|
--- |
|
license: cc-by-nc-nd-4.0 |
|
tags: |
|
- moe |
|
- merge |
|
- medical |
|
- mergekit |
|
- sethuiyer/Dr_Samantha_7b_mistral |
|
- fblgit/UNA-TheBeagle-7b-v1 |
|
language: |
|
- en |
|
datasets: |
|
- medmcqa |
|
- cognitivecomputations/samantha-data |
|
- jondurbin/bagel-v0.3 |
|
library_name: transformers |
|
pipeline_tag: text-generation |
|
--- |
|
|
|
# MedleyMD |
|
|
|
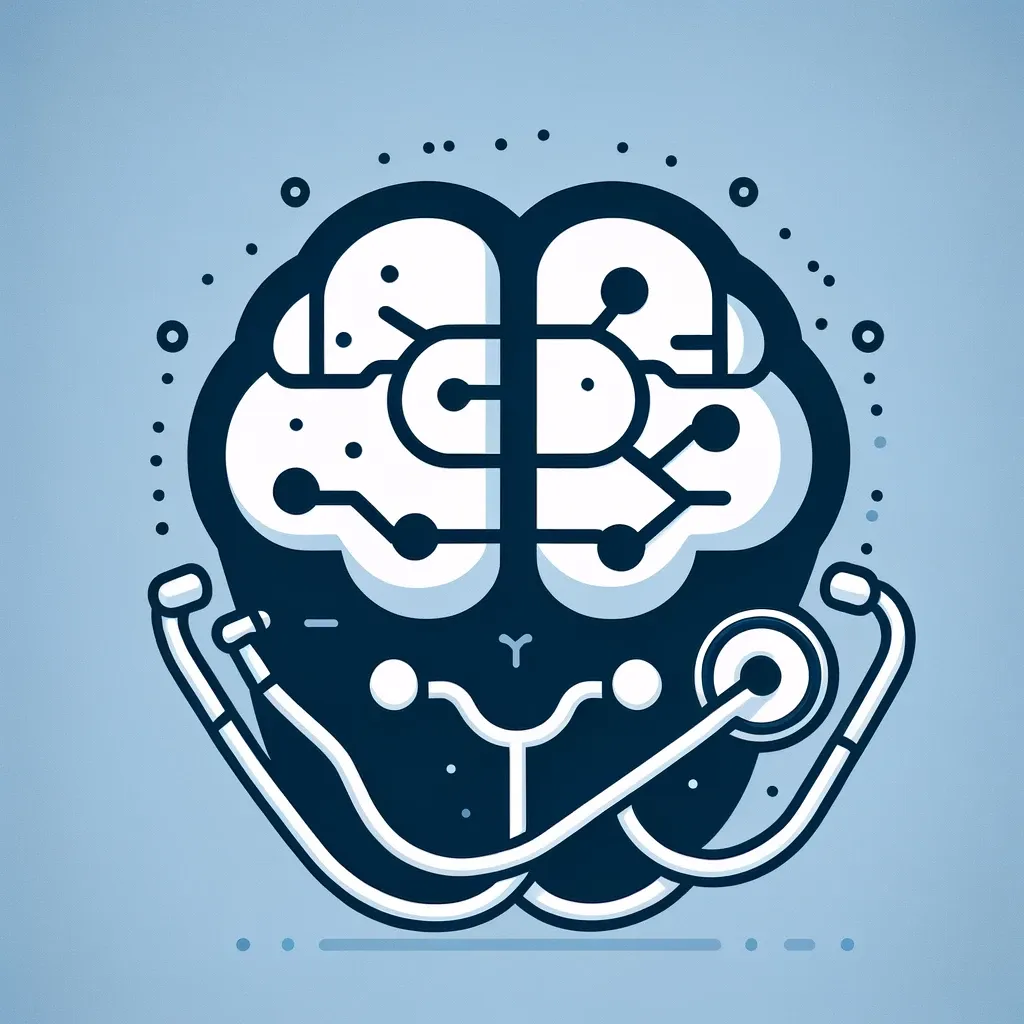 |
|
|
|
|
|
MedleyMD is a Mixure of Experts (MoE) made with the following models using [LazyMergekit](https://colab.research.google.com/drive/1obulZ1ROXHjYLn6PPZJwRR6GzgQogxxb?usp=sharing): |
|
* [sethuiyer/Dr_Samantha_7b_mistral](https://huggingface.co/sethuiyer/Dr_Samantha_7b_mistral) |
|
* [fblgit/UNA-TheBeagle-7b-v1](https://huggingface.co/fblgit/UNA-TheBeagle-7b-v1) |
|
|
|
These models were chosen because `fblgit/UNA-TheBeagle-7b-v1` has excellent performance for a 7B parameter model and Dr.Samantha has capabilities of a medical knowledge-focused model (trained on USMLE databases and doctor-patient interactions) with the philosophical, psychological, and relational understanding, scoring 68.82% in topics related to clinical domain and psychology. |
|
|
|
## Benchmark |
|
|
|
On a synthetic benchmark of 15 medical diagnosis questions generated by GPT-4, GPT-4 also being an evaluator, MedleyMD scored **94.25/100**. |
|
|
|
Nous Benchmark numbers shall be available soon. |
|
|
|
|
|
## 🧩 Configuration |
|
|
|
```yaml |
|
base_model: OpenPipe/mistral-ft-optimized-1227 |
|
gate_mode: hidden |
|
dtype: bfloat16 |
|
|
|
experts: |
|
- source_model: sethuiyer/Dr_Samantha_7b_mistral |
|
positive_prompts: ["differential diagnosis", "Clinical Knowledge", "Medical Genetics", "Human Aging", "Human Sexuality", "College Medicine", "Anatomy", "College Biology", "High School Biology", "Professional Medicine", "Nutrition", "High School Psychology", "Professional Psychology", "Virology"] |
|
|
|
- source_model: fblgit/UNA-TheBeagle-7b-v1 |
|
positive_prompts: ["How do you", "Explain the concept of", "Give an overview of", "Compare and contrast between", "Provide information about", "Help me understand", "Summarize", "Make a recommendation on", "chat", "math", "reason", "mathematics", "solve", "count", "python", "javascript", "programming", "algorithm", "tell me", "assistant"] |
|
|
|
``` |
|
|
|
## GGUF |
|
1. [medleymd.Q4_K_M](https://huggingface.co/sethuiyer/MedleyMD-GGUF/resolve/main/medleymd.Q4_K_M.gguf) [7.2GB] |
|
2. [medleymd.Q5_K_M](https://huggingface.co/sethuiyer/MedleyMD-GGUF/resolve/main/medleymd.Q5_K_M.gguf) [9.13GB] |
|
|
|
|
|
## Ollama |
|
|
|
MedleyMD can be used in ollama by running```ollama run stuehieyr/medleymd``` in your terminal. |
|
|
|
If you have limited computing resources, check out this [video](https://www.youtube.com/watch?v=Qa1h7ygwQq8) to learn how to run it on |
|
a Google Colab backend. |
|
|
|
## 💻 Usage |
|
|
|
```python |
|
!pip install -qU transformers bitsandbytes accelerate |
|
|
|
from transformers import AutoTokenizer |
|
import transformers |
|
import torch |
|
|
|
model = "sethuiyer/MedleyMD" |
|
|
|
tokenizer = AutoTokenizer.from_pretrained(model) |
|
pipeline = transformers.pipeline( |
|
"text-generation", |
|
model=model, |
|
model_kwargs={"torch_dtype": torch.bfloat16, "load_in_4bit": True}, |
|
) |
|
|
|
generation_kwargs = { |
|
"max_new_tokens": 512, |
|
"do_sample": True, |
|
"temperature": 0.7, |
|
"top_k": 50, |
|
"top_p": 95, |
|
} |
|
|
|
messages = [{"role":"system", "content":"You are an helpful AI assistant. Please use </s> when you want to end the answer."}, |
|
{"role": "user", "content": "Explain what a Mixture of Experts is in less than 100 words."}] |
|
prompt = pipeline.tokenizer.apply_chat_template(messages, tokenize=False, add_generation_prompt=True) |
|
outputs = pipeline(prompt, **generation_kwargs) |
|
print(outputs[0]["generated_text"]) |
|
``` |
|
|
|
```text |
|
A Mixture of Experts (Mixout) is a neural network architecture that combines the strengths of multiple expert networks to make a more accurate and robust prediction. |
|
It is composed of a topmost gating network that assigns weights to each expert network based on their performance on past input samples. |
|
The expert networks are trained independently, and the gating network learns to choose the best combination of these experts to make the final prediction. |
|
Mixout demonstrates a stronger ability to handle complex data distributions and is more efficient in terms of training time and memory usage compared to a |
|
traditional ensemble approach. |
|
``` |