Spaces:
Runtime error
Runtime error
File size: 4,716 Bytes
8366b03 |
1 2 3 4 5 6 7 8 9 10 11 12 13 14 15 16 17 18 19 20 21 22 23 24 25 26 27 28 29 30 31 32 33 34 35 36 37 38 39 40 41 42 43 44 45 46 47 48 49 50 51 52 53 54 55 56 57 58 59 60 61 62 63 64 65 66 67 68 69 70 71 72 73 74 75 76 77 78 79 80 81 82 83 84 85 86 87 88 89 90 91 92 93 94 95 96 97 98 99 100 101 102 103 104 105 106 107 108 109 110 111 112 113 114 115 116 117 118 119 120 121 122 123 124 125 126 127 128 129 130 131 132 133 134 135 136 137 138 139 140 141 142 143 144 145 146 147 148 149 150 151 152 153 154 155 156 |
# ImageBind: One Embedding Space To Bind Them All
**[FAIR, Meta AI](https://ai.facebook.com/research/)**
Rohit Girdhar*,
Alaaeldin El-Nouby*,
Zhuang Liu,
Mannat Singh,
Kalyan Vasudev Alwala,
Armand Joulin,
Ishan Misra*
To appear at CVPR 2023 (*Highlighted paper*)
[[`Paper`](https://facebookresearch.github.io/ImageBind/paper)] [[`Blog`](https://ai.facebook.com/blog/imagebind-six-modalities-binding-ai/)] [[`Demo`](https://imagebind.metademolab.com/)] [[`Supplementary Video`](https://dl.fbaipublicfiles.com/imagebind/imagebind_video.mp4)] [[`BibTex`](#citing-imagebind)]
PyTorch implementation and pretrained models for ImageBind. For details, see the paper: **[ImageBind: One Embedding Space To Bind Them All](https://facebookresearch.github.io/ImageBind/paper)**.
ImageBind learns a joint embedding across six different modalities - images, text, audio, depth, thermal, and IMU data. It enables novel emergent applications ‘out-of-the-box’ including cross-modal retrieval, composing modalities with arithmetic, cross-modal detection and generation.
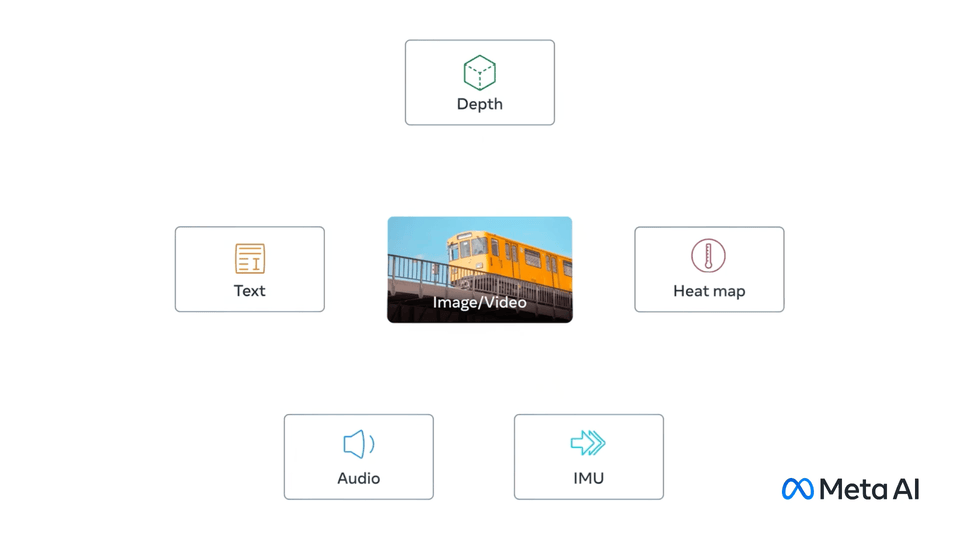
## ImageBind model
Emergent zero-shot classification performance.
<table style="margin: auto">
<tr>
<th>Model</th>
<th><span style="color:blue">IN1k</span></th>
<th><span style="color:purple">K400</span></th>
<th><span style="color:green">NYU-D</span></th>
<th><span style="color:LightBlue">ESC</span></th>
<th><span style="color:orange">LLVIP</span></th>
<th><span style="color:purple">Ego4D</span></th>
<th>download</th>
</tr>
<tr>
<td>imagebind_huge</td>
<td align="right">77.7</td>
<td align="right">50.0</td>
<td align="right">54.0</td>
<td align="right">66.9</td>
<td align="right">63.4</td>
<td align="right">25.0</td>
<td><a href="https://dl.fbaipublicfiles.com/imagebind/imagebind_huge.pth">checkpoint</a></td>
</tr>
</table>
## Usage
Install pytorch 1.13+ and other 3rd party dependencies.
```shell
conda create --name imagebind python=3.8 -y
conda activate imagebind
pip install -r requirements.txt
```
For windows users, you might need to install `soundfile` for reading/writing audio files. (Thanks @congyue1977)
```
pip install soundfile
```
Extract and compare features across modalities (e.g. Image, Text and Audio).
```python
import data
import torch
from models import imagebind_model
from models.imagebind_model import ModalityType
text_list=["A dog.", "A car", "A bird"]
image_paths=[".assets/dog_image.jpg", ".assets/car_image.jpg", ".assets/bird_image.jpg"]
audio_paths=[".assets/dog_audio.wav", ".assets/car_audio.wav", ".assets/bird_audio.wav"]
device = "cuda:0" if torch.cuda.is_available() else "cpu"
# Instantiate model
model = imagebind_model.imagebind_huge(pretrained=True)
model.eval()
model.to(device)
# Load data
inputs = {
ModalityType.TEXT: data.load_and_transform_text(text_list, device),
ModalityType.VISION: data.load_and_transform_vision_data(image_paths, device),
ModalityType.AUDIO: data.load_and_transform_audio_data(audio_paths, device),
}
with torch.no_grad():
embeddings = model(inputs)
print(
"Vision x Text: ",
torch.softmax(embeddings[ModalityType.VISION] @ embeddings[ModalityType.TEXT].T, dim=-1),
)
print(
"Audio x Text: ",
torch.softmax(embeddings[ModalityType.AUDIO] @ embeddings[ModalityType.TEXT].T, dim=-1),
)
print(
"Vision x Audio: ",
torch.softmax(embeddings[ModalityType.VISION] @ embeddings[ModalityType.AUDIO].T, dim=-1),
)
# Expected output:
#
# Vision x Text:
# tensor([[9.9761e-01, 2.3694e-03, 1.8612e-05],
# [3.3836e-05, 9.9994e-01, 2.4118e-05],
# [4.7997e-05, 1.3496e-02, 9.8646e-01]])
#
# Audio x Text:
# tensor([[1., 0., 0.],
# [0., 1., 0.],
# [0., 0., 1.]])
#
# Vision x Audio:
# tensor([[0.8070, 0.1088, 0.0842],
# [0.1036, 0.7884, 0.1079],
# [0.0018, 0.0022, 0.9960]])
```
## Model card
Please see the [model card](model_card.md) for details.
## License
ImageBind code and model weights are released under the CC-BY-NC 4.0 license. See [LICENSE](LICENSE) for additional details.
## Contributing
See [contributing](CONTRIBUTING.md) and the [code of conduct](CODE_OF_CONDUCT.md).
## Citing ImageBind
If you find this repository useful, please consider giving a star :star: and citation
```
@inproceedings{girdhar2023imagebind,
title={ImageBind: One Embedding Space To Bind Them All},
author={Girdhar, Rohit and El-Nouby, Alaaeldin and Liu, Zhuang
and Singh, Mannat and Alwala, Kalyan Vasudev and Joulin, Armand and Misra, Ishan},
booktitle={CVPR},
year={2023}
}
```
|