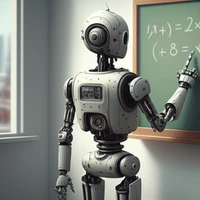
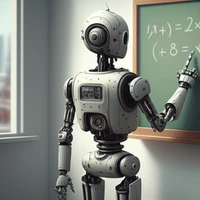
sc-genrm-scaling
AI & ML interests
None defined yet.
Recent Activity
Data and models accompanying the paper When To Solve, When To Verify: Compute-Optimal Problem Solving and Generative Verification for LLM Reasoning, containing:
- Finetuned generative verifiers (i.e., GenRM-FT) for math reasoning.
- Synthetic verification data generated by GPT-4o for math reasoning to train your own generative verifiers.
- Solutions and verifications generated by various models for math and science reasoning.
MATH Dataset
We use Llama-3.1-8B-Instruct and Qwen-2.5-7B-Instruct to generate solutions for problems in the training split of the MATH dataset. Then, we use GPT-4o to verify these solutions. We filter out the verifications whose verdict doesn't match the ground-truth correctness of the solution, and balance the dataset to have equal 'yes' and 'no' verifications in the dataset. This results in these datasets:
Training data for GenRM-FT
- Llama-3.1-8B-Instruct: https://huggingface.co/datasets/sc-genrm-scaling/genrm_gpt4o_verifs_llama_3p1_8b_solns_math_train
- Qwen-2.5.-7B-Instruct: https://huggingface.co/datasets/sc-genrm-scaling/genrm_gpt4o_verifs_qwen_2p5_7b_solns_math_train
We fine-tune the two models on their respective datasets using LoRA, resulting in these fine-tuned GenRMs:
Finetuned Verifiers:
- Llama-3.1-8B-Instruct: https://huggingface.co/sc-genrm-scaling/llama_3.1_8b_genrm_ft
- Qwen-2.5.-7B-Instruct: https://huggingface.co/sc-genrm-scaling/qwen_2.5_7b_genrm_ft
You can follow this example of how to do inference with these models.
We use these generative verifiers (without fine-tuning in the case of Llama-3.3-70B-Instruct) on solutions from the MATH test set to obtain this data, which we analyse in the paper:
Solutions and Verifications for Test-set
Llama-3.1-8B-Instruct:
- Solutions: https://huggingface.co/datasets/sc-genrm-scaling/MATH128_Solutions_Llama-3.1-8B-Instruct
- Verifications (Finetuned Verifier): https://huggingface.co/datasets/sc-genrm-scaling/MATH128_verifications_GenRM-FT_Llama-3.1-8B-Instruct
Llama-3.3-70B-Instruct:
Qwen-2.5-7B-Instruct:
- Solutions: https://huggingface.co/datasets/sc-genrm-scaling/MATH128_Solutions_Qwen-2.5-7B-Instruct
- Verifications (Finetuned Verifier): https://huggingface.co/datasets/sc-genrm-scaling/MATH128_verifications_GenRM-FT_Qwen-2.5-7B-Instruct
AIME25
Solutions and Verifications
- QwQ-32B:
- Solutions: https://huggingface.co/datasets/sc-genrm-scaling/AIME25_Solutions_QwQ-32B
- Verifications (Without Finetuning): https://huggingface.co/datasets/sc-genrm-scaling/AIME25_verifications_QwQ32B
GPQA
Solutions and Verifications
- Llama-3.3-70B-Instruct:
models
2
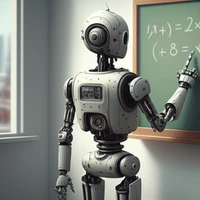
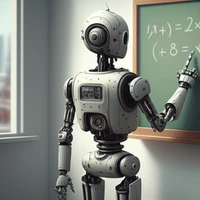