File size: 4,966 Bytes
1ef680a fc78143 164aee6 f15013b 1ef680a 2c8ad5c 1ef680a 2c8ad5c 424abe1 2c8ad5c 0b8fea2 2c8ad5c 424abe1 208de4c 2c8ad5c 1ef680a 2c8ad5c 1ef680a 2c8ad5c 1ef680a 2c8ad5c 1ef680a 2c8ad5c 1ef680a 2c8ad5c 1ef680a 0b8fea2 376bb59 1ef680a 0b8fea2 1ef680a 376bb59 1ef680a 376bb59 3ac748f 376bb59 51b99cc 376bb59 51b99cc |
1 2 3 4 5 6 7 8 9 10 11 12 13 14 15 16 17 18 19 20 21 22 23 24 25 26 27 28 29 30 31 32 33 34 35 36 37 38 39 40 41 42 43 44 45 46 47 48 49 50 51 52 53 54 55 56 57 58 59 60 61 62 63 64 65 66 67 68 69 70 71 72 73 74 75 76 77 78 79 80 81 82 83 84 85 86 87 88 89 90 91 92 93 94 95 96 97 98 99 100 101 102 103 104 105 106 107 108 109 110 111 112 113 114 115 116 117 118 119 120 121 122 123 124 125 126 127 128 129 |
---
tags:
- generated_from_trainer
model-index:
- name: EUBERT
results: []
language:
- bg
- cs
- da
- de
- el
- en
- es
- et
- fi
- fr
- ga
- hr
- hu
- it
- lt
- lv
- mt
- nl
- pl
- pt
- ro
- sk
- sl
- sv
widget:
- text: "The transition to a climate neutral, sustainable, energy and resource-efficient, circular and fair economy is key to ensuring the long-term competitiveness of the economy of the union and the well-being of its peoples. In 2016, the Union concluded the Paris Agreement2. Article 2(1), point (c), of the Paris Agreement sets out the objective of strengthening the response to climate change by, among other means, making finance flows consistent with a pathway towards low greenhouse gas [MASK] and climate resilient development."
---
<!-- This model card has been generated automatically according to the information the Trainer had access to. You
should probably proofread and complete it, then remove this comment. -->
## Model Card: EUBERT
### Overview
- **Model Name**: EUBERT
- **Model Version**: 1.1
- **Date of Release**: 16 October 2023
- **Model Architecture**: BERT (Bidirectional Encoder Representations from Transformers)
- **Training Data**: Documents registered by the European Publications Office
- **Model Use Case**: Text Classification, Question Answering, Language Understanding
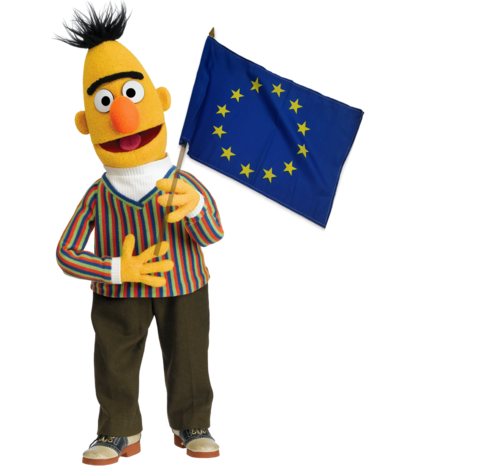
### Model Description
EUBERT is a pretrained BERT uncased model that has been trained on a vast corpus of documents registered by the [European Publications Office](https://op.europa.eu/).
These documents span the last 30 years, providing a comprehensive dataset that encompasses a wide range of topics and domains.
EUBERT is designed to be a versatile language model that can be fine-tuned for various natural language processing tasks,
making it a valuable resource for a variety of applications.
### Intended Use
EUBERT serves as a starting point for building more specific natural language understanding models.
Its versatility makes it suitable for a wide range of tasks, including but not limited to:
1. **Text Classification**: EUBERT can be fine-tuned for classifying text documents into different categories, making it useful for applications such as sentiment analysis, topic categorization, and spam detection.
2. **Question Answering**: By fine-tuning EUBERT on question-answering datasets, it can be used to extract answers from text documents, facilitating tasks like information retrieval and document summarization.
3. **Language Understanding**: EUBERT can be employed for general language understanding tasks, including named entity recognition, part-of-speech tagging, and text generation.
### Performance
The specific performance metrics of EUBERT may vary depending on the downstream task and the quality and quantity of training data used for fine-tuning.
Users are encouraged to fine-tune the model on their specific task and evaluate its performance accordingly.
### Considerations
- **Data Privacy and Compliance**: Users should ensure that the use of EUBERT complies with all relevant data privacy and compliance regulations, especially when working with sensitive or personally identifiable information.
- **Fine-Tuning**: The effectiveness of EUBERT on a given task depends on the quality and quantity of the training data, as well as the fine-tuning process. Careful experimentation and evaluation are essential to achieve optimal results.
- **Bias and Fairness**: Users should be aware of potential biases in the training data and take appropriate measures to mitigate bias when fine-tuning EUBERT for specific tasks.
### Conclusion
EUBERT is a pretrained BERT model that leverages a substantial corpus of documents from the European Publications Office. It offers a versatile foundation for developing natural language processing solutions across a wide range of applications, enabling researchers and developers to create custom models for text classification, question answering, and language understanding tasks. Users are encouraged to exercise diligence in fine-tuning and evaluating the model for their specific use cases while adhering to data privacy and fairness considerations.
---
## Training procedure
Dedicated Word Piece tokenizer vocabulary size 2**16,
### Training hyperparameters
The following hyperparameters were used during training:
- learning_rate: 5e-05
- train_batch_size: 32
- eval_batch_size: 32
- seed: 42
- optimizer: Adam with betas=(0.9,0.999) and epsilon=1e-08
- lr_scheduler_type: linear
- num_epochs: 1.85
### Training results
Coming soon
### Framework versions
- Transformers 4.33.3
- Pytorch 2.0.1+cu117
- Datasets 2.14.5
- Tokenizers 0.13.3
### Infrastructure
- **Hardware Type:** 4 x GPUs 24GB
- **GPU Days:** 16
- **Cloud Provider:** EuroHPC
- **Compute Region:** Meluxina
# Author(s)
Sébastien Campion <sebastien.campion@europarl.europa.eu>
|