bge-large-en-v1.5-quant
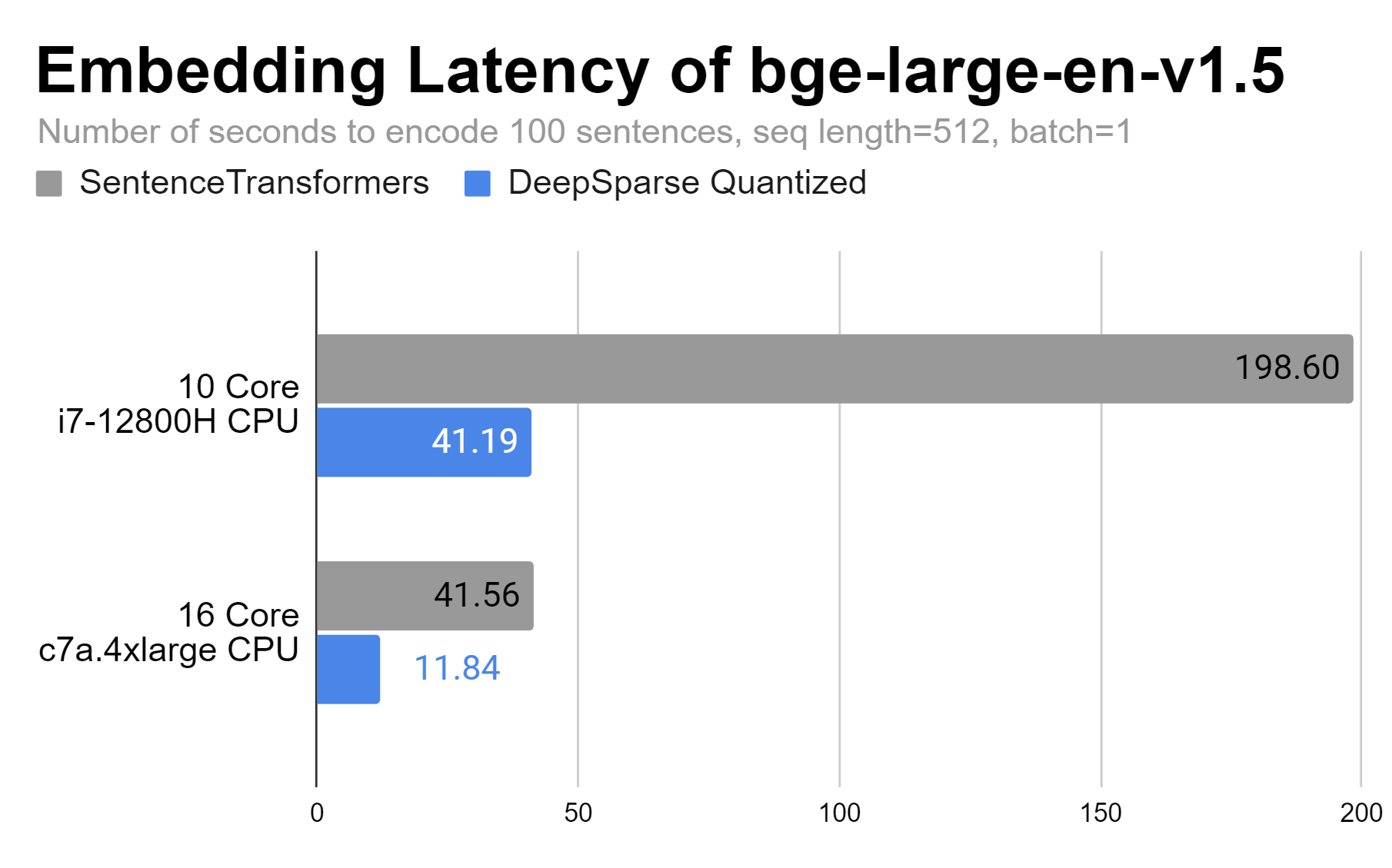
DeepSparse is able to improve latency performance on a 10 core laptop by 4.8X and up to 3.5X on a 16 core AWS instance.
Usage
This is the quantized (INT8) ONNX variant of the bge-large-en-v1.5 embeddings model accelerated with Sparsify for quantization and DeepSparseSentenceTransformers for inference.
pip install -U deepsparse-nightly[sentence_transformers]
from deepsparse.sentence_transformers import DeepSparseSentenceTransformer
model = DeepSparseSentenceTransformer('neuralmagic/bge-large-en-v1.5-quant', export=False)
# Our sentences we like to encode
sentences = ['This framework generates embeddings for each input sentence',
'Sentences are passed as a list of string.',
'The quick brown fox jumps over the lazy dog.']
# Sentences are encoded by calling model.encode()
embeddings = model.encode(sentences)
# Print the embeddings
for sentence, embedding in zip(sentences, embeddings):
print("Sentence:", sentence)
print("Embedding:", embedding.shape)
print("")
For general questions on these models and sparsification methods, reach out to the engineering team on our community Slack.
- Downloads last month
- 1,294
This model does not have enough activity to be deployed to Inference API (serverless) yet. Increase its social
visibility and check back later, or deploy to Inference Endpoints (dedicated)
instead.
Spaces using neuralmagic/bge-large-en-v1.5-quant 3
Evaluation results
- accuracy on MTEB AmazonCounterfactualClassification (en)test set self-reported75.537
- ap on MTEB AmazonCounterfactualClassification (en)test set self-reported38.306
- f1 on MTEB AmazonCounterfactualClassification (en)test set self-reported69.428
- cos_sim_pearson on MTEB BIOSSEStest set self-reported89.273
- cos_sim_spearman on MTEB BIOSSEStest set self-reported88.365
- euclidean_pearson on MTEB BIOSSEStest set self-reported86.831
- euclidean_spearman on MTEB BIOSSEStest set self-reported87.562
- manhattan_pearson on MTEB BIOSSEStest set self-reported86.593
- manhattan_spearman on MTEB BIOSSEStest set self-reported87.707
- cos_sim_pearson on MTEB SICK-Rtest set self-reported86.190