-icd` may be needed.
- For Windows, a pre-built SDK is available on the [OpenCL Releases](https://github.com/KhronosGroup/OpenCL-SDK/releases) page.
- <details>
<summary>Installing the OpenCL SDK from source</summary>
```sh
git clone --recurse-submodules https://github.com/KhronosGroup/OpenCL-SDK.git
cd OpenCL-SDK
cmake -B build -DBUILD_DOCS=OFF \
-DBUILD_EXAMPLES=OFF \
-DBUILD_TESTING=OFF \
-DOPENCL_SDK_BUILD_SAMPLES=OFF \
-DOPENCL_SDK_TEST_SAMPLES=OFF
cmake --build build
cmake --install build --prefix /some/path
```
</details>
##### Installing CLBlast
Pre-built CLBlast binaries may be found on the [CLBlast Releases](https://github.com/CNugteren/CLBlast/releases) page. For Unix variants, it may also be found in your operating system's packages.
Linux packaging:
Fedora Linux:
```bash
sudo dnf install clblast
```
Alternatively, they may be built from source.
- <details>
<summary>Windows:</summary>
```cmd
set OPENCL_SDK_ROOT="C:/OpenCL-SDK-v2023.04.17-Win-x64"
git clone https://github.com/CNugteren/CLBlast.git
cd CLBlast
cmake -B build -DBUILD_SHARED_LIBS=OFF -DOVERRIDE_MSVC_FLAGS_TO_MT=OFF -DTUNERS=OFF -DOPENCL_ROOT=%OPENCL_SDK_ROOT% -G "Visual Studio 17 2022" -A x64
cmake --build build --config Release
cmake --install build --prefix C:/CLBlast
```
(note: `--config Release` at build time is the default and only relevant for Visual Studio builds - or multi-config Ninja builds)
- <details>
<summary>Unix:</summary>
```sh
git clone https://github.com/CNugteren/CLBlast.git
cd CLBlast
cmake -B build -DBUILD_SHARED_LIBS=OFF -DTUNERS=OFF
cmake --build build --config Release
cmake --install build --prefix /some/path
```
Where `/some/path` is where the built library will be installed (default is `/usr/local`).
</details>
##### Building Llama with CLBlast
- Build with make:
```sh
make LLAMA_CLBLAST=1
```
- CMake (Unix):
```sh
cmake -B build -DLLAMA_CLBLAST=ON -DCLBlast_DIR=/some/path
cmake --build build --config Release
```
- CMake (Windows):
```cmd
set CL_BLAST_CMAKE_PKG="C:/CLBlast/lib/cmake/CLBlast"
git clone https://github.com/ggerganov/llama.cpp
cd llama.cpp
cmake -B build -DBUILD_SHARED_LIBS=OFF -DLLAMA_CLBLAST=ON -DCMAKE_PREFIX_PATH=%CL_BLAST_CMAKE_PKG% -G "Visual Studio 17 2022" -A x64
cmake --build build --config Release
cmake --install build --prefix C:/LlamaCPP
```
##### Running Llama with CLBlast
The CLBlast build supports `--gpu-layers|-ngl` like the CUDA version does.
To select the correct platform (driver) and device (GPU), you can use the environment variables `GGML_OPENCL_PLATFORM` and `GGML_OPENCL_DEVICE`.
The selection can be a number (starting from 0) or a text string to search:
```sh
GGML_OPENCL_PLATFORM=1 ./main ...
GGML_OPENCL_DEVICE=2 ./main ...
GGML_OPENCL_PLATFORM=Intel ./main ...
GGML_OPENCL_PLATFORM=AMD GGML_OPENCL_DEVICE=1 ./main ...
```
The default behavior is to find the first GPU device, but when it is an integrated GPU on a laptop, for instance, the selectors are useful.
Using the variables it is possible to select a CPU-based driver as well, if so desired.
You can get a list of platforms and devices from the `clinfo -l` command, etc.
- #### Vulkan
**With docker**:
You don't need to install Vulkan SDK. It will be installed inside the container.
```sh
# Build the image
docker build -t llama-cpp-vulkan -f .devops/main-vulkan.Dockerfile .
# Then, use it:
docker run -it --rm -v "$(pwd):/app:Z" --device /dev/dri/renderD128:/dev/dri/renderD128 --device /dev/dri/card1:/dev/dri/card1 llama-cpp-vulkan -m "/app/models/YOUR_MODEL_FILE" -p "Building a website can be done in 10 simple steps:" -n 400 -e -ngl 33
```
**Without docker**:
Firstly, you need to make sure you have installed [Vulkan SDK](https://vulkan.lunarg.com/doc/view/latest/linux/getting_started_ubuntu.html)
For example, on Ubuntu 22.04 (jammy), use the command below:
```bash
wget -qO - https://packages.lunarg.com/lunarg-signing-key-pub.asc | apt-key add -
wget -qO /etc/apt/sources.list.d/lunarg-vulkan-jammy.list https://packages.lunarg.com/vulkan/lunarg-vulkan-jammy.list
apt update -y
apt-get install -y vulkan-sdk
# To verify the installation, use the command below:
vulkaninfo
```
Alternatively your package manager might be able to provide the appropiate libraries. For example for Ubuntu 22.04 you can install `libvulkan-dev` instead.
Then, build llama.cpp using the cmake command below:
```bash
cmake -B build -DLLAMA_VULKAN=1
cmake --build build --config Release
# Test the output binary (with "-ngl 33" to offload all layers to GPU)
./bin/main -m "PATH_TO_MODEL" -p "Hi you how are you" -n 50 -e -ngl 33 -t 4
# You should see in the output, ggml_vulkan detected your GPU. For example:
# ggml_vulkan: Using Intel(R) Graphics (ADL GT2) | uma: 1 | fp16: 1 | warp size: 32
```
### Prepare and Quantize
To obtain the official LLaMA 2 weights please see the <a href="#obtaining-and-using-the-facebook-llama-2-model">Obtaining and using the Facebook LLaMA 2 model</a> section. There is also a large selection of pre-quantized `gguf` models available on Hugging Face.
```bash
# obtain the official LLaMA model weights and place them in ./models
ls ./models
llama-2-7b tokenizer_checklist.chk tokenizer.model
# [Optional] for models using BPE tokenizers
ls ./models
<folder containing weights and tokenizer json> vocab.json
# [Optional] for PyTorch .bin models like Mistral-7B
ls ./models
<folder containing weights and tokenizer json>
# install Python dependencies
python3 -m pip install -r requirements.txt
# convert the model to ggml FP16 format
python3 convert.py models/mymodel/
# [Optional] for models using BPE tokenizers
python convert.py models/mymodel/ --vocab-type bpe
# quantize the model to 4-bits (using Q4_K_M method)
./quantize ./models/mymodel/ggml-model-f16.gguf ./models/mymodel/ggml-model-Q4_K_M.gguf Q4_K_M
# update the gguf filetype to current version if older version is now unsupported
./quantize ./models/mymodel/ggml-model-Q4_K_M.gguf ./models/mymodel/ggml-model-Q4_K_M-v2.gguf COPY
```
### Run the quantized model
```bash
# start inference on a gguf model
./main -m ./models/mymodel/ggml-model-Q4_K_M.gguf -n 128
```
When running the larger models, make sure you have enough disk space to store all the intermediate files.
### Running on Windows with prebuilt binaries
You will find prebuilt Windows binaries on the release page.
Simply download and extract the latest zip package of choice: (e.g. `llama-b1380-bin-win-avx2-x64.zip`)
From the unzipped folder, open a terminal/cmd window here and place a pre-converted `.gguf` model file. Test out the main example like so:
```
.\main -m llama-2-7b.Q4_0.gguf -n 128
```
### Memory/Disk Requirements
As the models are currently fully loaded into memory, you will need adequate disk space to save them and sufficient RAM to load them. At the moment, memory and disk requirements are the same.
| Model | Original size | Quantized size (Q4_0) |
|------:|--------------:|----------------------:|
| 7B | 13 GB | 3.9 GB |
| 13B | 24 GB | 7.8 GB |
| 30B | 60 GB | 19.5 GB |
| 65B | 120 GB | 38.5 GB |
### Quantization
Several quantization methods are supported. They differ in the resulting model disk size and inference speed.
*(outdated)*
| Model | Measure | F16 | Q4_0 | Q4_1 | Q5_0 | Q5_1 | Q8_0 |
|------:|--------------|-------:|-------:|-------:|-------:|-------:|-------:|
| 7B | perplexity | 5.9066 | 6.1565 | 6.0912 | 5.9862 | 5.9481 | 5.9070 |
| 7B | file size | 13.0G | 3.5G | 3.9G | 4.3G | 4.7G | 6.7G |
| 7B | ms/tok @ 4th | 127 | 55 | 54 | 76 | 83 | 72 |
| 7B | ms/tok @ 8th | 122 | 43 | 45 | 52 | 56 | 67 |
| 7B | bits/weight | 16.0 | 4.5 | 5.0 | 5.5 | 6.0 | 8.5 |
| 13B | perplexity | 5.2543 | 5.3860 | 5.3608 | 5.2856 | 5.2706 | 5.2548 |
| 13B | file size | 25.0G | 6.8G | 7.6G | 8.3G | 9.1G | 13G |
| 13B | ms/tok @ 4th | - | 103 | 105 | 148 | 160 | 131 |
| 13B | ms/tok @ 8th | - | 73 | 82 | 98 | 105 | 128 |
| 13B | bits/weight | 16.0 | 4.5 | 5.0 | 5.5 | 6.0 | 8.5 |
- [k-quants](https://github.com/ggerganov/llama.cpp/pull/1684)
- recent k-quants improvements and new i-quants
- [#2707](https://github.com/ggerganov/llama.cpp/pull/2707)
- [#2807](https://github.com/ggerganov/llama.cpp/pull/2807)
- [#4773 - 2-bit i-quants (inference)](https://github.com/ggerganov/llama.cpp/pull/4773)
- [#4856 - 2-bit i-quants (inference)](https://github.com/ggerganov/llama.cpp/pull/4856)
- [#4861 - importance matrix](https://github.com/ggerganov/llama.cpp/pull/4861)
- [#4872 - MoE models](https://github.com/ggerganov/llama.cpp/pull/4872)
- [#4897 - 2-bit quantization](https://github.com/ggerganov/llama.cpp/pull/4897)
- [#4930 - imatrix for all k-quants](https://github.com/ggerganov/llama.cpp/pull/4930)
- [#4951 - imatrix on the GPU](https://github.com/ggerganov/llama.cpp/pull/4957)
- [#4969 - imatrix for legacy quants](https://github.com/ggerganov/llama.cpp/pull/4969)
- [#4996 - k-qunats tuning](https://github.com/ggerganov/llama.cpp/pull/4996)
- [#5060 - Q3_K_XS](https://github.com/ggerganov/llama.cpp/pull/5060)
- [#5196 - 3-bit i-quants](https://github.com/ggerganov/llama.cpp/pull/5196)
- [quantization tuning](https://github.com/ggerganov/llama.cpp/pull/5320), [another one](https://github.com/ggerganov/llama.cpp/pull/5334), and [another one](https://github.com/ggerganov/llama.cpp/pull/5361)
### Perplexity (measuring model quality)
You can use the `perplexity` example to measure perplexity over a given prompt (lower perplexity is better).
For more information, see [https://huggingface.co/docs/transformers/perplexity](https://huggingface.co/docs/transformers/perplexity).
The perplexity measurements in table above are done against the `wikitext2` test dataset (https://paperswithcode.com/dataset/wikitext-2), with context length of 512.
The time per token is measured on a MacBook M1 Pro 32GB RAM using 4 and 8 threads.
#### How to run
1. Download/extract: https://huggingface.co/datasets/ggml-org/ci/resolve/main/wikitext-2-raw-v1.zip
2. Run `./perplexity -m models/7B/ggml-model-q4_0.gguf -f wiki.test.raw`
3. Output:
```
perplexity : calculating perplexity over 655 chunks
24.43 seconds per pass - ETA 4.45 hours
[1]4.5970,[2]5.1807,[3]6.0382,...
```
And after 4.45 hours, you will have the final perplexity.
### Interactive mode
If you want a more ChatGPT-like experience, you can run in interactive mode by passing `-i` as a parameter.
In this mode, you can always interrupt generation by pressing Ctrl+C and entering one or more lines of text, which will be converted into tokens and appended to the current context. You can also specify a *reverse prompt* with the parameter `-r "reverse prompt string"`. This will result in user input being prompted whenever the exact tokens of the reverse prompt string are encountered in the generation. A typical use is to use a prompt that makes LLaMA emulate a chat between multiple users, say Alice and Bob, and pass `-r "Alice:"`.
Here is an example of a few-shot interaction, invoked with the command
```bash
# default arguments using a 7B model
./examples/chat.sh
# advanced chat with a 13B model
./examples/chat-13B.sh
# custom arguments using a 13B model
./main -m ./models/13B/ggml-model-q4_0.gguf -n 256 --repeat_penalty 1.0 --color -i -r "User:" -f prompts/chat-with-bob.txt
```
Note the use of `--color` to distinguish between user input and generated text. Other parameters are explained in more detail in the [README](examples/main/README.md) for the `main` example program.
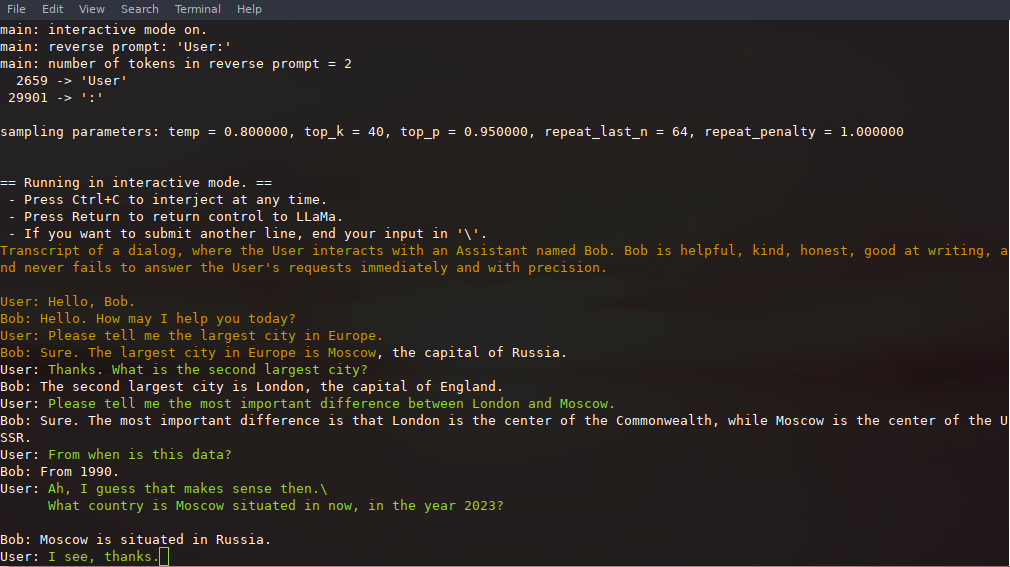
### Persistent Interaction
The prompt, user inputs, and model generations can be saved and resumed across calls to `./main` by leveraging `--prompt-cache` and `--prompt-cache-all`. The `./examples/chat-persistent.sh` script demonstrates this with support for long-running, resumable chat sessions. To use this example, you must provide a file to cache the initial chat prompt and a directory to save the chat session, and may optionally provide the same variables as `chat-13B.sh`. The same prompt cache can be reused for new chat sessions. Note that both prompt cache and chat directory are tied to the initial prompt (`PROMPT_TEMPLATE`) and the model file.
```bash
# Start a new chat
PROMPT_CACHE_FILE=chat.prompt.bin CHAT_SAVE_DIR=./chat/default ./examples/chat-persistent.sh
# Resume that chat
PROMPT_CACHE_FILE=chat.prompt.bin CHAT_SAVE_DIR=./chat/default ./examples/chat-persistent.sh
# Start a different chat with the same prompt/model
PROMPT_CACHE_FILE=chat.prompt.bin CHAT_SAVE_DIR=./chat/another ./examples/chat-persistent.sh
# Different prompt cache for different prompt/model
PROMPT_TEMPLATE=./prompts/chat-with-bob.txt PROMPT_CACHE_FILE=bob.prompt.bin \
CHAT_SAVE_DIR=./chat/bob ./examples/chat-persistent.sh
```
### Constrained output with grammars
`llama.cpp` supports grammars to constrain model output. For example, you can force the model to output JSON only:
```bash
./main -m ./models/13B/ggml-model-q4_0.gguf -n 256 --grammar-file grammars/json.gbnf -p 'Request: schedule a call at 8pm; Command:'
```
The `grammars/` folder contains a handful of sample grammars. To write your own, check out the [GBNF Guide](./grammars/README.md).
For authoring more complex JSON grammars, you can also check out https://grammar.intrinsiclabs.ai/, a browser app that lets you write TypeScript interfaces which it compiles to GBNF grammars that you can save for local use. Note that the app is built and maintained by members of the community, please file any issues or FRs on [its repo](http://github.com/intrinsiclabsai/gbnfgen) and not this one.
### Instruct mode
1. First, download and place the `ggml` model into the `./models` folder
2. Run the `main` tool like this:
```
./examples/alpaca.sh
```
Sample run:
```
== Running in interactive mode. ==
- Press Ctrl+C to interject at any time.
- Press Return to return control to LLaMA.
- If you want to submit another line, end your input in '\'.
Below is an instruction that describes a task. Write a response that appropriately completes the request.
> How many letters are there in the English alphabet?
There 26 letters in the English Alphabet
> What is the most common way of transportation in Amsterdam?
The majority (54%) are using public transit. This includes buses, trams and metros with over 100 lines throughout the city which make it very accessible for tourists to navigate around town as well as locals who commute by tram or metro on a daily basis
> List 5 words that start with "ca".
cadaver, cauliflower, cabbage (vegetable), catalpa (tree) and Cailleach.
>
```
### Obtaining and using the Facebook LLaMA 2 model
- Refer to [Facebook's LLaMA download page](https://ai.meta.com/resources/models-and-libraries/llama-downloads/) if you want to access the model data.
- Alternatively, if you want to save time and space, you can download already converted and quantized models from [TheBloke](https://huggingface.co/TheBloke), including:
- [LLaMA 2 7B base](https://huggingface.co/TheBloke/Llama-2-7B-GGUF)
- [LLaMA 2 13B base](https://huggingface.co/TheBloke/Llama-2-13B-GGUF)
- [LLaMA 2 70B base](https://huggingface.co/TheBloke/Llama-2-70B-GGUF)
- [LLaMA 2 7B chat](https://huggingface.co/TheBloke/Llama-2-7B-chat-GGUF)
- [LLaMA 2 13B chat](https://huggingface.co/TheBloke/Llama-2-13B-chat-GGUF)
- [LLaMA 2 70B chat](https://huggingface.co/TheBloke/Llama-2-70B-chat-GGUF)
### Seminal papers and background on the models
If your issue is with model generation quality, then please at least scan the following links and papers to understand the limitations of LLaMA models. This is especially important when choosing an appropriate model size and appreciating both the significant and subtle differences between LLaMA models and ChatGPT:
- LLaMA:
- [Introducing LLaMA: A foundational, 65-billion-parameter large language model](https://ai.facebook.com/blog/large-language-model-llama-meta-ai/)
- [LLaMA: Open and Efficient Foundation Language Models](https://arxiv.org/abs/2302.13971)
- GPT-3
- [Language Models are Few-Shot Learners](https://arxiv.org/abs/2005.14165)
- GPT-3.5 / InstructGPT / ChatGPT:
- [Aligning language models to follow instructions](https://openai.com/research/instruction-following)
- [Training language models to follow instructions with human feedback](https://arxiv.org/abs/2203.02155)
### Android
#### Building the Project using Android NDK
You can easily run `llama.cpp` on Android device with [termux](https://termux.dev/).
First, install the essential packages for termux:
```
pkg install clang wget git cmake
```
Second, obtain the [Android NDK](https://developer.android.com/ndk) and then build with CMake:
You can execute the following commands on your computer to avoid downloading the NDK to your mobile. Of course, you can also do this in Termux.
```
$ mkdir build-android
$ cd build-android
$ export NDK=<your_ndk_directory>
$ cmake -DCMAKE_TOOLCHAIN_FILE=$NDK/build/cmake/android.toolchain.cmake -DANDROID_ABI=arm64-v8a -DANDROID_PLATFORM=android-23 -DCMAKE_C_FLAGS=-march=armv8.4a+dotprod ..
$ make
```
Install [termux](https://termux.dev/) on your device and run `termux-setup-storage` to get access to your SD card.
Finally, copy these built `llama` binaries and the model file to your device storage. Because the file permissions in the Android sdcard cannot be changed, you can copy the executable files to the `/data/data/com.termux/files/home/bin` path, and then execute the following commands in Termux to add executable permission:
(Assumed that you have pushed the built executable files to the /sdcard/llama.cpp/bin path using `adb push`)
```
$cp -r /sdcard/llama.cpp/bin /data/data/com.termux/files/home/
$cd /data/data/com.termux/files/home/bin
$chmod +x ./*
```
Download model [llama-2-7b-chat.Q4_K_M.gguf](https://huggingface.co/TheBloke/Llama-2-7B-Chat-GGUF/blob/main/llama-2-7b-chat.Q4_K_M.gguf), and push it to `/sdcard/llama.cpp/`, then move it to `/data/data/com.termux/files/home/model/`
```
$mv /sdcard/llama.cpp/llama-2-7b-chat.Q4_K_M.gguf /data/data/com.termux/files/home/model/
```
Now, you can start chatting:
```
$cd /data/data/com.termux/files/home/bin
$./main -m ../model/llama-2-7b-chat.Q4_K_M.gguf -n 128 -cml
```
Here is a demo of an interactive session running on Pixel 5 phone:
https://user-images.githubusercontent.com/271616/225014776-1d567049-ad71-4ef2-b050-55b0b3b9274c.mp4
#### Building the Project using Termux (F-Droid)
Termux from F-Droid offers an alternative route to execute the project on an Android device. This method empowers you to construct the project right from within the terminal, negating the requirement for a rooted device or SD Card.
Outlined below are the directives for installing the project using OpenBLAS and CLBlast. This combination is specifically designed to deliver peak performance on recent devices that feature a GPU.
If you opt to utilize OpenBLAS, you'll need to install the corresponding package.
```
apt install libopenblas
```
Subsequently, if you decide to incorporate CLBlast, you'll first need to install the requisite OpenCL packages:
```
apt install ocl-icd opencl-headers opencl-clhpp clinfo
```
In order to compile CLBlast, you'll need to first clone the respective Git repository, which can be found at this URL: https://github.com/CNugteren/CLBlast. Alongside this, clone this repository into your home directory. Once this is done, navigate to the CLBlast folder and execute the commands detailed below:
```
cmake .
make
cp libclblast.so* $PREFIX/lib
cp ./include/clblast.h ../llama.cpp
```
Following the previous steps, navigate to the LlamaCpp directory. To compile it with OpenBLAS and CLBlast, execute the command provided below:
```
cp /data/data/com.termux/files/usr/include/openblas/cblas.h .
cp /data/data/com.termux/files/usr/include/openblas/openblas_config.h .
make LLAMA_CLBLAST=1 //(sometimes you need to run this command twice)
```
Upon completion of the aforementioned steps, you will have successfully compiled the project. To run it using CLBlast, a slight adjustment is required: a command must be issued to direct the operations towards your device's physical GPU, rather than the virtual one. The necessary command is detailed below:
```
GGML_OPENCL_PLATFORM=0
GGML_OPENCL_DEVICE=0
export LD_LIBRARY_PATH=/vendor/lib64:$LD_LIBRARY_PATH
```
(Note: some Android devices, like the Zenfone 8, need the following command instead - "export LD_LIBRARY_PATH=/system/vendor/lib64:$LD_LIBRARY_PATH". Source: https://www.reddit.com/r/termux/comments/kc3ynp/opencl_working_in_termux_more_in_comments/ )
For easy and swift re-execution, consider documenting this final part in a .sh script file. This will enable you to rerun the process with minimal hassle.
Place your desired model into the `~/llama.cpp/models/` directory and execute the `./main (...)` script.
### Docker
#### Prerequisites
* Docker must be installed and running on your system.
* Create a folder to store big models & intermediate files (ex. /llama/models)
#### Images
We have three Docker images available for this project:
1. `ghcr.io/ggerganov/llama.cpp:full`: This image includes both the main executable file and the tools to convert LLaMA models into ggml and convert into 4-bit quantization. (platforms: `linux/amd64`, `linux/arm64`)
2. `ghcr.io/ggerganov/llama.cpp:light`: This image only includes the main executable file. (platforms: `linux/amd64`, `linux/arm64`)
3. `ghcr.io/ggerganov/llama.cpp:server`: This image only includes the server executable file. (platforms: `linux/amd64`, `linux/arm64`)
Additionally, there the following images, similar to the above:
- `ghcr.io/ggerganov/llama.cpp:full-cuda`: Same as `full` but compiled with CUDA support. (platforms: `linux/amd64`)
- `ghcr.io/ggerganov/llama.cpp:light-cuda`: Same as `light` but compiled with CUDA support. (platforms: `linux/amd64`)
- `ghcr.io/ggerganov/llama.cpp:server-cuda`: Same as `server` but compiled with CUDA support. (platforms: `linux/amd64`)
- `ghcr.io/ggerganov/llama.cpp:full-rocm`: Same as `full` but compiled with ROCm support. (platforms: `linux/amd64`, `linux/arm64`)
- `ghcr.io/ggerganov/llama.cpp:light-rocm`: Same as `light` but compiled with ROCm support. (platforms: `linux/amd64`, `linux/arm64`)
- `ghcr.io/ggerganov/llama.cpp:server-rocm`: Same as `server` but compiled with ROCm support. (platforms: `linux/amd64`, `linux/arm64`)
The GPU enabled images are not currently tested by CI beyond being built. They are not built with any variation from the ones in the Dockerfiles defined in [.devops/](.devops/) and the GitHub Action defined in [.github/workflows/docker.yml](.github/workflows/docker.yml). If you need different settings (for example, a different CUDA or ROCm library, you'll need to build the images locally for now).
#### Usage
The easiest way to download the models, convert them to ggml and optimize them is with the --all-in-one command which includes the full docker image.
Replace `/path/to/models` below with the actual path where you downloaded the models.
```bash
docker run -v /path/to/models:/models ghcr.io/ggerganov/llama.cpp:full --all-in-one "/models/" 7B
```
On completion, you are ready to play!
```bash
docker run -v /path/to/models:/models ghcr.io/ggerganov/llama.cpp:full --run -m /models/7B/ggml-model-q4_0.gguf -p "Building a website can be done in 10 simple steps:" -n 512
```
or with a light image:
```bash
docker run -v /path/to/models:/models ghcr.io/ggerganov/llama.cpp:light -m /models/7B/ggml-model-q4_0.gguf -p "Building a website can be done in 10 simple steps:" -n 512
```
or with a server image:
```bash
docker run -v /path/to/models:/models -p 8000:8000 ghcr.io/ggerganov/llama.cpp:server -m /models/7B/ggml-model-q4_0.gguf --port 8000 --host 0.0.0.0 -n 512
```
### Docker With CUDA
Assuming one has the [nvidia-container-toolkit](https://github.com/NVIDIA/nvidia-container-toolkit) properly installed on Linux, or is using a GPU enabled cloud, `cuBLAS` should be accessible inside the container.
#### Building Locally
```bash
docker build -t local/llama.cpp:full-cuda -f .devops/full-cuda.Dockerfile .
docker build -t local/llama.cpp:light-cuda -f .devops/main-cuda.Dockerfile .
docker build -t local/llama.cpp:server-cuda -f .devops/server-cuda.Dockerfile .
```
You may want to pass in some different `ARGS`, depending on the CUDA environment supported by your container host, as well as the GPU architecture.
The defaults are:
- `CUDA_VERSION` set to `11.7.1`
- `CUDA_DOCKER_ARCH` set to `all`
The resulting images, are essentially the same as the non-CUDA images:
1. `local/llama.cpp:full-cuda`: This image includes both the main executable file and the tools to convert LLaMA models into ggml and convert into 4-bit quantization.
2. `local/llama.cpp:light-cuda`: This image only includes the main executable file.
3. `local/llama.cpp:server-cuda`: This image only includes the server executable file.
#### Usage
After building locally, Usage is similar to the non-CUDA examples, but you'll need to add the `--gpus` flag. You will also want to use the `--n-gpu-layers` flag.
```bash
docker run --gpus all -v /path/to/models:/models local/llama.cpp:full-cuda --run -m /models/7B/ggml-model-q4_0.gguf -p "Building a website can be done in 10 simple steps:" -n 512 --n-gpu-layers 1
docker run --gpus all -v /path/to/models:/models local/llama.cpp:light-cuda -m /models/7B/ggml-model-q4_0.gguf -p "Building a website can be done in 10 simple steps:" -n 512 --n-gpu-layers 1
docker run --gpus all -v /path/to/models:/models local/llama.cpp:server-cuda -m /models/7B/ggml-model-q4_0.gguf --port 8000 --host 0.0.0.0 -n 512 --n-gpu-layers 1
```
### Contributing
- Contributors can open PRs
- Collaborators can push to branches in the `llama.cpp` repo and merge PRs into the `master` branch
- Collaborators will be invited based on contributions
- Any help with managing issues and PRs is very appreciated!
- Make sure to read this: [Inference at the edge](https://github.com/ggerganov/llama.cpp/discussions/205)
- A bit of backstory for those who are interested: [Changelog podcast](https://changelog.com/podcast/532)
### Coding guidelines
- Avoid adding third-party dependencies, extra files, extra headers, etc.
- Always consider cross-compatibility with other operating systems and architectures
- Avoid fancy looking modern STL constructs, use basic `for` loops, avoid templates, keep it simple
- There are no strict rules for the code style, but try to follow the patterns in the code (indentation, spaces, etc.). Vertical alignment makes things more readable and easier to batch edit
- Clean-up any trailing whitespaces, use 4 spaces for indentation, brackets on the same line, `void * ptr`, `int & a`
- See [good first issues](https://github.com/ggerganov/llama.cpp/issues?q=is%3Aissue+is%3Aopen+label%3A%22good+first+issue%22) for tasks suitable for first contributions
- Tensors store data in row-major order. We refer to dimension 0 as columns, 1 as rows, 2 as matrices
- Matrix multiplication is unconventional: [`C = ggml_mul_mat(ctx, A, B)`](https://github.com/ggerganov/llama.cpp/blob/880e352277fc017df4d5794f0c21c44e1eae2b84/ggml.h#L1058-L1064) means $C^T = A B^T \Leftrightarrow C = B A^T.$

### Docs
- [main](./examples/main/README.md)
- [server](./examples/server/README.md)
- [jeopardy](./examples/jeopardy/README.md)
- [BLIS](./docs/BLIS.md)
- [Performance troubleshooting](./docs/token_generation_performance_tips.md)
- [GGML tips & tricks](https://github.com/ggerganov/llama.cpp/wiki/GGML-Tips-&-Tricks)
- [GBNF grammars](./grammars/README.md)
|