File size: 2,909 Bytes
58e473a 29016d4 58e473a 3bca8df 7b864d8 3a518f4 7b864d8 b87e7e0 0033227 7b864d8 0b08dc0 e04a520 3bca8df 73bc272 3bca8df 73bc272 3bca8df 3a518f4 |
1 2 3 4 5 6 7 8 9 10 11 12 13 14 15 16 17 18 19 20 21 22 23 24 25 26 27 28 29 30 31 32 33 34 35 36 37 38 39 40 41 42 43 44 45 46 47 48 49 50 51 52 53 54 55 56 57 58 59 |
---
license: mit
pipeline_tag: zero-shot-image-classification
---
## Quick Start with AutoModel
For this image, 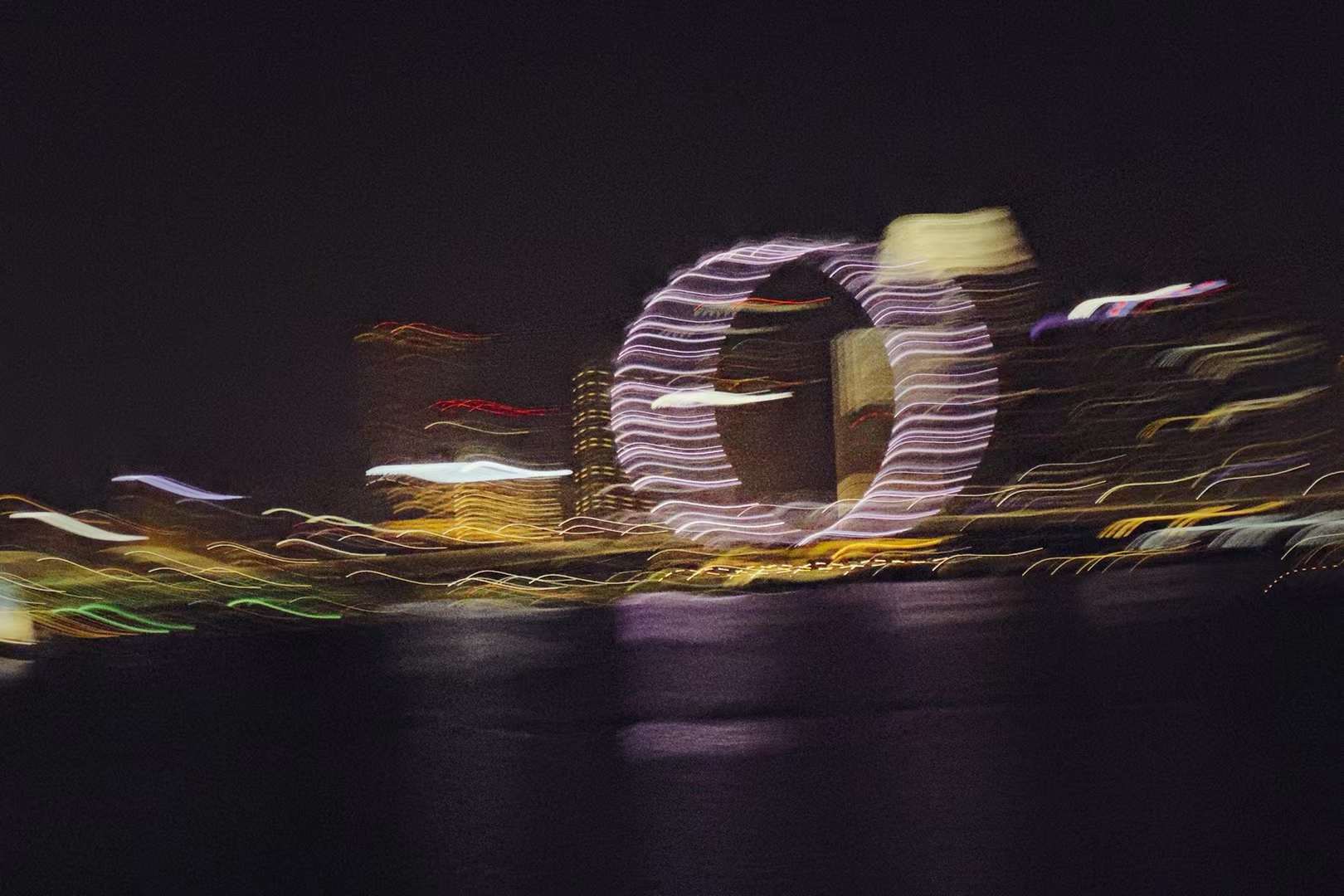 start an AutoModel scorer with `transformers==4.36.1`:
```python
import requests
import torch
from transformers import AutoModelForCausalLM
model = AutoModelForCausalLM.from_pretrained("q-future/one-align", trust_remote_code=True, attn_implementation="eager",
torch_dtype=torch.float16, device_map="auto")
from PIL import Image
url = "https://raw.githubusercontent.com/Q-Future/Q-Align/main/fig/singapore_flyer.jpg"
image = Image.open(requests.get(url,stream=True).raw)
model.score([image], task_="quality", input_="image")
# task_ : quality | aesthetics; # input_: image | video
```
Result should be 1.911 (in range [1,5], higher is better).
From paper: `arxiv.org/abs/2312.17090`.
## Syllabus
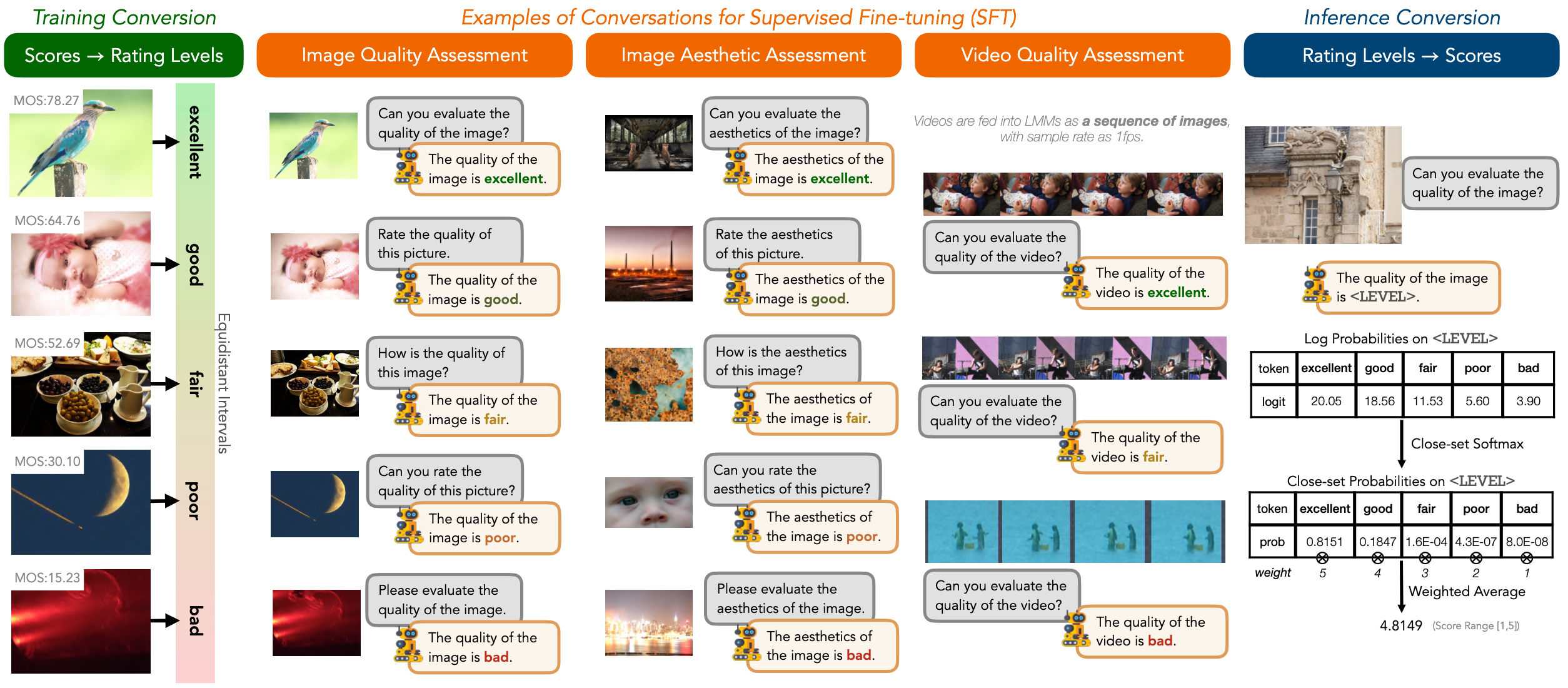
## IQA Results (Spearman/Pearson/Kendall)
|Datasets | KonIQ (NR-IQA, seen) | SPAQ (NR-IQA, Seen) | KADID (FR-IQA, Seen) | LIVE-C (NR-IQA, *Unseen*) | LIVE (FR-IQA, *Unseen*) | CSIQ (FR-IQA, *Unseen*) | AGIQA (AIGC, *Unseen*)|
| --- | --- | --- | --- | --- | --- | --- | --- |
| *Previous SOTA* | 0.916/0.928 (MUSIQ, ICCV2021) | 0.922/0.919 (LIQE, CVPR2023) | 0.934/0.937 (CONTRIQUE, TIP2022) | NA | NA | NA | NA |
| Q-Align (IQA) | 0.937/0.945/0.785 | 0.931/0.933/0.763 | 0.934/0.934/0.777 | 0.887/0.896/0.706 | 0.874/0.840/0.682 | 0.845/0.876/0.654 | 0.731/0.791/0.529 |
| Q-Align (IQA+VQA) | **0.944**/0.949/**0.797** | 0.931/0.934/0.764 | **0.952**/**0.953**/**0.809** | **0.892**/**0.899**/**0.715** | 0.874/0.846/0.684 | 0.852/0.876/0.663 | 0.739/0.782/0.526 |
| **OneAlign** (IQA+IAA+VQA) | 0.941/**0.950**/0.791 | **0.932**/**0.935**/**0.766** | 0.941/0.942/0.791 | 0.881/0.894/0.699 | **0.887**/**0.856**/**0.699** | **0.881**/**0.906**/**0.699** | **0.801**/**0.838**/**0.602** |
## IAA Results (Spearman/Pearson)
| Dataset | AVA_test |
| --- | --- |
| *VILA (CVPR, 2023)* | 0.774/0.774 |
| *LIQE (CVPR, 2023)* | 0.776/0.763 |
| *Aesthetic Predictor (retrained on AVA_train)* | 0.721/0.723 |
| Q-Align (IAA) | 0.822/0.817 |
| **OneAlign** (IQA+IAA+VQA) | **0.823**/**0.819** |
## VQA Results (Spearman/Pearson)
| Datasets | LSVQ_test | LSVQ_1080p | KoNViD-1k | MaxWell_test |
| --- | --- | --- | --- | --- |
| *SimpleVQA (ACMMM, 2022)* | 0.867/0.861 | 0.764/0.803 | 0.840/0.834 | 0.720/0.715 |
| *FAST-VQA (ECCV 2022)* | 0.876/0.877 | 0.779/0.814 | 0.859/0.855 | 0.721/0.724 |
| Q-Align (VQA) | 0.883/0.882 | 0.797/0.830 | 0.865/0.877 | 0.780/0.782 |
| Q-Align (IQA+VQA) | 0.885/0.883 | 0.802/0.829 | 0.867/0.880 | **0.781**/**0.787** |
| **OneAlign** (IQA+IAA+VQA) | **0.886**/**0.886** | **0.803**/**0.837** | **0.876**/**0.888** | **0.781**/0.786| |